LSTM+CRF序列标注
我们希望得到这个模型来对词进行标注,B是开始,I是实体词的非开始,O是非实体词。
我们首先需要lstm对序列里token的记忆,和计算每个token发到crf的分数,发完了再退出来,最后形成1模型。那么细节我就不感兴趣,直接说训练数据
训练数据就是这样被空格所分开,然后就可以去训练。
word_to_idx[word] = len(word_to_idx)
把这些词都放到词表,每个字来一个数字对应
,他们的目标值label也就是B,I,O,对应的数字0,1,2
grad_fn = ms.value_and_grad(model, None, optimizer.parameters)
表示得到梯度函数,None是不需要指定参数标签。
由于要求导,所以grad_fn 的3个参数都是tensor
每次前进一步
train_step 每次训练完,model的权重就有了,可以预测
这样就使用了。预测了BIO
再查字典,翻译为B,I,O
RNN实现情感分类
def http_get(url: str, temp_file: IO):
"""使用requests库下载数据,并使用tqdm库进行流程可视化"""
req = requests.get(url, stream=True)
content_length = req.headers.get('Content-Length')
total = int(content_length) if content_length is not None else None
progress = tqdm(unit='B', total=total)
for chunk in req.iter_content(chunk_size=1024):
if chunk:
progress.update(len(chunk))
temp_file.write(chunk)
progress.close()
def download(file_name: str, url: str):
"""下载数据并存为指定名称"""
if not os.path.exists(cache_dir):
os.makedirs(cache_dir)
cache_path = os.path.join(cache_dir, file_name)
cache_exist = os.path.exists(cache_path)
if not cache_exist:
with tempfile.NamedTemporaryFile() as temp_file:
http_get(url, temp_file)
temp_file.flush()
temp_file.seek(0)
with open(cache_path, 'wb') as cache_file:
shutil.copyfileobj(temp_file, cache_file)
return cache_path
这2哥代码非常有用,我先记下来,可以把url的文件下到临时目录
数据进行拆分
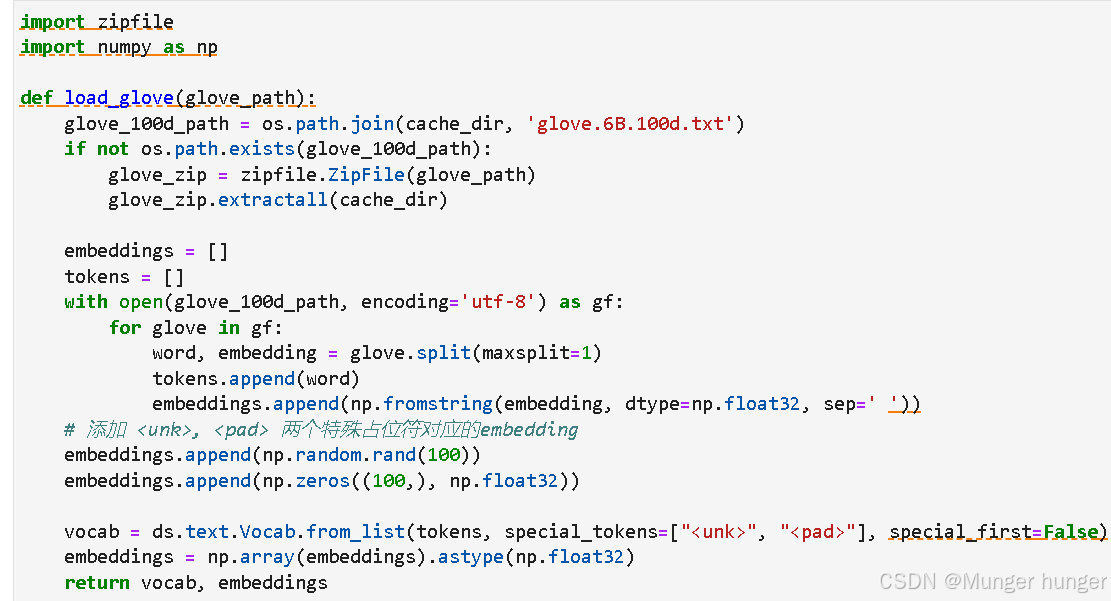
glove.6B.100d.txt 这个词表可以对词做embedding
embeddings.append(np.random.rand(100))
embeddings.append(np.zeros((100,), np.float32))
让embeddings 有可能的所有向量
glove_path = download('glove.6B.zip', 'https://mindspore-website.obs.myhuaweicloud.com/notebook/datasets/glove.6B.zip')
vocab, embeddings = load_glove(glove_path)
len(vocab.vocab())
拿到真正的模型,去得到扩展了的模型
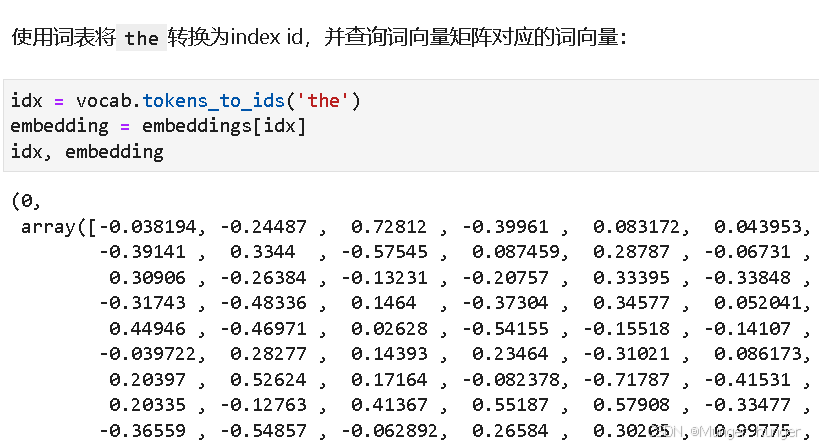
id是0,第一个词。对应的向量是100纬度
经过一系列的操作,把文本和标签分贝好
由于RNN的循环特性,和自然语言文本的序列特性(句子是由单词组成的序列)十分匹配,因此被大量应用于自然语言处理研究中。下图为RNN的结构拆解
RNN也有梯度消失,就有了lstm来解决这个问题
RNN的结构如上。
最后输出是正向的还是负向的影视评论,所以纬度是1
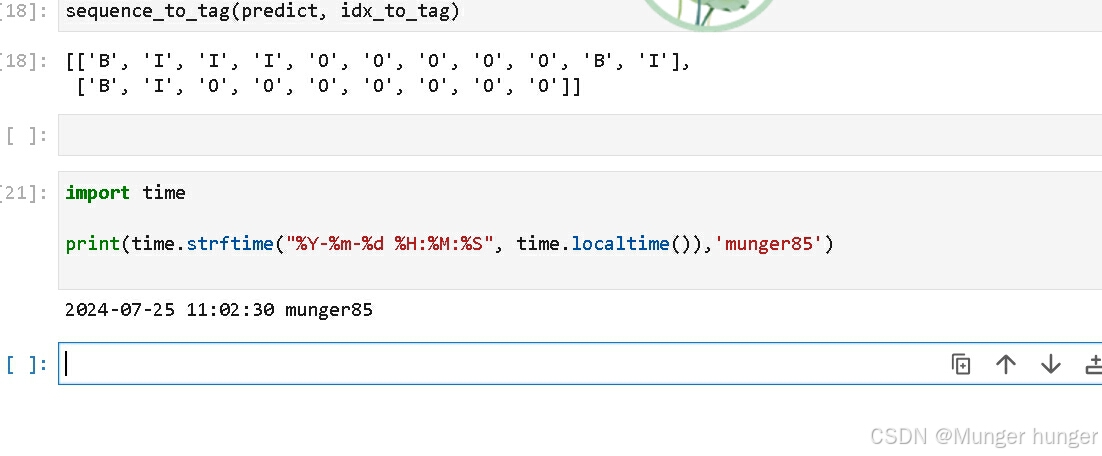