目录
- [1. 数据去重](#1. 数据去重)
-
- [1.1 原理](#1.1 原理)
- [1.2 pom.xml中依赖配置](#1.2 pom.xml中依赖配置)
- [1.3 工具类util](#1.3 工具类util)
- [1.4 去重代码](#1.4 去重代码)
- [1.5 结果](#1.5 结果)
- [2. 数据排序](#2. 数据排序)
-
- [2.1 原理](#2.1 原理)
- [2.2 排序代码](#2.2 排序代码)
- [2.3 结果](#2.3 结果)
- [3. 计算均值](#3. 计算均值)
-
- [3.1 原理](#3.1 原理)
- [3.2 自定义序列化数据类型DecimalWritable](#3.2 自定义序列化数据类型DecimalWritable)
- [3.3 计算平均值](#3.3 计算平均值)
- [3.4 结果](#3.4 结果)
- 参考
1. 数据去重
待去重的两个文本内容如下。
txt
2012-3-1 a
2012-3-2 b
2012-3-3 c
2012-3-4 d
2012-3-5 a
2012-3-6 b
2012-3-7 c
2012-3-3 c
txt
2012-3-1 b
2012-3-2 a
2012-3-3 b
2012-3-4 d
2012-3-5 a
2012-3-6 c
2012-3-7 d
2012-3-3 c
1.1 原理
利用MapReduce处理过程中键值唯一的特性,即可完成数据去重任务,只需把Map读入的<LongWritable, Text>键值对中的值作为Map输出键值对中的键,而输出键值对中的值设置为NullWritable类型,Reduce只需把Map输出的键值对直接原封不动输出即可。
1.2 pom.xml中依赖配置
xml
<dependencies>
<dependency>
<groupId>junit</groupId>
<artifactId>junit</artifactId>
<version>4.11</version>
<scope>test</scope>
</dependency>
<dependency>
<groupId>org.apache.hadoop</groupId>
<artifactId>hadoop-common</artifactId>
<version>3.3.6</version>
<exclusions>
<exclusion>
<groupId>org.slf4j</groupId>
<artifactId>slf4j-log4j12</artifactId>
</exclusion>
</exclusions>
</dependency>
<dependency>
<groupId>org.apache.hadoop</groupId>
<artifactId>hadoop-mapreduce-client-core</artifactId>
<version>3.3.6</version>
<type>pom</type>
</dependency>
<dependency>
<groupId>org.apache.hadoop</groupId>
<artifactId>hadoop-mapreduce-client-jobclient</artifactId>
<version>3.3.6</version>
</dependency>
</dependencies>
1.3 工具类util
java
import java.net.URI;
import java.util.regex.Matcher;
import java.util.regex.Pattern;
import org.apache.hadoop.conf.Configuration;
import org.apache.hadoop.fs.FSDataInputStream;
import org.apache.hadoop.fs.FileStatus;
import org.apache.hadoop.fs.FileSystem;
import org.apache.hadoop.fs.Path;
import org.apache.hadoop.io.IOUtils;
public class util {
public static FileSystem getFileSystem(String uri, Configuration conf) throws Exception {
URI add = new URI(uri);
return FileSystem.get(add, conf);
}
public static void removeALL(String uri, Configuration conf, String path) throws Exception {
FileSystem fs = getFileSystem(uri, conf);
if (fs.exists(new Path(path))) {
boolean isDeleted = fs.delete(new Path(path), true);
System.out.println("Delete Output Folder? " + isDeleted);
}
}
public static void showResult(String uri, Configuration conf, String path) throws Exception {
FileSystem fs = getFileSystem(uri, conf);
String regex = "part-r-";
Pattern pattern = Pattern.compile(regex);
if (fs.exists(new Path(path))) {
FileStatus[] files = fs.listStatus(new Path(path));
for (FileStatus file : files) {
Matcher matcher = pattern.matcher(file.getPath().toString());
if (matcher.find()) {
System.out.println(file.getPath() + ":");
FSDataInputStream openStream = fs.open(file.getPath());
IOUtils.copyBytes(openStream, System.out, 1024);
openStream.close();
}
}
}
}
}
1.4 去重代码
java
import java.io.IOException;
import org.apache.hadoop.conf.Configuration;
import org.apache.hadoop.fs.Path;
import org.apache.hadoop.io.LongWritable;
import org.apache.hadoop.io.NullWritable;
import org.apache.hadoop.io.Text;
import org.apache.hadoop.mapreduce.Job;
import org.apache.hadoop.mapreduce.Mapper;
import org.apache.hadoop.mapreduce.Reducer;
import org.apache.hadoop.mapreduce.lib.input.FileInputFormat;
import org.apache.hadoop.mapreduce.lib.output.FileOutputFormat;
public class App {
public static class MyMapper extends Mapper<LongWritable, Text, Text, NullWritable> {
@Override
protected void map(LongWritable key, Text value, Context context)
throws IOException, InterruptedException {
context.write(value, NullWritable.get());
}
}
public static class MyReducer extends Reducer<Text, NullWritable, Text, NullWritable> {
@Override
protected void reduce(Text key, Iterable<NullWritable> values, Context context)
throws IOException, InterruptedException {
context.write(key, NullWritable.get());
}
}
public static void main(String[] args) throws Exception {
Configuration conf = new Configuration();
String[] myArgs = {
"file:///home/developer/CodeArtsProjects/data-deduplication/dedup1.txt",
"file:///home/developer/CodeArtsProjects/data-deduplication/dedup2.txt",
"hdfs://localhost:9000/user/developer/data-deduplication/output"
};
util.removeALL("hdfs://localhost:9000", conf, myArgs[myArgs.length - 1]);
Job job = Job.getInstance(conf, "DataDeduplication");
job.setJarByClass(App.class);
job.setMapperClass(MyMapper.class);
job.setCombinerClass(MyReducer.class);
job.setReducerClass(MyReducer.class);
job.setOutputKeyClass(Text.class);
job.setOutputValueClass(NullWritable.class);
for (int i = 0; i < myArgs.length - 1; i++) {
FileInputFormat.addInputPath(job, new Path(myArgs[i]));
}
FileOutputFormat.setOutputPath(job, new Path(myArgs[myArgs.length - 1]));
int res = job.waitForCompletion(true) ? 0 : 1;
if (res == 0) {
System.out.println("数据去重的结果为:");
util.showResult("hdfs://localhost:9000", conf, myArgs[myArgs.length - 1]);
}
System.exit(res);
}
}
1.5 结果
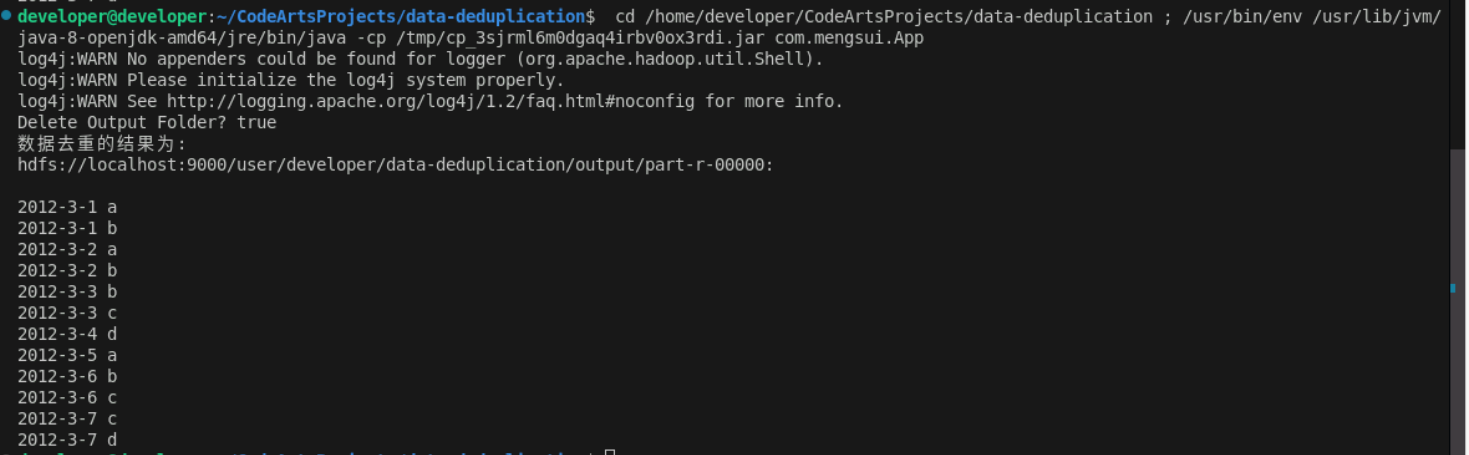
2. 数据排序
pom.xml中依赖配置、工具类util代码同1中。三个排序文本的内容如下。
txt
2
32
654
32
15
756
65223
6
txt
5956
22
650
92
54
txt
26
54
6
32
2.1 原理
利用MapReduce过程中Shuffle会对键值对排序的功能,只需要设置一个Reduce,把Map读入的<LongWritable, Text>键值对中的值处理后变为IntWritable类型,并作Map输出的键,同时Map输出的值设置为new IntWritable(1),这样便可以处理多个重复值的排序。然后设置一个静态计数器,作为Reduce输出的键,Reduce输出的值为Map输出的键。
2.2 排序代码
java
import java.io.IOException;
import org.apache.hadoop.conf.Configuration;
import org.apache.hadoop.fs.Path;
import org.apache.hadoop.io.IntWritable;
import org.apache.hadoop.io.LongWritable;
import org.apache.hadoop.io.Text;
import org.apache.hadoop.mapreduce.Job;
import org.apache.hadoop.mapreduce.Mapper;
import org.apache.hadoop.mapreduce.Reducer;
import org.apache.hadoop.mapreduce.lib.input.FileInputFormat;
import org.apache.hadoop.mapreduce.lib.output.FileOutputFormat;
public class App {
static int count = 0;
public static class MyMapper extends Mapper<LongWritable, Text, IntWritable, IntWritable> {
protected void map(LongWritable key, Text value, Context context)
throws IOException, InterruptedException {
int val = Integer.parseInt(value.toString());
context.write(new IntWritable(val), new IntWritable(1));
};
}
public static class MyReducer extends Reducer<IntWritable, IntWritable, IntWritable, IntWritable> {
protected void reduce(IntWritable key, Iterable<IntWritable> values, Context context)
throws IOException, InterruptedException {
for (IntWritable val : values) {
context.write(new IntWritable(++count) , key);
}
};
}
public static void main(String[] args) throws Exception {
Configuration conf = new Configuration();
String[] myArgs = {
"file:///home/developer/CodeArtsProjects/data-sort/sort1.txt",
"file:///home/developer/CodeArtsProjects/data-sort/sort2.txt",
"file:///home/developer/CodeArtsProjects/data-sort/sort3.txt",
"hdfs://localhost:9000/user/developer/DataSort/output"
};
util.removeALL("hdfs://localhost:9000", conf, myArgs[myArgs.length - 1]);
Job job = Job.getInstance(conf, "DataSort");
job.setJarByClass(App.class);
job.setMapperClass(MyMapper.class);
job.setReducerClass(MyReducer.class);
job.setOutputKeyClass(IntWritable.class);
job.setOutputValueClass(IntWritable.class);
for (int i = 0; i < myArgs.length - 1; i++) {
FileInputFormat.addInputPath(job, new Path(myArgs[i]));
}
FileOutputFormat.setOutputPath(job, new Path(myArgs[myArgs.length - 1]));
int res = job.waitForCompletion(true) ? 0 : 1;
if (res == 0) {
System.out.println("数据排序的结果为:");
util.showResult("hdfs://localhost:9000", conf, myArgs[myArgs.length - 1]);
}
System.exit(res);
}
}
2.3 结果
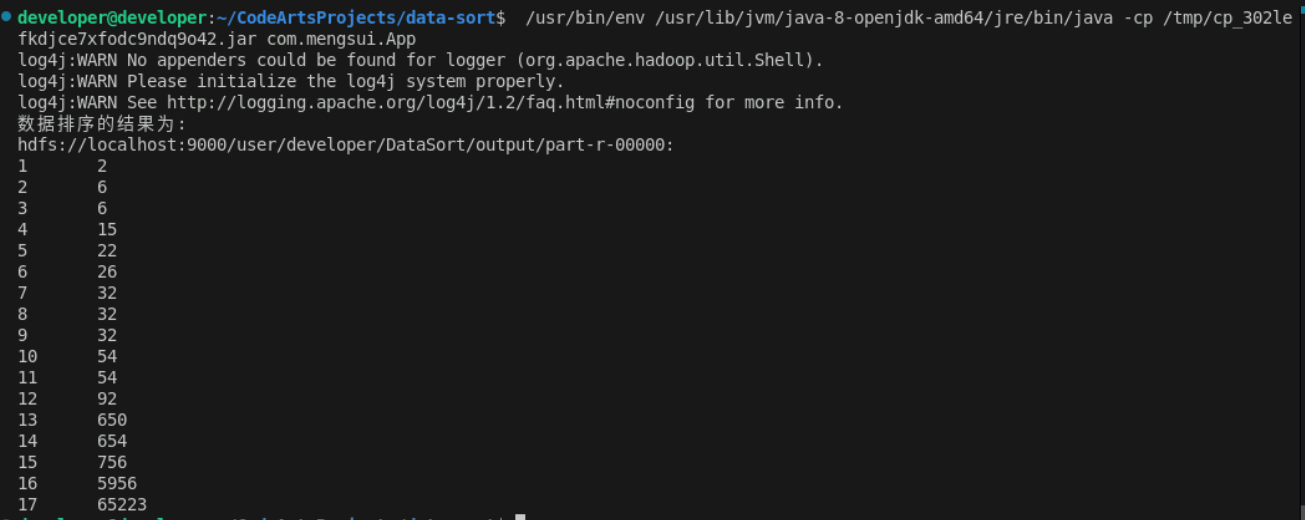
3. 计算均值
pom.xml中依赖配置、工具类util代码同1中。三门课的成绩文本如下。
txt
张三 78
李四 89
王五 96
赵六 67
txt
张三 88
李四 99
王五 66
赵六 77
txt
张三 80
李四 82
王五 84
赵六 86
3.1 原理
为了实现计算过程中的精度,利用java.math.BigDecimal
实现了一个自定义序列化数据类型DecimalWritable,方便在结果中保留指定小数位数。计算平均值首先把Map读入的<LongWritable, Text>键值对拆分成<Text, DecimalWritable>键值对,人名作为键,成绩作为值。Reduce将输入键值对中值列表进行累加再求平均,人名作为输出的键,平均值作为输出的值。
3.2 自定义序列化数据类型DecimalWritable
java
import java.io.DataInput;
import java.io.DataOutput;
import java.io.IOException;
import java.math.BigDecimal;
import java.math.RoundingMode;
import org.apache.hadoop.io.WritableComparable;
public class DecimalWritable implements WritableComparable<DecimalWritable> {
private BigDecimal value;
private int bit;
public void setValue(double value) {
this.value = new BigDecimal(value);
}
public BigDecimal getValue() {
return value;
}
public void setBit(int bit) {
this.bit = bit;
}
public int getBit() {
return bit;
}
DecimalWritable() {
super();
}
DecimalWritable(double value, int bit) {
super();
setValue(value);
setBit(bit);
}
DecimalWritable(BigDecimal value, int bit) {
super();
this.value = value;
setBit(bit);
}
@Override
public String toString() {
return value.setScale(bit, RoundingMode.HALF_UP).toString();
}
@Override
public void write(DataOutput out) throws IOException {
out.writeUTF(value.setScale(bit, RoundingMode.HALF_UP).toString());
out.writeInt(bit);
}
@Override
public int hashCode() {
return value.hashCode();
}
@Override
public boolean equals(Object obj) {
if (this == obj)
return true;
if (!(obj instanceof DecimalWritable))
return false;
DecimalWritable o = (DecimalWritable) obj;
return getValue().compareTo(o.getValue()) == 0;
}
@Override
public void readFields(DataInput in) throws IOException {
value = new BigDecimal(in.readUTF());
bit = in.readInt();
}
@Override
public int compareTo(DecimalWritable o) {
int res = getValue().compareTo(o.getValue());
if (res == 0)
return 0;
else if (res > 0)
return 1;
else
return -1;
}
}
3.3 计算平均值
java
import java.io.IOException;
import java.math.BigDecimal;
import java.math.RoundingMode;
import org.apache.hadoop.conf.Configuration;
import org.apache.hadoop.fs.Path;
import org.apache.hadoop.io.LongWritable;
import org.apache.hadoop.io.Text;
import org.apache.hadoop.mapreduce.Job;
import org.apache.hadoop.mapreduce.Mapper;
import org.apache.hadoop.mapreduce.Reducer;
import org.apache.hadoop.mapreduce.lib.input.FileInputFormat;
import org.apache.hadoop.mapreduce.lib.output.FileOutputFormat;
public class App {
public static class MyMapper extends Mapper<LongWritable, Text, Text, DecimalWritable> {
@Override
protected void map(LongWritable key, Text value, Context context)
throws IOException, InterruptedException {
String[] splitStr = value.toString().split(" ");
DecimalWritable grade = new DecimalWritable(Double.parseDouble(splitStr[1]), 2);
Text name = new Text(splitStr[0]);
context.write(name, grade);
}
}
public static class MyReducer extends Reducer<Text, DecimalWritable, Text, DecimalWritable> {
@Override
protected void reduce(Text key, Iterable<DecimalWritable> values, Context context)
throws IOException, InterruptedException {
BigDecimal sum = BigDecimal.ZERO;
for (DecimalWritable val : values) {
sum = sum.add(val.getValue());
}
sum = sum.divide(new BigDecimal(3), RoundingMode.HALF_UP);
context.write(key, new DecimalWritable(sum, 2));
}
}
public static void main(String[] args) throws Exception {
Configuration conf = new Configuration();
String[] myArgs = {
"file:///home/developer/CodeArtsProjects/cal-average-grade/chinese.txt",
"file:///home/developer/CodeArtsProjects/cal-average-grade/english.txt",
"file:///home/developer/CodeArtsProjects/cal-average-grade/math.txt",
"hdfs://localhost:9000/user/developer/CalAverageGrade/output"
};
util.removeALL("hdfs://localhost:9000", conf, myArgs[myArgs.length - 1]);
Job job = Job.getInstance(conf, "CalAverageGrade");
job.setJarByClass(App.class);
job.setMapperClass(MyMapper.class);
job.setReducerClass(MyReducer.class);
job.setOutputKeyClass(Text.class);
job.setOutputValueClass(DecimalWritable.class);
for (int i = 0; i < myArgs.length - 1; i++) {
FileInputFormat.addInputPath(job, new Path(myArgs[i]));
}
FileOutputFormat.setOutputPath(job, new Path(myArgs[myArgs.length - 1]));
int res = job.waitForCompletion(true) ? 0 : 1;
if (res == 0) {
System.out.println("平均成绩结果为:");
util.showResult("hdfs://localhost:9000", conf, myArgs[myArgs.length - 1]);
}
System.exit(res);
}
}
3.4 结果
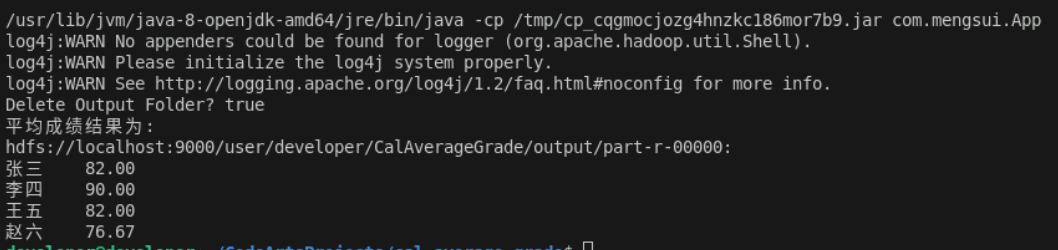
参考
吴章勇 杨强著 大数据Hadoop3.X分布式处理实战