- 🍨 本文为 🔗365天深度学习训练营中的学习记录博客
- 🍖 原作者: K同学啊
1.检查GPU
import torch
import torch.nn as nn
import matplotlib.pyplot as plt
import torchvision
device=torch.device("cuda" if torch.cuda.is_available() else "cpu")
device
2.查看数据
import os,PIL,random,pathlib
data_dir='data/45-data/'
data_dir=pathlib.Path(data_dir)
data_paths=list(data_dir.glob('*'))
classNames=[str(path).split('\\')[2] for path in data_paths]
classNames
3.划分数据集
python
total_datadir='data/45-data/'
train_trainsforms=transforms.Compose([
transforms.Resize([224,224]),
transforms.ToTensor(),
transforms.Normalize(
mean=[0.485, 0.456, 0.406],
std=[0.229, 0.224, 0.225]
),
])
total_data=datasets.ImageFolder(total_datadir,train_trainsforms)
total_data
import torch.utils
train_size=int(0.8*len(total_data))
test_size=len(total_data)-train_size
train_dataset,test_dataset=torch.utils.data.random_split(total_data,[train_size,test_size])
train_dataset,test_dataset
import torch.utils.data
import torch.utils.data.dataloader
batch_size=32
train_dl=torch.utils.data.DataLoader(train_dataset,
batch_size,
shuffle=True,
num_workers=1)
test_dl=torch.utils.data.DataLoader(test_dataset,
batch_size,
shuffle=True,
num_workers=1)
for X,y in test_dl:
print('shape of X [N C H W]',X.shape)
print('shape of y:',y.shape)
break

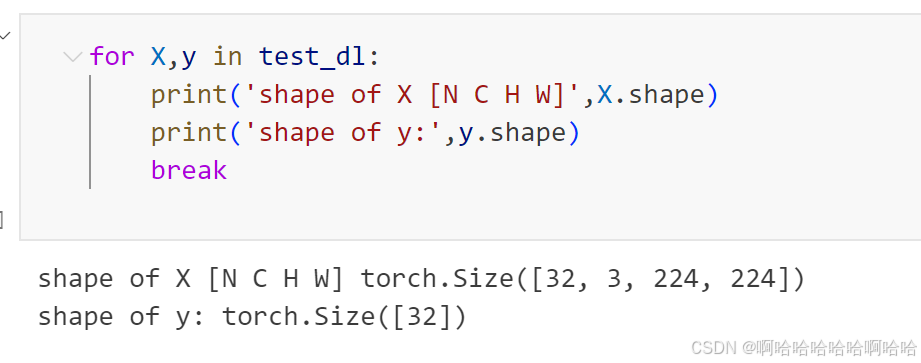
4.构建模型
python
import torch.nn.functional as F
class Network_bn(nn.Module):
def __init__(self):
super(Network_bn, self).__init__()
self.conv1 = nn.Conv2d(in_channels=3, out_channels=12, kernel_size=5, stride=1, padding=0)
self.bn1 = nn.BatchNorm2d(12)
self.conv2 = nn.Conv2d(in_channels=12, out_channels=12, kernel_size=5, stride=1, padding=0)
self.bn2 = nn.BatchNorm2d(12)
self.pool1 = nn.MaxPool2d(2,2)
self.conv4 = nn.Conv2d(in_channels=12, out_channels=24, kernel_size=5, stride=1, padding=0)
self.bn4 = nn.BatchNorm2d(24)
self.conv5 = nn.Conv2d(in_channels=24, out_channels=24, kernel_size=5, stride=1, padding=0)
self.bn5 = nn.BatchNorm2d(24)
self.pool2 = nn.MaxPool2d(2,2)
self.fc1 = nn.Linear(24*50*50, len(classNames))
def forward(self, x):
x = F.relu(self.bn1(self.conv1(x)))
x = F.relu(self.bn2(self.conv2(x)))
x = self.pool1(x)
x = F.relu(self.bn4(self.conv4(x)))
x = F.relu(self.bn5(self.conv5(x)))
x = self.pool2(x)
x = x.view(-1, 24*50*50)
x = self.fc1(x)
return x
device = "cuda" if torch.cuda.is_available() else "cpu"
print("Using {} device".format(device))
model = Network_bn().to(device)
model
5.编译及训练模型
python
loss_fn=nn.CrossEntropyLoss()
learn_rate=1e-3
opt=torch.optim.SGD(model.parameters(),lr=learn_rate)
def train(dataloader, model, loss_fn, optimizer):
size = len(dataloader.dataset)
num_batches = len(dataloader)
train_loss, correct = 0, 0
model.train()
for X, y in dataloader:
X, y = X.to(device), y.to(device)
pred = model(X)
loss = loss_fn(pred, y)
optimizer.zero_grad()
loss.backward()
optimizer.step()
train_loss += loss.item()
correct += (pred.argmax(1) == y).type(torch.float).sum().item()
train_loss /= num_batches
train_acc = correct / size
return train_acc, train_loss
def test(dataloader, model, loss_fn):
size = len(dataloader.dataset)
num_batches = len(dataloader)
model.eval()
test_loss, correct = 0, 0
with torch.no_grad():
for imgs, target in dataloader:
imgs, target = imgs.to(device), target.to(device)
target_pred = model(imgs)
loss = loss_fn(target_pred, target)
test_loss += loss.item()
correct += (target_pred.argmax(1) == target).type(torch.float).sum().item()
test_loss /= num_batches
test_acc = correct / size
return test_acc, test_loss
def save_best_model(model, best_acc, current_acc, path='best_model.pth'):
if current_acc > best_acc:
best_acc = current_acc
torch.save(model.state_dict(), path)
print(f"Best model saved with accuracy: {best_acc*100:.2f}%")
return best_acc
epochs = 20
best_test_acc = 0.0
train_losses = []
train_accuracies = []
test_losses = []
test_accuracies = []
for epoch in range(epochs):
model.train()
epoch_train_acc, epoch_train_loss = train(train_dl, model, loss_fn, opt)
model.eval()
epoch_test_acc, epoch_test_loss = test(test_dl, model, loss_fn)
# 保存最佳模型
best_test_acc = save_best_model(model, best_test_acc, epoch_test_acc)
# 存储结果用于绘图
train_losses.append(epoch_train_loss)
train_accuracies.append(epoch_train_acc)
test_losses.append(epoch_test_loss)
test_accuracies.append(epoch_test_acc)
print(f'Epoch:{epoch+1:2d}, Train_acc:{epoch_train_acc*100:.1f}%, Train_loss:{epoch_train_loss:.3f}, '
f'Test_acc:{epoch_test_acc*100:.1f}%, Test_loss:{epoch_test_loss:.3f}')
print('Finished Training')
6.结果可视化
python
import matplotlib.pyplot as plt
# 绘制训练和测试的损失与准确率变化趋势
plt.figure(figsize=(12, 5))
# 绘制损失变化趋势
plt.subplot(1, 2, 1)
plt.plot(range(1, epochs + 1), train_losses, label='Train Loss')
plt.plot(range(1, epochs + 1), test_losses, label='Test Loss', linestyle='--')
plt.xlabel('Epoch')
plt.ylabel('Loss')
plt.title('Loss over Epochs')
plt.legend()
# 绘制准确率变化趋势
plt.subplot(1, 2, 2)
plt.plot(range(1, epochs + 1), [acc * 100 for acc in train_accuracies], label='Train Accuracy')
plt.plot(range(1, epochs + 1), [acc * 100 for acc in test_accuracies], label='Test Accuracy', linestyle='--')
plt.xlabel('Epoch')
plt.ylabel('Accuracy (%)')
plt.title('Accuracy over Epochs')
plt.legend()
plt.tight_layout()
plt.show()
7.加载本地模型并预测本地图片
python
from torch.utils.data import DataLoader
def load_best_model_and_predict(image_path, model, transform=None):
# 加载最佳模型
model.load_state_dict(torch.load('best_model.pth'))
model.eval()
# 对单张图片进行预测
if transform is None:
transform = transforms.Compose([
transforms.Resize((224, 224)), # 根据模型需求调整尺寸
transforms.ToTensor(),
])
image = datasets.ImageFolder(image_path, transform=transform)
image_loader = DataLoader(image, batch_size=1, shuffle=False)
with torch.no_grad():
for img, _ in image_loader:
img = img.to(device)
output = model(img)
_, predicted = torch.max(output, 1)
print(f'Predicted class: {predicted.item()}')
break # 我们只预测一张图片
return output,predicted
# 加载最佳模型并预测本地图片
image_path = 'data/猴痘预测'
output,predicted=load_best_model_and_predict(image_path, model)
print(output)
print(predicted)
总结:
1.保存最优模型参数到本地
python
def save_best_model(model, best_acc, current_acc, path='best_model.pth'):
if current_acc > best_acc:
best_acc = current_acc
torch.save(model.state_dict(), path)
print(f"Best model saved with accuracy: {best_acc*100:.2f}%")
return best_acc
2.使用本地模型参数预测本地图片
python
from torch.utils.data import DataLoader
def load_best_model_and_predict(image_path, model, transform=None):
# 加载最佳模型
model.load_state_dict(torch.load('best_model.pth'))
model.eval()
# 对单张图片进行预测
if transform is None:
transform = transforms.Compose([
transforms.Resize((224, 224)), # 根据模型需求调整尺寸
transforms.ToTensor(),
])
image = datasets.ImageFolder(image_path, transform=transform)
image_loader = DataLoader(image, batch_size=1, shuffle=False)
with torch.no_grad():
for img, _ in image_loader:
img = img.to(device)
output = model(img)
_, predicted = torch.max(output, 1)
print(f'Predicted class: {predicted.item()}')
break # 我们只预测一张图片
return output,predicted
# 加载最佳模型并预测本地图片
image_path = 'data/猴痘预测'
output,predicted=load_best_model_and_predict(image_path, model)
print(output)
print(predicted)