本地部署 faster-whisper
- [1. 创建虚拟环境](#1. 创建虚拟环境)
- [2. 安装依赖模块](#2. 安装依赖模块)
- [3. 创建 Web UI](#3. 创建 Web UI)
- [4. 启动 Web UI](#4. 启动 Web UI)
- [5. 访问 Web UI](#5. 访问 Web UI)
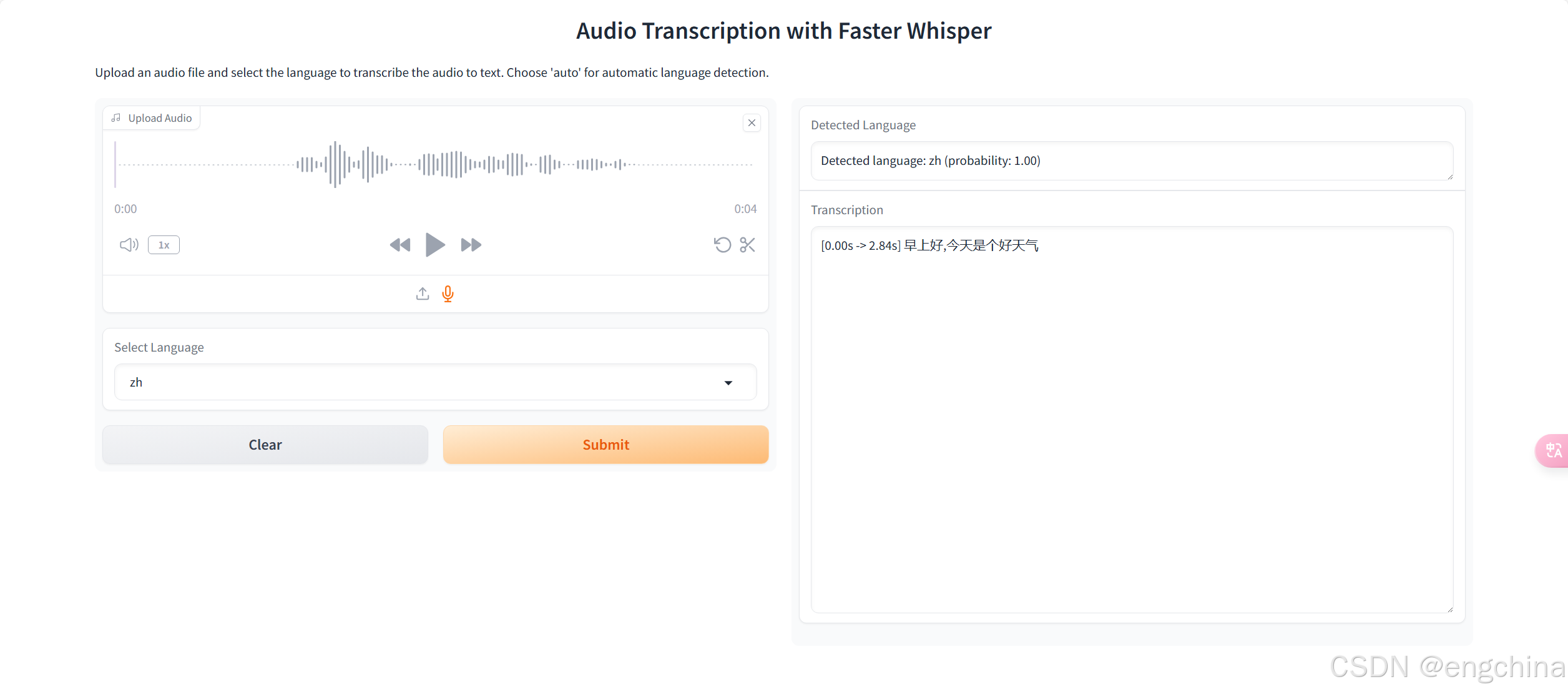
1. 创建虚拟环境
conda create -n faster-whisper python=3.11 -y
conda activate faster-whisper
2. 安装依赖模块
pip install torch==2.2.2 torchvision==0.17.2 torchaudio==2.2.2 --index-url https://download.pytorch.org/whl/cu118
pip install faster-whisper
conda install matplotlib
pip install gradio
3. 创建 Web UI
# webui.py
import gradio as gr
from faster_whisper import WhisperModel
# Initialize the model
# model_size = "large-v3"
model_size = "Systran/faster-whisper-large-v3"
model = WhisperModel(model_size, device="cuda", compute_type="float16")
def transcribe_audio(audio_file, language):
# Transcribe the audio
segments, info = model.transcribe(audio_file, beam_size=5, language=language)
# Prepare the output
transcription = ""
for segment in segments:
transcription += f"[{segment.start:.2f}s -> {segment.end:.2f}s] {segment.text}\n"
detected_language = f"Detected language: {info.language} (probability: {info.language_probability:.2f})"
return detected_language, transcription
# Define Gradio interface
iface = gr.Interface(
fn=transcribe_audio,
inputs=[
gr.Audio(type="filepath", label="Upload Audio"),
gr.Dropdown(["en", "zh", "ja"], label="Select Language", value="en")
],
outputs=[
gr.Textbox(label="Detected Language"),
gr.Textbox(label="Transcription", lines=20)
],
allow_flagging='never',
title="Audio Transcription with Faster Whisper",
description="Upload an audio file and select the language to transcribe the audio to text. Choose 'auto' for automatic language detection."
)
# Launch the interface
iface.launch()
4. 启动 Web UI
python webui.py
5. 访问 Web UI
使用浏览器打开 http://localhost:7860,
reference: