欢迎关注我的CSDN:https://spike.blog.csdn.net/
免责声明:本文来源于个人知识与公开资料,仅用于学术交流,欢迎讨论,不支持转载。
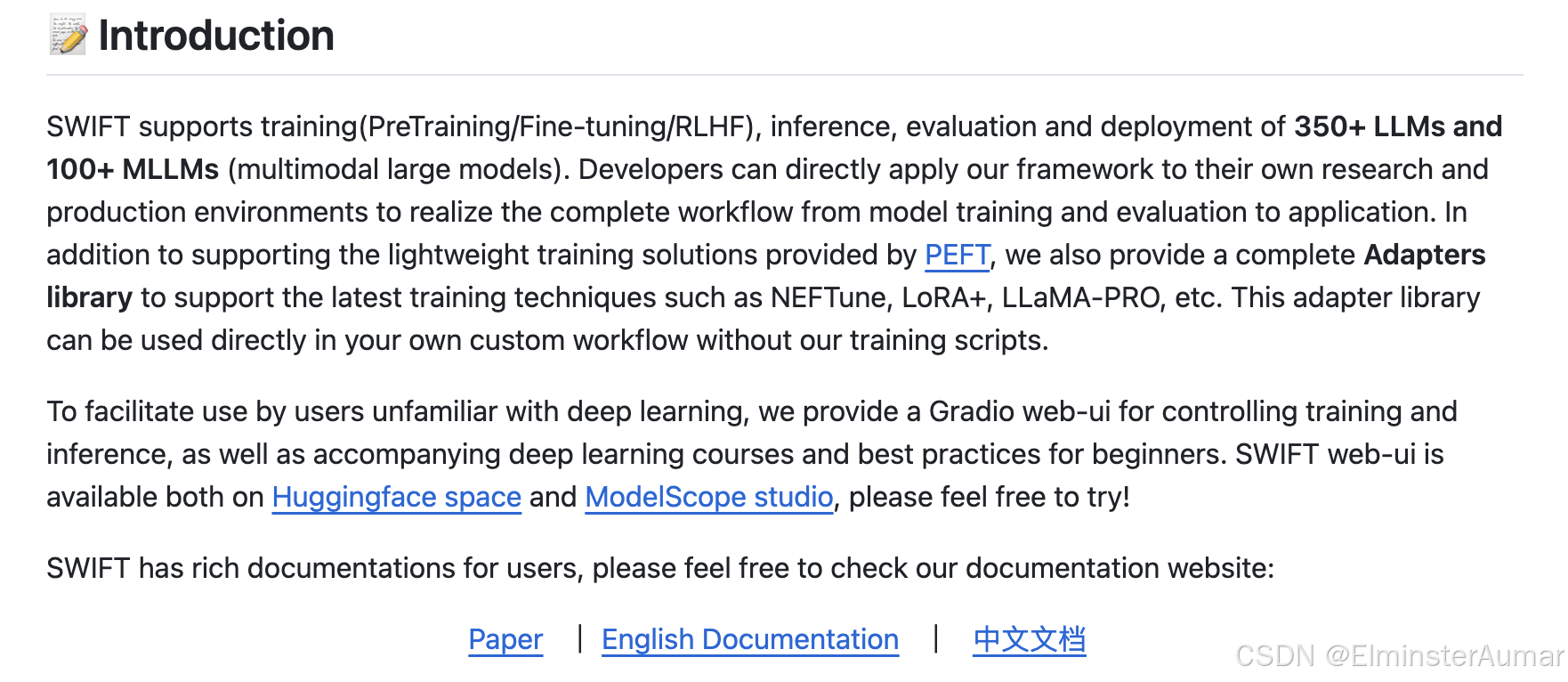
SWIFT 即 Scalable lightWeight Infrastructure for FineTuning (可扩展轻量级微调基础设施),是高效、轻量级的模型微调和推理框架,支持大语言模型(LLM) 和 多模态大型模型(MLLM) 的训练、推理、评估和部署。可以将 SWIFT 框架直接应用到研究和生产环境中,实现从模型训练和评估到应用的完整工作流程。
GitHub: modelscope/ms-swift
1. 数据集
测试数据集,视频文本数据集,即:
示例:
bash
Row 0: {'video_name': 'v_p1QGn0IzfW0', 'question_1': 'What equipment is visible in the gym where the boy is doing his routine?', 'question_2': 'None', 'answer': 'There is other equipment visible in the gym like a high bar and still rings.'}
视频:
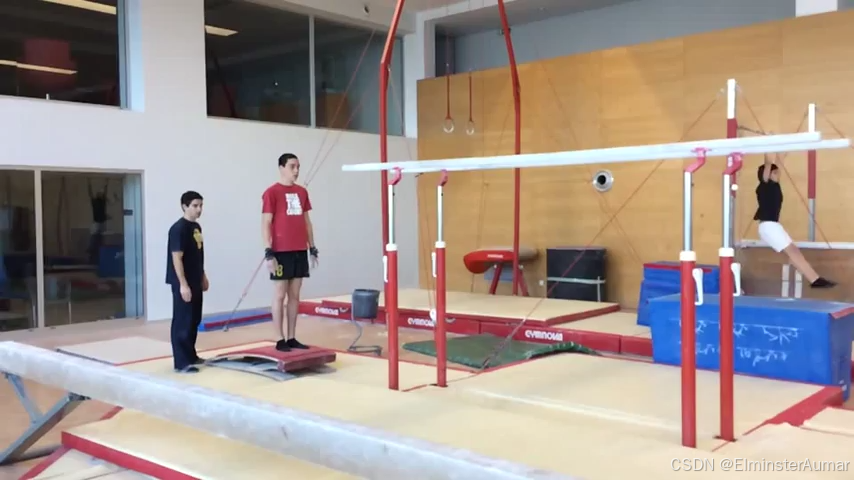
测试命令:
bash
NFRAMES=24 MAX_PIXELS=100352 CUDA_VISIBLE_DEVICES=0 swift infer --model_type qwen2-vl-7b-instruct
<video>What equipment is visible in the gym where the boy is doing his routine?
[your path]/modelscope_models/media_resources/v_p1QGn0IzfW0.mp4
# 模型输出
The video shows a red and white pole, a red and white bar, and a red and white pole in the gym where the boy is doing his routine.
# Fine-Tuning 模型输出 (明显更优)
Other equipment visible in the gym includes a high bar, still rings, and a platform with foam mats on the floor.
video_chatgpt
数据集处理函数:
python
def _preprocess_video_chatgpt(dataset: DATASET_TYPE) -> DATASET_TYPE:
url = 'https://modelscope.cn/datasets/swift/VideoChatGPT/resolve/master/videos.zip'
local_dir = MediaCache.download(url, 'video_chatgpt')
local_dir = os.path.join(local_dir, 'Test_Videos')
# only `.mp4`
mp4_set = [file[:-4] for file in os.listdir(local_dir) if file.endswith('mp4')]
def _process(d):
if d['video_name'] not in mp4_set:
return {'query': None, 'response': None, 'videos': None}
return {
'query': d['question'] or d['question_1'] or d['question_2'],
'response': d['answer'],
'videos': [os.path.join(local_dir, f"{d['video_name']}.mp4")]
}
return dataset.map(_process).filter(lambda row: row['query'] is not None)
支持提前使用 ModelScope 命令下载 VideoChatGPT
数据集,即:
bash
modelscope download --dataset swift/VideoChatGPT --local_dir swift/VideoChatGPT
复制到 ModelScope 的缓存目录:MODELSCOPE_CACHE
,缓存位置如下:
bash
[your folder]/modelscope_models/media_resources/video_chatgpt
需要进行重命名 VideoChatGPT
-> video_chatgpt
,同时,提前解压视频文件 videos.zip
-> Test_Videos
,这样就可以直接使用数据集:
bash
--dataset video-chatgpt
2. 微调
视频描述任务,指令微调的训练脚本,如下:
NFRAMES
使用的视频帧数MAX_PIXELS
最大像素数量,100352 = 1024x98
CUDA_VISIBLE_DEVICES=0,1,2,3,4,5,6,7 NPROC_PER_NODE=8
,多卡参数--dataset video-chatgpt
数据集
即:
bash
NFRAMES=24 MAX_PIXELS=100352 CUDA_VISIBLE_DEVICES=0,1,2,3,4,5,6,7 NPROC_PER_NODE=8 nohup swift sft \
--model_type qwen2-vl-7b-instruct \
--model_id_or_path qwen/Qwen2-VL-7B-Instruct \
--sft_type lora \
--dataset video-chatgpt \
--deepspeed default-zero2 \
--num_train_epochs 2 \
--batch_size 2 \
--eval_steps 100 \
--save_steps 100 \
> nohup.video-chatgpt.out &
训练日志:
bash
Train: 100%|████████████████████████████████████████████████████████████████████████████████████████████████████████████████████████████████| 398/398 [4:09:59<00:00, 21.84s/it]
{'eval_loss': 1.29713297, 'eval_acc': 0.63649852, 'eval_runtime': 83.265, 'eval_samples_per_second': 0.36, 'eval_steps_per_second': 0.024, 'epoch': 2.0, 'global_step/max_steps': '398/398', 'percentage': '100.00%', 'elapsed_time': '4h 11m 22s', 'remaining_time': '0s'}
Val: 100%|████████████████████████████████████████████████████████████████████████████████████████████████████████████████████████████████████████| 2/2 [00:02<00:00, 1.47s/it]
[INFO:swift] Saving model checkpoint to [your path]/llm/ms-swift/output/qwen2-vl-7b-instruct/v18-20241013-065323/checkpoint-398
{'train_runtime': 15092.9517, 'train_samples_per_second': 0.421, 'train_steps_per_second': 0.026, 'train_loss': 1.21231406, 'epoch': 2.0, 'global_step/max_steps': '398/398', 'percentage': '100.00%', 'elapsed_time': '4h 11m 32s', 'remaining_time': '0s'}
Train: 100%|████████████████████████████████████████████████████████████████████████████████████████████████████████████████████████████████| 398/398 [4:11:32<00:00, 37.92s/it]
[INFO:swift] last_model_checkpoint: [your path]/llm/ms-swift/output/qwen2-vl-7b-instruct/v18-20241013-065323/checkpoint-398
[INFO:swift] best_model_checkpoint: [your path]/llm/ms-swift/output/qwen2-vl-7b-instruct/v18-20241013-065323/checkpoint-398
[INFO:swift] images_dir: [your path]/llm/ms-swift/output/qwen2-vl-7b-instruct/v18-20241013-065323/images
[INFO:swift] End time of running main: 2024-10-13 11:15:43.850041
训练输出:
bash
[your path]/llm/ms-swift/output/qwen2-vl-7b-instruct/v18-20241013-065323/
TensorBoard 可视化模型输出:
bash
tensorboard --logdir="runs" --host=0.0.0.0 --port=6006
训练 Loss:
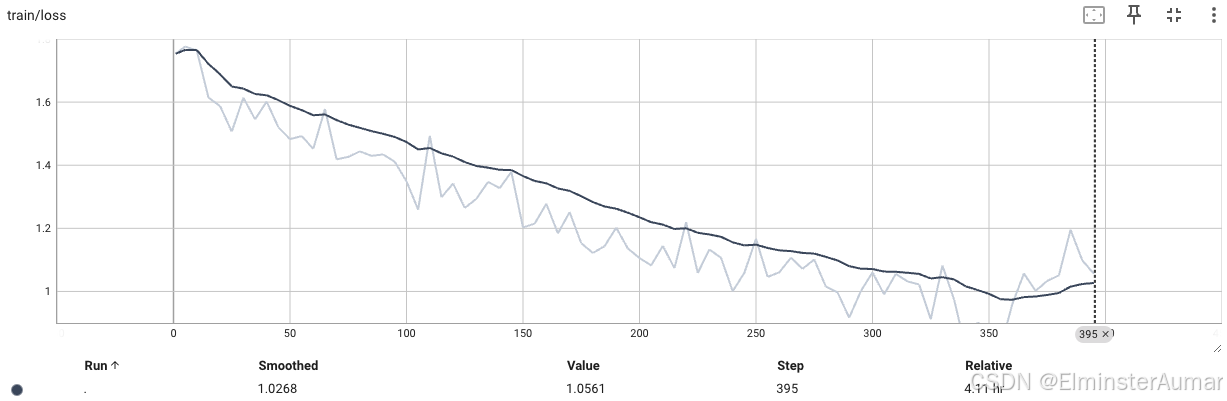
验证集 Loss:
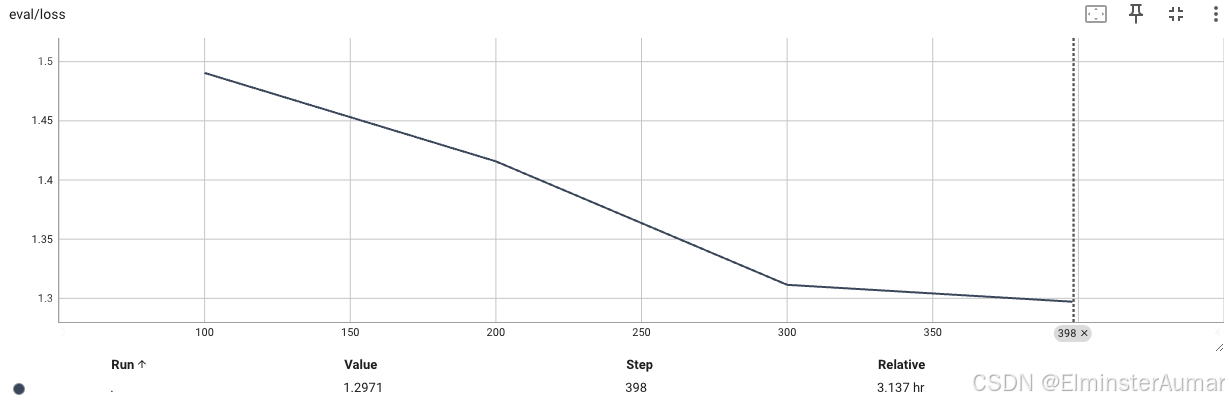
GPU 占用 (53.4G):
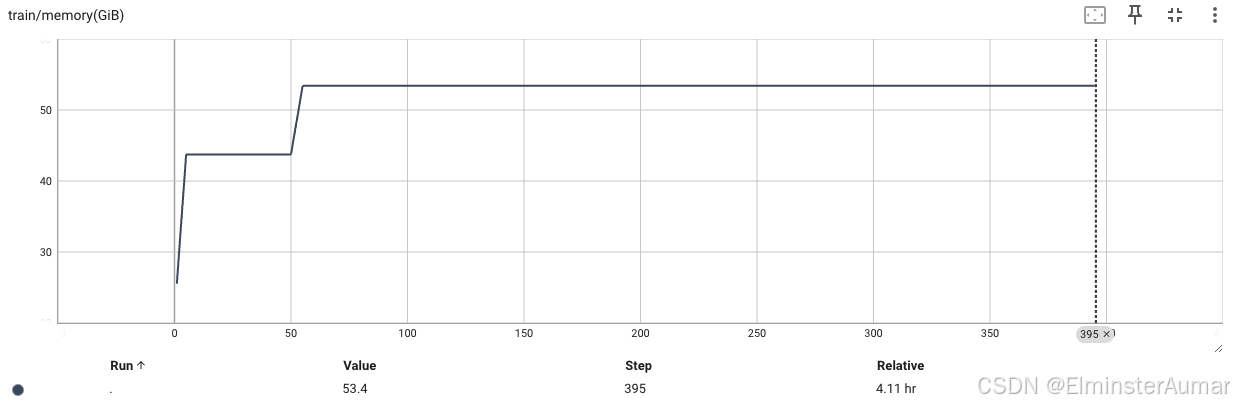
合并 LoRA 模型:
bash
CUDA_VISIBLE_DEVICES=0,1,2 swift infer \
--ckpt_dir [your path]/llm/ms-swift/output/qwen2-vl-7b-instruct/v18-20241013-065323/checkpoint-398/ \
--merge_lora true
# --load_dataset_config true
# 直接评估模型
使用 LoRA 模型:
bash
NFRAMES=24 MAX_PIXELS=100352 CUDA_VISIBLE_DEVICES=0,1,2 swift infer --ckpt_dir [your path]/llm/ms-swift/output/qwen2-vl-7b-instruct/v18-20241013-065323/checkpoint-398-merged/