一、ragflow相关信息
git地址:https://github.com/infiniflow/ragflow
文档地址:https://ragflow.io/docs/dev/
二、部署
git clone https://github.com/infiniflow/ragflow.gi
docker compose -f docker/docker-compose.yml up -d
在浏览器中对应的IP地址并登录RAGFlow 默认打开ragflow地址 http://localhost:80
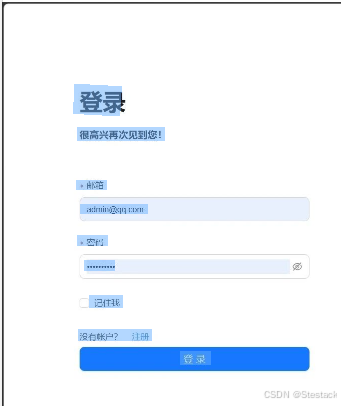
附件代码
import streamlit as st
from langchain_community.document_loaders import PDFPlumberLoader
from langchain_experimental.text_splitter import SemanticChunker
from langchain_community.embeddings import HuggingFaceEmbeddings
from langchain_community.vectorstores import FAISS
from langchain_community.llms import Ollama
from langchain.prompts import PromptTemplate
from langchain.chains.llm import LLMChain
from langchain.chains.combine_documents.stuff import StuffDocumentsChain
from langchain.chains import RetrievalQA
# color palette
primary_color = "#1E90FF"
secondary_color = "#FF6347"
background_color = "#F5F5F5"
text_color = "#4561e9"
# Custom CSS
st.markdown(f"""
<style>
.stApp {{
background-color: {background_color};
color: {text_color};
}}
.stButton>button {{
background-color: {primary_color};
color: white;
border-radius: 5px;
border: none;
padding: 10px 20px;
font-size: 16px;
}}
.stTextInput>div>div>input {{
border: 2px solid {primary_color};
border-radius: 5px;
padding: 10px;
font-size: 16px;
}}
.stFileUploader>div>div>div>button {{
background-color: {secondary_color};
color: white;
border-radius: 5px;
border: none;
padding: 10px 20px;
font-size: 16px;
}}
</style>
""", unsafe_allow_html=True)
# Streamlit app title
st.title("Build a RAG System with DeepSeek R1 & Ollama")
# Load the PDF
uploaded_file = st.file_uploader("Upload a PDF file", type="pdf")
if uploaded_file is not None:
# Save the uploaded file to a temporary location
with open("temp.pdf", "wb") as f:
f.write(uploaded_file.getvalue())
# Load the PDF
loader = PDFPlumberLoader("temp.pdf")
docs = loader.load()
# Split into chunks
text_splitter = SemanticChunker(HuggingFaceEmbeddings())
documents = text_splitter.split_documents(docs)
# Instantiate the embedding model
embedder = HuggingFaceEmbeddings()
# Create the vector store and fill it with embeddings
vector = FAISS.from_documents(documents, embedder)
retriever = vector.as_retriever(search_type="similarity", search_kwargs={"k": 3})
# Define llm
llm = Ollama(model="deepseek-r1")
# Define the prompt
prompt = """
1. Use the following pieces of context to answer the question at the end.
2. If you don't know the answer, just say that "I don't know" but don't make up an answer on your own.\n
3. Keep the answer crisp and limited to 3,4 sentences.
Context: {context}
Question: {question}
Helpful Answer:"""
QA_CHAIN_PROMPT = PromptTemplate.from_template(prompt)
llm_chain = LLMChain(
llm=llm,
prompt=QA_CHAIN_PROMPT,
callbacks=None,
verbose=True)
document_prompt = PromptTemplate(
input_variables=["page_content", "source"],
template="Context:\ncontent:{page_content}\nsource:{source}",
)
combine_documents_chain = StuffDocumentsChain(
llm_chain=llm_chain,
document_variable_name="context",
document_prompt=document_prompt,
callbacks=None)
qa = RetrievalQA(
combine_documents_chain=combine_documents_chain,
verbose=True,
retriever=retriever,
return_source_documents=True)
# User input
user_input = st.text_input("Ask a question related to the PDF :")
# Process user input
if user_input:
with st.spinner("Processing..."):
response = qa(user_input)["result"]
st.write("Response:")
st.write(response)
else:
st.write("Please upload a PDF file to proceed.")