说一些坑,本来之前准备用milvus,但是发现win搞不了(docker都配好了)。然后转头搞chromadb。这里面还有就是embedding一般都是本地部署,但我电脑是cpu的没法玩,我就选了jina的embedding性能较优(也可以换glm的embedding但是要改代码)。最后问题出在deepseek与llamaindex的适配,因为采用openai的接口,这里面改了openai库的源码然后对llamaindex加了配置项才完全跑通。国内小伙伴如果使用我这套方案可以抄,给我点个赞谢谢。
主要环境:
bash
os:win11
python3.10
llamaindex 0.11.20
chromadb 0.5.15
这个文件是官方例子,自己弄个也成
源码如下:
python
# %%
from llama_index.core import VectorStoreIndex, SimpleDirectoryReader
from llama_index.vector_stores.chroma import ChromaVectorStore
from llama_index.core import StorageContext
from llama_index.embeddings.huggingface import HuggingFaceEmbedding
from IPython.display import Markdown, display
from llama_index.llms.openai import OpenAI
import chromadb
# %%
import openai
openai.api_key = "sk"
openai.api_base = "https://api.deepseek.com/v1"
llm = OpenAI(model='deepseek-chat',api_key=openai.api_key, base_url=openai.base_url)
from llama_index.core import Settings
# llm = OpenAI(api_key=openai.api_key, base_url=openai.base_url)
Settings.llm = OpenAI(model="deepseek-chat",api_key=openai.api_key, base_url=openai.base_url)
# %%
import os
jinaai_api_key = "jina"
os.environ["JINAAI_API_KEY"] = jinaai_api_key
from llama_index.embeddings.jinaai import JinaEmbedding
text_embed_model = JinaEmbedding(
api_key=jinaai_api_key,
model="jina-embeddings-v3",
# choose `retrieval.passage` to get passage embeddings
task="retrieval.passage",
)
# %%
# create client and a new collection
chroma_client = chromadb.EphemeralClient()
chroma_collection = chroma_client.create_collection("quickstart")
# %%
# define embedding function
embed_model = text_embed_model
# load documents
documents = SimpleDirectoryReader("./data/paul_graham/").load_data()
# save to disk
db = chromadb.PersistentClient(path="./chroma_db")
chroma_collection = db.get_or_create_collection("quickstart")
vector_store = ChromaVectorStore(chroma_collection=chroma_collection)
storage_context = StorageContext.from_defaults(vector_store=vector_store)
index = VectorStoreIndex.from_documents(
documents, storage_context=storage_context, embed_model=embed_model
)
# load from disk
db2 = chromadb.PersistentClient(path="./chroma_db")
chroma_collection = db2.get_or_create_collection("quickstart")
vector_store = ChromaVectorStore(chroma_collection=chroma_collection)
index = VectorStoreIndex.from_vector_store(
vector_store,
embed_model=embed_model,
)
# Query Data from the persisted index
query_engine = index.as_query_engine()
response = query_engine.query("What did the author do growing up?")
print('response:',response)
1.llamaindex如何配置deepseek
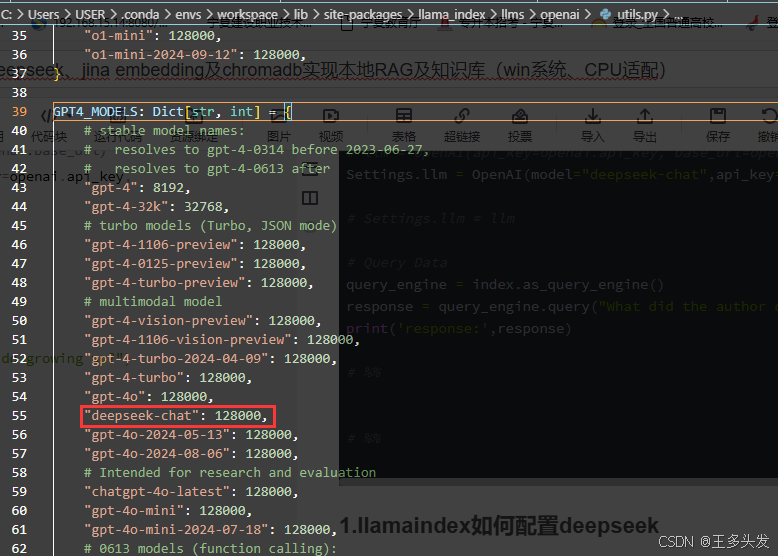
找到llama_index下面的openai的utils配置里,加入"deepseek-chat":128000,
路径C:\Users\USER.conda\envs\workspace\lib\site-packages\llama_index\llms\openai\utils.py
python
from llama_index.llms.openai import OpenAI
llm = OpenAI(model="deepseek-chat", base_url="https://api.deepseek.com/v1", api_key="sk-")
response = llm.complete("见到你很高兴")
print(str(response))
2.llama使用jina
python
# Initilise with your api key
import os
jinaai_api_key = "jina_"
os.environ["JINAAI_API_KEY"] = jinaai_api_key
from llama_index.embeddings.jinaai import JinaEmbedding
text_embed_model = JinaEmbedding(
api_key=jinaai_api_key,
model="jina-embeddings-v3",
# choose `retrieval.passage` to get passage embeddings
task="retrieval.passage",
)
embeddings = text_embed_model.get_text_embedding("This is the text to embed")
print("Text dim:", len(embeddings))
print("Text embed:", embeddings[:5])
query_embed_model = JinaEmbedding(
api_key=jinaai_api_key,
model="jina-embeddings-v3",
# choose `retrieval.query` to get query embeddings, or choose your desired task type
task="retrieval.query",
# `dimensions` allows users to control the embedding dimension with minimal performance loss. by default it is 1024.
# A number between 256 and 1024 is recommended.
dimensions=512,
)
embeddings = query_embed_model.get_query_embedding(
"This is the query to embed"
)
print("Query dim:", len(embeddings))
print("Query embed:", embeddings[:5])
3.llamaindex 使用chromadb
python
# %%
from llama_index.core import VectorStoreIndex, SimpleDirectoryReader
from llama_index.vector_stores.chroma import ChromaVectorStore
from llama_index.core import StorageContext
from llama_index.embeddings.huggingface import HuggingFaceEmbedding
from IPython.display import Markdown, display
from llama_index.llms.openai import OpenAI
import chromadb
# %%
import openai
openai.api_key = "sk-"
openai.api_base = "https://api.deepseek.com/v1"
from llama_index.core import Settings
# llm = OpenAI(api_key=openai.api_key, base_url=openai.base_url)
Settings.llm = OpenAI(model="deepseek-chat",api_key=openai.api_key, base_url=openai.base_url)
# %%
import os
jinaai_api_key = "jina_"
os.environ["JINAAI_API_KEY"] = jinaai_api_key
from llama_index.embeddings.jinaai import JinaEmbedding
text_embed_model = JinaEmbedding(
api_key=jinaai_api_key,
model="jina-embeddings-v3",
# choose `retrieval.passage` to get passage embeddings
task="retrieval.passage",
)
# %%
# create client and a new collection
chroma_client = chromadb.EphemeralClient()
chroma_collection = chroma_client.create_collection("quickstart")
# %%
# define embedding function
embed_model = text_embed_model
# load documents
documents = SimpleDirectoryReader("./data/paul_graham/").load_data()
# %%
# set up ChromaVectorStore and load in data
vector_store = ChromaVectorStore(chroma_collection=chroma_collection)
# %%
storage_context = StorageContext.from_defaults(vector_store=vector_store)
# %%
index = VectorStoreIndex.from_documents(
documents, storage_context=storage_context, embed_model=embed_model
)
# Settings.llm = llm
# Query Data
query_engine = index.as_query_engine()
response = query_engine.query("What did the author do growing up?")
print('response:',response)