模块出处
ICLR 25 Submission\] [\[link\]](https://openreview.net/forum?id=BefqqrgdZ1) UltraLightUNet: Rethinking U-shaped Network with Multi-kernel Lightweight Convolutions for Medical Image Segmentation *** ** * ** *** ##### 模块名称 Grouped Attention Gate (GAG) *** ** * ** *** ##### 模块作用 轻量特征融合 *** ** * ** *** ##### 模块结构 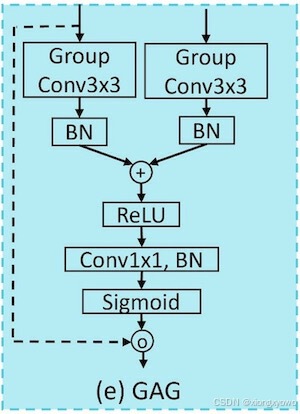 *** ** * ** *** ##### 模块特点 * 特征融合前使用Group Conv进行处理,比标准卷积更加轻量 * 将融合得到的粗特征视为Spatial Attention Map, 并与Encoder特征相乘,从而实现名字中"Gate"的效果 * 相较于特征融合模块,也可以视为一种利用辅助信息(Decoder)特征以增强Encoder特征的增强模块 *** ** * ** *** ##### 模块代码 ```python import torch import torch.nn as nn import torch.nn.functional as F class GAG(nn.Module): def __init__(self, F_g, F_l, F_int, kernel_size=1, groups=1): super(GAG,self).__init__() if kernel_size == 1: groups = 1 self.W_g = nn.Sequential( nn.Conv2d(F_g, F_int, kernel_size=kernel_size,stride=1,padding=kernel_size//2,groups=groups, bias=True), nn.BatchNorm2d(F_int) ) self.W_x = nn.Sequential( nn.Conv2d(F_l, F_int, kernel_size=kernel_size,stride=1,padding=kernel_size//2,groups=groups, bias=True), nn.BatchNorm2d(F_int) ) self.psi = nn.Sequential( nn.Conv2d(F_int, 1, kernel_size=1,stride=1,padding=0,bias=True), nn.BatchNorm2d(1), nn.Sigmoid() ) self.activation = nn.ReLU(inplace=True) def forward(self,g,x): g1 = self.W_g(g) x1 = self.W_x(x) psi = self.activation(g1+x1) psi = self.psi(psi) return x*psi if __name__ == '__main__': x1 = torch.randn([1, 64, 44, 44]) x2 = torch.randn([1, 64, 44, 44]) gag = GAG(F_g=64, F_l=64, F_int=64//2, kernel_size=3, groups=64//2) out = gag(x1, x2) print(out.shape) # [1, 64, 44, 44] ``` *** ** * ** ***