文章目录
图像的几何变换
目标
- 学习将不同的几何变换应用于图像,如平移、旋转、仿射变换等。
您将看到这些函数:cv.getPerspectiveTransform
变换
OpenCV 提供了两个变换函数,cv.warpAffine 和 cv.warpPerspective ,您可以使用它们执行各种变换。cv.warpAffine 采用 2x3 变换矩阵,而 cv.warpPerspective 采用 3x3 变换矩阵作为输入。
缩放
缩放只是调整图像的大小。 OpenCV 自带了一个函数 cv.resize() 用于此目的。可以手动指定图像的大小,也可以指定缩放因子。使用不同的插值方法。首选插值方法是 cv.INTER_AREA 用于缩小和 cv.INTER_CUBIC (慢速)和 cv.INTER_LINEAR 用于缩放。默认情况下,插值方法 cv.INTER_LINEAR 用于所有调整大小目的。您可以使用以下任一方法调整输入图像的大小:
python
import numpy as np
import cv2 as cv
img = cv.imread('messi5.jpg')
res = cv.resize(img,None,fx=2, fy=2, interpolation = cv.INTER_CUBIC)
#OR
height, width = img.shape[:2]
res = cv.resize(img,(2*width, 2*height), interpolation = cv.INTER_CUBIC)
平移
平移是物体位置的移动。如果您知道 (x,y) 方向的偏移量,并将其设为 ( t x , t y ) (t_x,t_y) (tx,ty),则可以按如下方式创建变换矩阵 M \textbf{M} M:
M = \[ 1 0 t x 0 1 t y \] \] \[M = \\begin{bmatrix} 1 \& 0 \& t_x \\\\ 0 \& 1 \& t_y \\end{bmatrix}\] \[M=\[1001txty\]
您可以将其转换为 np.float32 类型的 Numpy 数组,并将其传递给 cv.warpAffine() 函数。请参阅以下 (100,50) 偏移量的示例:
python
import numpy as np
import cv2 as cv
img = cv.imread('messi5.jpg',0)
rows,cols = img.shape
M = np.float32([[1,0,100],[0,1,50]])
dst = cv.warpAffine(img,M,(cols,rows))
cv.imshow('img',dst)
cv.waitKey(0)
cv.destroyAllWindows()
警告
cv.warpAffine() 函数的第三个参数是输出图像的大小,应采用 (width, height) 的形式。请记住,宽度 = 列数,高度 = 行数。
参见以下结果:
旋转
图像旋转角度 θ \theta θ 是通过以下形式的变换矩阵实现的
M = \[ c o s θ − s i n θ s i n θ c o s θ \] \] \[M = \\begin{bmatrix} cos\\theta \& -sin\\theta \\\\ sin\\theta \& cos\\theta \\end{bmatrix}\] \[M=\[cosθsinθ−sinθcosθ\]
但 OpenCV 提供了可调整旋转中心的缩放旋转,因此您可以在任何您喜欢的位置进行旋转。修改后的变换矩阵由以下公式给出
\[ α β ( 1 − α ) ⋅ c e n t e r . x − β ⋅ c e n t e r . y − β α β ⋅ c e n t e r . x + ( 1 − α ) ⋅ c e n t e r . y \] \] \[\\begin{bmatrix} \\alpha \& \\beta \& (1- \\alpha ) \\cdot center.x - \\beta \\cdot center.y \\\\ - \\beta \& \\alpha \& \\beta \\cdot center.x + (1- \\alpha ) \\cdot center.y \\end{bmatrix}\] \[\[α−ββα(1−α)⋅center.x−β⋅center.yβ⋅center.x+(1−α)⋅center.y\]
其中:
α = s c a l e ⋅ cos θ , β = s c a l e ⋅ sin θ \] \[\\begin{array}{l} \\alpha = scale \\cdot \\cos \\theta , \\\\ \\beta = scale \\cdot \\sin \\theta \\end{array}\] \[α=scale⋅cosθ,β=scale⋅sinθ
为了找到这个变换矩阵,OpenCV 提供了一个函数 cv.getRotationMatrix2D。查看下面的示例,该示例将图像相对于中心旋转 90 度,没有任何缩放。
python
img = cv.imread('messi5.jpg',0)
rows,cols = img.shape
# cols-1 and rows-1 are the coordinate limits.
M = cv.getRotationMatrix2D(((cols-1)/2.0,(rows-1)/2.0),90,1)
dst = cv.warpAffine(img,M,(cols,rows))
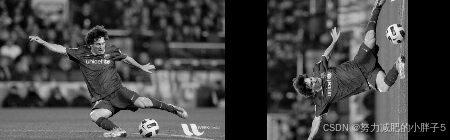
仿射变换
在仿射变换中,原始图像中的所有平行线在输出图像中仍将是平行的。要找到变换矩阵,我们需要输入图像中的三个点及其在输出图像中的对应位置。然后 cv.getAffineTransform 将创建一个 2x3 矩阵,该矩阵将传递给 cv.warpAffine。
查看以下示例,并查看我选择的点(标记为绿色):
python
img = cv.imread('drawing.png')
rows,cols,ch = img.shape
pts1 = np.float32([[50,50],[200,50],[50,200]])
pts2 = np.float32([[10,100],[200,50],[100,250]])
M = cv.getAffineTransform(pts1,pts2)
dst = cv.warpAffine(img,M,(cols,rows))
plt.subplot(121),plt.imshow(img),plt.title('Input')
plt.subplot(122),plt.imshow(dst),plt.title('Output')
plt.show()
查看结果:
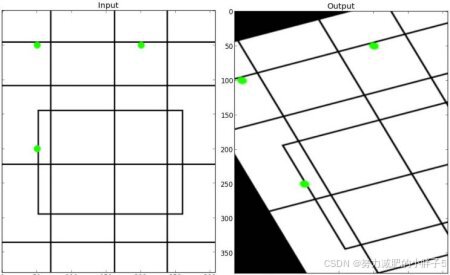
透视变换
对于透视变换,您需要一个 3x3 变换矩阵。直线在变换后仍将保持笔直。要找到此变换矩阵,您需要输入图像上的 4 个点和输出图像上的对应点。在这 4 个点中,其中 3 个不应共线。然后可以通过函数 cv.getPerspectiveTransform 找到变换矩阵。然后使用这个 3x3 变换矩阵应用cv.warpPerspective。
请参阅以下代码:
python
img = cv.imread('sudoku.png')
rows,cols,ch = img.shape
pts1 = np.float32([[56,65],[368,52],[28,387],[389,390]])
pts2 = np.float32([[0,0],[300,0],[0,300],[300,300]])
M = cv.getPerspectiveTransform(pts1,pts2)
dst = cv.warpPerspective(img,M,(300,300))
plt.subplot(121),plt.imshow(img),plt.title('Input')
plt.subplot(122),plt.imshow(dst),plt.title('Output')
plt.show()
结果:
其他资源
- "计算机视觉:算法与应用",Richard Szeliski