说在前面
本节介绍检索增强生成(Retrieval Augmented Generation,RAG)的最后一个阶段Output。
Main Content
在Output阶段,我们会将检索(Retrieval)阶段获得的chunk,连同用户的问题一起喂给LLM,最后由LLM返回最后的答案。
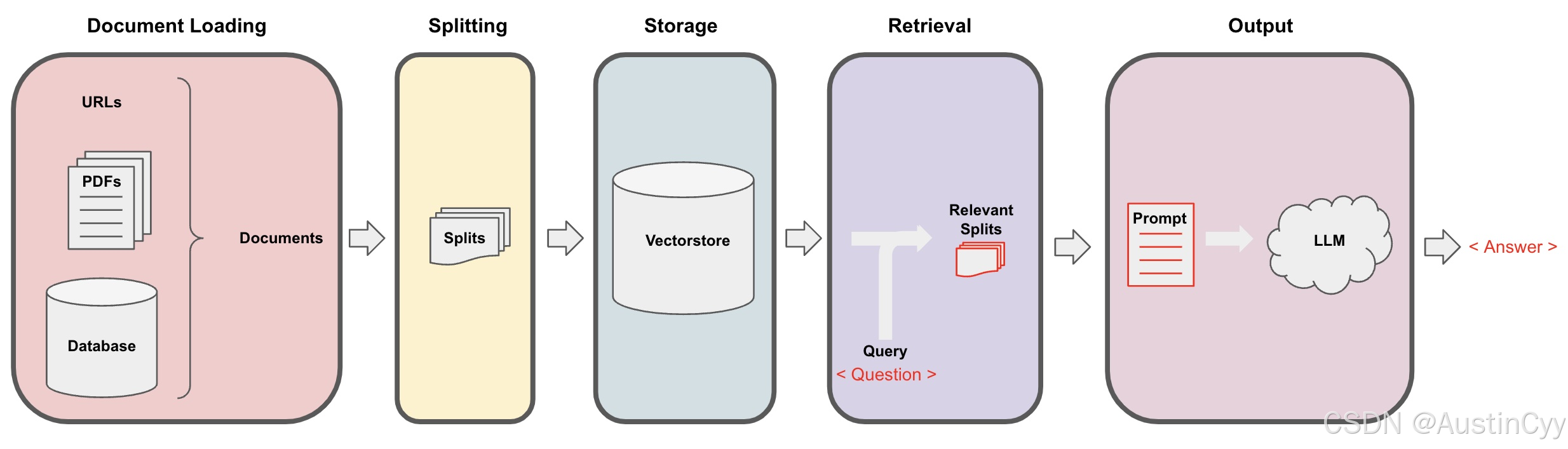
前置工作
1.配置环境变量。
python
import os
import openai
import sys
sys.path.append('../..')
from dotenv import load_dotenv, find_dotenv
_ = load_dotenv(find_dotenv()) # read local .env file
openai.api_key = os.environ['OPENAI_API_KEY']
2.检查时间更新llm版本。
python
import datetime
current_date = datetime.datetime.now().date()
if current_date < datetime.date(2023, 9, 2):
llm_name = "gpt-3.5-turbo-0301"
else:
llm_name = "gpt-3.5-turbo"
print(llm_name)
RetrievalQA chain
1.加载向量数据库、设置用户问题、创建llm。
python
from langchain.vectorstores import Chroma
from langchain.embeddings.openai import OpenAIEmbeddings
persist_directory = 'docs/chroma/'
embedding = OpenAIEmbeddings()
vectordb = Chroma(persist_directory=persist_directory, embedding_function=embedding)
question = "What are major topics for this class?"
docs = vectordb.similarity_search(question,k=3)
len(docs)
from langchain.chat_models import ChatOpenAI
llm = ChatOpenAI(model_name=llm_name, temperature=0)
2.使用RetrievalQA和LLM创建一个问答链。
python
from langchain.chains import RetrievalQA
qa_chain = RetrievalQA.from_chain_type(
llm,
retriever=vectordb.as_retriever()
)
3.使用问答链处理一个查询问题,并返回结果。
python
result = qa_chain({"query": question})
result["result"]

Prompt
为了让RetrievalQA给出格式化答案,我们创建一个prompt,告诉LLM应该输出什么样的答案。
python
from langchain.prompts import PromptTemplate
# Build prompt
template = """Use the following pieces of context to answer the question at the end. If you don't know the answer, just say that you don't know, don't try to make up an answer. Use three sentences maximum. Keep the answer as concise as possible. Always say "thanks for asking!" at the end of the answer.
{context}
Question: {question}
Helpful Answer:"""
QA_CHAIN_PROMPT = PromptTemplate.from_template(template)
# Run chain
qa_chain = RetrievalQA.from_chain_type(
llm,
retriever=vectordb.as_retriever(),
return_source_documents=True,
chain_type_kwargs={"prompt": QA_CHAIN_PROMPT}
)
-
return_source_documents=True
:这个参数指示RetrievalQA
实例在返回结果时,除了答案外,还应该返回源文档。 -
chain_type_kwargs
:一个字典,包含了传递给特定链类型的额外参数。 -
prompt
: QA_CHAIN_PROMPT:在这个字典中,prompt
键指定了用于问答链的提示模板。QA_CHAIN_PROMPT 包含了用于生成答案的提示文本。
python
question = "Is probability a class topic?"
result = qa_chain({"query": question})
result["result"]
result["source_documents"][0]
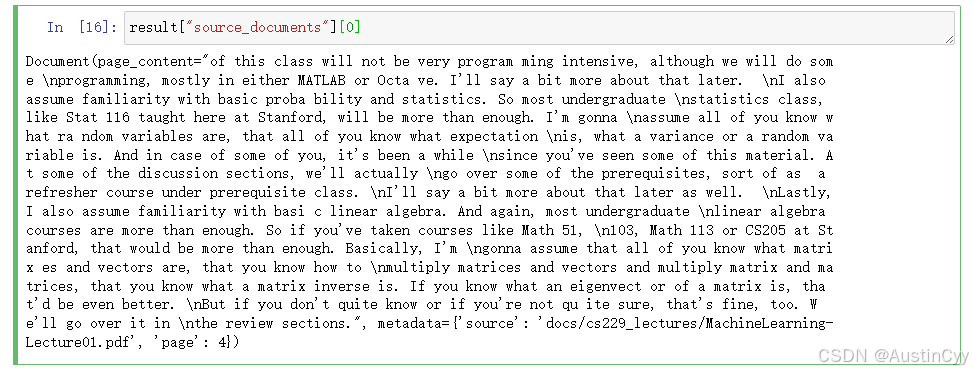
qa_chain 根据 prompt template 给出简洁的答案,并且按照prompt template的要求在末尾加了结束语 "Thanks for asking!"。
RetrievalQA chain types
RetrievalQA的chain_type参数默认为stuff,还有 map_reduce
, refine
和 map_rerank
三种模式可选。
python
qa_chain_mr = RetrievalQA.from_chain_type(
llm,
retriever=vectordb.as_retriever(),
chain_type="map_reduce"
)
python
result = qa_chain_mr({"query": question})
python
result["result"]

RetrievalQA limitations
qa_chain的缺点是不能保存对话的历史记录。
1.创建一个QA链。
python
qa_chain = RetrievalQA.from_chain_type(
llm,
retriever=vectordb.as_retriever()
)
2.首先提问"Is probability a class topic?",qa_chain给出回答。
python
question = "Is probability a class topic?"
result = qa_chain({"query": question})
result["result"]
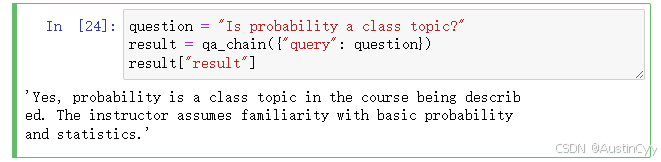
3.然后接着追问"why are those prerequesites needed?",qa_chain给出与'probability'毫不相干的回答,可见qa_chain对之前的对话完全没有记忆。
python
question = "why are those prerequesites needed?"
result = qa_chain({"query": question})
result["result"]
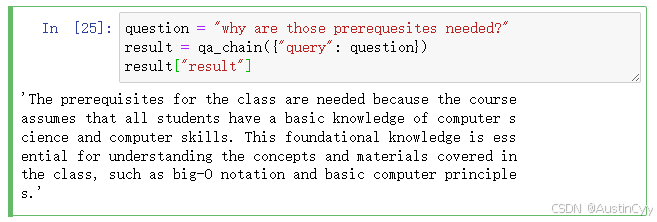
总结
本节简单阐述了有关LLM 的 Question Answering 部分,使用了 RetrievalQA 库来创建问答链,并且通过设定 Prompt 来规范LLM 的输出。同时我们知道 QA 链的局限性为其没有记忆上下文的能力。