《Towards Black-Box Membership Inference Attack for Diffusion Models》
Abstract
- 识别艺术品是否用于训练扩散模型的挑战,重点是人工智能生成的艺术品中的成员推断攻击------copyright protection
- 不需要访问内部模型组件的新型黑盒攻击方法
- 展示了在评估 DALL-E 生成的数据集方面的卓越性能。
作者主张
previous methods are not yet ready for copyright protection in diffusion models.
Contributions(文章里有三点,我觉得只有两点)
- ReDiffuse:using the model's variation API to alter an image and compare it with the original one.
- A new MIA evaluation dataset:use the image titles from LAION-5B as prompts for DALL-E's API [31] to generate images of the same contents but different styles.
Algorithm Design
target model:DDIM
为什么要强行引入一个版权保护的概念???
定义black-box variation API
x ^ = V θ ( x , t ) \hat{x}=V_{\theta}(x,t) x^=Vθ(x,t)
细节如下:


总结为: x x x加噪变为 x t x_t xt,再通过DDIM连续降噪变为 x ^ \hat{x} x^
intuition
Our key intuition comes from the reverse SDE dynamics in continuous diffusion models.
one simplified form of the reverse SDE (i.e., the denoise step)
X t = ( X t / 2 − ∇ x log p ( X t ) ) + d W t , t ∈ [ 0 , T ] (3) X_t=(X_t/2-\nabla_x\log p(X_t))+dW_t,t\in[0,T]\tag{3} Xt=(Xt/2−∇xlogp(Xt))+dWt,t∈[0,T](3)
The key guarantee is that when the score function is learned for a data point x, then the reconstructed image x ^ i \hat{x}_i x^i is an unbiased estimator of x x x.(算是过拟合的另一种说法吧)
Hence,averaging over multiple independent samples x ^ i \hat{x}_i x^i would greatly reduce the estimation error (see Theorem 1).
On the other hand, for a non-member image x ′ x' x′, the unbiasedness of the denoised image is not guaranteed.
details of algorithm:
- independently apply the black-box variation API n times with our target image x as input
- average the output images
- compare the average result x ^ \hat{x} x^ with the original image.
evaluate the difference between the images using an indicator function:
f ( x ) = 1 [ D ( x , x ^ ) < τ ] f(x)=1[D(x,\hat{x})<\tau] f(x)=1[D(x,x^)<τ]
A sample is classified to be in the training set if D ( x , x ^ ) D(x,\hat{x}) D(x,x^) is smaller than a threshold τ \tau τ ( D ( x , x ^ ) D(x,\hat{x}) D(x,x^) represents the difference between the two images)
ReDiffuse
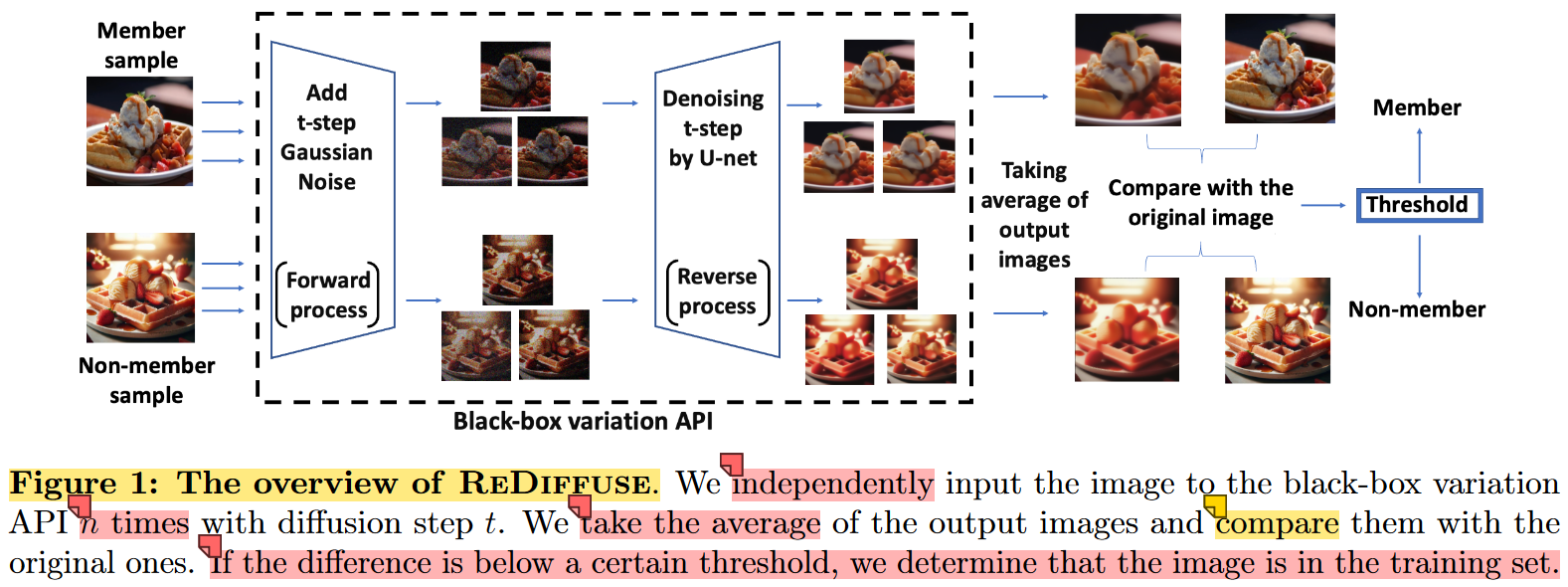
Theoretical Analysis
什么是sampling interval???
MIA on Latent Diffusion Models
泛化到latent diffusion model,即Stable Diffusion
ReDiffuse+
variation API for stable diffusion is different from DDIM, as it includes the encoder-decoder process.
z = E n c o d e r ( x ) , z t = α ‾ t z + 1 − α ‾ t ϵ , z ^ = Φ θ ( z t , 0 ) , x ^ = D e c o d e r ( z ^ ) (4) z={\rm Encoder}(x),\quad z_t=\sqrt{\overline{\alpha}_t}z+\sqrt{1-\overline{\alpha}t}\epsilon,\quad \hat{z}=\Phi{\theta}(z_t,0),\quad \hat{x}={\rm Decoder}(\hat{z})\tag{4} z=Encoder(x),zt=αt z+1−αt ϵ,z^=Φθ(zt,0),x^=Decoder(z^)(4)
modification of the algorithm
independently adding random noise to the original image twice and then comparing the differences between the two restored images x ^ 1 \hat{x}_1 x^1 and x ^ 2 \hat{x}_2 x^2:
f ( x ) = 1 [ D ( x ^ 1 , x ^ 2 ) < τ ] f(x)=1[D(\hat{x}_1,\hat{x}_2)<\tau] f(x)=1[D(x^1,x^2)<τ]
Experiments
Evaluation Metrics
- AUC
- ASR
- TPR@1%FPR
same experiment's setup in previous papers [5, 18].
target model | DDIM | Stable Diffusion |
---|---|---|
version | 《Are diffusion models vulnerable to membership inference attacks?》 | original:stable diffusion-v1-5 provided by Huggingface |
dataset | CIFAR10/100,STL10-Unlabeled,Tiny-Imagenet | member set:LAION-5B,corresponding 500 images from LAION-5;non-member set:COCO2017-val,500 images from DALL-E3 |
T | 1000 | 1000 |
k | 100 | 10 |
baseline methods | [5]Are diffusion models vulnerable to membership inference attacks?: SecMIA | [18]An efficient membership inference attack for the diffusion model by proximal initialization. | [28]Membership inference attacks against diffusion models |
---|---|---|---|
publication | International Conference on Machine Learning | arXiv preprint | 2023 IEEE Security and Privacy Workshops (SPW) |
Ablation Studies
- The impact of average numbers
- The impact of diffusion steps
- The impact of sampling intervals