在上一篇文章中,我介绍了 Sdcb Chats 如何集成 DeepSeek-R1 模型,并利用其思维链(Chain of Thought, CoT)功能增强 AI 推理的透明度。DeepSeek-R1 强大的思维链能力给用户留下了深刻印象。本文将深入剖析 Sdcb Chats 实现这一功能的技术细节,重点介绍如何基于 OpenAI .NET SDK 解析 DeepSeek-R1 返回的思维链数据。
Chats的思维链演示:
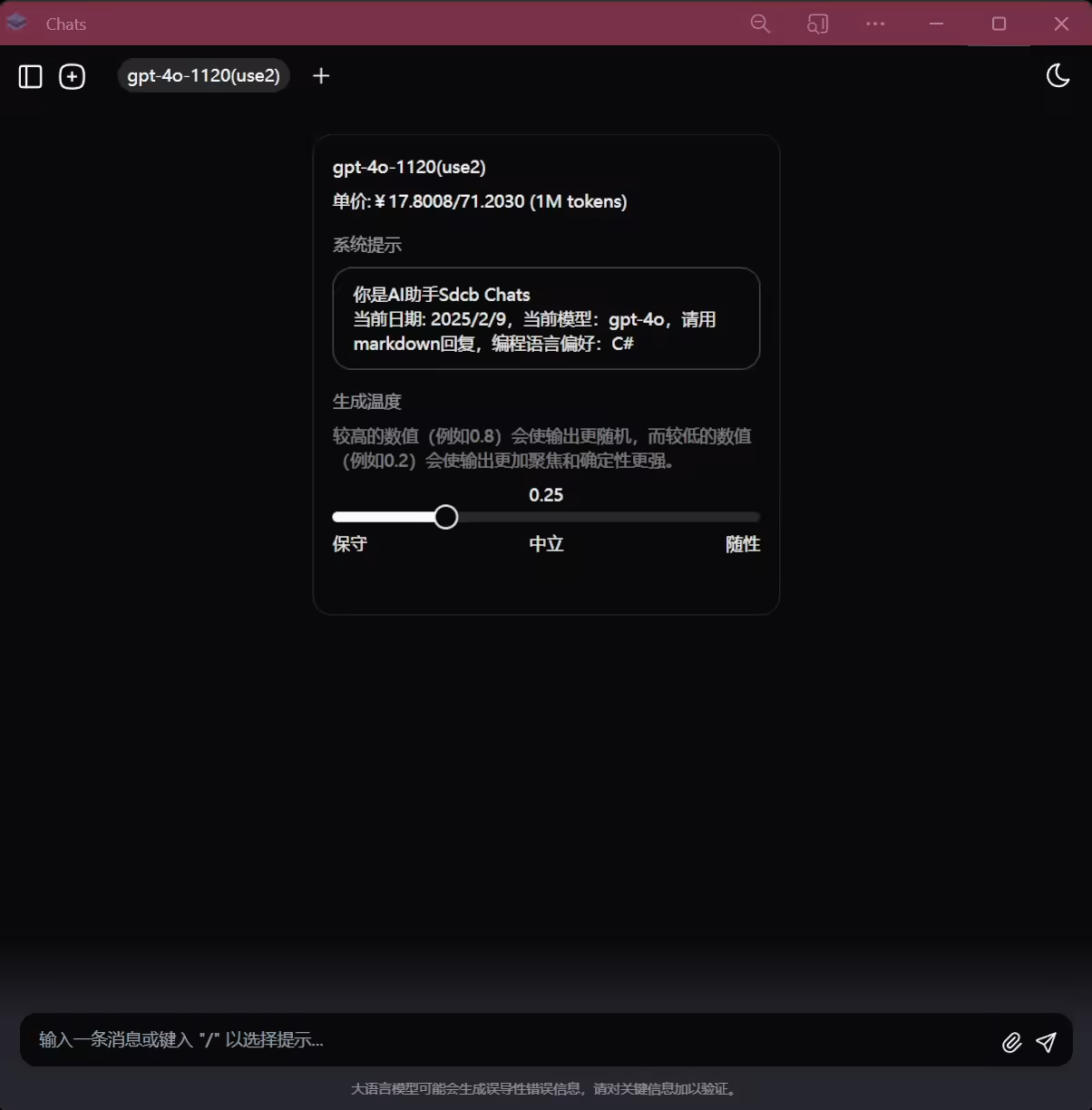
DeepSeek-R1 有两种思维链格式:
-
官方预处理型 :通过
reasoning_content
字段(与content
同级)显示思维链。官网和硅基流动(SiliconFlow)采用此方式。示例:json{ "id": "0194e3241b719f91d183976240e08067", "object": "chat.completion.chunk", "created": 1738977581, "model": "Pro/deepseek-ai/DeepSeek-R1", "choices": [ { "index": 0, "delta": { "content": null, "reasoning_content": "嗯", "role": "assistant" }, "finish_reason": null, "content_filter_results": { "hate": { "filtered": false }, "self_harm": { "filtered": false }, "sexual": { "filtered": false }, "violence": { "filtered": false } } } ], "system_fingerprint": "", "usage": { "prompt_tokens": 17, "completion_tokens": 1, "total_tokens": 18 } }
-
开源常见型 :通过
content
中的<think>
标签展示思维链。Gitee AI、NVIDIA NIM、GitHub Models、Azure AI、Azure AI Foundry 等采用此方式。示例:html<think> 好的,用户说......(这一部分是思维链) </think> 总的来说......(这一部分是响应)
然而,OpenAI SDK 在 API 支持方面进展缓慢,这给思维链的支持带来了一些挑战。作为 OpenAI .NET SDK 的主要使用者,我面临以下三个主要挑战:
-
max_tokens
参数不一致 :OpenAI 官方已将max_tokens
替换为max_response_token
,但许多国产模型仍使用max_tokens
。这导致在适配不同模型时需要额外处理,增加了开发成本和复杂度。 -
缺乏原生思维链支持:如前所述,OpenAI .NET SDK 并未提供对思维链返回的原生支持。这意味着我们需要手动解析响应内容,提取思维链信息。
-
工具功能不完善:OpenAI .NET SDK 同样不支持诸如互联网搜索之类的工具功能,限制了某些模型的应用能力。
尽管存在这些问题,我仍然选择使用 OpenAI .NET SDK,主要基于以下考虑:
- 高质量与官方维护:OpenAI .NET SDK 代码质量较高,且由官方团队维护,提供了更好的稳定性和技术支持。
- 广泛兼容性:许多模型提供商优先支持 OpenAI 兼容的 API。使用 OpenAI .NET SDK 可以更好地兼容不同模型,避免为每个模型单独编写代码。
- 性能优势:下文将详细阐述。
- AOT 支持:最新 2.2.0-beta1 版本的 OpenAI .NET SDK 支持 AOT (Ahead-of-Time) 编译。AOT 编译能显著提升性能和安全性,熟悉 .NET 的朋友都知道,AOT 编译在一定程度上是不支持反射的,所以对 JSON 序列化、反序列化等操作需要特殊处理。
综上,OpenAI .NET SDK 的优点大于缺点。选择它意味着在享受高质量和广泛兼容性的同时,可以通过一些技巧和变通来解决思维链解析等问题,从而实现更强大的 AI 应用。
下面我将分别介绍如何利用 OpenAI .NET SDK 处理 DeepSeek-R1 的两种思维链格式。
官方预处理型 - reasoning_content
这种格式的处理相对简单。OpenAI .NET SDK 的对象序列化和反序列化并未使用 Newtonsoft.JSON 或 System.Text.Json 的 JsonSerializer,我认为这是为了提高性能,因为字段都是预定义的------避免了反射。
要解析 SSE 输出,可以这样编写代码(示例):
csharp
// 解析 SSE 输出示例
StreamingChatCompletionUpdate delta = ModelReaderWriter.Read<StreamingChatCompletionUpdate>(BinaryData.FromString("""
{"id":"0194e3241b719f91d183976240e08067","object":"chat.completion.chunk","created":1738977581,"model":"Pro/deepseek-ai/DeepSeek-R1","choices":[{"index":0,"delta":{"content":null,"reasoning_content":"嗯","role":"assistant"},"finish_reason":null,"content_filter_results":{"hate":{"filtered":false},"self_harm":{"filtered":false},"sexual":{"filtered":false},"violence":{"filtered":false}}}],"system_fingerprint":"","usage":{"prompt_tokens":17,"completion_tokens":1,"total_tokens":18}}
"""));
输出的 StreamingChatCompletionUpdate
对象结构如下(使用 LINQPad 的 Dump 方法展示):
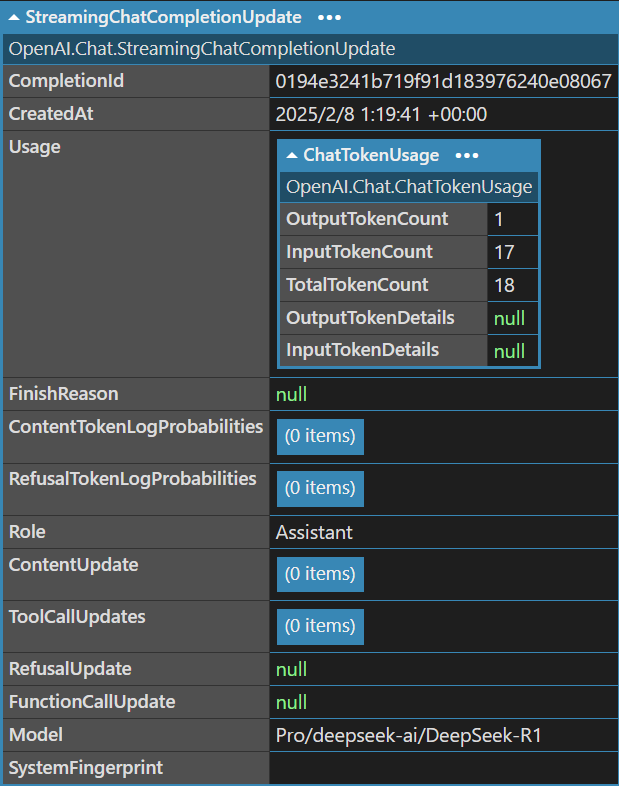
可以看到,content_filter_results
和 reasoning_content
中的"嗯"等信息并未直接显示。
要获取这些信息,可以使用反射。通过 LINQPad 的 .Uncapsulate()
方法(一个自定义扩展方法,用于显示对象的内部结构),可以查看对象的完整结构:
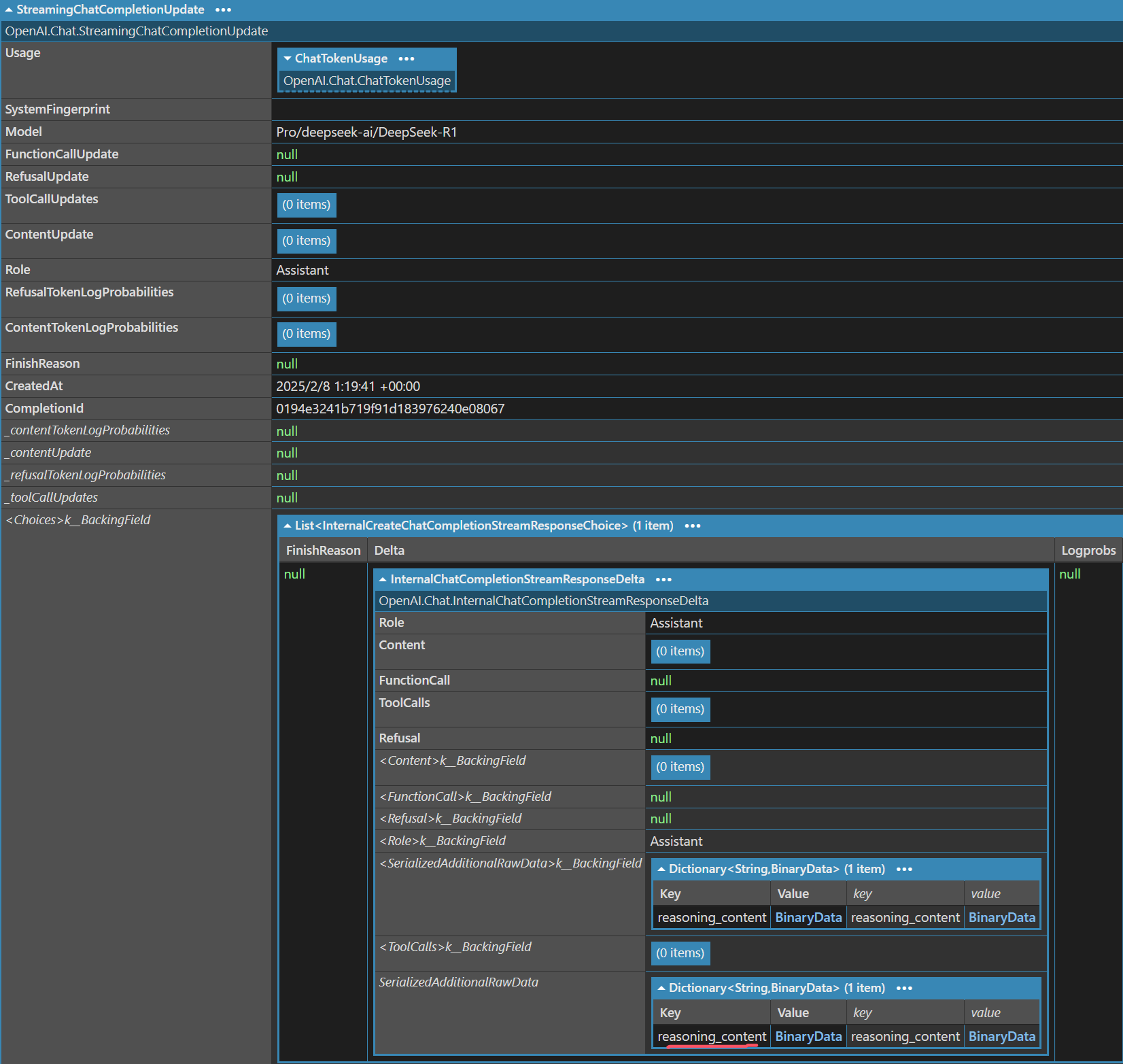
其中,Choices[0].Delta.SerializedAdditionalRawData
-> reasoning_content
就是我们需要的数据。通过反射即可获取其值:
以下是相关代码(源码链接):
csharp
/// <summary>
/// 创建一个从 StreamingChatCompletionUpdate 对象中提取 reasoning_content 的委托。
/// 如果未找到或无法解析,则返回 null。
/// </summary>
/// <returns>Func<StreamingChatCompletionUpdate, string?></returns>
public static Func<StreamingChatCompletionUpdate, string?> CreateStreamingReasoningContentAccessor()
{
// 1. 获取 StreamingChatCompletionUpdate 类型
Type streamingChatType = typeof(StreamingChatCompletionUpdate);
// 2. 获取 internal 属性 "Choices"
// 类型:IReadOnlyList<InternalCreateChatCompletionStreamResponseChoice>
PropertyInfo? choicesProp = streamingChatType.GetProperty(
"Choices",
BindingFlags.Instance | BindingFlags.NonPublic | BindingFlags.Public
) ?? throw new InvalidOperationException("Unable to reflect property 'Choices' in StreamingChatCompletionUpdate.");
// 3. 获取 Choices 的泛型参数 T = InternalCreateChatCompletionStreamResponseChoice
Type? choicesPropType = choicesProp.PropertyType ?? throw new InvalidOperationException("Unable to determine the property type of 'Choices'."); // IReadOnlyList<T>
if (!choicesPropType.IsGenericType || choicesPropType.GetGenericArguments().Length != 1)
{
throw new InvalidOperationException("Property 'Choices' is not the expected generic type IReadOnlyList<T>.");
}
// 取得 T
Type choiceType = choicesPropType.GetGenericArguments()[0];
// 4. 从 choiceType 中获取 internal 属性 "Delta"
PropertyInfo? deltaProp = choiceType.GetProperty(
"Delta",
BindingFlags.Instance | BindingFlags.NonPublic | BindingFlags.Public
) ?? throw new InvalidOperationException("Unable to reflect property 'Delta' in choice type.");
// 5. 获取 Delta 对象的类型,然后从中获取 "SerializedAdditionalRawData"
Type deltaType = deltaProp.PropertyType;
PropertyInfo? rawDataProp = deltaType.GetProperty(
"SerializedAdditionalRawData",
BindingFlags.Instance | BindingFlags.NonPublic | BindingFlags.Public
) ?? throw new InvalidOperationException("Unable to reflect property 'SerializedAdditionalRawData' in delta type.");
// ---
// 创建并返回委托,在委托中使用上述缓存的 PropertyInfo
// ---
return streamingChatObj =>
{
if (streamingChatObj == null)
{
return null;
}
// 拿到 choices 数据
object? choicesObj = choicesProp.GetValue(streamingChatObj);
if (choicesObj is not IEnumerable choicesEnumerable)
{
return null;
}
foreach (object? choice in choicesEnumerable)
{
if (choice == null) continue;
// 获取 Delta 对象
object? deltaObj = deltaProp.GetValue(choice);
if (deltaObj == null) continue;
// 获取字典 SerializedAdditionalRawData
object? rawDataValue = rawDataProp.GetValue(deltaObj);
if (rawDataValue is not Dictionary<string, BinaryData> dict) continue;
// 从字典里查找 "reasoning_content"
if (dict.TryGetValue("reasoning_content", out BinaryData? binaryData))
{
return binaryData.ToObjectFromJson<string>();
}
}
// 如果所有 Choice 中都没有找到则返回 null
return null;
};
}
这段代码返回一个 Func<StreamingChatCompletionUpdate, string?>
委托,而不是直接返回 reasoning_content
。这样做的好处是,可以在需要时才提取 reasoning_content
,避免不必要的性能开销。
在使用时(源码链接):
csharp
// 缓存委托,避免重复反射
static Func<StreamingChatCompletionUpdate, string?> StreamingReasoningContentAccessor { get; } = ReasoningContentFactory.CreateStreamingReasoningContentAccessor();
这样可以确保反射代码只执行一次,避免每次调用都进行反射,从而提升性能。实际使用中,只需调用 StreamingReasoningContentAccessor(delta)
即可获取 reasoning_content
的值(如果不存在则返回 null
)。
这种方法虽然使用了反射,但由于只在程序启动时执行一次,对整体性能影响很小。同时,它避免了修改 OpenAI .NET SDK 源代码,保持了代码的整洁性和可维护性。
此外,将反射逻辑封装在 ReasoningContentFactory
中,实现了代码解耦。如果 OpenAI .NET SDK 内部结构发生变化,只需修改 ReasoningContentFactory
中的代码,无需改动其他部分。
总之,这种方法既解决了 OpenAI .NET SDK 缺少 reasoning_content
支持的问题,又保证了代码的性能和可维护性,是一种优雅的解决方案。
开源常见型 - <think>
标签
开源常见型思维链的解析较为复杂,因为信息嵌入在 <think>
标签中,需要进行字符串解析。下面我将分享如何使用 C# 实现流式解析,并处理内容。
通常,段落内容格式如下:
html
<think>
好的,用户说......(这一部分是思维链)
</think>
总的来说......(这一部分是响应)
为了提取 <think>
标签中的思维链,常见的思路是使用正则表达式。但对于流式返回的数据,正则表达式并不可取。正则表达式依赖于完整的输入,必须等待所有数据接收完毕才能匹配,这在高延迟或大数据量场景下会严重影响用户体验。我们需要一种增量解析方案,即每接收到新的数据片段,就立即解析,而不是等待所有数据。
因此,我没有选择正则表达式,而是编写了一个简单的状态机解析器来处理流式文本。解析器会不断读取输入流,根据当前状态判断是否进入或退出 <think>
标签,并提取标签内的内容。
状态机解析器的实现颇具挑战性,最终我借助了 Sdcb Chats 来完成代码编写(用自己的开源项目提高生产力,这很合理)。
但即使是 AI,也需要清晰的需求定义。我设计了以下测试用例,分别对应不同的测试场景:
测试用例 1 - 增量解析 <think>
标签与内容
输入 | 输出 | 说明 |
---|---|---|
\n |
无输出 | 空白行不产生输出 |
<thi |
无输出 | 尚未识别为完整的 <think> 标签 |
nk> t |
think: " t" |
进入 <think> 模式,输出标签内内容 |
est |
think: " est" |
继续输出 <think> 标签内的内容 |
<think> |
think: "<think>" |
进入 <think> 模式,输出标签内内容 |
</think> |
think: "</think>" |
识别为 <think> 模式结束,但无换行符不退出模式 |
\ntest |
think: "\ntest" |
输出 <think> 标签内的内容 |
\n |
无输出 | 空白行不产生输出 |
</think> |
无输出 | 完全匹配 \n</think> 后退出 <think> 模式 |
<think> |
response: "<think>" |
进入响应模式,输出内容 |
</think> |
response: "</think>" |
继续输出响应模式内容 |
blabla |
response: "blabla" |
继续输出响应模式内容 |
测试用例 2 - 直接进入响应模式的处理
输入 | 输出 | 说明 |
---|---|---|
\n |
无输出 | 空白行不产生输出 |
</think> |
response: "</think>" |
未进入 <think> 模式,直接输出响应模式内容 |
测试用例 3 - 响应模式下的 <think>
标签解析
输入 | 输出 | 说明 |
---|---|---|
\nwww |
response: "\nwww" |
输出响应模式内容 |
<think> |
response: "<think>" |
已在响应模式,继续输出内容 |
测试用例 4 - 单次输入下的 <think>
标签与响应内容解析(适用于非 stream 模式)
输入 | 输出 | 说明 |
---|---|---|
<think>test\n</think>\nresp |
think: "think content" |
输出 <think> 标签内的内容 |
response: "this is response" |
输出 <think> 标签后的响应内容 |
这些表格展示了不同输入情况下的输出结果,有助于理解如何通过状态机解析器处理流式文本中的 <think>
标签和响应内容。
解析器接口定义如下:
csharp
// 定义 Think/Response 数据结构,两者可以同时存在(但不会同时不存在)
public record ThinkAndResponseSegment
{
public string? Think { get; init; }
public string? Response { get; init; }
}
public static class ThinkTagParser
{
// 流式输入、流式输出
public static async IAsyncEnumerable<ThinkAndResponseSegment> Parse(
IAsyncEnumerable<string> tokenYielder,
[EnumeratorCancellation] CancellationToken cancellationToken = default)
{
// todo: 状态机实现
}
}
这段代码的难点在于其内部状态管理,包括:
- 等待
<think>
模式 - 进入
<think>
解析模式 - 等待离开
<think>
模式 - 进入响应模式
在上述提示下,AI 成功实现了代码(我使用了 o3-mini 模型),并且是一次性成功。
具体实现请参考源码。
总结与展望
本文详细介绍了 Sdcb Chats 如何解析 DeepSeek-R1 的思维链,并分享了在使用 OpenAI .NET SDK 时遇到的挑战和解决方案。尽管 OpenAI .NET SDK 在思维链支持方面存在局限,但通过一些技巧和变通,我们仍能有效解析和处理 DeepSeek-R1 的思维链。
针对 DeepSeek-R1 的两种思维链格式(官方预处理型和开源常见型),我们分别采用了反射和流式文本解析器。反射方法虽然使用了反射,但通过缓存机制将性能影响降至最低,同时保持了代码的整洁性和可维护性。流式文本解析器则避免了正则表达式的局限性,能在高延迟或大数据量场景下提供良好的用户体验。
未来,Sdcb Chats 将继续优化思维链解析性能,并探索更多 AI 模型集成和应用场景。
如果您对这些技术细节感兴趣,或希望支持本项目,请访问 Sdcb Chats 的 GitHub 仓库 并点赞(Star)。您的支持将是项目持续改进和完善的动力!