STAR: Spatial-Temporal Augmentation with Text-to-Video Models for Real-World Video Super-Resolution
关键词:
text-to-video (T2V)
Local Information Enhancement Module (LIEM)
Dynamic Frequency (DF)
引言:
VSR: 传统VSR分两大类recurrent-based和sliding-window-based
T2V: U-Net based 和 Dit based ( CogVid)
PASD [61] and SeeSR [57] 在U-Net中嵌入语义信息引导diffusion
保真度可分为两种类型:1)低频保真度,包括大型结构和实例。2)高频保真度,包括边缘和纹理,符合去噪过程的特性。
sliding-window-based
创新:
- 引入Spatio-Temporal quality Augmentation framework, the first to integrate diverse, powerful
text-to-video diffusion priors into real-world VSR, 空间细节和时间一致性, 主要通过两个loss来实现的(LIEM loss 和 DF loss)
- 引入局部信息增强模块, 引入Dynamic Frequency loss学习diffusion steps中的特定信息, 解耦
fidelity 和提升最终fidelity.
实现:
框架(按照经验来说, 该框架起主要作用的是ControlNet)
Loss设计:
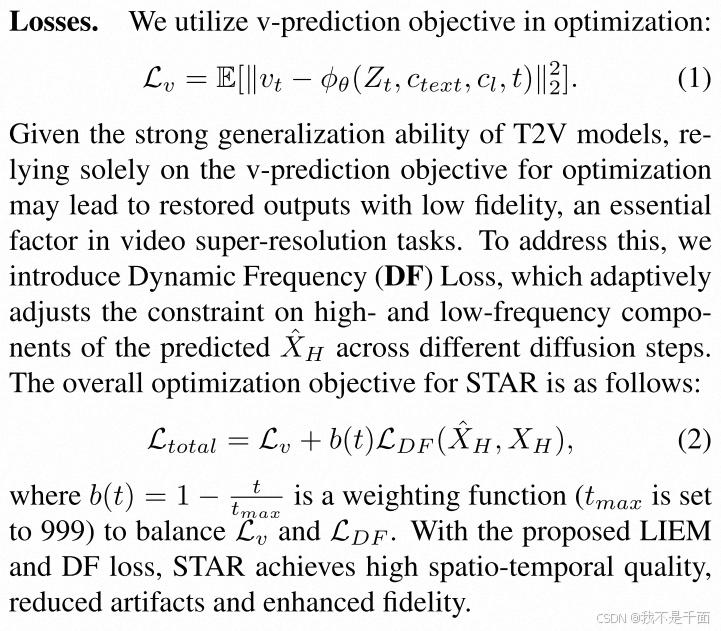
Local Information Enhancement Module (LIEM)的实现:

Dynamic Frequency (DF) Loss 的实现:
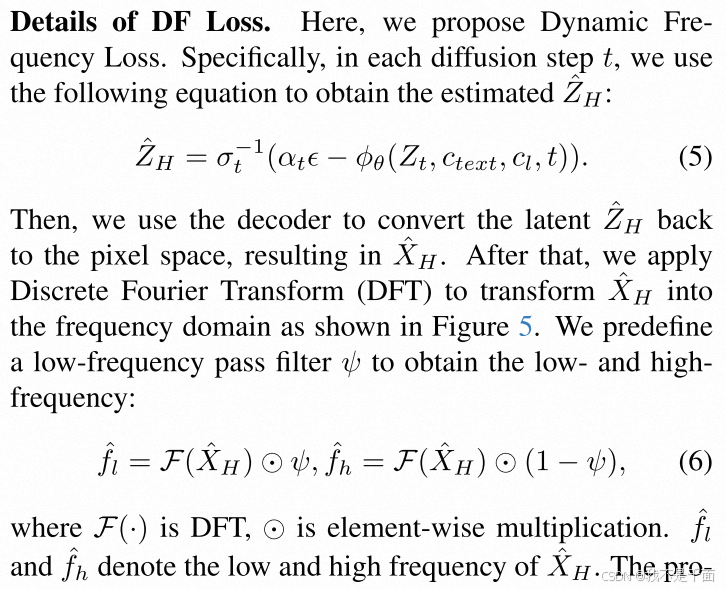
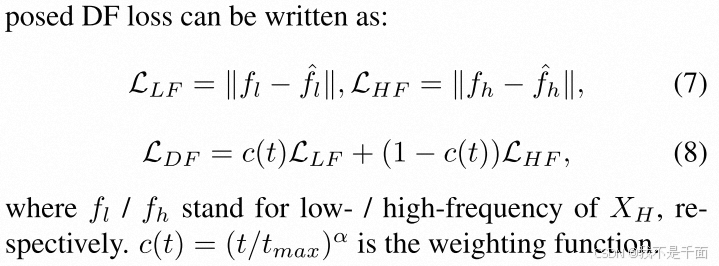