2022.DKE.Anomaly explanation: A review
- paper
- [explanation by feature importance](#explanation by feature importance)
-
- [main idea](#main idea)
- [Non-weighted feature importance](#Non-weighted feature importance)
- [explanation by feature values](#explanation by feature values)
-
- [main idea](#main idea)
- [explanation by data points comparison](#explanation by data points comparison)
-
- [main idea](#main idea)
- [explanation by structure analysis](#explanation by structure analysis)
-
- [main idea](#main idea)
paper
explanation by feature importance
main idea
to explain that anomaly to the user, we can just say that attribute f1 contributed to the abnormality of the square data point.
Non-weighted feature importance
1999.VLDB.Finding intensional knowledge of distance-based outliers
- define the outlier categories C = {"trivial outlier," "weak outlier," "strongest outlier"} to help gain better insights about the nature of outliers.
explanation by feature values
main idea
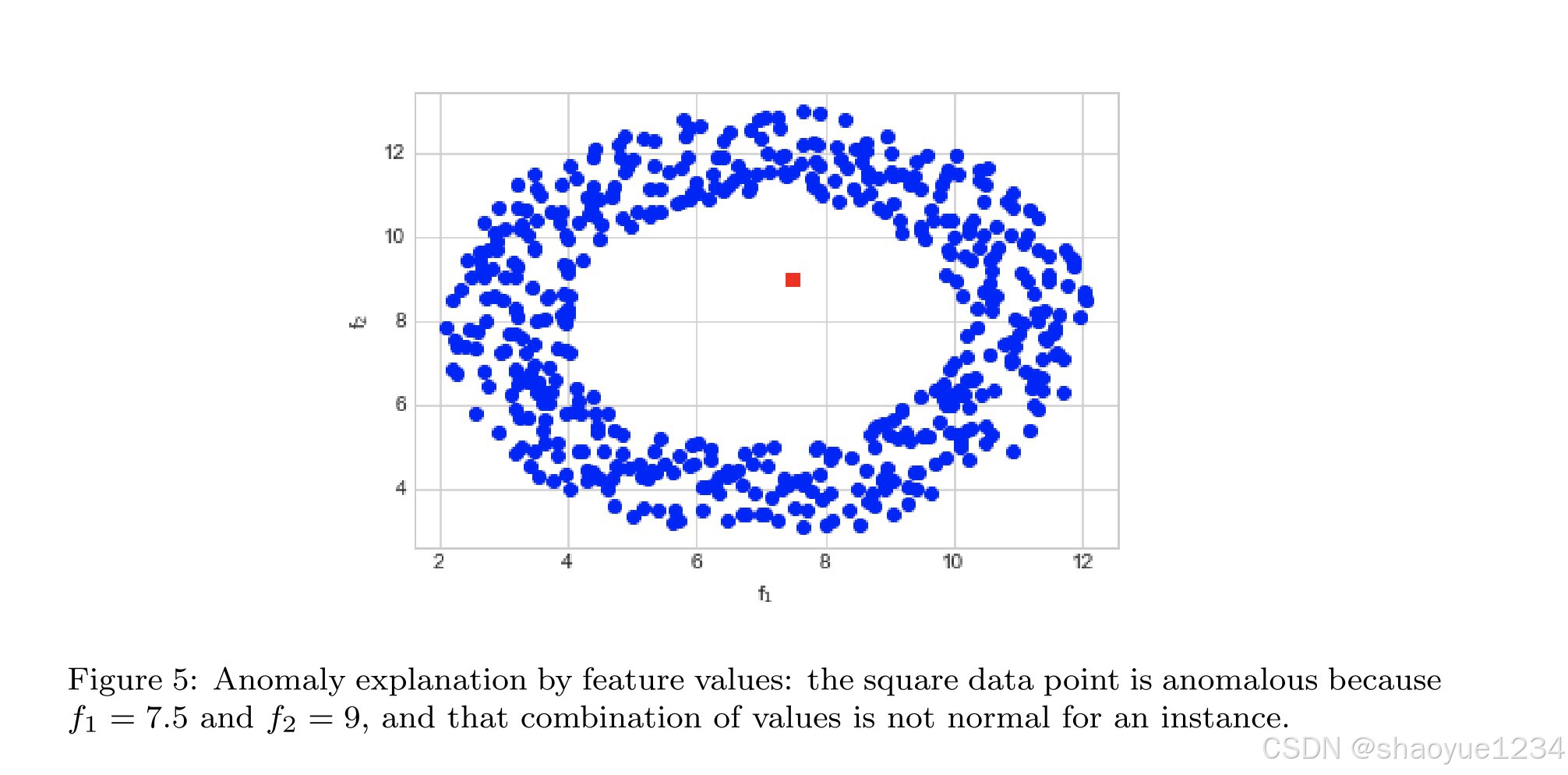
explanation by data points comparison
main idea
what is the difference between anomalies and regular data points.
explanation by structure analysis
main idea
x1 and x2 are anomalies for the cluster of round instances and why it is the case, that y is an anomaly for the the triangles and why, and finally that z is an anomaly
for the squares and why.