React Agent 是指 LLM 对问题自行推理并调用外部工具解决问题,如下图所示,通过一些推理步骤最终找到想要的答案。
LlamaIndex 提供了实现 React Agent 的框架,通过框架可以轻松的实现上图中的步骤。那么,如果不用 LlamaIndex 应该如何实现一个 Agent 呢?首先,需要将需要调用的外部 API 和问题提供给LLM,LLM 判断得到结果是否需要调用API,如果需要调用,将 API 名称和参数相关信息返回到应用端,应用端执行API 并将结果再次传给 LLM,LLM 再次判断是否需要调用外部工具,这是个循环的过程,直到 LLM 得到答案。通过代码,分析一下 LlamaIndex 是如何实现 ReactAgent 多轮对话的。
用 LlamaIndex 实现 ReactAgent 代码如下:
from llama_index.core.agent import ReActAgent
from llama_index.llms.openai import OpenAI
from llama_index.core.tools import BaseTool, FunctionTool
from utils import init_model
import sys, os
sys.path.append(os.path.abspath(os.path.join(os.path.dirname(__file__), '..', 'parent_directory')))
def multiply(a: int, b: int) -> int:
"""Multiply two integers and returns the result integer"""
return a * b
multiply_tool = FunctionTool.from_defaults(fn=multiply)
def add(a: int, b: int) -> int:
"""Add two integers and returns the result integer"""
return a + b
add_tool = FunctionTool.from_defaults(fn=add)
init_model()
agent = ReActAgent.from_tools([multiply_tool, add_tool], verbose=True)
response = agent.chat("What is 20+(2*4)? Calculate step by step ")
实现 Agent 主要通过 FunctionTool 和 ReactAgent,分别看一下两个类的主要作用。
FunctionTool
FunctionTool 用来定义外部工具,包括 Function 的详细信息,功能描述、入参和出参等信息,通过跟踪可以看到,代码首先创建了两个 FunctionTool,ToolMetadata 定义了工具的详细信息。
ReactAgent
创建 ReactAgent,传入 tools、llm,max_iteration 是指最大推理次数,默认为 10 次。
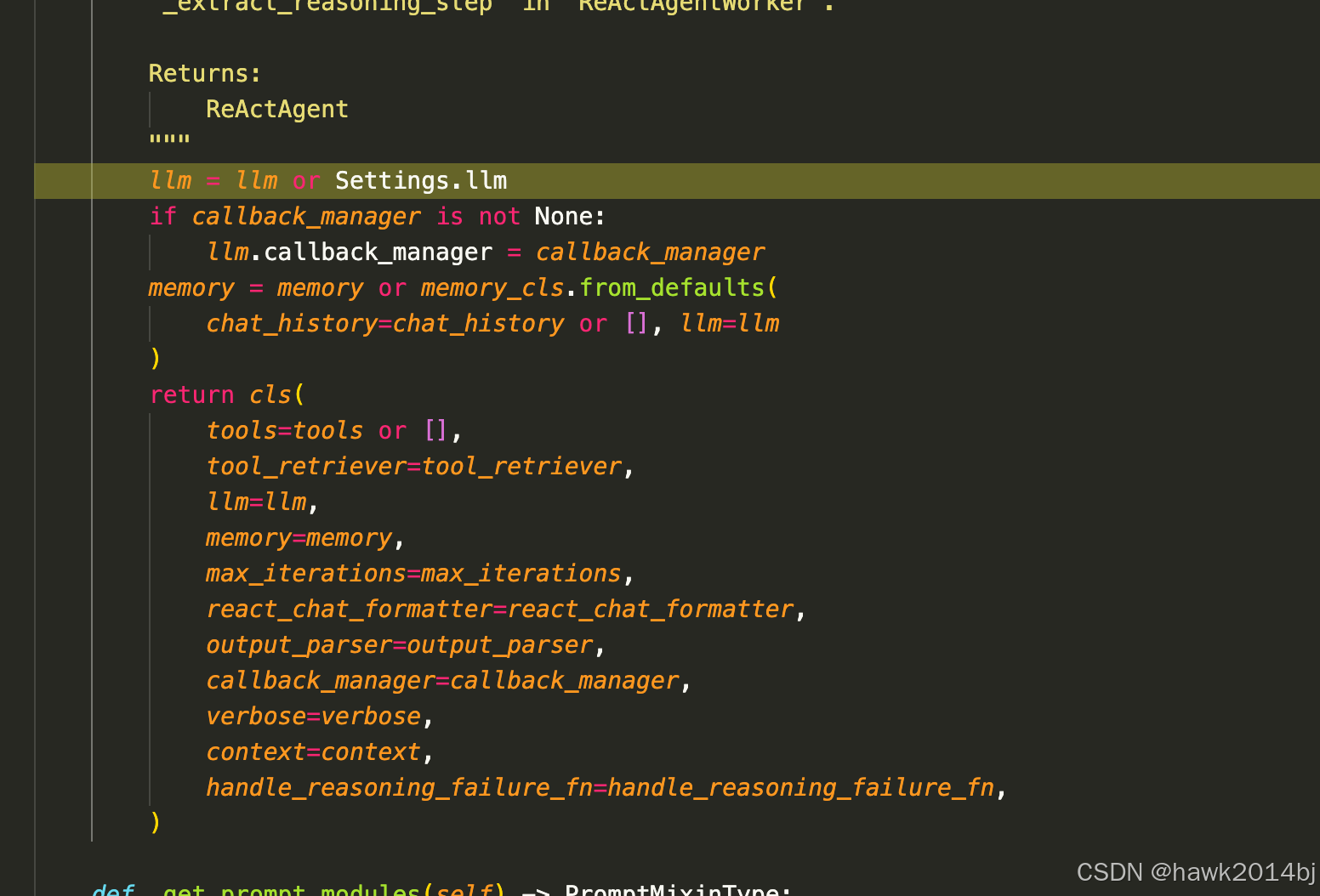
调用 self._react_chat_formatter 准备提示词,提示词准备完成之后,调用 LLM大模型进行处理。
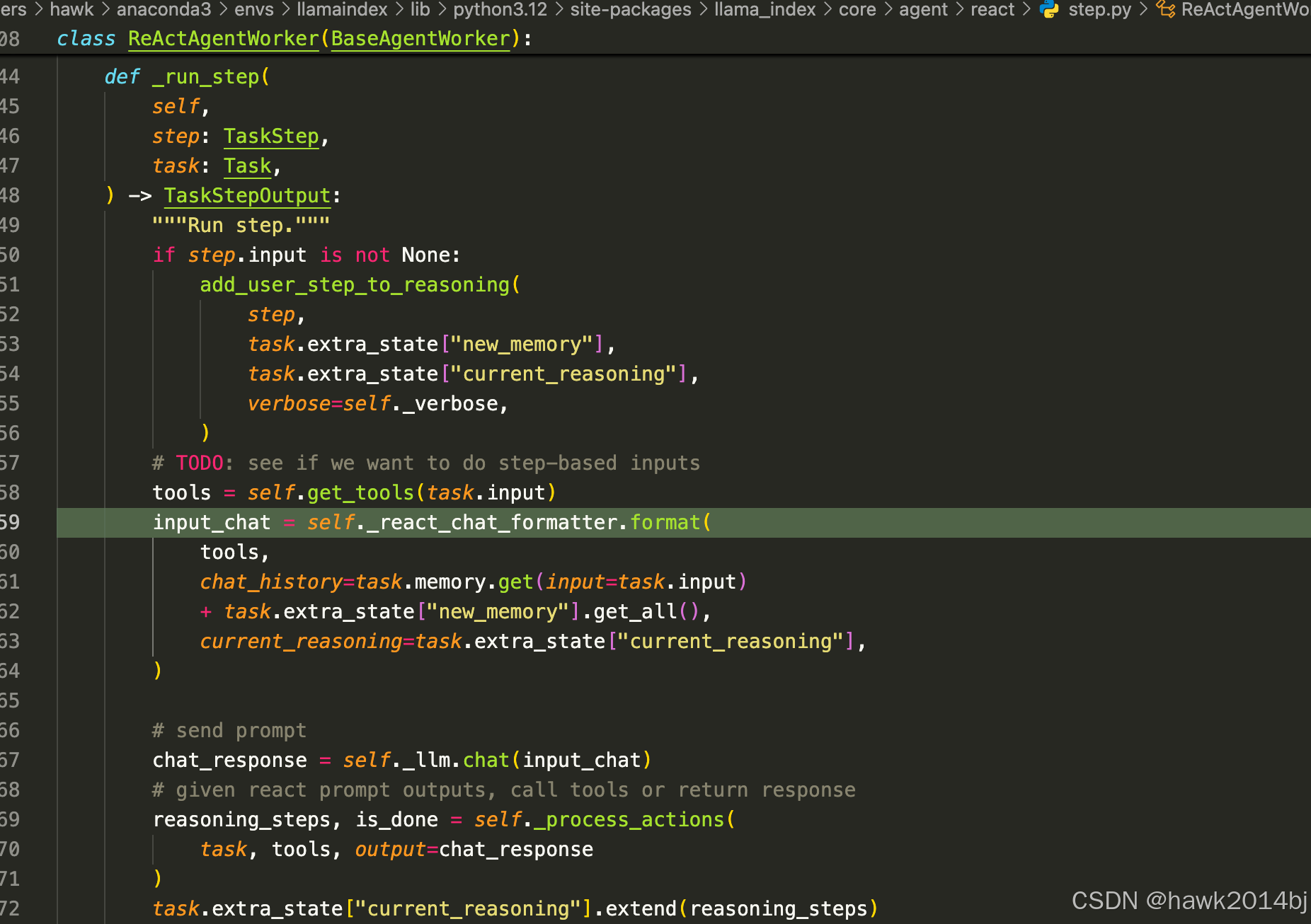
解析大模型返回结果并调用对应 Function。
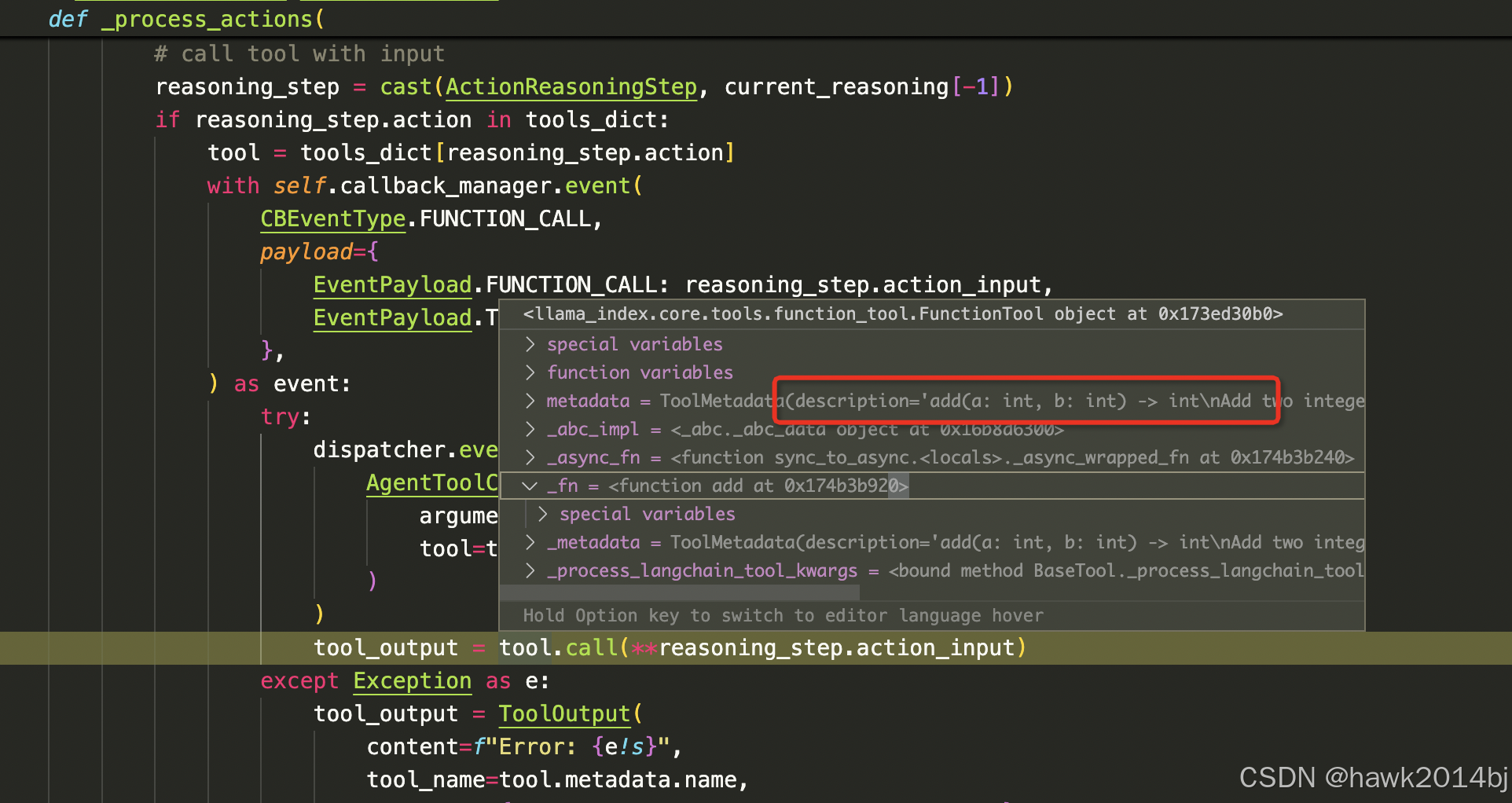
每一步的结果都会存储在 Memory 中,作为历史在下一步中传给 LLM 进行处理。
总结
LlamaIndex 通过提示词将问题与工具进行关联,通过多轮推理得到最终答案。核心还是在提示词,无论 RAG 还是 Agent,都是作为桥梁,底层逻辑还是通过提示词的方式把大模型的能力外部应用能力打通并进行整合。