说明
之前好几个企业都提过飞机在飞的时候换发动机的例子,来比喻变革是多么无奈和危险。还有的说法更直接:不变等死,变就是找死,总得选一样。
后来我自己的体会也差不多是这样,总有一些窘境让你抉择:是忽忽悠悠瞎搞完,轻松点,还是大刀阔斧,不厌其烦的重设计、重构、自定义开发...
我总是觉得算法、架构这些coding的事是我喜欢的,也可能因为这样,我会想的更长远一些 。所以我不太接受被动式的做法,事实上由于技术的飞速进步,固守等死的deadline甚至比想象中来的更快。我之前以为固守的人很少,但事实上比我想象的多太多,甚至我都看不到几个愿意像我一样冒险、折腾的。我的臆测是,他们的潜意识在自我催眠,绝不走出舒适区,虽然水位一直上涨,都快到鼻子了...。
言归正传,反正我又换了一次发动机,过程当然是很曲折的。
ps: 大半夜的,刚充完电回来,在路上碰到两个红灯,四下无人,非常安静。突然觉得DataFlow的意义有点像红绿灯,虽然有的时候降低了理论的最大通行效率,但正是有这样的规则在,才使得汽车与人和谐相处,整个大的交通体系才得以存在。
内容
1 过程
开局是一台基本上全裸的服务器,还是Redhat系统(我熟悉的是Ubuntu)。总体感觉下来,还是ubuntu感觉好。
大概装了以下一些服务
- 1 redis(7) -- local yum port(55555)
- 2 clickhouse -- docker port(19000,18123)
- 3 flask-celery -- local conda systemd port(24104)
- /opt/flask_celery
-
- s2ch:将stream内容同步到clickhouse
- 4 *flask-aps -- docker port(24010)
- /opt/flask_aps/sync_tasks.py 手工执行任务发布
- 5 *message_hub: 提供对外的缓冲请求服务(异步):只允许扁平化字典列表
- rec_id
- task_for
- function_type
- data
大致的功能就是将Redis作为消息队列(效率真挺高),将ClickHouse作为持久化数据库(
对我来说,这次最核心的并不是处理逻辑,而是数据流转体系的实证。
2 目的
Q: 为什么需要数据流转体系?
在跨过了初级阶段后,我发现几乎所有的任务都不可能一步完成,而且很多时候会呈现一种很长的处理链条。所以自然而然地,一个完整的工作需要切分成很多段,分很多次完成。而且如果恰好有些处理阶段之后的数据形态很有用,那么就会又延伸出一条链。最终就是一张网,而这张网显然是超出人的管理能力范围的。
如果说每一步的结果形成了一个数据点,那么数据流转体系的核心就是把这些数据点连接起来,形成一个有向流动的网络。
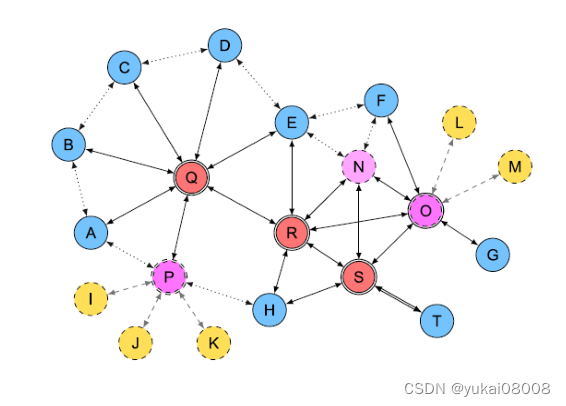
这样整体的工作就分为了两类:
- 1 数据流设计
- 2 逻辑处理
数据流设计是面向整体的需求,确保了功能的完整、严密以及节约(数据复用);而逻辑处理就是指从A到B所进行的特定加工。数据流设计根据业务需求而定,在针对不同任务时会有不同的数据库选型:
- 1 Mongo: 适合偏前端的合并存储。因为Mongo本身的结构很灵活,对于哪些需要灵活拓展的关节点,比较适合用Mongo。
- 2 Clickhouse: 适合偏后端的应用存储。列式存储使得特定的查询以及存储都很有优势,在数据处理到后端时,用于给人进行决策或者程序进行数据块读取时都很方便。
- 3 Milvus。适合偏中段的特征存储。例如一些文本经过令牌化,称为特征,以及数据进行特征处理形成的向量。
更完成的图结构还包含了任意两个点之间的连接:这是数据点和逻辑之间的衔接。
模式1:单步Web请求
将复杂的处理封装为异步服务。数据通过WebEntry进入处理体系,其API负责将用户的请求数据输入Stream In。Worker只盯着Stream In,处理后存放到Stream Out。
有一个同步程序,将数据从Stream Out写入ClickHouse。
模式2:数据库同步
本例中不存在,但可以想象,这是一种合理的方式。
模式3:队列分级
当业务或处理比较复杂时,可能需要再分为很多子类型讨论。所以有Stream到Stream的分发性同步。这种模式特别适合超复杂场景。
3 实际场景
tom11r作为中转和存储的中心,完成异步任务的分发和持久化。由于数据总量很大,且结果需要进行持久化保存,所以对于冷数据,还需要挪到tom8r。
mongo(MG)、clickhouse(CH)是持久化库,用于做处理流程的节点:保存定义完善(且相对固定)的数据结构。mongo用于更有弹性的数据存储,clickhouse用于更刚性的存储。mongo更倾向于写入,clickhouse更倾向于读取、分析。
milvas(MV)在这个任务中暂时用不到,MV用于暂存大量的中间结果(只适合用向量的形式)。
流转分为两大类型:简单流转与变换流转,但从形式上可以进行统一(看起来步骤会多一层,但是可以容纳所有的模式。
bash
Rule1: 节点不会直接通往另一个节点
Rule2: 节点的出口都是Stream
Rule3: 节点的入口都是Stream
Rule4: Stream分为In和Out两种类型
Rule5: 处理Stream的只有worker
在本例中,只涉及到Stream和Clickhouse。
- 1 队列入口:通过一个API提交数据,进入队列(stream_in)。服务会判定队列的可写入性,并发写入,如果有问题会在返回消息中提示。主要是防止队列溢出。
- 2 Worker: 逻辑处理程序的接口就是获取数据,处理后写入队列(stream_out)。到这里worker的任务就完成了,这样逻辑所需要的数据IO非常简单。
- 3 队列到ClichHouse。数据通过固定的定时-异步任务转存到数据库。这里要注意,任务需要拿到存库的回执,确保正确后才可以删除消息。
Q: Stream会到Stream吗?
A: 这是完全可能的。当Stream为一个总的入口时(混杂多任务)可以被进一步分流到另一个层级更低的Stream。原则上,一个worker应该是专门处理一个类型的任务的,这样会比较节约资源,且逻辑上比较清晰易懂。
Q: ClickHouse会到ClickHouse吗?
A: 技术上可行,但不在数据流中考虑。如果要同步,仍然是先将数据取到Stream中,然后再通过Stream到ClickHouse。
Note:Stream和ClickHouse的效率都接近内存。
3.1 环境
在AnyGPU的租用主机上执行测试
3.1.1 Redis
使用Redis的Stream作简易消息队列,速度快。为简易起见,摆脱配置文件(就算一定要有,我也埋到镜像里)
python
image_name="registry.cn-hangzhou.aliyuncs.com/YOURS_REP/redis7:v1"
docker run -d --name=p24008_redis\
-v /etc/localtime:/etc/localtime \
-v /etc/timezone:/etc/timezone\
-e "LANG=C.UTF-8"\
-v /data/aprojects/Redis_24008/data:/data\
-p 24008:6379\
${image_name}\
redis-server \
--appendonly yes\
--requirepass YOURPASSWD \
--maxclients 100000
在部署机上执行测试:
python
import redis
lq = redis.Redis(host='172.17.0.1', port=24008, decode_responses=True,password='YOURS')
lq.info()
3.1.2 RedisAgent
python
docker run -d \
--restart=always \
--name=tornado_redis_agent_24118 \
-p 24118:8000 \
-v /etc/localtime:/etc/localtime -v /etc/timezone:/etc/timezone -v /etc/hostname:/etc/hostname -e "LANG=C.UTF-8" -w /workspace \
registry.cn-hangzhou.aliyuncs.com/YOURS/server.andy.tornado_redis_agent_24118:v101 \
sh -c "python3 server.py"
安装基本包
python
wget -NO Basefuncs-1.10-py3-none-any.whl http://YOURS/downup/view008_download_from_folder/pys.Basefuncs-1.10-py3-none-any.whl
测试:
python
from Basefuncs import *
qm = QManager(redis_agent_host = 'http://172.17.0.1:24118/', redis_connection_hash = None)
qm.info()
{'msg': 'ok',
'status': True,
'data': {'cluster_enabled': 0,
'role': 'master',
'total_system_memory_human': '94.28G',
'used_memory_peak_human': '6.02M',
'used_memory_human': '6.02M',
'connected_clients': 1}}
3.1.3 ClickHouse
clickhouse将成为结构化存储库的高效核心
python
docker run -d \
--restart=always \
--name=click_house_server \
--ulimit nofile=262144:262144 \
-e LOG_SIZE=100M -e LOG_COUNT=10 \
-p 18123:8123 \
-p 19000:9000/tcp \
-v /etc/hostname:/etc/hostname \
-e "LANG=C.UTF-8" \
-v /data/clickhouse_19000/data:/var/lib/clickhouse/ \
-v /data/clickhouse_19000/log:/var/log/clickhouse-server/ \
-e CLICKHOUSE_DB=my_database \
-e CLICKHOUSE_USER=YOURS \
-e CLICKHOUSE_DEFAULT_ACCESS_MANAGEMENT=1 \
-e CLICKHOUSE_PASSWORD=YOURS \
clickhouse/clickhouse-server
测试
命令行
python
echo 'SELECT version()' | curl --user USER:PASSWORD 'http://localhost:18123/' --data-binary @-
21.12.3.32
ipython
python
from clickhouse_driver import Client
client = Client(host='127.0.0.1', port=19000, database='my_database',user='YOURS' ,password='PWD')
sql = 'SHOW TABLES'
res = client.execute(sql)
sql = 'show databases'
res = client.execute(sql)
[('INFORMATION_SCHEMA',),
('default',),
('information_schema',),
('my_database',),
('system',)]
创建、写入数据表并读取
python
create_table = '''
CREATE TABLE my_table
(
id UInt32,
name String
)
ENGINE = MergeTree()
ORDER BY id;
'''
from Basefuncs import *
host = '172.17.0.1'
port = 19000
database = 'my_database'
user = 'USER'
password = 'PASSWORD'
name = 'tem'
chc = CHClient(host = host, port = port , database = database, user = user, password = password, name = name )
chc._exe_sql(create_table)
chc._exe_sql('show tables')
data_listofdict = [{'id':1, 'name':'John Doe'},
{'id':2,'name':'Jane Smith'}]
data_df = pd.DataFrame(data_listofdict )
insert_num = chc.insert_df2table('my_table', some_df = data_df, pid_name = 'id', cols = ['id','name'])
获取数据
python
data_listoftuple = chc.get_table('my_table', cols = ['id','name'])
data_df = pd.DataFrame(data_listoftuple, columns =['id','name'] )
id name
0 1 John Doe
1 2 Jane Smith
3.1.4 Flask-Celery
作为基本执行单元,我希望这个服务在宿主机上安装,这样在执行任务的时候除了docker任务,还可以执行基础的脚本任务。我是以systemd的方式执行安装(以及开机自启动的)
项目路径 /opt/flask_celery/server_single_v2.py
要注意:
- 1 如果不是默认的redis数据库,要修改配置
- 2 千万不要用 sys.args位置参数,这会破坏celery本身的命令格式(
celery -A ...
) - 3 另外也感谢踩的坑,我稍微更新了一下celery的启动配置(应该在启动时会重试一下数据库连接,这样可能在整个服务器重启时有用)
python
from flask import Flask,request
from celery import Celery
from celery.result import AsyncResult
from Basefuncs import *
import requests as req
import time
# 如果有定制的redis服务用位置参数传入
celery_broker = 'redis://:YOURS@127.0.0.1:24008/1'
app = Flask(__name__)
# 旧版
# 用以储存消息队列
#app.config['CELERY_BROKER_URL'] = celery_broker
# 用以储存处理结果
#app.config['CELERY_RESULT_BACKEND'] =celery_broker
# 新版
# 用以储存消息队列
app.config['broker_url'] = celery_broker
# 用以储存处理结果
app.config['result_backend'] = celery_broker
# 增加:在启动时重试连接
app.config['broker_connection_retry_on_startup'] =True
# 旧版
# celery_ = Celery(app.name, broker=app.config['CELERY_BROKER_URL'])
# 新版
celery_ = Celery(app.name, broker=app.config['broker_url'])
celery_.conf.update(app.config)
# 后台任务,模拟耗时任务
@celery_.task
def my_background_task(arg1, arg2):
# 两数相加
time.sleep(10)
return arg1+arg2
# 执行任务的路由 GET
@app.route("/sum/<arg1>/<arg2>")
def sum(arg1, arg2):
# 发送任务到celery,并返回任务ID,后续可以根据此任务ID获取任务结果
result = my_background_task.delay(int(arg1), int(arg2))
return result.id
# 执行任务的路由 POST
@app.route("/sum_post/", methods=['GET','POST'] )
def sum_post():
input_data = request.get_json()
arg1 = input_data['arg1']
arg2 = input_data['arg2']
# 发送任务到celery,并返回任务ID,后续可以根据此任务ID获取任务结果
result = my_background_task.delay(int(arg1), int(arg2))
return result.id
# 获取任务结果
@app.route("/get_result/<result_id>")
def get_result(result_id):
# 根据任务ID获取任务结果
result = AsyncResult(id=result_id)
return str(result.get())
# =======================以下是正式的内容
# s2s: stream to stream
@celery_.task
def s2s_handler(cfg_dict = None):
cfg = cfg_dict
# read
source_qm = QManager(batch_size =cfg['source_read_batch_num'],
redis_agent_host = cfg['source_redis_agent_host'],
redis_connection_hash = cfg['source_connection_hash']
)
# write
target_qm = QManager(batch_size =cfg['target_write_batch_num'],
redis_agent_host = cfg['target_redis_agent_host'],
redis_connection_hash = cfg['target_connection_hash']
)
print('source q len ', source_qm.stream_len(cfg['source_stream']))
print('target q len ', target_qm.stream_len(cfg['target_stream']))
for _ in range(cfg['max_exec_cnt']):
if target_qm._is_q_available(cfg['target_stream']):
print('target q ok')
msg_num_limit = min(cfg['source_read_batch_num'],cfg['target_write_batch_num'])
msg_list = source_qm.xrange(cfg['source_stream'], count=msg_num_limit)['data']
if len(msg_list) == 0:
print('source q empty')
break
else:
# 写入目标队列
target_qm.parrallel_write_msg(cfg['target_stream'], data_listofdict= msg_list)
# 将写入的消息从源队列删除
to_del_msg_id_list = source_qm.extract_msg_id(msg_list)
source_qm.xdel(cfg['source_stream'], mid_or_list= to_del_msg_id_list)
else:
break
# 执行任务的路由 POST
@app.route("/s2s/", methods=['GET','POST'] )
def s2s():
input_data = request.get_json()
# 发送任务到celery,并返回任务ID,后续可以根据此任务ID获取任务结果
result = s2s_handler.delay(input_data)
return result.id
# s2ch: stream to clickhouse
@celery_.task
def s2ch_handler(cfg_dict = None):
cfg = cfg_dict
# read from stream
source_qm = QManager(batch_size =cfg['source_read_batch_num'],
redis_agent_host = cfg['source_redis_agent_host'],
redis_connection_hash = cfg['source_connection_hash']
)
# write to clickhouse
target_chc = CHClient(name='tem',host=cfg['target_ch_host'],
port=cfg['target_ch_port'],
database=cfg['target_ch_database'],
user=cfg['target_ch_user'],
password=cfg['target_ch_pwd'],
)
source_q_len = source_qm.stream_len(cfg['source_stream'])
print('source q len ', source_q_len)
for _ in range(cfg['max_exec_cnt']):
msg_list = source_qm.xrange(cfg['source_stream'])['data']
if len(msg_list) == 0:
print('source q empty')
break
else:
# 写入目标数据库
insert_cols = cfg['target_ch_insert_cols']
insert_pid_name = cfg['target_ch_insert_pid_name']
insert_table_name = cfg['target_ch_insert2table_name']
insert_df = pd.DataFrame(msg_list)[insert_cols].dropna()
intert_num = target_chc.insert_df2table(insert_table_name, some_df = insert_df, cols = insert_cols, pid_name = insert_pid_name)
# 当成功插入时,才删除队列中的消息
if intert_num > 0:
# 将写入的消息从源队列删除
to_del_msg_id_list = source_qm.extract_msg_id(msg_list)
source_qm.xdel(cfg['source_stream'], mid_or_list= to_del_msg_id_list)
# 执行任务的路由 POST
@app.route("/s2ch/", methods=['GET','POST'] )
def s2ch():
input_data = request.get_json()
# 发送任务到celery,并返回任务ID,后续可以根据此任务ID获取任务结果
result = s2ch_handler.delay(input_data)
return result.id
目前写了两类固定的celery任务,整个功能将作为服务发布,使用接口调用。如果宿主机没有python环境,那么可以安装miniconda,再安装一系列的包。常见的包如下:
python
pip3 install ipython -i https://mirrors.aliyun.com/pypi/simple/
pip3 install requests -i https://mirrors.aliyun.com/pypi/simple/
pip3 install clickhouse_driver -i https://mirrors.aliyun.com/pypi/simple/
pip3 install pandas -i https://mirrors.aliyun.com/pypi/simple/
pip3 install numpy -i https://mirrors.aliyun.com/pypi/simple/
pip3 install redis -i https://mirrors.aliyun.com/pypi/simple/
pip3 install pydantic -i https://mirrors.aliyun.com/pypi/simple/
pip3 install nest_asyncio -i https://mirrors.aliyun.com/pypi/simple/
pip3 install aiohttp -i https://mirrors.aliyun.com/pypi/simple/
pip3 install Flask -i https://mirrors.aliyun.com/pypi/simple/
pip3 install Flask-APScheduler -i https://mirrors.aliyun.com/pypi/simple/
pip3 install celery -i https://mirrors.aliyun.com/pypi/simple/
pip3 install gunicorn -i https://mirrors.aliyun.com/pypi/simple/
pip3 install mongoengine -i https://mirrors.aliyun.com/pypi/simple/
pip3 install apscheduler -i https://mirrors.aliyun.com/pypi/simple/
pip3 install tornado -i https://mirrors.aliyun.com/pypi/simple/
pip3 install Pillow -i https://mirrors.aliyun.com/pypi/simple/
pip3 install markdown -i https://mirrors.aliyun.com/pypi/simple/
pip3 install pymysql -i https://mirrors.aliyun.com/pypi/simple/
pip3 install gevent -i https://mirrors.aliyun.com/pypi/simple/
然后可以试着在环境下调起服务
- 1 启动flask
python
gunicorn server_single_v2:app -b 0.0.0.0:24104
[2024-06-10 22:01:42 +0800] [182895] [INFO] Starting gunicorn 22.0.0
[2024-06-10 22:01:42 +0800] [182895] [INFO] Listening at: http://0.0.0.0:24104 (182895)
[2024-06-10 22:01:42 +0800] [182895] [INFO] Using worker: sync
[2024-06-10 22:01:42 +0800] [182896] [INFO] Booting worker with pid: 182896
- 2 启动celery
python
celery -A server_single_v2.celery_ worker
└─ $ celery -A server_single_v2.celery_ worker
/root/anaconda3/lib/python3.11/site-packages/celery/platforms.py:829: SecurityWarning: You're running the worker with superuser privileges: this is
absolutely not recommended!
Please specify a different user using the --uid option.
User information: uid=0 euid=0 gid=0 egid=0
warnings.warn(SecurityWarning(ROOT_DISCOURAGED.format(
-------------- celery@tccy2sls.vm v5.4.0 (opalescent)
--- ***** -----
-- ******* ---- Linux-5.15.0-60-generic-x86_64-with-glibc2.35 2024-06-11 09:59:36
- *** --- * ---
- ** ---------- [config]
- ** ---------- .> app: server_single_v2:0x7f5068c41690
- ** ---------- .> transport: redis://:**@127.0.0.1:24008/1
- ** ---------- .> results: redis://:**@127.0.0.1:24008/1
- *** --- * --- .> concurrency: 12 (prefork)
-- ******* ---- .> task events: OFF (enable -E to monitor tasks in this worker)
--- ***** -----
-------------- [queues]
.> celery exchange=celery(direct) key=celery
- 3 配置systemd自启动
创建启动脚本 cd ~ && vim start_flask_celery.sh
,在当前环境下(anaconda3)默认会启动基础环境,我暂时不研究了,理论上应该在脚本中先激活环境再执行。
注意:启动脚本中的 #!/bin/bash
不可少。
python
#!/bin/bash
# miniconda 环境
#source /root/miniconda3/etc/profile.d/conda.sh
# 激活 base 环境(或你创建的特定环境)
#conda activate base
#!/bin/bash
#anaconda环境
# 运行 Python 服务脚本
cd /opt/flask_celery
nohup gunicorn server_single_v2:app -b 0.0.0.0:24104 >/dev/null 2>&1 &
nohup celery -A server_single_v2.celery_ worker >/dev/null 2>&1 &
将脚本改为(任何用户)可执行
chmod +x ~/start_flask_celery.sh
注册服务vim /lib/systemd/system/flask_celery.service
,同样的,本次注释掉环境部分
python
[Unit]
Description=flask_celery_service
After=network.target network-online.target syslog.target
Wants=network.target network-online.target
[Service]
#启动服务的命令
Type=forking
ExecStart=/root/start_flask_celery.sh
Restart=always
RestartSec=5
# miniconda
#Environment="PATH=/root/miniconda3/bin:/usr/local/sbin:/usr/local/bin:/usr/sbin:/usr/bin:/sbin:/bin"
# anaconda
Environment="PATH=/root/anaconda3/bin:/usr/local/sbin:/usr/local/bin:/usr/sbin:/usr/bin:/sbin:/bin"
[Install]
WantedBy=multi-user.target
启动并设置为开机自启动
python
systemctl daemon-reload
systemctl start flask_celery
systemctl enable flask_celery
失败时杀掉进程
python
ps aux | grep 24104
ps aux | grep celery_
查看状态
bash
● flask_celery.service - flask_celery_service
Loaded: loaded (/lib/systemd/system/flask_celery.service; enabled; vendor preset: enabled)
Active: active (running) since Tue 2024-06-11 11:48:15 CST; 30s ago
Process: 194723 ExecStart=/root/start_flask_celery.sh (code=exited, status=0/SUCCESS)
Tasks: 33 (limit: 115619)
Memory: 774.6M
CPU: 3.210s
CGroup: /system.slice/flask_celery.service
├─194724 /root/anaconda3/bin/python /root/anaconda3/bin/gunicorn server_single_v2:app -b 0.0.0.0:24104
├─194725 /root/anaconda3/bin/python /root/anaconda3/bin/celery -A server_single_v2.celery_ worker
├─194727 /root/anaconda3/bin/python /root/anaconda3/bin/gunicorn server_single_v2:app -b 0.0.0.0:24104
├─194747 /root/anaconda3/bin/python /root/anaconda3/bin/celery -A server_single_v2.celery_ worker
├─194748 /root/anaconda3/bin/python /root/anaconda3/bin/celery -A server_single_v2.celery_ worker
├─194749 /root/anaconda3/bin/python /root/anaconda3/bin/celery -A server_single_v2.celery_ worker
├─194750 /root/anaconda3/bin/python /root/anaconda3/bin/celery -A server_single_v2.celery_ worker
├─194751 /root/anaconda3/bin/python /root/anaconda3/bin/celery -A server_single_v2.celery_ worker
├─194752 /root/anaconda3/bin/python /root/anaconda3/bin/celery -A server_single_v2.celery_ worker
├─194753 /root/anaconda3/bin/python /root/anaconda3/bin/celery -A server_single_v2.celery_ worker
├─194754 /root/anaconda3/bin/python /root/anaconda3/bin/celery -A server_single_v2.celery_ worker
├─194755 /root/anaconda3/bin/python /root/anaconda3/bin/celery -A server_single_v2.celery_ worker
├─194756 /root/anaconda3/bin/python /root/anaconda3/bin/celery -A server_single_v2.celery_ worker
├─194757 /root/anaconda3/bin/python /root/anaconda3/bin/celery -A server_single_v2.celery_ worker
└─194758 /root/anaconda3/bin/python /root/anaconda3/bin/celery -A server_single_v2.celery_ worker
Jun 11 11:48:15 tccy2sls.vm systemd[1]: Starting flask_celery_service...
Jun 11 11:48:15 tccy2sls.vm systemd[1]: Started flask_celery_service.
- 4 flask-aps
为了方便起见,也将这个服务封装到镜像里。
先通过miniconda3构造一个基础容器
python
docker run -it --name=p24010_supervisor_build \
-v /etc/localtime:/etc/localtime -v /etc/timezone:/etc/timezone -v /etc/hostname:/etc/hostname -e "LANG=C.UTF-8" -w /workspace \
registry.cn-hangzhou.aliyuncs.com/YOURS/andy.base.miniconda3:v100 \
bash
考入文件并挪个位置
python
docker cp /opt/aprojects/SimpleAPI_v1_Supervisor_24010 p24010_supervisor_build:/workspace/
cd /workspace/SimpleAPI_v1_Supervisor_24010
mv * ..
补充安装一些和时间相关的包
python
sudo ln -sf /usr/share/zoneinfo/Asia/Shanghai /etc/localtime
echo "Asia/Shanghai" | sudo tee /etc/timezone
wget -NO make_a_request.py http://YOURS/downup/view008_download_from_folder/pys.make_a_request.py
将任务发布
python
import requests as req
host_port = '127.0.0.1:5555'
data = {}
data['func_name'] = 'make_a_request'
with open('./make_a_request.py', 'r') as f:
data['func_body'] = f.read()
resp = req.post('http://%s/add_task_type/' % host_port, json=data)
print(resp.text)
将镜像提交
docker commit p24010_supervisor_build registry.cn-hangzhou.aliyuncs.com/YOURS/andy.base.miniconda3:v101
docker push registry.cn-hangzhou.aliyuncs.com/YOURS/andy.base.miniconda3:v101
这样在远端服务器时就可以简单启动了
python
# 测试态
docker run -it --name=p24010_supervisor \
-v /etc/localtime:/etc/localtime -v /etc/timezone:/etc/timezone -v /etc/hostname:/etc/hostname -e "LANG=C.UTF-8" -w /workspace \
-p 24010:5555 \
registry.cn-hangzhou.aliyuncs.com/YOURS/andy.base.miniconda3:v101 \
bash
# 启动服务
python3 entry_py.py
>>>当前环境变量 test
>>>当前环境变量 None
Note:运行环境变量不可为空(以位置参数附加在entry_sh.sh 启动)
ITASK Current Slot 28635770
* Serving Flask app 'entry_py'
* Debug mode: on
WARNING: This is a development server. Do not use it in a production deployment. Use a production WSGI server instead.
* Running on all addresses (0.0.0.0)
* Running on http://127.0.0.1:5555
* Running on http://172.17.0.10:5555
Press CTRL+C to quit
* Restarting with stat
>>>当前环境变量 test
>>>当前环境变量 None
Note:运行环境变量不可为空(以位置参数附加在entry_sh.sh 启动)
ITASK Current Slot 28635770
* Debugger is active!
* Debugger PIN: 145-660-447
采用守护态启动
python
docker run -d --name=p24010_supervisor \
--restart=always \
-v /etc/localtime:/etc/localtime -v /etc/timezone:/etc/timezone -v /etc/hostname:/etc/hostname -e "LANG=C.UTF-8" -w /workspace \
-p 24010:5555 \
registry.cn-hangzhou.aliyuncs.com/YOURS/andy.base.miniconda3:v101 \
sh -c "sh entry_sh.sh test"
1ed1ff7761ca0a60aab64b0d36f06160231f5a101185ab2f93b6cee76c3202fa
建立队列进行测试,task004将专门用于服务的搭建测试。
python
from Basefuncs import QManager
# wan
cfg ={
'target_q_max_len': 100000,'source_read_batch_num':10,'target_write_batch_num':10,
'source_redis_agent_host':'http://172.17.0.1:24118/','source_connection_hash':None,
'target_redis_agent_host':'http://172.17.0.1:24118/','target_connection_hash':None,
'source_stream':'BUFF.andy.test0.stream_out',
'target_stream':'BUFF.andy.test1.stream_in',
'max_exec_cnt':10}
# read
source_qm = QManager(batch_size =cfg['source_read_batch_num'],
redis_agent_host = cfg['source_redis_agent_host'],
redis_connection_hash = cfg['source_connection_hash']
)
# write
target_qm = QManager(batch_size =cfg['target_write_batch_num'],
redis_agent_host = cfg['target_redis_agent_host'],
redis_connection_hash = cfg['target_connection_hash']
)
source_qm.ensure_group(cfg['source_stream'])
target_qm.ensure_group(cfg['target_stream'])
# debug - 样例数据写入源队列
data_listofdict = [{'msg_id': i, 'data':'test'} for i in range(1000)]
source_qm.parrallel_write_msg(cfg['source_stream'], data_listofdict= data_listofdict)
print('source q len ', source_qm.stream_len(cfg['source_stream']))
print('target q len ', target_qm.stream_len(cfg['target_stream']))
启动服务,这部分还没有精简,看起来比较多。里面糅杂了几部分内容:
- 1 使用WFlaskAPS来控制flask-aps的接口
- 2 使用FlaskAPSTask来实现状态机
- 3 TaskTable是基于Mongoengine的对象
python
# part1
from Basefuncs import *
from datetime import datetime
from typing import List, Optional
from pydantic import BaseModel
import requests as req
# part2
import pandas as pd
# part3
from mongoengine import connect, Document, StringField, IntField,DictField
# 操作Flask apscheduler
class WFlaskAPS(BaseModel):
flask_aps_agent:str = 'xxxx:24010'
# 获取当前的任务列表
def get_jobs(self):
url = 'http://%s/get_jobs' % self.flask_aps_agent
return req.get(url).json()
# 删除某个任务
def remove_a_task(self, task_name = None):
url = 'http://%s/remove_a_task/' % self.flask_aps_agent
data_dict ={}
data_dict['task_id'] = task_name
return req.post(url, json=data_dict).json()
# 获取任务状态
def get_jobs_status(self):
url = 'http://%s/get_jobs_status/' % self.flask_aps_agent
return req.get(url).json()
# 发布一个任务(task)
# task_name 其实是job type
# task_id 任务的唯一编号
# task_type 原来的服务只实现了cron方式,因为cron方式可以实现其他两种方式。
'''
date:在指定的日期和时间运行一次
interval:在指定时间间隔内运行
cron:使用Cron表达式运行
'''
def publish_a_task(self,task_id= None ,task_name = None, task_type ='cron', task_kwargs = {},
year = None, month = None, day = None, week = None, day_of_week = None,
hour = None,minute = None, second = None, start_date = None, end_date = None,
):
url = 'http://%s/publish_a_task/' % self.flask_aps_agent
data_dict = {'task_id':task_id,
'task_name':task_name,
'task_type':task_type,
'task_kwargs':task_kwargs,
'year':year,
'month':month,
'day':day,
'week':week,
'day_of_week':day_of_week,
'hour':hour,
'minute':minute,
'second':second,
'start_date':start_date,
'end_date':end_date
}
return req.post(url, json=data_dict).json()
def pause_a_task(self, task_id = None):
data_dict = {}
url = 'http://%s/pause_a_task/' % self.flask_aps_agent
data_dict['task_id'] = task_id
return req.post(url, json=data_dict).json()
def resume_a_task(self, task_id = None):
data_dict = {}
data_dict['task_id'] = task_id
url = 'http://%s/resume_a_task/' % self.flask_aps_agent
return req.post(url, json=data_dict).json()
# task_name
def add_a_job(self, fpath = None, func_name = None):
url = 'http://%s/add_task_type/' % self.flask_aps_agent
data = {}
data['func_name'] = func_name
with open(fpath, 'r') as f:
data['func_body'] = f.read()
return req.post(url, json=data).json()
import re
def is_standard_time_format(time_str=None):
# 定义正则表达式
pattern = r'^\d{4}-\d{2}-\d{2} \d{2}:\d{2}:\d{2}$'
# 使用正则表达式匹配输入的字符串
match = re.match(pattern, time_str)
# 返回匹配结果
return bool(match)
class FlaskAPSTask:
def get_method_name(self):
method_name = inspect.stack()[1].function
return method_name
@staticmethod
def get_sources(some_df = None, some_trigger = None):
the_souce_list = sorted(list(some_df[some_df['trigger'] == some_trigger]['source']))
return the_souce_list
@staticmethod
def is_ok_to_take_action(ele , elist):
if '*' in elist:
return True
return ele in elist
def __init__(self, state = 'init', transitions = None, wflask = None,task_id = None ):
self.state = state
self.transition_df = pd.DataFrame(transitions)
self.wflask = wflask
# 在初始化时,自动获取最新的状态
self.task_id = task_id
self.state = self.get_a_task_status(task_id)
# 省略了一些cron的时间参数
def publish(self, task_id = None, task_name = None, task_kwargs =None ,start_date = None, end_date = None , day = None, hour = None, minute = None, second = None):
print('Publish a task')
# 必须确保开始时间
if is_standard_time_format(str(start_date)):start_date = start_date
if is_standard_time_format(str(end_date)):end_date = end_date
start_date = start_date or get_time_str1()
end_date = end_date or '2999-01-01 00:00:00'
self.wflask.publish_a_task(task_id = task_id,
task_name= task_name,
task_kwargs=task_kwargs,
start_date=start_date,
end_date = end_date,
day = day,
hour = hour,
minute = minute,
second =second)
self.state = 'running'
def pause(self, task_id = None):
print('Pause a task')
self.wflask.pause_a_task(task_id = task_id)
self.state = 'paused'
def delete(self, task_id = None):
print('Delete A Task')
self.wflask.remove_a_task(task_name = task_id)
self.state = 'init'
def resume(self, task_id = None):
print('Resume A Task')
self.wflask.resume_a_task(task_id = task_id)
self.state = 'running'
# 在每一个回合, FlaskAPSTask都会回写当前的状态,这样就会形成一个规则链
# 1 当 running_status 为空时,暂不执行任何操作
# 2 当 set_to_status 为running , 而running_status 为init时,执行publish
# 3 当set_to_status 为running ,而 running_status 为 paused 时,执行 resume
# 4 当set_to_status=paused, 而 running_status 为 running 时,执行 pause
# 5 当set_to_status = init , 如果 running_status 为running和pause时,都执行delete
def action_rule(self,task_obj = None):
set_to_status = task_obj.set_to_status
if set_to_status == self.state:
print('set_to_status:%s same as running' % set_to_status)
print('set to status', set_to_status, ' current state ', self.state)
if self.state == 'running':
if set_to_status == 'init':
self.delete(task_id = task_obj.task_id)
elif set_to_status == 'paused':
self.pause(task_id = task_obj.task_id)
if self.state == 'paused':
if set_to_status == 'running':
self.resume(task_id = task_obj.task_id)
elif set_to_status == 'init':
self.delete(task_id = task_obj.task_id)
if self.state == 'init':
if set_to_status == 'running':
_task_id = task_obj.task_id
_task_name = task_obj.job_name
_start_date = the_task_obj.start_dt
_end_date = the_task_obj.end_dt
_interval_para = the_task_obj.interval_para
_task_kwargs = the_task_obj.task_kwargs
if not isinstance(_task_kwargs, dict):_task_kwargs =None
self.publish(task_id = _task_id, task_name = _task_name, start_date = _start_date, end_date = _end_date,task_kwargs= _task_kwargs,**_interval_para)
return True
# 获取活动的任务列表
def _get_running_job_status(self):
return self.wflask.get_jobs_status()['data']
# 获取总的任务列表
def _get_total_job_list(self):
return self.wflask.get_jobs()['data']
def get_a_task_status(self, task_id = None):
## 当前活动中的tasks
running_job_status = self._get_running_job_status()
if len(running_job_status):
running_job_list = sorted(list(running_job_status.keys()))
else:
running_job_list = []
## 全部的tasks(包括被暂停的)
total_job_list =self._get_total_job_list()
return self._current_task_status(some_task_id = task_id, running_tasks = running_job_status, all_tasks = total_job_list)
@staticmethod
def _current_task_status(some_task_id = None ,running_tasks = None, all_tasks = None):
is_task_in_running = some_task_id in running_tasks
is_task_in_total = some_task_id in all_tasks
if is_task_in_running:
return 'running'
if is_task_in_total and not is_task_in_running:
return 'paused'
return 'init'
class TaskTable(Document):
# pid = machine.task_id
pid = StringField(required=True,primary_key=True)
machine = StringField(required=True)
task_id = StringField(required=True)
description = StringField()
job_name = StringField()
set_to_status = StringField()
running_status = StringField()
interval_para = DictField()
start_dt = StringField()
end_dt = StringField()
task_kwargs = DictField()
is_enable = IntField(default = 1)
create_time = StringField()
update_time = StringField()
python
flask_aps_agent = '127.0.0.1:24010'
machine = 'm999'
flask_aps_db = 'flask_aps'
# 定义状态机的转换规则
transitions = [
{'trigger': 'publish', 'source': 'init', 'dest': 'running'},
{'trigger': 'pause', 'source': 'running', 'dest': 'paused'},
{'trigger': 'delete', 'source': '*', 'dest': 'init'},
{'trigger': 'resume', 'source': 'paused', 'dest': 'running'},
]
# 定义状态机的状态
states = ['init', 'running', 'paused']
# 初始化连接对象
wf = WFlaskAPS(flask_aps_agent = flask_aps_agent)
# Left: 从myemta读取
# 1. 连接,简单起见并不区分lan和wan
connect(
host='YOURS Mongo' % flask_aps_db
)
通过任务对象来初始化、转换状态并同步状态
python
def exe_a_task(the_task_obj):
the_task_fsm = FlaskAPSTask(transitions = transitions, wflask=wf, task_id = the_task_obj.task_id)
the_task_fsm.action_rule(the_task_obj)
current_task_status = the_task_fsm.get_a_task_status(task_id = the_task_obj.task_id)
return the_task_obj.update(set__update_time=get_time_str1(), set__running_status =current_task_status)
执行
python
the_task_obj = TaskTable.objects(machine='m999',task_id ='task004').first()
exe_a_task(the_task_obj)
In [12]: wf.get_jobs_status()
Out[12]:
{'data': {'task004': {'next_run_time': '2024-06-11 23:58:40',
'pending': False}},
'duration': 0,
'msg': 'ok',
'name': 'get_jobs_status',
'status': True}
4 结语
总算把这部分搞完了,实在有点长。总体上,实现了较为便捷的搭建方式,即使是新主机上也可以很快的部署配置。
用:
- 1 我会基于此,构造一个随时可启动的Stream,方便后续的逻辑接入
- 2 对于某一项具体的工程,肯定是先构造数据流模型,然后使用这部分工具完成默认的连接。Stream2Stream通常可以用于跨主机间的数据共享,而 Stream2ClickHouse肯定是比较重要的一种数据持久化。之后还需要补充一些,例如Stream2Mongo,或者反过来,Mongo2Stream。