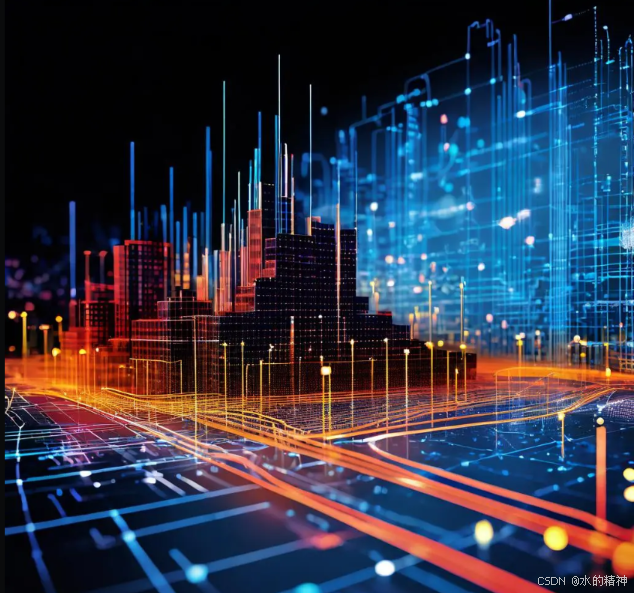
一、什么是相关性模型?
相关性模型主要关注的是query和doc的相关性。例如给定query,和1000个doc,找到哪个doc是好query最相关的。
二、为什么需要相关性模型?
熟悉es的应该都熟悉BM25相关性算法。它是一个很简单的相关性算法。我们实际使用中,经常会遇到一个问题就是如何。搜的内容和我给定的query不相关。换句话说,返回的结果不包含先要的结果。
相关性是一个复杂的问题。不是简单的Bm25算法。我们需要相关性模型来更好的做这件事。
三、相关性模型
交叉BERT模型,准确性好,推理代价大,更适合用在粗排或者精排阶段。
双塔BERT模型,不够准确,但是推理代价小。适合用来召回阶段。
3.1 交叉BERT模型
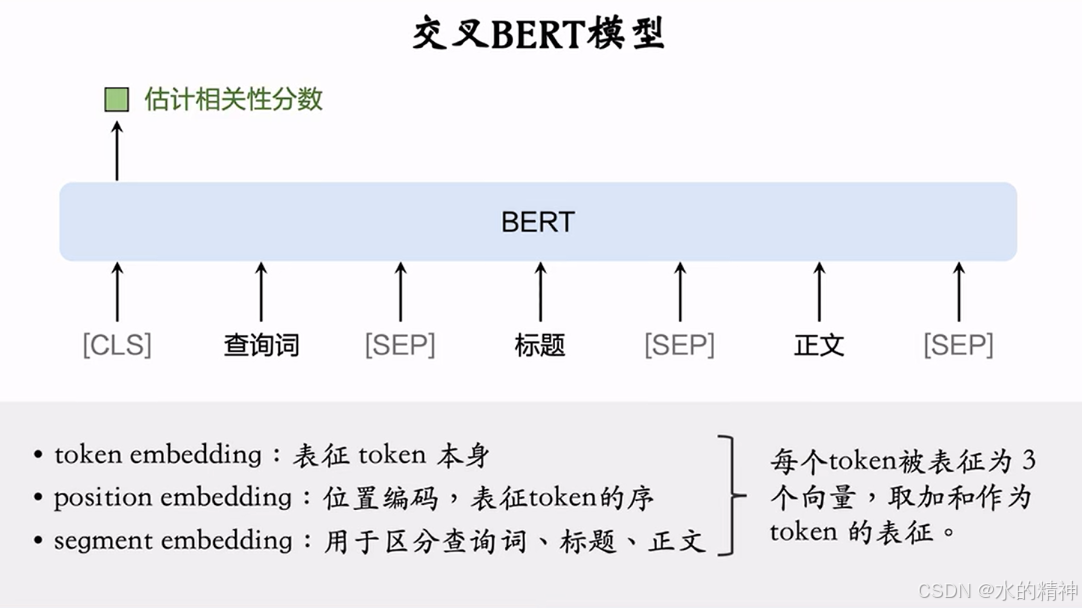
分词:字粒度与词粒度
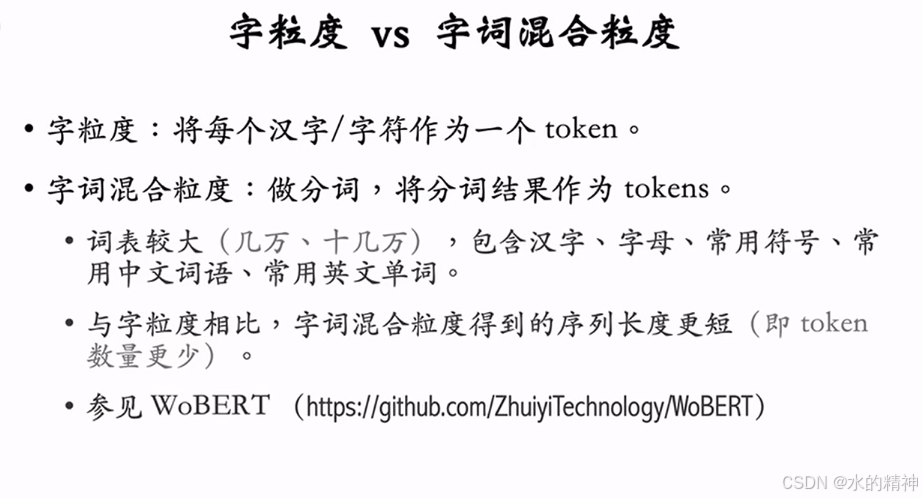
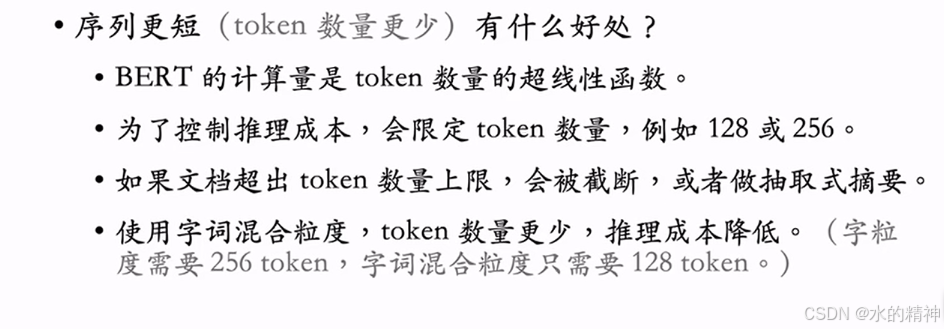
如何减低交叉BERT模型的推理成本
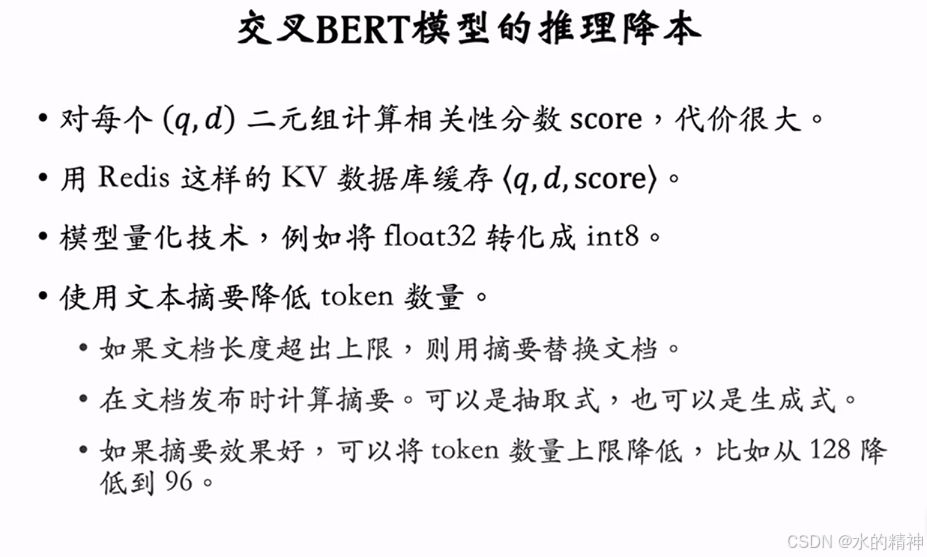
3.2 双塔BERT模型
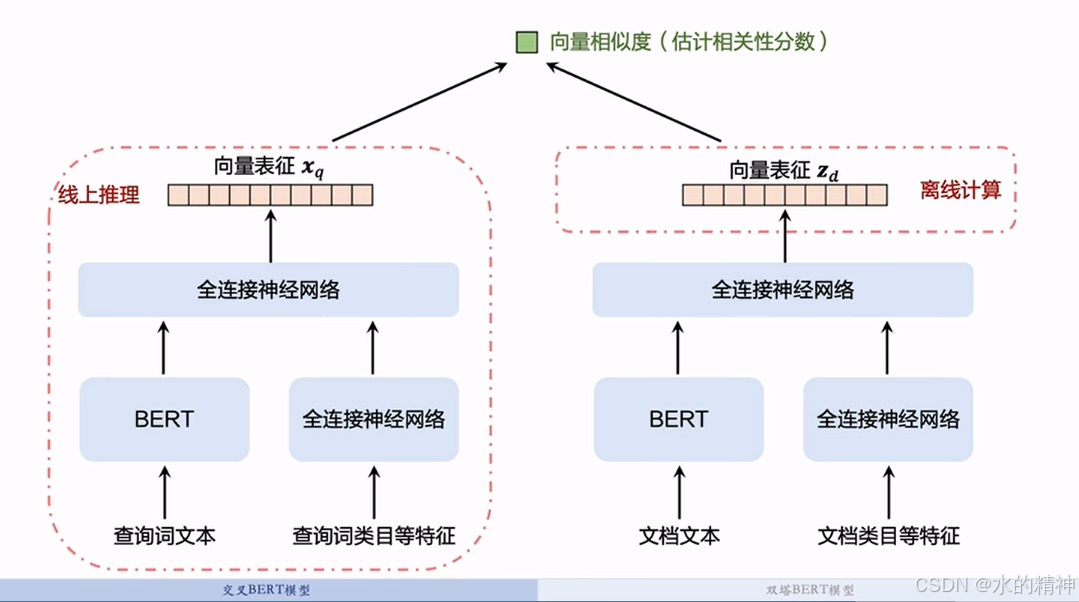
四、训练相关性模型
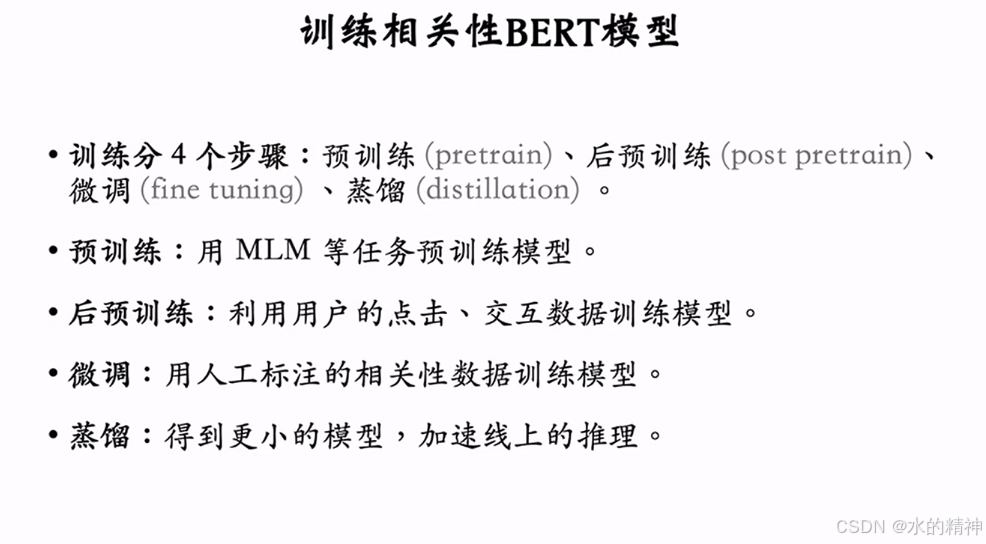
4.1 微调
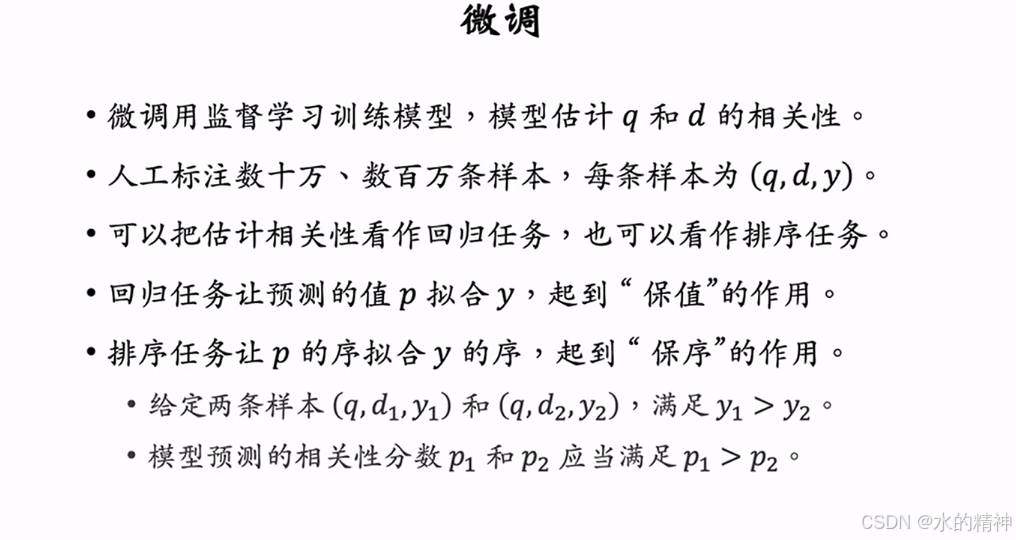
回归任务
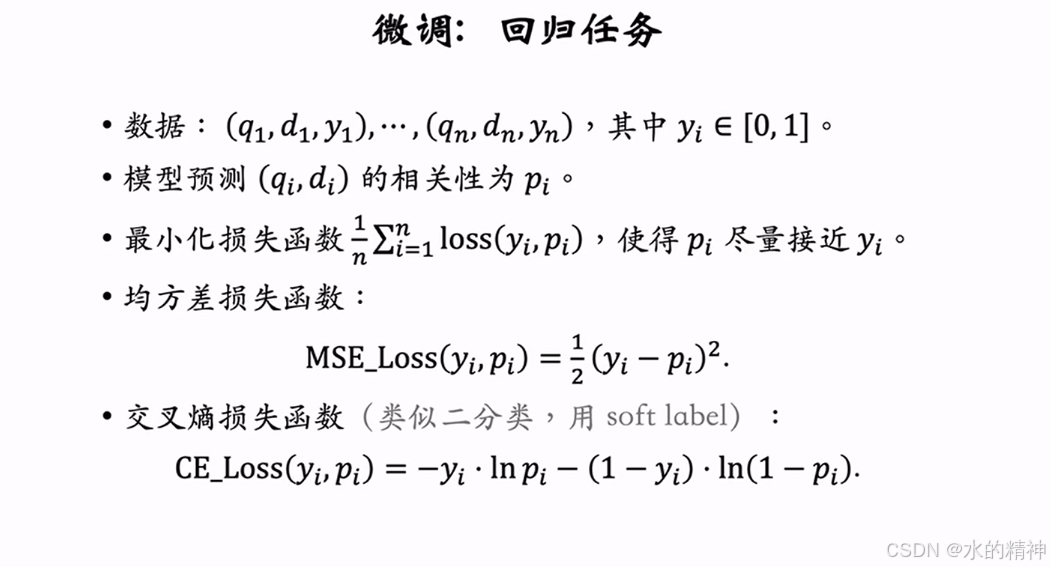
排序任务
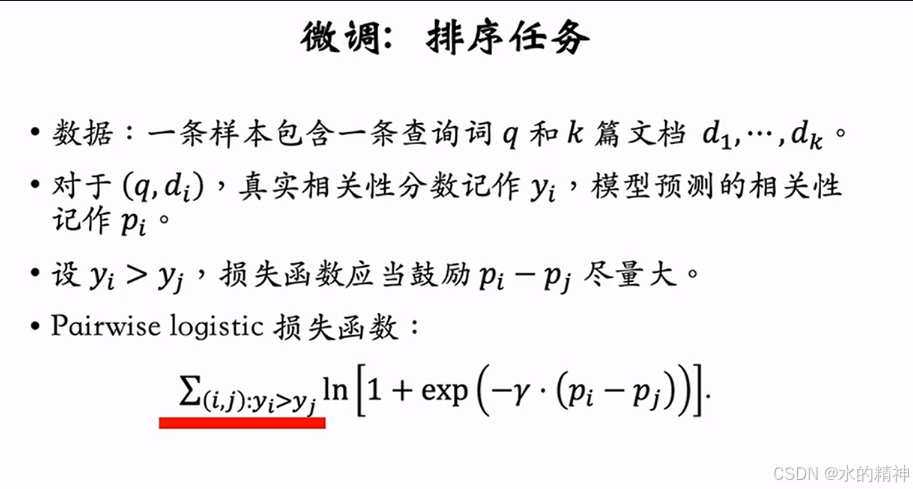
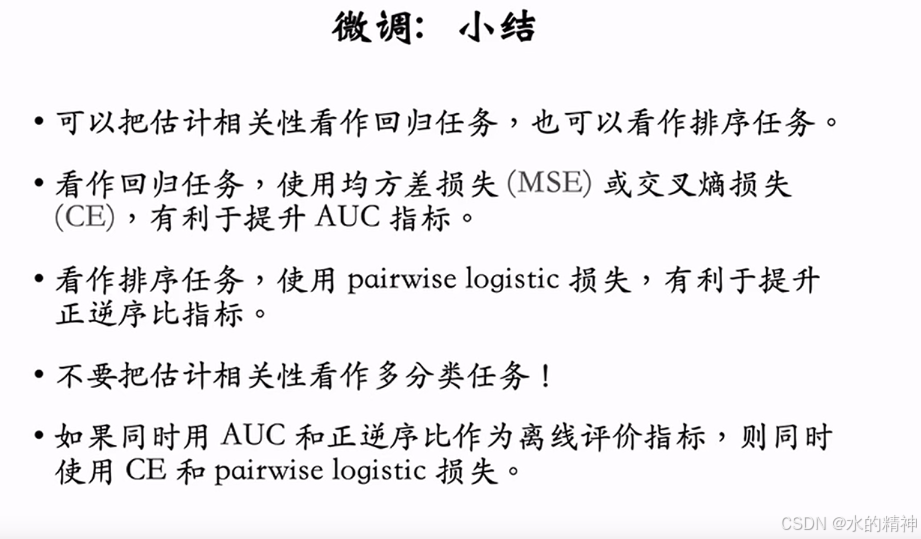
4.2 后预训练
从事发后,从以发生的事中,去再提升当前的能力。通过用户的点击行为来有优化模型。
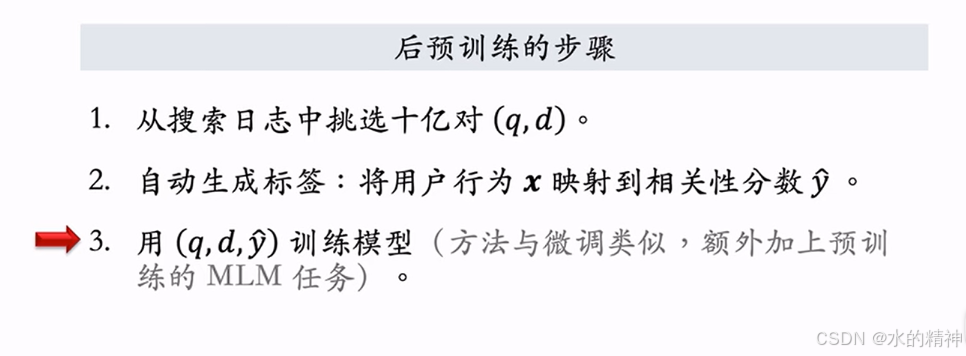
4.2.1 数据筛选
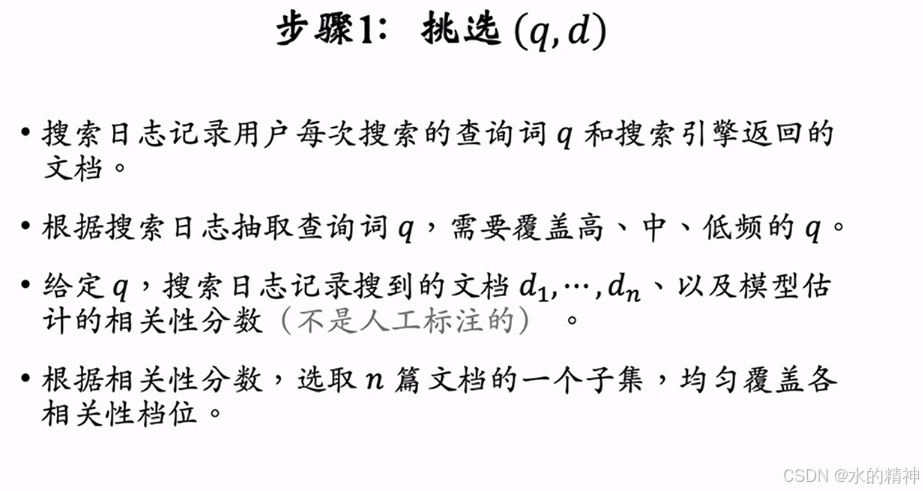
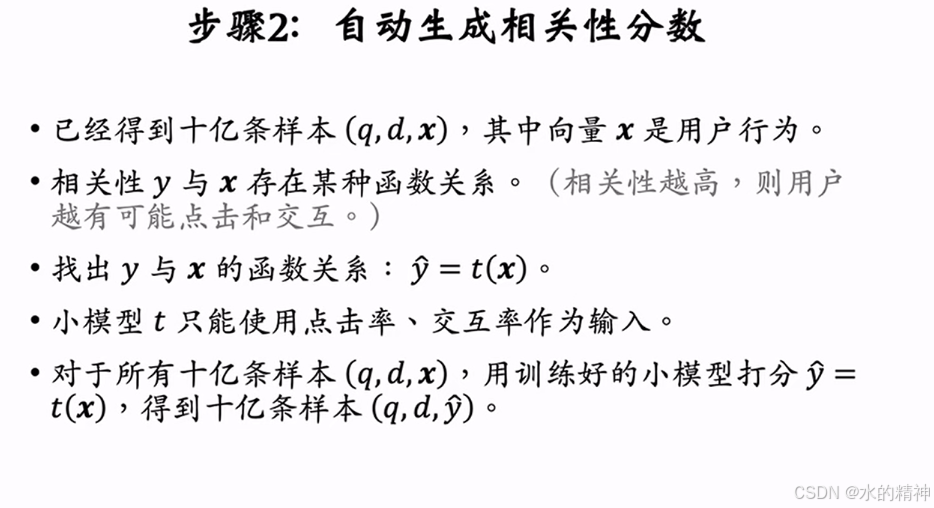
4.2.2 后训练,构造训练数据
挑选用户的点击数据。得到query,topk的doc,以及用户的点击行为x。然后抽样几万条数据,去人工标注,标注得到相关性y,然后用这几万条数据去训练一个小模型来计算点击行为x和相关性y的关系。然后再用这个小模型去预测10亿条数据的相关性分数。
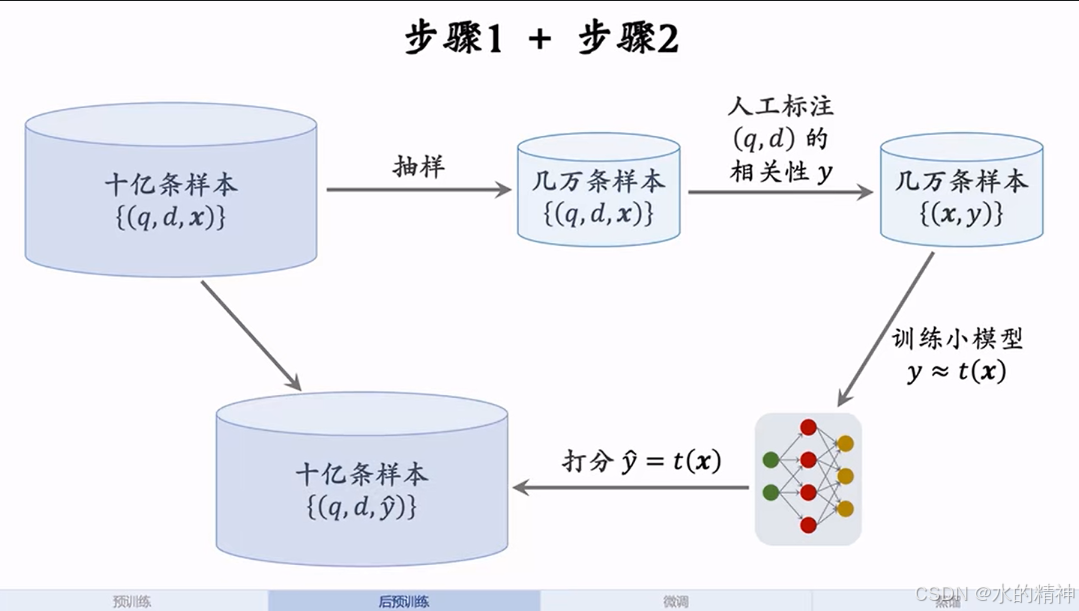
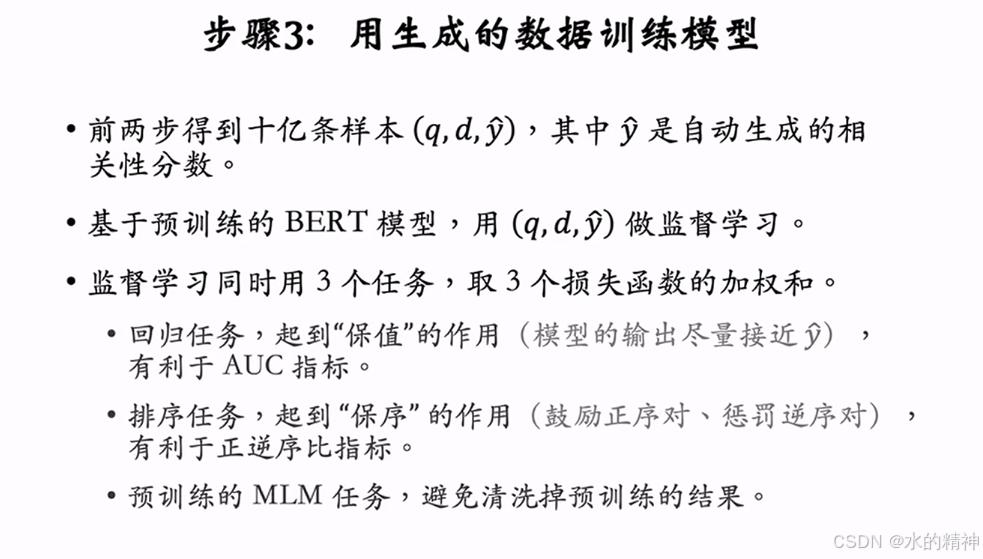
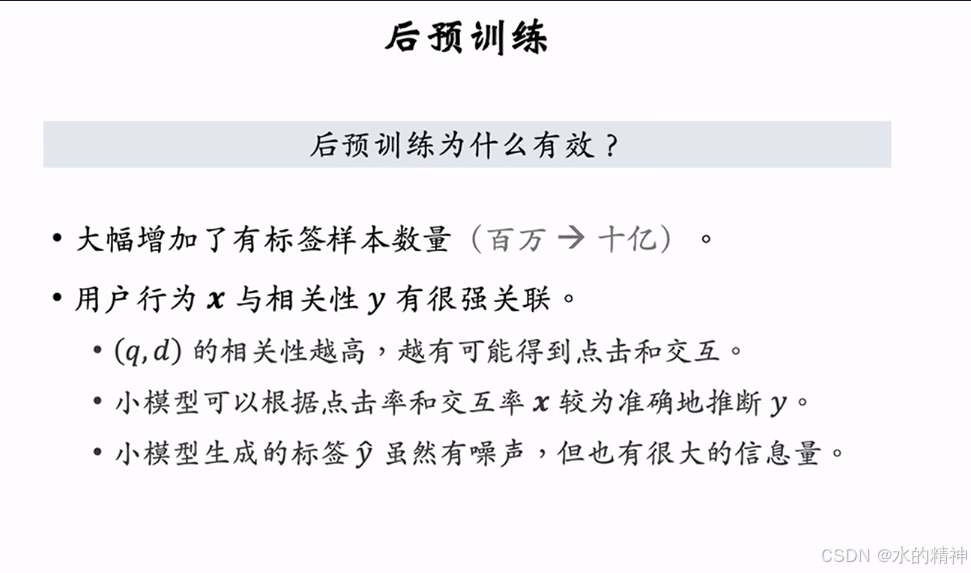
4.3 蒸馏
蒸馏是把一个大模型变成一个小模型。为什么要蒸馏,模型越大,推理成本越大,时间成本也越大,当然也越准确。
4.3.1 为什么要蒸馏?
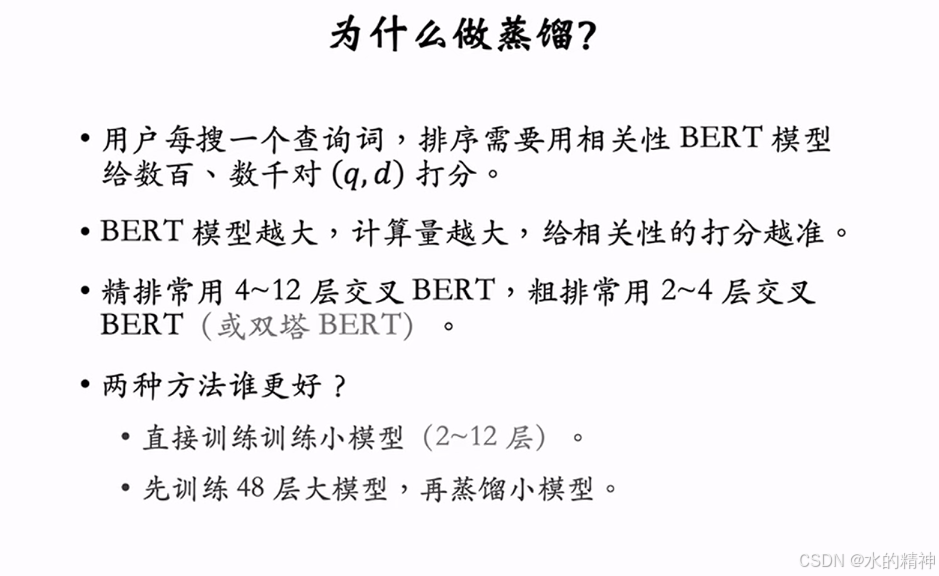
用大模型,蒸馏得到小模型,最终的效果比直接训练一个小模型得到的效果更好。
4.3.2 如何做蒸馏
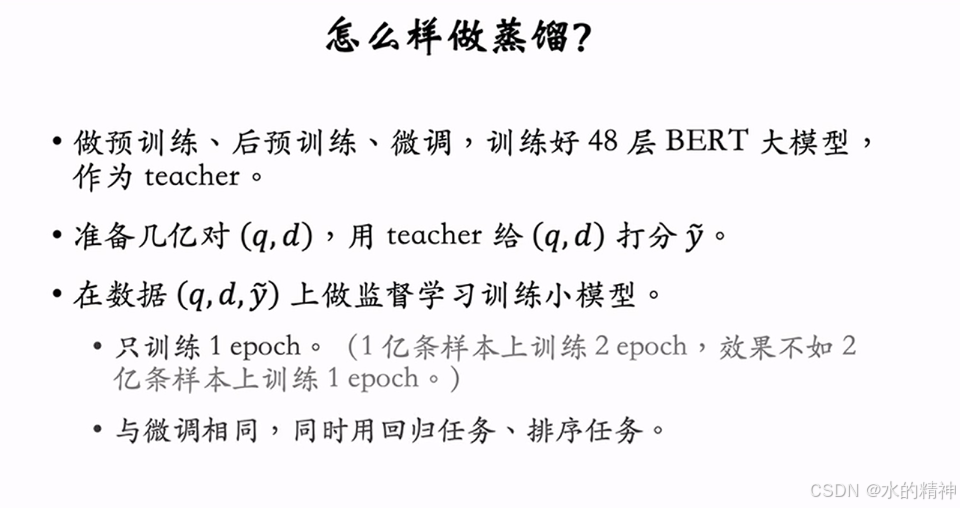
蒸馏的技巧
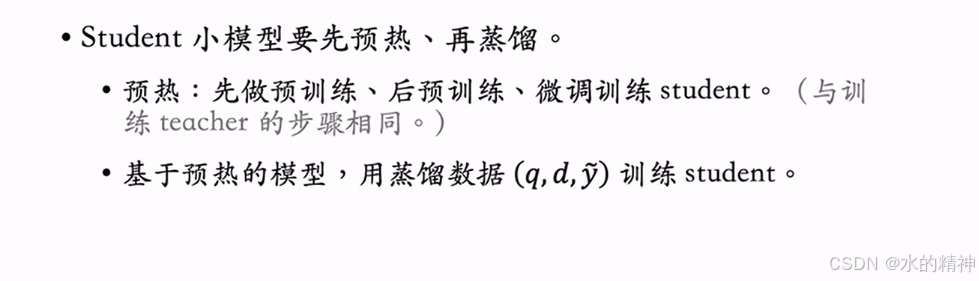
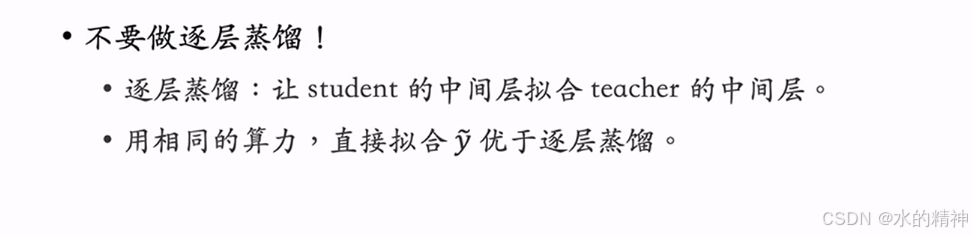
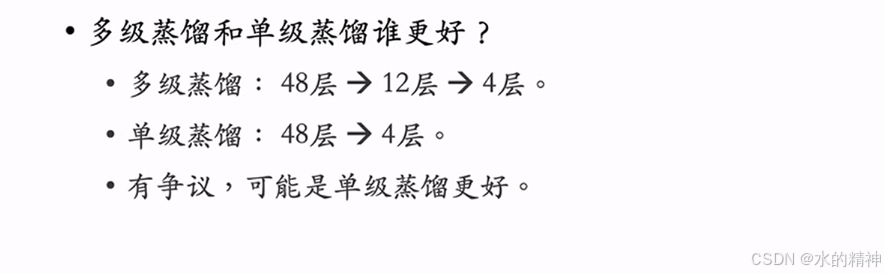