KKB_2020.tif
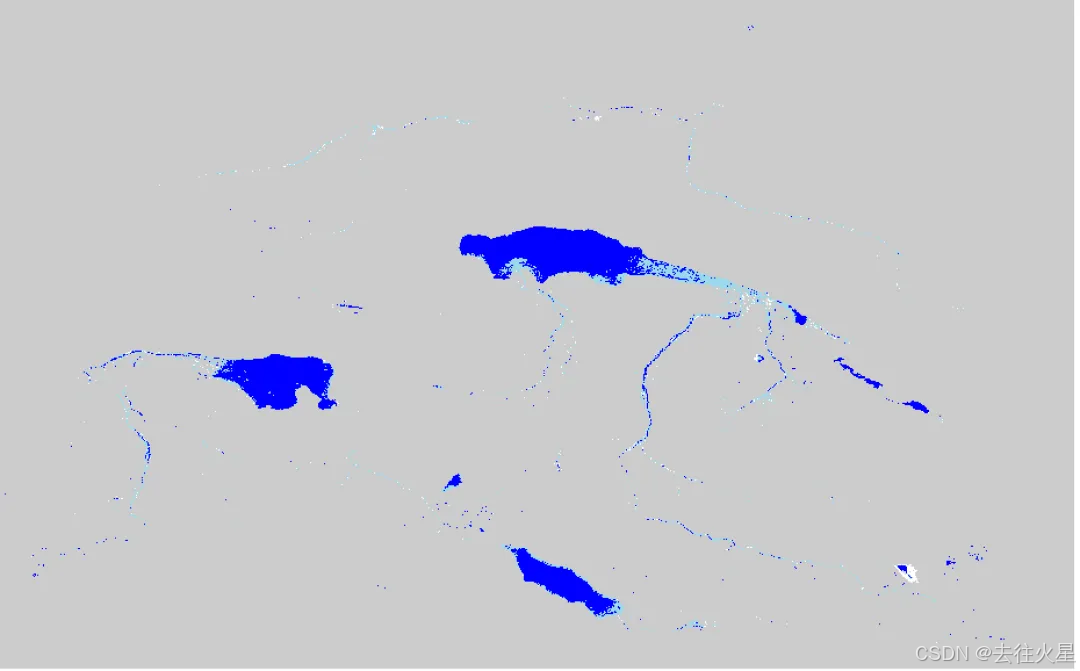
KKB_2020_JRC.tif
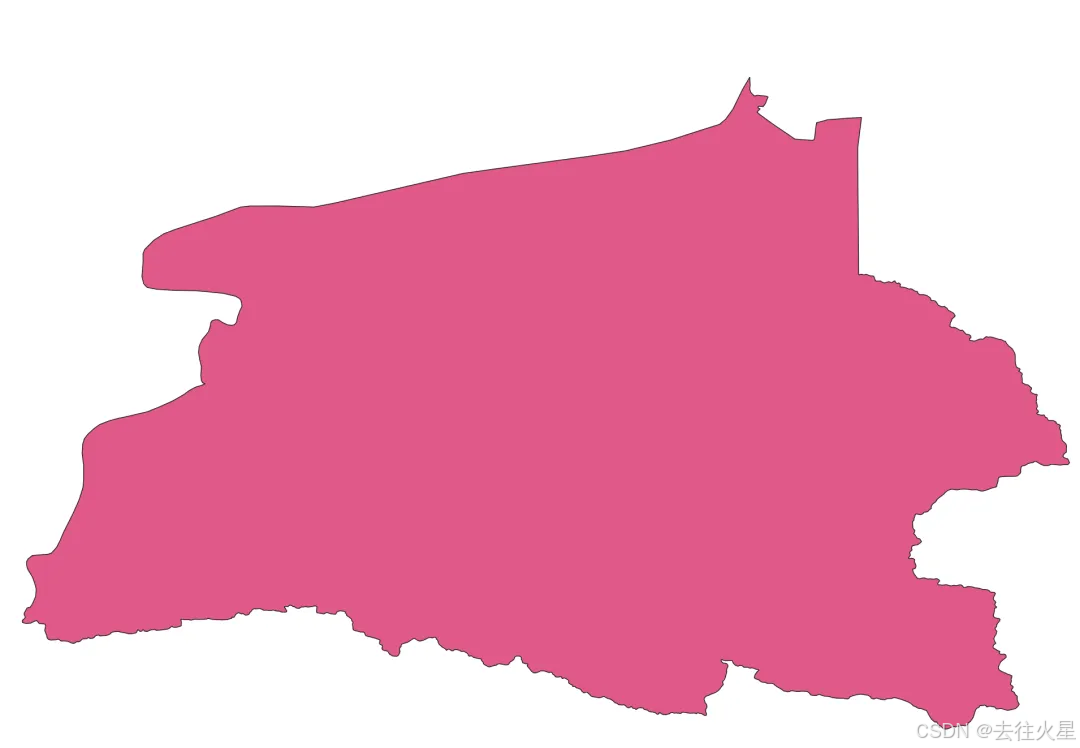
kkb.geojson
所用到的包:(我嫌geopandas安装太麻烦colab做的。。
cpp
import rasterio
import geopandas as gpd
import numpy as np
import pandas as pd
import matplotlib.pyplot as plt
from sklearn.metrics import confusion_matrix, ConfusionMatrixDisplay
from rasterio.mask import mask
定义所需要的函数
cpp
# 裁剪栅格数据的函数
def clip_raster(raster_file, geojson):
with rasterio.open(raster_file) as src:
out_image, out_transform = mask(src, geojson.geometry, crop=True)
out_meta = src.meta.copy()
out_meta.update({"driver": "GTiff",
"height": out_image.shape[1],
"width": out_image.shape[2],
"transform": out_transform})
return out_image[0], out_meta
# 将tif转为二分类
def convert_to_binary(data):
return np.where(data != 0, 1, 0) # Non-zero values represent water
# 创建对比图,0为背景值,1为正确值
def create_comparison_map(true_data, predicted_data):
comparison_map = np.zeros_like(true_data)
comparison_map[(true_data == 1) & (predicted_data == 1)] = 1 # Correct identification
comparison_map[(true_data == 0) & (predicted_data == 0)] = 0 # Background value
comparison_map[(true_data == 1) & (predicted_data == 0)] = 2 # Missed identification
comparison_map[(true_data == 0) & (predicted_data == 1)] = 3 # Over identification
return comparison_map
# 计算精度
def calculate_accuracy_metrics(confusion_matrix):
accuracy = np.diag(confusion_matrix).sum() / confusion_matrix.sum()
producer_accuracy = np.diag(confusion_matrix) / confusion_matrix.sum(axis=1)
user_accuracy = np.diag(confusion_matrix) / confusion_matrix.sum(axis=0)
return accuracy, producer_accuracy, user_accuracy
# 保存tif
def save_raster(data, meta, filename):
meta.update({"count": 1})
with rasterio.open(filename, 'w', **meta) as dst:
dst.write(data, 1)
加载数据并按研究区裁剪,目的是不让背景值影响计算,并将tif转为2分类,最后调用sklearn计算混淆矩阵。
cpp
# 加载数据
file_kkb_2020 = '/content/KKB_2020.tif'
file_kkb_2020_jrc = '/content/KKB_2020_JRC.tif'
file_geojson = '/content/kkb.geojson'
geojson = gpd.read_file(file_geojson)
# 裁剪tif
kkb_2020, meta = clip_raster(file_kkb_2020, geojson)
kkb_2020_jrc, meta = clip_raster(file_kkb_2020_jrc, geojson)
# 转化为0,1值
kkb_2020_binary = convert_to_binary(kkb_2020) # Non-NaN values represent water
kkb_2020_jrc_binary = convert_to_binary(kkb_2020_jrc) # Non-zero values represent water
# 调用sklearn方法计算混淆矩阵
cm = confusion_matrix(kkb_2020_binary.flatten(), kkb_2020_jrc_binary.flatten())
print(cm)
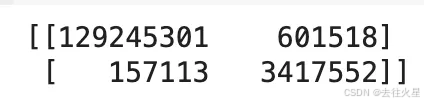
展示混淆矩阵与对比图,结果如下图所示
cpp
# 展示混淆矩阵
disp = ConfusionMatrixDisplay(confusion_matrix=cm, display_labels=["No Water", "Water"])
disp.plot()
plt.title('Confusion Matrix')
plt.show()
# 创建对比图
comparison_map = create_comparison_map(kkb_2020_binary, kkb_2020_jrc_binary)
# 展示对比图
plt.imshow(comparison_map, cmap='viridis', interpolation='nearest')
plt.colorbar()
plt.title('Comparison Map')
plt.show()
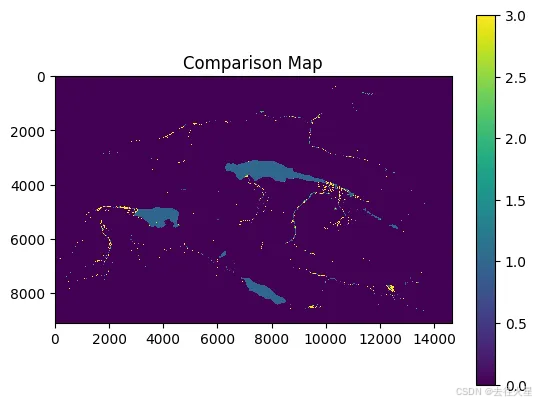
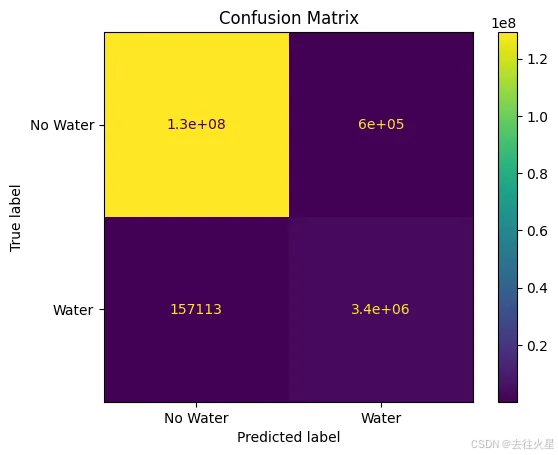
计算精度指标与保存对比图
cpp
# 计算精度指标
accuracy, producer_accuracy, user_accuracy = calculate_accuracy_metrics(cm)
accuracy_metrics = pd.DataFrame({
'Metric': ['Overall Accuracy', 'Producer Accuracy', 'User Accuracy'],
'Value': [accuracy, producer_accuracy.mean(), user_accuracy.mean()]
})
# 保存对比图
meta.update({"count": 1})
with rasterio.open('/content/comparison_map.tif', 'w', **meta) as dst:
dst.write(comparison_map, 1)
print(accuracy_metrics)
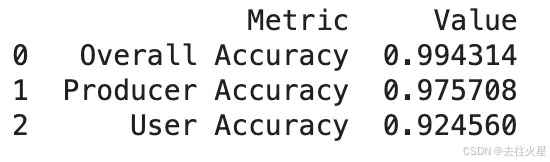
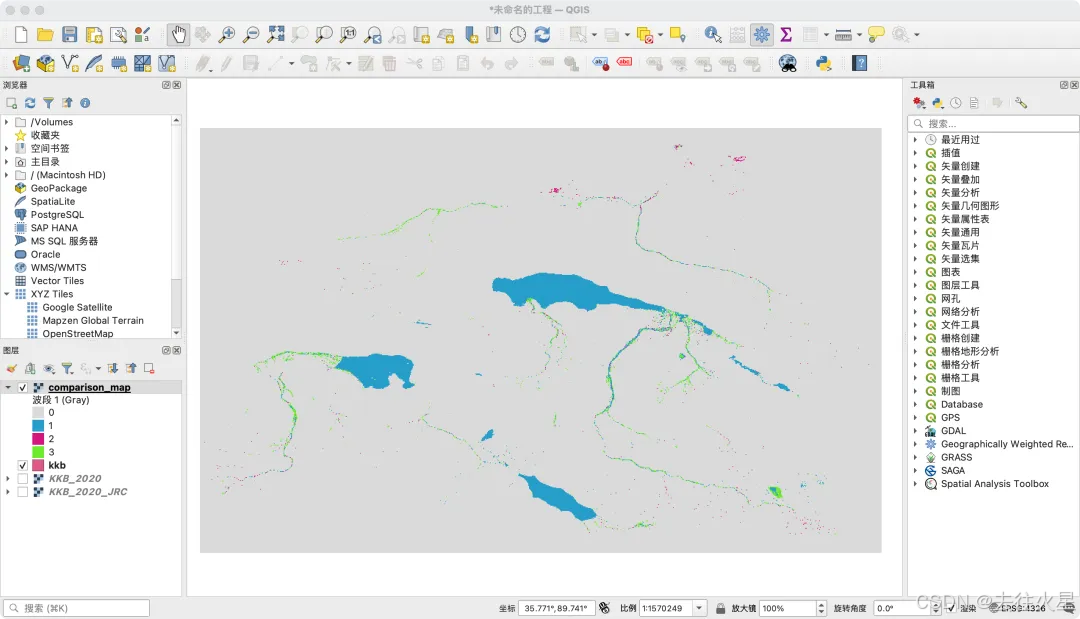
数据与代码存放在:
https://drive.google.com/drive/folders/1ECbBZTlPlLPNiBkA67GCbCf_6otl83jN?usp=sharing
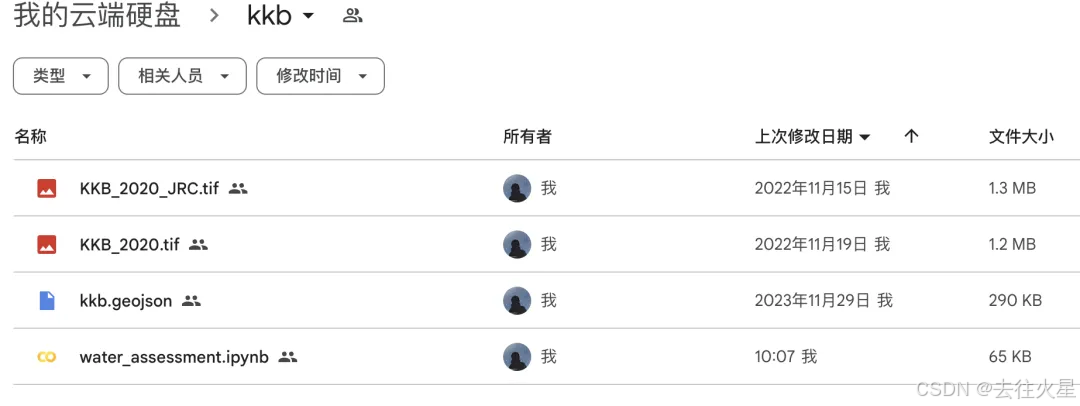