摘要
Machine learning models(机器学习模型) are vulnerable(容易受到) to adversarial examples(对抗样本). For the black-box setting(对于黑盒设置), current substitute attacks(目前替代攻击) need pre-trained models(预训练模型) to generate adversarial examples(生成对抗样本). However, pre-trained models(预训练模型) are hard to obtain(很难获得) in real-world tasks(在实际任务中). In this paper, we propose a data-free substitute training method(无数据替代训练方法) (DaST) to obtain substitute models(获得替代模型) for adversarial black-box attacks(对抗性黑盒攻击) without the requirement of any real data(不需要任何真实数据). To achieve this, DaST utilizes specially designed(专门设计) generative adversarial networks(生成对抗网络) (GANs) to train the substitute models. In particular(特别地), we design a multi-branch architecture(多分支架构) and label-control loss(标签控制损失) for the generative model to deal with(处理) the uneven distribution(不均匀分布) of synthetic samples(生成样本). The substitute model is then trained by the synthetic samples(合成样本) generated by the generative model(生成模型), which are labeled by the attacked model subsequently(随后). The experiments demonstrate(实验表明) the substitute models produced by DaST can achieve competitive performance(达到有竞争力的性能) compared with the baseline models(基线模型) which are trained by the same train set(相同的训练集) with attacked models(攻击模型). Additionally(此外), to evaluate the practicability(评估实用性) of the proposed method(所提出的方法) on the real-world task(在现实世界任务), we attack an online machine learning model(在线机器学习模型) on the Microsoft Azure platform. The remote model(远程模型) misclassifies(错误分类) 98.35% of the adversarial examples crafted(制作) by our method. To the best of our knowledge(据我们所知), we are the first(第一个) to train a substitute model for adversarial attacks(对抗样本) without any real data(没有任何真实数据).
方法
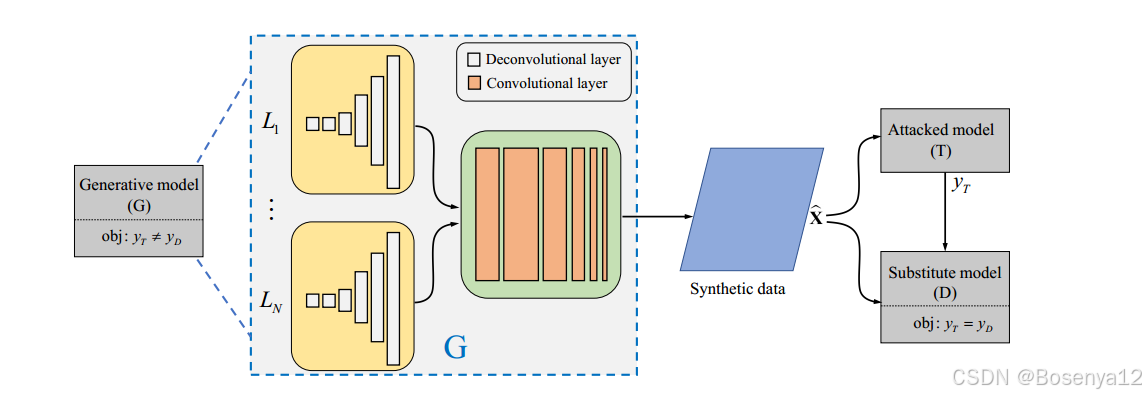
总结
We have presented(提出) a data-free method DaST to train substitute models(替代模型) for adversarial attacks(对抗性攻击). DaST reduces(降低) the prerequisites(先决条件) of adversarial substitute attacks(对抗性攻击) by utilizing(利用) GANs to generate synthetic samples(生成合成样本). This is the first method that can train substitute models without the requirement of any real data(不需要任何真实数据). The experiments showed(实验表明) the effectiveness(有效性) of our method. It presented(表明) that machine learning systems have significant risks(存在重大风险), attackers can train substitute models even when the real input data is hard to collect(即使难以手机真实的输入数据).
The proposed DaST cannot generate adversarial examples alone(不能单独生成对抗性样本), it should be used with other gradient-based attack methods(应该与其他基于梯度的攻击方法一起使用). In future work, we will design a new substitute training method, which can generate attacks directly(直接). Furthermore, we will explore(探索) the defense for DaST.