训练完成后,把最后的权重文件,模型配置文件都放在一个文件夹下:
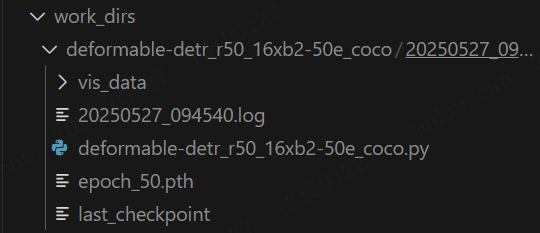
官方给出的测试及推理命令如下:
python
python tools/test.py <配置文件路径> <权重文件路径>
bash
python tools/test.py work_dirs/deformable-detr_r50_16xb2-50e_coco/20250527_094540/deformable-detr_r50_16xb2-50e_coco.py work_dirs/deformable-detr_r50_16xb2-50e_coco/20250527_094540/epoch_50.pth
看到输出结果如下:
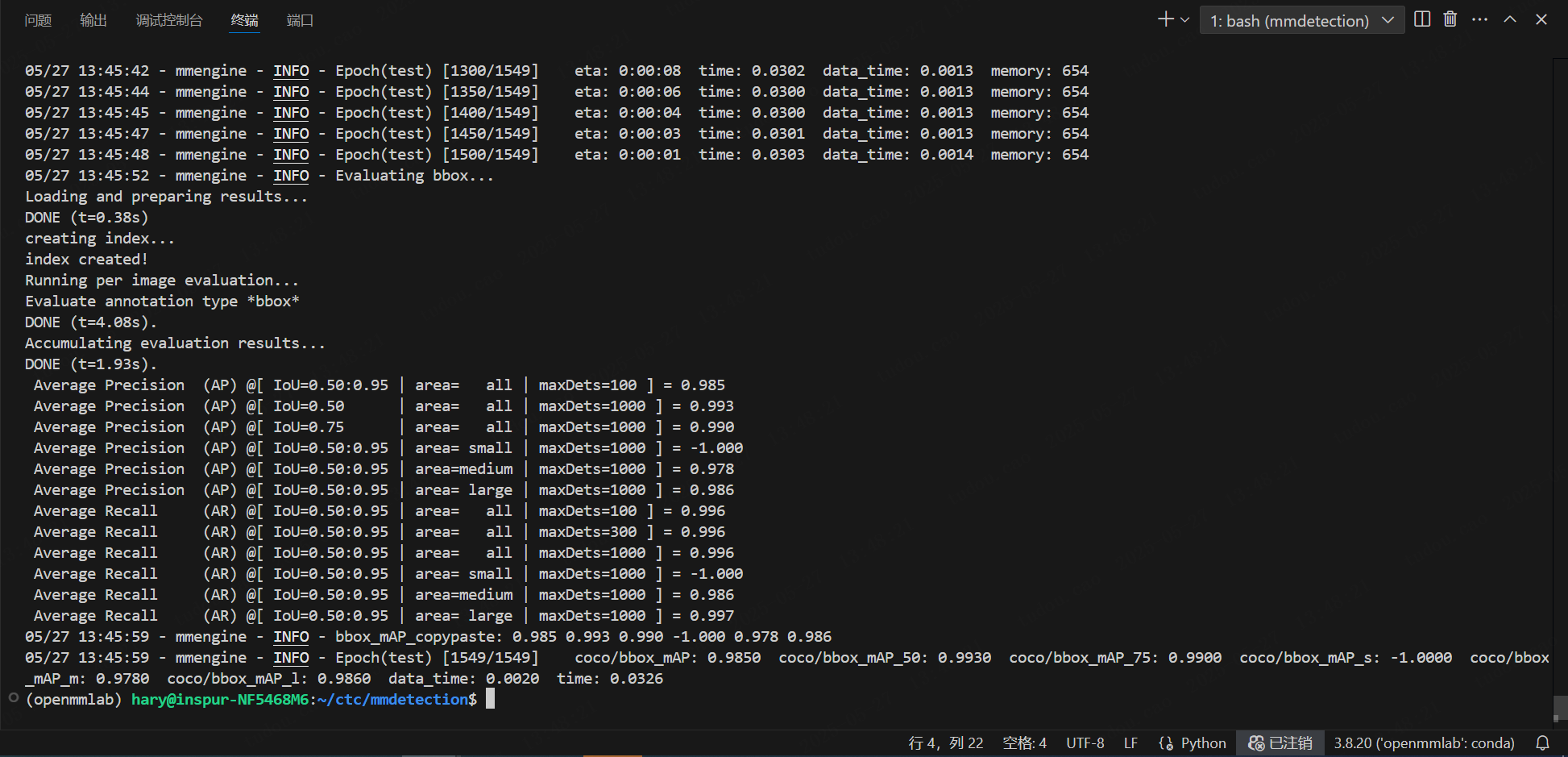
bash
Average Precision (AP) @[ IoU=0.50:0.95 | area= all | maxDets=100 ] = 0.985
Average Precision (AP) @[ IoU=0.50 | area= all | maxDets=1000 ] = 0.993
Average Precision (AP) @[ IoU=0.75 | area= all | maxDets=1000 ] = 0.990
Average Precision (AP) @[ IoU=0.50:0.95 | area= small | maxDets=1000 ] = -1.000
Average Precision (AP) @[ IoU=0.50:0.95 | area=medium | maxDets=1000 ] = 0.978
Average Precision (AP) @[ IoU=0.50:0.95 | area= large | maxDets=1000 ] = 0.986
Average Recall (AR) @[ IoU=0.50:0.95 | area= all | maxDets=100 ] = 0.996
Average Recall (AR) @[ IoU=0.50:0.95 | area= all | maxDets=300 ] = 0.996
Average Recall (AR) @[ IoU=0.50:0.95 | area= all | maxDets=1000 ] = 0.996
Average Recall (AR) @[ IoU=0.50:0.95 | area= small | maxDets=1000 ] = -1.000
Average Recall (AR) @[ IoU=0.50:0.95 | area=medium | maxDets=1000 ] = 0.986
Average Recall (AR) @[ IoU=0.50:0.95 | area= large | maxDets=1000 ] = 0.997
05/27 13:45:59 - mmengine - INFO - bbox_mAP_copypaste: 0.985 0.993 0.990 -1.000 0.978 0.986
05/27 13:45:59 - mmengine - INFO - Epoch(test) [1549/1549] coco/bbox_mAP: 0.9850 coco/bbox_mAP_50: 0.9930 coco/bbox_mAP_75: 0.9900 coco/bbox_mAP_s: -1.0000 coco/bbox_mAP_m: 0.9780 coco/bbox_mAP_l: 0.9860 data_time: 0.0020 time: 0.0326
并输出模型配置和日志记录文件夹:
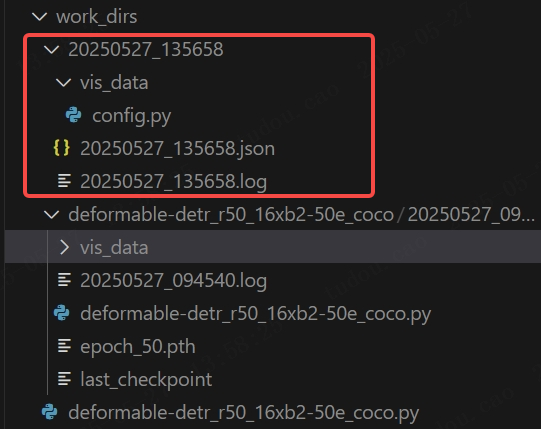
以上只是一个简单的验证,若需要查看每张图片的结果,需要另写代码文件:
我们对开始你的第一步 --- MMDetection 3.3.0 文档中测试安装成功的代码稍作改动,主要是打印结果信息:
bash
from mmdet.apis import init_detector, inference_detector
config_file = '/home/hary/ctc/mmdetection/work_dirs/deformable-detr_r50_16xb2-50e_coco/20250527_094540/deformable-detr_r50_16xb2-50e_coco.py' # 模型配置文件路径
checkpoint_file = '/home/hary/ctc/mmdetection/work_dirs/deformable-detr_r50_16xb2-50e_coco/20250527_094540/epoch_50.pth' # 模型权重文件路径
model = init_detector(config_file, checkpoint_file, device='cpu') # or device='cuda:0'
img_path = '/home/hary/ctc/mmdetection/Dataset_depth_COCO/val2017/1112_9-rgb.png' # 图片路径
result = inference_detector(model, img_path)
print('---------------------------------')
print(result)
打印结果:
bash
---------------------------------
<DetDataSample(
META INFORMATION
batch_input_shape: (800, 1067)
ori_shape: (960, 1280)
pad_shape: (800, 1067)
img_id: 0
img_path: '/home/hary/ctc/mmdetection/Dataset_depth_COCO/val2017/1112_9-rgb.png'
scale_factor: (0.83359375, 0.8333333333333334)
img_shape: (800, 1067)
DATA FIELDS
pred_instances: <InstanceData(
META INFORMATION
DATA FIELDS
scores: tensor([9.6636e-01, 8.6282e-01, 5.0045e-02, 3.0949e-02, 2.1291e-03, 1.8354e-03,
1.4797e-03, 9.5198e-04, 9.4724e-04, 9.0408e-04, 8.4457e-04, 7.7178e-04,
7.5072e-04, 7.4246e-04, 7.2029e-04, 7.0234e-04, 6.9444e-04, 6.6890e-04,
6.6067e-04, 6.5956e-04, 6.4741e-04, 6.2452e-04, 6.2145e-04, 6.0680e-04,
6.0662e-04, 5.8978e-04, 5.7643e-04, 5.6989e-04, 5.6188e-04, 5.6018e-04,
5.4903e-04, 5.3914e-04, 5.3539e-04, 5.3505e-04, 5.1647e-04, 5.1595e-04,
5.1284e-04, 5.0697e-04, 5.0271e-04, 4.9641e-04, 4.9617e-04, 4.9153e-04,
4.8392e-04, 4.6943e-04, 4.6823e-04, 4.6747e-04, 4.6432e-04, 4.6207e-04,
4.6087e-04, 4.5963e-04, 4.5072e-04, 4.4785e-04, 4.4583e-04, 4.4582e-04,
4.3889e-04, 4.3656e-04, 4.3481e-04, 4.3422e-04, 4.2235e-04, 4.1829e-04,
4.1680e-04, 4.1591e-04, 4.1196e-04, 4.0530e-04, 4.0237e-04, 3.9507e-04,
3.9482e-04, 3.8735e-04, 3.8713e-04, 3.8407e-04, 3.8263e-04, 3.8178e-04,
3.8151e-04, 3.7959e-04, 3.7872e-04, 3.7765e-04, 3.7668e-04, 3.7320e-04,
3.7057e-04, 3.6245e-04, 3.6220e-04, 3.6162e-04, 3.5402e-04, 3.5096e-04,
3.5083e-04, 3.5054e-04, 3.4927e-04, 3.4678e-04, 3.4543e-04, 3.4240e-04,
3.4200e-04, 3.4044e-04, 3.3560e-04, 3.3540e-04, 3.3506e-04, 3.3181e-04,
3.2372e-04, 3.2135e-04, 3.2047e-04, 3.1865e-04])
bboxes: tensor([[3.8038e+02, 3.3829e+02, 8.7101e+02, 5.1814e+02],
[8.0331e-01, 3.3403e+02, 2.0168e+02, 7.1068e+02],
[3.8038e+02, 3.3829e+02, 8.7101e+02, 5.1814e+02],
[8.0331e-01, 3.3403e+02, 2.0168e+02, 7.1068e+02],
[4.0823e+02, 3.4763e+02, 8.7735e+02, 5.2347e+02],
[4.0823e+02, 3.4763e+02, 8.7735e+02, 5.2347e+02],
[4.1606e+02, 3.4775e+02, 8.7032e+02, 5.2471e+02],
[4.1606e+02, 3.4775e+02, 8.7032e+02, 5.2471e+02],
[4.2050e+02, 3.5188e+02, 8.8307e+02, 5.3558e+02],
[3.9088e+02, 3.9543e+02, 8.6223e+02, 5.3254e+02],
[0.0000e+00, 4.1365e+02, 4.3029e+02, 6.4290e+02],
[3.2440e+02, 8.4257e+02, 7.7319e+02, 9.6000e+02],
[3.8511e+02, 4.8201e+02, 8.7394e+02, 5.7323e+02],
[3.6575e+02, 5.1804e+02, 8.5130e+02, 5.9951e+02],
[3.3422e+02, 6.9991e+02, 8.4247e+02, 7.3495e+02],
[3.6575e+02, 5.1804e+02, 8.5130e+02, 5.9951e+02],
[3.8627e+02, 4.8445e+02, 8.6102e+02, 5.7369e+02],
[4.1046e+02, 4.9052e+02, 8.6503e+02, 5.8720e+02],
[4.2050e+02, 3.5188e+02, 8.8307e+02, 5.3558e+02],
[3.9088e+02, 3.9543e+02, 8.6223e+02, 5.3254e+02],
[3.7638e+02, 7.6128e+02, 8.9853e+02, 7.8412e+02],
[0.0000e+00, 8.4846e+02, 4.7391e+02, 9.6000e+02],
[0.0000e+00, 3.9305e+02, 4.1092e+02, 7.4043e+02],
[3.7064e+02, 6.1048e+02, 8.7357e+02, 6.6218e+02],
[3.8627e+02, 4.8445e+02, 8.6102e+02, 5.7369e+02],
[3.5878e+02, 8.5559e+02, 8.2981e+02, 9.6000e+02],
[0.0000e+00, 4.0445e+02, 4.1523e+02, 6.2125e+02],
[3.8181e+02, 7.6912e+02, 9.2646e+02, 7.9225e+02],
[2.6032e+02, 8.4527e+02, 7.3658e+02, 9.6000e+02],
[4.0113e+02, 5.7291e+02, 8.7452e+02, 6.3975e+02],
[0.0000e+00, 4.2017e+02, 4.4482e+02, 6.2521e+02],
[3.2440e+02, 8.4257e+02, 7.7319e+02, 9.6000e+02],
[0.0000e+00, 4.0378e+02, 4.1072e+02, 7.2026e+02],
[4.2259e+02, 8.0411e+02, 9.6577e+02, 8.2172e+02],
[3.4414e+02, 6.9423e+02, 8.6352e+02, 7.2694e+02],
[3.9884e+02, 3.8749e+02, 8.6840e+02, 5.4867e+02],
[0.0000e+00, 4.0742e+02, 4.3495e+02, 6.3147e+02],
[3.5916e+02, 6.9879e+02, 8.7993e+02, 7.3169e+02],
[6.6263e+02, 5.6504e+02, 9.8699e+02, 6.6078e+02],
[5.6836e+02, 6.8688e+02, 1.0009e+03, 7.3245e+02],
[4.0486e+02, 3.5922e+02, 8.6717e+02, 5.2445e+02],
[4.0486e+02, 3.5922e+02, 8.6717e+02, 5.2445e+02],
[5.2048e+02, 8.6254e+02, 9.8947e+02, 9.6000e+02],
[0.0000e+00, 3.9575e+02, 4.0728e+02, 6.2371e+02],
[0.0000e+00, 4.0989e+02, 4.3611e+02, 6.5765e+02],
[0.0000e+00, 3.8116e+02, 3.6796e+02, 6.8372e+02],
[3.9884e+02, 3.8749e+02, 8.6840e+02, 5.4867e+02],
[4.1046e+02, 4.9052e+02, 8.6503e+02, 5.8720e+02],
[0.0000e+00, 4.0378e+02, 4.1072e+02, 7.2026e+02],
[0.0000e+00, 3.9417e+02, 3.9502e+02, 6.1512e+02],
[0.0000e+00, 3.8116e+02, 3.6796e+02, 6.8372e+02],
[0.0000e+00, 3.9305e+02, 4.1092e+02, 7.4043e+02],
[3.4248e+02, 6.9715e+02, 8.7662e+02, 7.2740e+02],
[5.6609e+02, 8.6611e+02, 1.0528e+03, 9.6000e+02],
[0.0000e+00, 4.1365e+02, 4.3029e+02, 6.4290e+02],
[0.0000e+00, 3.9575e+02, 4.0728e+02, 6.2371e+02],
[0.0000e+00, 4.0742e+02, 4.3495e+02, 6.3147e+02],
[4.4883e+02, 8.5760e+02, 9.2626e+02, 9.6000e+02],
[0.0000e+00, 4.0445e+02, 4.1523e+02, 6.2125e+02],
[3.3355e+02, 7.0234e+02, 8.5813e+02, 7.3405e+02],
[1.2862e+02, 8.3867e+02, 6.2651e+02, 9.6000e+02],
[0.0000e+00, 4.2017e+02, 4.4482e+02, 6.2521e+02],
[4.1458e+02, 3.6223e+02, 8.6780e+02, 5.4675e+02],
[0.0000e+00, 0.0000e+00, 5.6674e+02, 2.2335e+02],
[0.0000e+00, 4.1518e+02, 4.2211e+02, 7.2022e+02],
[0.0000e+00, 8.4846e+02, 4.7391e+02, 9.6000e+02],
[1.0618e+03, 3.3472e+02, 1.2800e+03, 6.7609e+02],
[0.0000e+00, 4.1638e+02, 4.3290e+02, 6.9870e+02],
[0.0000e+00, 0.0000e+00, 5.2049e+02, 2.4524e+02],
[0.0000e+00, 3.8412e+02, 3.9710e+02, 6.0965e+02],
[0.0000e+00, 8.6693e+02, 4.3433e+02, 9.6000e+02],
[0.0000e+00, 3.9417e+02, 3.9502e+02, 6.1512e+02],
[0.0000e+00, 2.3495e+02, 3.4734e+02, 5.3374e+02],
[0.0000e+00, 4.0989e+02, 4.3611e+02, 6.5765e+02],
[2.8229e+02, 7.3323e+02, 8.4686e+02, 7.5988e+02],
[3.2823e+02, 0.0000e+00, 8.0437e+02, 2.8706e+02],
[0.0000e+00, 4.1518e+02, 4.2211e+02, 7.2022e+02],
[0.0000e+00, 3.8926e+02, 3.5770e+02, 6.0124e+02],
[8.3593e+02, 0.0000e+00, 1.2800e+03, 2.7487e+02],
[0.0000e+00, 8.6693e+02, 4.3433e+02, 9.6000e+02],
[0.0000e+00, 0.0000e+00, 5.3297e+02, 2.4709e+02],
[0.0000e+00, 0.0000e+00, 4.9581e+02, 1.9596e+02],
[2.0668e+02, 8.5584e+02, 7.0114e+02, 9.6000e+02],
[5.5672e+02, 8.6939e+02, 1.0421e+03, 9.6000e+02],
[3.8511e+02, 4.8201e+02, 8.7394e+02, 5.7323e+02],
[6.2638e+02, 5.2157e+02, 9.5959e+02, 6.4872e+02],
[8.2546e+02, 0.0000e+00, 1.2800e+03, 2.6732e+02],
[4.0113e+02, 5.7291e+02, 8.7452e+02, 6.3975e+02],
[5.7526e+02, 4.9513e+02, 9.3053e+02, 6.2974e+02],
[0.0000e+00, 0.0000e+00, 5.3319e+02, 2.2862e+02],
[8.2112e+02, 0.0000e+00, 1.2800e+03, 2.1693e+02],
[5.2048e+02, 8.6254e+02, 9.8947e+02, 9.6000e+02],
[0.0000e+00, 0.0000e+00, 5.3928e+02, 1.9326e+02],
[0.0000e+00, 8.6855e+02, 4.3088e+02, 9.6000e+02],
[0.0000e+00, 3.8412e+02, 3.9710e+02, 6.0965e+02],
[3.9847e+02, 0.0000e+00, 9.7181e+02, 2.6527e+02],
[0.0000e+00, 0.0000e+00, 4.9859e+02, 1.9115e+02],
[3.9252e+02, 3.6897e+02, 8.5414e+02, 5.6715e+02],
[3.2823e+02, 0.0000e+00, 8.0437e+02, 2.8706e+02],
[0.0000e+00, 0.0000e+00, 5.4149e+02, 2.5021e+02]])
labels: tensor([0, 1, 1, 0, 0, 1, 1, 0, 1, 0, 0, 1, 1, 1, 1, 0, 1, 1, 0, 1, 1, 0, 1, 1,
0, 1, 0, 1, 1, 1, 0, 0, 0, 1, 1, 0, 0, 1, 1, 1, 1, 0, 1, 1, 0, 1, 1, 0,
1, 1, 0, 0, 1, 1, 1, 0, 1, 1, 1, 1, 1, 1, 0, 0, 0, 1, 0, 0, 0, 1, 1, 0,
1, 1, 1, 1, 1, 1, 0, 0, 0, 0, 1, 1, 0, 1, 0, 0, 1, 0, 0, 0, 0, 0, 0, 1,
0, 1, 0, 0])
) at 0x7f58f8515d90>
ignored_instances: <InstanceData(
META INFORMATION
DATA FIELDS
labels: tensor([], dtype=torch.int64)
bboxes: tensor([], size=(0, 4))
) at 0x7f58f8515ee0>
gt_instances: <InstanceData(
META INFORMATION
DATA FIELDS
labels: tensor([], dtype=torch.int64)
bboxes: tensor([], size=(0, 4))
) at 0x7f58f8515fa0>
) at 0x7f58f85ee220>
可以看到,打印结果包含以下信息:
1.META INFORMATION(元数据信息)
-
batch_input_shape: (800, 1067)
:模型实际接收的输入图像尺寸 -
ori_shape: (960, 1280)
:原始图像的尺寸(高度960px,宽度1280px) -
pad_shape: (800, 1067)
:预处理后填充调整后的尺寸 -
img_path
: 输入图像绝对路径 -
scale_factor: (0.83359375, 0.8333333333333334)
:图像缩放比例(原始尺寸到输入尺寸的缩放比例) -
img_shape: (800, 1067)
:实际输入模型的图像尺寸
2.DATA FIELDS(核心数据字段)
-
pred_instances
:预测结果实例-
scores
:置信度分数张量(共100个预测结果,按置信度降序排列)- 最高置信度:0.96636(约96.6%),最低置信度:0.00034289(约0.034%)
-
bboxes
:边界框坐标(XYXY格式) -
labels
:类别标签(共100个,对应数据集的类别ID)
-
-
ignored_instances
:被忽略的实例(当前为空,表示没有设置忽略区域) -
gt_instances
:真实标注(推理模式下为空,仅在训练/验证时包含标注信息)
分析:模型输出了100个预测结果(默认保留top100),而有效预测只集中在前几个高置信度结果(前2个预测置信度 >0.8,第3个预测置信度骤降至0.05),说明存在大量低质量预测(置信度 <0.01),增加阈值过滤让结果变得"干净"一些:
python
from mmdet.apis import init_detector, inference_detector
config_file = '/home/hary/ctc/mmdetection/work_dirs/deformable-detr_r50_16xb2-50e_coco/20250527_094540/deformable-detr_r50_16xb2-50e_coco.py'
checkpoint_file = '/home/hary/ctc/mmdetection/work_dirs/deformable-detr_r50_16xb2-50e_coco/20250527_094540/epoch_50.pth'
model = init_detector(config_file, checkpoint_file, device='cpu') # or device='cuda:0'
img_path = '/home/hary/ctc/mmdetection/Dataset_depth_COCO/val2017/1112_9-rgb.png'
result = inference_detector(model, img_path)
print('---------------------------------')
# 让我们稍作一点改动:过滤置信度大于0.3的结果
valid_idx = result.pred_instances.scores > 0.3
filtered_bboxes = result.pred_instances.bboxes[valid_idx]
filtered_scores = result.pred_instances.scores[valid_idx]
filtered_labels = result.pred_instances.labels[valid_idx]
print(filtered_bboxes)
print(filtered_scores)
print(filtered_labels)
打印结果,这个时候就过滤掉了大量的无效预测:
bash
---------------------------------
tensor([[3.8038e+02, 3.3829e+02, 8.7101e+02, 5.1814e+02],
[8.0331e-01, 3.3403e+02, 2.0168e+02, 7.1068e+02]])
tensor([0.9664, 0.8628])
tensor([0, 1])
我们来打印下json文件里的真实标签:
python
from pycocotools.coco import COCO
import os
# 标注文件路径和图片目录
ann_file = 'Dataset_depth_COCO/annotations/instances_val2017.json' # 替换为你的标注文件路径
image_dir = 'Dataset_depth_COCO/val2017' # 替换为图片文件夹路径
# 初始化 COCO API
coco = COCO(ann_file)
# 指定要查询的图片文件名或 ID
target_image_name = '1112_9-rgb.png' # 示例图片文件名
# target_image_id = 391895 # 如果已知图片ID,直接使用
# 根据文件名查找图片ID
target_image_id = None
for img_id in coco.imgs:
img_info = coco.loadImgs(img_id)[0]
if img_info['file_name'] == target_image_name:
target_image_id = img_id
break
if target_image_id is None:
print(f"图片 {target_image_name} 不存在!")
exit()
# 获取该图片的所有标注信息
ann_ids = coco.getAnnIds(imgIds=target_image_id)
annotations = coco.loadAnns(ann_ids)
# 打印标签信息
print(f"图片 {target_image_name} (ID: {target_image_id}) 的标签:")
for ann in annotations:
category_info = coco.loadCats(ann['category_id'])[0]
print(f"""
- 类别名称: {category_info['name']} (ID: {ann['category_id']})
- 边界框: {ann['bbox']} [x, y, width, height]
- 面积: {ann['area']}
- 分割掩码: {'存在' if 'segmentation' in ann else '无'}
""")
输出:
bash
loading annotations into memory...
Done (t=0.02s)
creating index...
index created!
图片 1112_9-rgb.png (ID: 683) 的标签:
- 类别名称: shallow_box (ID: 0)
- 边界框: [387.00096, 334.99968, 477.9993600000001, 181.00032] [x, y, width, height]
- 面积: 86518.0371197952
- 分割掩码: 存在
- 类别名称: shallow_half_box (ID: 1)
- 边界框: [1.0009600000000063, 335.00015999999994, 193.99936000000002, 373.99968] [x, y, width, height]
- 面积: 72555.69856020481
- 分割掩码: 存在
可以看到,模型的预测是较为精确的。
**提请注意:**COCO数据集中的(x,y,w,h)和YOLO格式的(x,y,w,h)有本质区别,COCO格式的(x,y)是左上角点,且COCO数据集标签不做归一化,YOLO格式的(x,y)是框中心点,并且标签做归一化处理:
左:YOLO格式,右:COCO格式
将几种标注格式放在一起,读者自行体会:
COCO数据集下本图片的标签:
bash
class_id x_coco y_coco w h
0 387.0009 334.9997 477.9994 181.0003
1 1.0009 335.0001 193.9993 373.9996
XYXY格式下本图片的标签:
bash
class_id x_min y_min x_max y_max
0 387.0009 334.9997 865.0003 516.0000
1 1.0009 335.0001 195.0003 708.9998
YOLO格式下本图片的标签(图片高宽:960*1280):
bash
class_id x_yolo y_yolo w h
0 0.489063 0.443229 0.373437 0.188542
1 0.076563 0.543750 0.151562 0.389583