一、PINN简介
PINN是一种利用神经网络求解偏微分方程的方法,其计算流程图如下图所示,这里以下方偏微分方程为例:
神经网络输入位置x和时间t的值,预测偏微分方程解u在这个时空条件下的数值解。
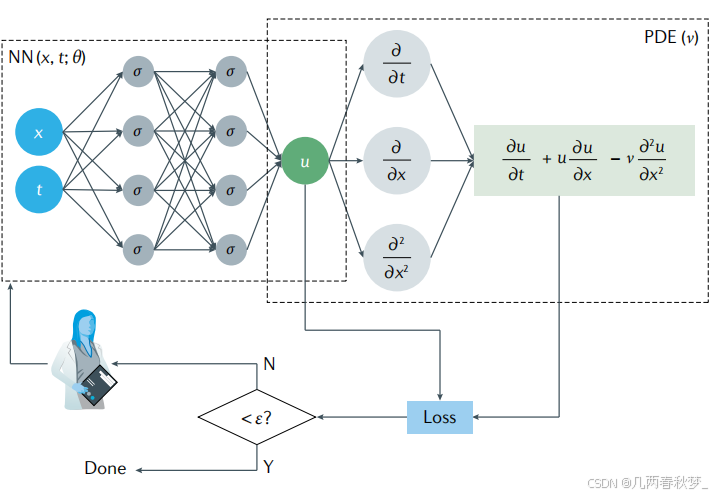
由上图可知,PINN的损失函数包含两部分内容,一部分来源于训练数据误差,另一部分来源于偏微分方程误差,可以记作以下公式:
其中,
二、偏微分方程实践
考虑偏微分方程如下:
考虑一下边界条件
以上偏微分方程真解为,在区域[0,1] × [0,1]上随机采样配置点和数据点,其中配置点用来构造PDE损失函数。
三、基于Pytroch实现代码
python
import torch
import matplotlib.pyplot as plt
from mpl_toolkits.mplot3d import Axes3D
epochs = 2000 # 训练代数
h = 100 # 画图网格密度
N = 1000 # 内点配置点数
N1 = 100 # 边界点配置点数
N2 = 1000 # PDE数据点
def setup_seed(seed):
torch.manual_seed(seed)
torch.cuda.manual_seed_all(seed)
torch.backends.cudnn.deterministic = True
# 设置随机数种子
setup_seed(888888)
# Domain and Sampling
def interior(n=N):
# 内点
x = torch.rand(n, 1)
y = torch.rand(n, 1)
cond = (2 - x ** 2) * torch.exp(-y)
return x.requires_grad_(True), y.requires_grad_(True), cond
def down_yy(n=N1):
# 边界 u_yy(x,0)=x^2
x = torch.rand(n, 1)
y = torch.zeros_like(x)
cond = x ** 2
return x.requires_grad_(True), y.requires_grad_(True), cond
def up_yy(n=N1):
# 边界 u_yy(x,1)=x^2/e
x = torch.rand(n, 1)
y = torch.ones_like(x)
cond = x ** 2 / torch.e
return x.requires_grad_(True), y.requires_grad_(True), cond
def down(n=N1):
# 边界 u(x,0)=x^2
x = torch.rand(n, 1)
y = torch.zeros_like(x)
cond = x ** 2
return x.requires_grad_(True), y.requires_grad_(True), cond
def up(n=N1):
# 边界 u(x,1)=x^2/e
x = torch.rand(n, 1)
y = torch.ones_like(x)
cond = x ** 2 / torch.e
return x.requires_grad_(True), y.requires_grad_(True), cond
def left(n=N1):
# 边界 u(0,y)=0
y = torch.rand(n, 1)
x = torch.zeros_like(y)
cond = torch.zeros_like(x)
return x.requires_grad_(True), y.requires_grad_(True), cond
def right(n=N1):
# 边界 u(1,y)=e^(-y)
y = torch.rand(n, 1)
x = torch.ones_like(y)
cond = torch.exp(-y)
return x.requires_grad_(True), y.requires_grad_(True), cond
def data_interior(n=N2):
# 内点
x = torch.rand(n, 1)
y = torch.rand(n, 1)
cond = (x ** 2) * torch.exp(-y)
return x.requires_grad_(True), y.requires_grad_(True), cond
# Neural Network
class MLP(torch.nn.Module):
def __init__(self):
super(MLP, self).__init__()
self.net = torch.nn.Sequential(
torch.nn.Linear(2, 32),
torch.nn.Tanh(),
torch.nn.Linear(32, 32),
torch.nn.Tanh(),
torch.nn.Linear(32, 32),
torch.nn.Tanh(),
torch.nn.Linear(32, 32),
torch.nn.Tanh(),
torch.nn.Linear(32, 1)
)
def forward(self, x):
return self.net(x)
# Loss
loss = torch.nn.MSELoss()
def gradients(u, x, order=1):
if order == 1:
return torch.autograd.grad(u, x, grad_outputs=torch.ones_like(u),
create_graph=True,
only_inputs=True, )[0]
else:
return gradients(gradients(u, x), x, order=order - 1)
# 以下7个损失是PDE损失
def l_interior(u):
# 损失函数L1
x, y, cond = interior()
uxy = u(torch.cat([x, y], dim=1))
return loss(gradients(uxy, x, 2) - gradients(uxy, y, 4), cond)
def l_down_yy(u):
# 损失函数L2
x, y, cond = down_yy()
uxy = u(torch.cat([x, y], dim=1))
return loss(gradients(uxy, y, 2), cond)
def l_up_yy(u):
# 损失函数L3
x, y, cond = up_yy()
uxy = u(torch.cat([x, y], dim=1))
return loss(gradients(uxy, y, 2), cond)
def l_down(u):
# 损失函数L4
x, y, cond = down()
uxy = u(torch.cat([x, y], dim=1))
return loss(uxy, cond)
def l_up(u):
# 损失函数L5
x, y, cond = up()
uxy = u(torch.cat([x, y], dim=1))
return loss(uxy, cond)
def l_left(u):
# 损失函数L6
x, y, cond = left()
uxy = u(torch.cat([x, y], dim=1))
return loss(uxy, cond)
def l_right(u):
# 损失函数L7
x, y, cond = right()
uxy = u(torch.cat([x, y], dim=1))
return loss(uxy, cond)
# 构造数据损失
def l_data(u):
# 损失函数L8
x, y, cond = data_interior()
uxy = u(torch.cat([x, y], dim=1))
return loss(uxy, cond)
# Training
u = MLP()
opt = torch.optim.Adam(params=u.parameters())
for i in range(epochs):
opt.zero_grad()
l = l_interior(u) \
+ l_up_yy(u) \
+ l_down_yy(u) \
+ l_up(u) \
+ l_down(u) \
+ l_left(u) \
+ l_right(u) \
+ l_data(u)
l.backward()
opt.step()
if i % 100 == 0:
print("Epoch: ", i, "Loss: ", l.item())
# Inference
xc = torch.linspace(0, 1, h)
xm, ym = torch.meshgrid(xc, xc)
xx = xm.reshape(-1, 1)
yy = ym.reshape(-1, 1)
xy = torch.cat([xx, yy], dim=1)
u_pred = u(xy)
u_real = xx * xx * torch.exp(-yy)
u_error = torch.abs(u_pred-u_real)
u_pred_fig = u_pred.reshape(h,h)
u_real_fig = u_real.reshape(h,h)
u_error_fig = u_error.reshape(h,h)
print("Max abs error is: ", float(torch.max(torch.abs(u_pred - xx * xx * torch.exp(-yy)))))
# 仅有PDE损失 Max abs error: 0.004852950572967529
# 带有数据点损失 Max abs error: 0.0018916130065917969
# 作PINN数值解图
fig = plt.figure()
ax = Axes3D(fig)
fig.add_axes(ax)
ax.plot_surface(xm.detach().numpy(), ym.detach().numpy(), u_pred_fig.detach().numpy())
ax.text2D(0.5, 0.9, "PINN", transform=ax.transAxes)
plt.show()
fig.savefig("PINN solve.png")
# 作真解图
fig = plt.figure()
ax = Axes3D(fig)
fig.add_axes(ax)
ax.plot_surface(xm.detach().numpy(), ym.detach().numpy(), u_real_fig.detach().numpy())
ax.text2D(0.5, 0.9, "real solve", transform=ax.transAxes)
plt.show()
fig.savefig("real solve.png")
# 误差图
fig = plt.figure()
ax = Axes3D(fig)
fig.add_axes(ax)
ax.plot_surface(xm.detach().numpy(), ym.detach().numpy(), u_error_fig.detach().numpy())
ax.text2D(0.5, 0.9, "abs error", transform=ax.transAxes)
plt.show()
fig.savefig("abs error.png")
torch.save(u.state_dict(),'model_uxt.pt')