Title
题目
Bridging multi-level gaps: Bidirectional reciprocal cycle framework fortext-guided label-efficient segmentation in echocardiography
弥合多层次差距:用于超声心动图中基于文本引导的标签高效分割的双向循环框架
01
文献速递介绍
文本引导方法是超声心动图中实现标签高效分割的一种潜在途径。标签高效分割对于超声心动图分析至关重要,尤其是在标签数量有限或稀缺的情况下(勒克莱尔等人,2019;李等人,2020)。在超声心动图分析中,缺乏大规模高质量的标签仍然是一个长期存在的问题(于等人,2023)。这是因为手动数据标注仍然既耗时又耗力。它需要长期的临床观察经验(李等人,2022;托马尔等人,2022)。与从有限数量的病例中学习相比,对复杂临床病例进行文本引导学习是一种更有效的方式。它可以通过文本中的明确知识获取临床专业知识,而无需从大量数据中总结隐含规律(例如,通过文本提示快速了解一个序列是单周期还是多周期)。这是因为临床文本包含了高度概括的临床经验。在这些情况下,文本添加了额外的先验信息(例如,高度概括的临床记录和经验),包括视图的变化、心脏结构以及超声心动图的动态变化等等(道格拉斯等人,2019)。此外,医疗记录中的配对文本和图像可以相互验证,以推动下游任务。文本中的信息可以与超声心动图的视觉线索相匹配(例如,心脏收缩功能的描述可以与左心室大小的视觉变化相匹配)。它们为特殊病例(例如,罕见的疾病病例和变异结构)提供了隐含的提示,并有助于临床指标计算的评估。因此,在超声心动图的标签高效分割中加入临床文本引导是很有必要的(见图1)。 然而,超声心动图中的文本引导分割仍然面临着多层次差距的挑战(李等人,2022;吕德克和埃克,2022;杨等人,2022;托马尔等人,2022;单等人,2023)。这导致了文本描述中的描述信息与超声心动图中的视觉线索之间存在多种不匹配关系(李等人,2022;吕德克和埃克,2022)。这种差距主要源于三个层面:空间层面、上下文层面和领域层面。首先,空间层面的差距意味着文本描述可能无法明确对应于空间细节信息(例如,图像中物体的形状和纹理)。这种信息因病例和视图而异。需要从大规模数据中进行观察。与其他模态相比,超声心动图通常分辨率较低。复杂的心脏结构和详细的解剖特征往往需要较高的空间分辨率。文本只能对位置或形状给出模糊的描述(例如,用文本信息重建超声心动图中一些不清楚的区域细节)(单等人,2023;托马尔等人,2022;李等人,2022)。很难将一般性的文本指令与细粒度的空间细节相匹配。其次,上下文层面的差距是指医学文本-图像对中观察结果与诊断之间的复杂关系。具体来说,超声心动图成像的动态特性捕捉了心脏的实时运动和功能。这引入了时间变异性和上下文依赖关系。心动周期内的这种上下文信息在引导超声心动图的分割和诊断中起着至关重要的作用。例如,心脏收缩功能可以通过观察左心室的舒张末期(ED)和收缩末期(ES)大小来诊断(道格拉斯等人,2019)。然而,这种关系依赖于复杂的临床专业知识。这通常需要从大量样本中进行总结。在缺乏大规模配对数据的情况下,很难将诊断假设与观察结果直接匹配。第三,领域层面的差距是指文本中的临床特定术语与图像中的视觉线索之间的间接关系。在超声心动图中,领域知识包括对超声成像中心脏解剖结构的深入理解。具体来说,这包括图像中心腔、瓣膜和心肌的独特外观等特定领域特征。例如,视觉结构(例如,心房和心室)与特定临床文本描述(例如,ED和ES)之间的差距(托马尔等人,2022;李等人,2022)。这通常在于通过大量的文本-图像对学习一种间接转换。然后,文本可以利用在转换中学习到的这些提取特征直接指导视觉任务。这些多层次的差距使得很难从临床文本中学习到有用的特征,并进一步指导超声心动图中的下游任务。 现有的文本引导方法仍然难以应对这一挑战。早期的方法将文本信息总结为视觉学习的目标。与其他自适应方法相比,它需要人工操作来弥合图像-文本的差距(例如,手动过滤文本中的冗余信息以设置明确的文本答案目标)(王等人,2022b)。最近的方法在文本-图像对中构建对比学习(拉德福德等人,2021;吕德克和埃克,2022;李等人,2022)。一种具有代表性的方法是对比语言-图像预训练CLIP(图2(a))(拉德福德等人,2021)。基于CLIP的方法可以匹配配对图像和文本的共同特征。这些方法可以通过区分配对和未配对的图像-文本进一步提取图像-文本特定特征(单等人,2023)。一些基于CLIP的方法设计了层次结构(图2(b))。这些方法旨在挖掘和对应图像-文本对中的全局和局部特征。此外,一些文本引导方法将文本嵌入到视觉任务解码器中以直接指导任务(图2(c))(王等人,2022a;黄等人,2021)。然而,这些方法仍然远未能弥合文本和图像之间的多层次差距,尤其是对于医学图像-文本对。这主要源于三个方面。首先,由于缺乏对空间关系的相应约束,它只能隐含地学习心脏的全局结构和区域病变(克里斯滕森等人,2024;吕德克和埃克,2022)。这可能无法确保在提取的特征表示中保留特定的空间信息。其次,现有方法专注于对齐跨模态特征,但缺乏对模态内上下文关系的建模(王等人,2022a)。这导致在特征提取中缺乏关键的上下文关系,尤其是从输入数据到表示的过程中。第三,这些方法缺乏对领域知识关系的特定建模(单等人,2023)。这阻碍了对这种医学专业知识的提取。因此,这需要在模态特征、模态内上下文和跨模态之间建立多层次的约束(见图3)。 我们提出了一种双向循环(BRC)框架,用于超声心动图中的标签高效分割。BRC通过循环映射实现多层次对齐,以弥合图像-文本对中的多层次差距。与现有的基于CLIP的方法侧重于单层次前向过程对齐不同,我们的BRC在于多层次反向过程对齐。具体来说,基于CLIP范式,我们添加了局部-全局金字塔式对比学习(图4(a)),并进一步分别为上下文特征和跨模态领域特征构建了回顾性映射(图4(b))和双向映射(图4(c))。其优点是利用图像丰富的局部-全局特征,并在特征提取过程中保持上下文一致性。此外,其有效性体现在三个过程中,包括金字塔双向对齐、模态内反向映射和跨模态传播。首先,BRC构建嵌入表示的金字塔双向对齐。这包括对全局-局部图像-文本对之间的关系进行建模。这有助于将复杂的医学专业知识与相应的视觉线索相匹配。其次,BRC强制从文本或图像到特征的前向推理与反向映射一致(例如,数据→特征与特征→数据一致)。这约束了前向特征提取与输入数据和特征表示之间的上下文关系紧密相连。第三,BRC构建了模态特征之间的映射关系。这促使对不同模态特征进行重建,以预测内在缺失的特征。这重建了图像和文本之间缺失的空间特征。在网络实现中,专门设计的文本引导注意力可以聚合跨模态多层次特征并保留细粒度信息。这包括模态内自注意力模块和跨模态自注意力模块。我们在每个尺度上构建这种文本引导注意力。文本信息在多个尺度上对视觉信息进行深度监督。这使得文本能够充分参与多个尺度上的密集预测任务。下游任务解码器独立于框架中的其他模块。它可以适应不同的下游任务。就实验结果而言,与最先进的方法相比,我们的BRC框架在标注数据为10%和100%时,分割精度在迪赛相似系数(DSC)上分别提高了3.4%和0.8%。 本文的贡献如下: - 我们的BRC是首个在超声心动图中进行文本引导分割的方法。它可以减少对大量人工数据标注的依赖。 - 我们的BRC基于从特征域到图像和文本域的循环映射构建了约束。它弥合了医学图像-文本对的多层次差距。此外,我们提出了一种文本引导网络以适应下游分割任务。 - 在六个数据集(11048名患者)上进行的大量实验表明,我们的BRC相较于22种最先进的方法取得了更优异的性能。
Aastract
摘要
Text-guided visual understanding is a potential solution for downstream task learning in echocardiography. Itcan reduce reliance on labeled large datasets and facilitate learning clinical tasks. This is because the text canembed highly condensed clinical information into predictions for visual tasks. The contrastive language-imagepretraining (CLIP) based methods extract image-text features by constructing a contrastive learning pre-trainprocess in a sequence of matched text and images. These methods adapt the pre-trained network parameters toimprove downstream task performance with text guidance. However, these methods still have the challenge ofthe multi-level gap between image and text. It mainly stems from spatial-level, contextual-level, and domainlevel gaps. It is difficult to deal with medical image--text pairs and dense prediction tasks. Therefore, we proposea bidirectional reciprocal cycle (BRC) framework to bridge the multi-level gaps. First, the BRC constructspyramid reciprocal alignments of embedded global and local image--text feature representations. This matchescomplex medical expertise with corresponding phenomena. Second, BRC enforces the forward inference to beconsistent with the reverse mapping (i.e., the text → feature is consistent with the feature → text or feature →image). This enforces the perception of the contextual relationship between input data and feature. Third, theBRC can adapt to the specific downstream segmentation task. This embeds complex text information to directlyguide downstream tasks with a cross-modal attention mechanism. Compared with 22 existing methods, ourBRC can achieve state-of-the-art performance on segmentation tasks (DSC = 95.2%). Extensive experimentson 11048 patients show that our method can significantly improve the accuracy and reduce the reliance onlabeled data (DSC increased from 81.5% to 86.6% with text assistance in 1% labeled proportion data)
基于文本引导的视觉理解是超声心动图下游任务学习的一种潜在解决方案。它可以减少对带有标注的大型数据集的依赖,并有助于学习临床任务。这是因为文本能够将高度浓缩的临床信息嵌入到视觉任务的预测当中。基于对比语言-图像预训练(CLIP)的方法通过在匹配的文本和图像序列中构建对比学习预训练过程来提取图像-文本特征。这些方法调整预训练的网络参数,以便在文本引导下提升下游任务的性能。然而,这些方法仍然面临着图像与文本之间多层次差距的挑战。这种差距主要源于空间层面、上下文层面以及领域层面的差异。处理医学图像-文本对以及密集预测任务存在一定难度。 因此,我们提出了一种双向循环(BRC)框架来弥合这些多层次的差距。首先,BRC构建了嵌入的全局和局部图像-文本特征表示的金字塔式双向对齐关系。这使得复杂的医学专业知识与相应的现象相匹配。其次,BRC强制前向推理与反向映射保持一致(即文本→特征与特征→文本或特征→图像的过程一致)。这加强了对输入数据和特征之间上下文关系的感知。第三,BRC能够适应特定的下游分割任务。它通过跨模态注意力机制嵌入复杂的文本信息,从而直接指导下游任务。 与22种现有方法相比,我们的BRC在分割任务上能够达到最先进的性能水平(迪赛相似系数DSC = 95.2%)。在11048名患者数据上进行的大量实验表明,我们的方法能够显著提高准确性,并减少对标注数据的依赖(在标注比例为1%的数据中,借助文本辅助,DSC从81.5%提高到了86.6%)。
Method
方法
3.1. Problem formulationWe formulate two key problems in visual--text alignment and textguided segmentation tasks. Let a labeled visual space {𝑥 ( 𝑣 𝑖) ∈ 𝑋𝑉 , 𝑖 ∈ 𝑁}and a corresponding labeled text space {𝑥 ( 𝑡 𝑖) ∈ 𝑋𝑇 , 𝑖 ∈ 𝑁}, where𝑁 is the size of the labeled dataset. Let an unlabeled visual space{𝑥 ∗( 𝑣 𝑗) ∈ 𝑋**𝑉 ∗ , 𝑗 ∈ 𝑀}, where 𝑀 is the size of the unlabeled dataset. Letthe visual encoder function is 𝐸𝑣 (⋅; 𝜀𝑣 ) and the task decoder function is𝐷. The overall learning problem can be formulated as:⎧⎪⎨⎪⎩ = arg min𝜀𝑣∑𝑁𝑖=0 𝑡 (𝐷[*𝐸𝑣* (𝑥**𝑣 (𝑖) ; 𝜀**𝑣 )], 𝑦)𝑦* ∗(𝑗) = 𝐷𝐸**𝑣 (𝑥 ∗ 𝑣 (𝑗) ; 𝜀𝑣 )where denotes the collection of encoder parameters, 𝑦 denotes thetask label. It designs the objective function 𝑡 to minimize the distanceof prediction 𝐷[𝐸𝑣 (𝑥 ( 𝑣 𝑖) , 𝜀𝑣 )] and label 𝑦 for the training stage withless labeled data. Furthermore, this uses trained model parameters topredict large-scale unlabeled data.
3.1 问题表述 我们阐述了视觉-文本对齐以及文本引导分割任务中的两个关键问题。假设有一个带标注的视觉空间({x(v_i) \in X^V, i \in N})以及一个相应的带标注的文本空间({x(t_i) \in X^T, i \in N}),其中(N)是带标注数据集的规模大小。假设有一个无标注的视觉空间({x^(v_j) \in X^{V}, j \in M}),其中(M)是无标注数据集的规模大小。设视觉编码器函数为(E^v(\cdot; \epsilon^v)),任务解码器函数为(D)。整体的学习问题可以表述为: (\begin{cases} \mathcal{E} = \underset{\epsilon^v}{\arg\min} \sum_{i = 0}^{N} \mathcal{L}^t(D[E^v(x^{v}(i); \epsilon^v)], y) \ y^(j) = D[E^v(x^v(j); \epsilon^v)] \end{cases}) (1) 其中,(\mathcal{E})表示编码器参数的集合,(y)表示任务标签。在标注数据较少的训练阶段,设计目标函数(\mathcal{L}^t)是为了最小化预测值(D[E^v(x(v_i), \epsilon^v)])与标签(y)之间的距离。此外,利用训练好的模型参数对大规模的无标注数据进行预测。
Conclusion
结论
In this paper, we propose a bidirectional reciprocal cycle (BRC)framework to address the multi-level gap for image--text understandingin echocardiography. Our BRC jointly performs multiple alignmentand reverse mapping approaches to bridge spatial-level, contextuallevel, and domain-level gaps in image--text pairs. Besides, we proposea text-guided network to adapt to downstream segmentation tasks.Extensive experiments on a large number of datasets demonstrate theeffectiveness of our BRC. Experiments on datasets with different labelratios demonstrate that our BRC can efficiently utilize labels.
在本文中,我们提出了一种双向循环(BRC)框架,以解决超声心动图中图像-文本理解方面的多层次差距问题。我们的BRC框架联合采用了多种对齐和反向映射方法,来弥合图像-文本对在空间层面、上下文层面以及领域层面的差距。此外,我们还提出了一种文本引导网络,以适配下游的分割任务。 在大量数据集上进行的广泛实验证明了我们的BRC框架的有效性。在具有不同标注比例的数据集上开展的实验表明,我们的BRC框架能够有效地利用标注数据。
Figure
图
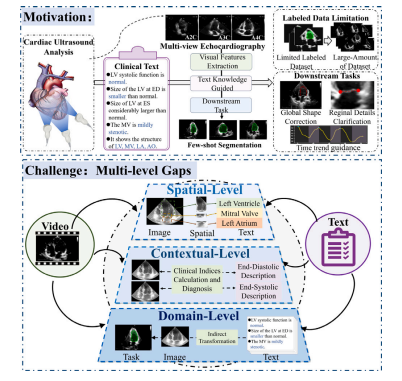
Fig. 1. The contribution of our BRC. (a) The BRC framework aims to extract multilevel features from the video and text, and further propagate these features to thedownstream tasks. It can reduce the reliance on labeled data and facilitate thedownstream task. (b) The challenge is the multi-level gaps in video--text pairs, includingspatial-level gaps, contextual-level gaps, and domain-level gaps
图1:我们的双向循环(BRC)框架的贡献。(a) BRC框架旨在从视频和文本中提取多层次特征,然后进一步将这些特征传播应用到下游任务中。它能够减少对标注数据的依赖,并推动下游任务的进展。(b) 目前面临的挑战是视频-文本对中存在多层次的差距,其中包括空间层面的差距、上下文层面的差距以及领域层面的差距。
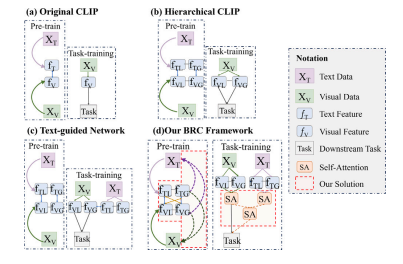
Fig. 2. Comparison with existing visual--text learning methods. (a) Original CLIParchitecture refers to constructing a contrastive process to align the correspondingimage feature 𝑓𝑉 and text feature 𝑓𝑇 in image--text pairs 𝑥𝑉 and 𝑥𝑇 . (b) Thehierarchical CLIP-based method refers to the alignment of local feature 𝑓𝑉 𝐿 and 𝑓𝑇 𝐿as well as global features 𝑓𝑉 𝐺 and 𝑓𝑇 𝐺. (c) The text-guided network refers to addingadditional text supervision (i.e., adding 𝑓𝑇 𝐿 and 𝑓𝑇 𝐺 to guided 𝑓𝑉 𝐿 and 𝑓𝑉 𝐺 in thedownstream task) information to the task. (d) Our BRC framework adds the multi-levelmatching mechanism in text--visual pre-training and the cross-modal feature attentionin downstream task training
图2. 与现有视觉-文本学习方法的对比。(a) 原始的对比语言-图像预训练(CLIP)架构是指构建一个对比过程,以使图像-文本对(x_V)和(x_T)中对应的图像特征(f_V)和文本特征(f_T)实现对齐。(b) 基于层次结构的CLIP方法是指对齐局部特征(f{V}^{L})和(f{T}^{L}),以及全局特征(f{V}^{G})和(f{T}^{G}) 。(c) 文本引导网络是指为任务添加额外的文本监督信息(即在下游任务中添加(f{T}^{L})和(f{T}^{G})来引导(f{V}^{L})和(f{V}^{G}) )。(d) 我们的双向循环(BRC)框架在文本-视觉预训练中添加了多层次匹配机制,并在下游任务训练中引入了跨模态特征注意力机制。
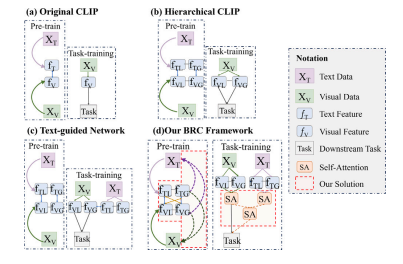
Fig. 3. The differences between our BRC and previous text-guided methods. (a)This shows the implementation of our BRC in text-vision pre-training. Our BRCframework constructs multi-level contrastive learning and intra-modal and inter-modalbacktracking mapping mechanisms. (b) This shows the implementation of our BRCframework on downstream segmentation tasks. Our BRC framework builds intra-modalattention mechanisms and inter-modal attention mechanisms. This propagates usefulinformation from text to dense prediction visual tasks
图3:我们的双向循环(BRC)框架与先前文本引导方法的差异。(a) 此图展示了我们的BRC在文本-视觉预训练中的实现方式。我们的BRC框架构建了多层次对比学习以及模态内和跨模态回溯映射机制。(b) 此图展示了我们的BRC框架在下游分割任务中的实现。我们的BRC框架建立了模态内注意力机制和跨模态注意力机制。这将文本中的有用信息传播到密集预测视觉任务中。
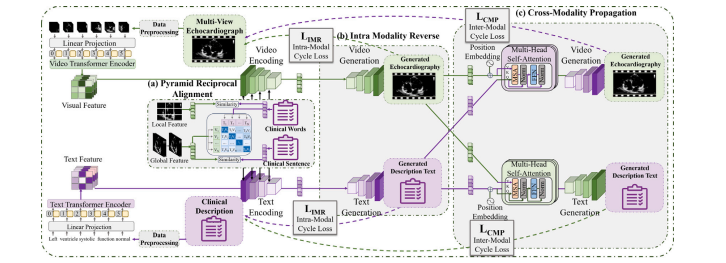
Fig. 4. The implement details of our BRC framework. (a) The pyramid reciprocal alignment process aligns relevant local and global features of the image. (b) Intra-modalityreverse mapping can map the features to the input text and image. It constrains the features consistent with the input. (c) Cross-modality propagation mapping can map thefeatures to the cross-modality input.
图4:我们的双向循环(BRC)框架的实现细节。(a) 金字塔双向对齐过程将图像的相关局部和全局特征进行对齐。(b) 模态内反向映射可以将特征映射回输入的文本和图像。它约束特征与输入保持一致。(c) 跨模态传播映射可以将特征映射到跨模态的输入中。
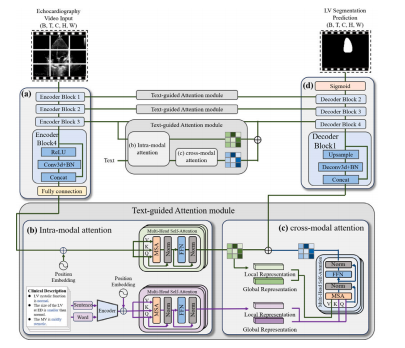
Fig. 5. The text-guided segmentation network implementation. The text featuresextracted by the BRC framework can facilitate downstream visual segmentationtasks through the text-guided segmentation network. It uses the U-net-like pyramidarchitecture with multiple attention mechanisms to adapt to the segmentation task.(a) The visual encoder extracts global and local visual features. (b) The intra-modalattention mechanism extracts global-local text and visual dependencies. (c) The crossmodal attention mechanism embeds global-local text features into the visual inferenceprocess. (d) The task decoder outputs the corresponding segmentation mask
图5:文本引导分割网络的实现。由双向循环(BRC)框架提取的文本特征能够通过文本引导分割网络助力下游的视觉分割任务。该网络采用类似U型网络(U-net)的金字塔架构,并配备多种注意力机制,以适配分割任务。 (a) 视觉编码器提取全局和局部的视觉特征。 (b) 模态内注意力机制提取全局与局部的文本和视觉依赖关系。 (c) 跨模态注意力机制将全局与局部的文本特征嵌入到视觉推理过程中。 (d) 任务解码器输出相应的分割掩码。
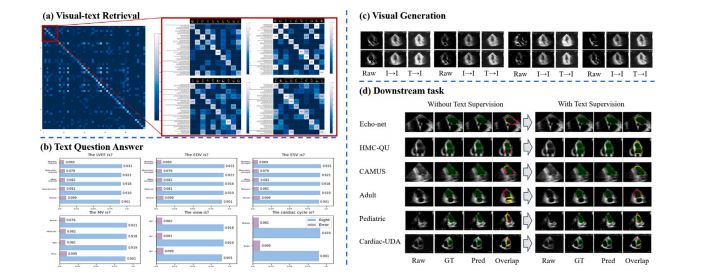
Fig. 6. Visualizations of outputs in different stages, including the results of visual--text retrieval, generated image-texts, and task labels versus predictions on all datasets. (a)Text and images are retrieved one by one based on contrastive learning. The value on the upper left to the lower right oblique indicates the accuracy of image--text pairing. (b)Text generated from the text feature. The text question consists of six aspect text descriptions. The blue bars indicate the number of correct predictions in the sample. (c) Imagegenerated from visual--text features (𝐼 represents image and 𝑇 represents text). (d) comparison of the predicted mask with the label mask and the overlay with the original image.The results are shown in green (true positive), yellow (true negative), and pink (false positive)
图6:不同阶段输出的可视化结果,包括视觉-文本检索的结果、生成的图像-文本以及所有数据集上的任务标签与预测结果的对比。(a) 基于对比学习,文本和图像逐一进行检索。从左上角到右下角的对角线上的值表示图像-文本配对的准确率。(b) 由文本特征生成的文本。文本问题由六个方面的文本描述组成。蓝色条形图表示样本中正确预测的数量。(c) 由视觉-文本特征生成的图像(𝐼 表示图像,𝑇 表示文本)。(d) 预测掩码与标签掩码的对比,以及与原始图像的叠加情况。结果以绿色(真阳性)、黄色(真阴性)和粉色(假阳性)显示。
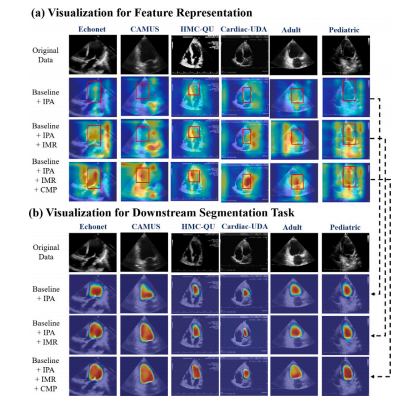
Fig. 7. Visualizations of the activation areas for each module in our BRC on differentdatasets through grad-cam. Figure (a) shows the high activation area of our BRC whenextracting feature representation. Figure (b) shows the highly activated regions of ourBRC when fine-tuned to downstream segmentation tasks
图7:通过梯度加权类激活映射(grad-cam)展示我们的双向循环(BRC)框架在不同数据集上各模块的激活区域可视化结果。图(a)展示了我们的BRC在提取特征表示时的高激活区域。图(b)展示了我们的BRC在微调以适配下游分割任务时的高激活区域。
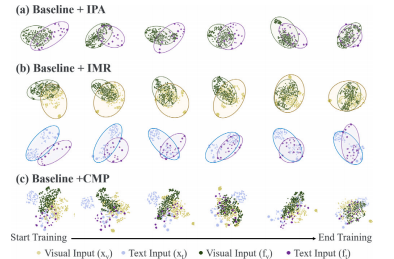
Fig. 8. The T-SNE visualization of the original data and feature extraction. Thisvisualizes the distance between the cross-modality features of each module of our BRC.(a) IPA module can aggregate the image and text features. (b) The 𝐼𝑀𝑅 module canfurther bridge feature representation and raw data. (c) The 𝐶𝑀𝑃 module can bridgeimage and text feature representations with the cross-modality data input.
图8:原始数据和特征提取的T分布随机邻域嵌入(T-SNE)可视化。这可视化展示了我们的双向循环(BRC)框架各模块的跨模态特征之间的距离。(a) 金字塔双向对齐(IPA)模块可以聚合图像和文本特征。(b) 模态内反向映射(𝐼𝑀𝑅)模块可以进一步弥合特征表示与原始数据之间的差距。(c) 跨模态传播(𝐶𝑀𝑃)模块可以通过跨模态数据输入弥合图像和文本特征表示之间的差距。
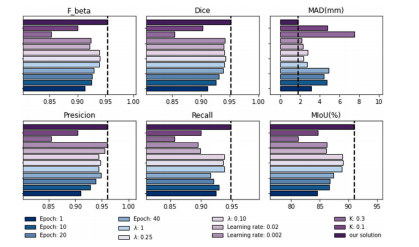
Fig. 9. Effectiveness evaluation for our network configurations. It includes commonlyused image--text baseline networks with different training epochs, learning rates, and𝜆 and 𝐾. The best results show the validity of the current configuration of the BRC.
图9:对我们网络配置的有效性评估。评估内容包括具有不同训练轮次、学习率以及参数λ和K的常用图像-文本基线网络。最佳结果表明了双向循环(BRC)当前配置的有效性。
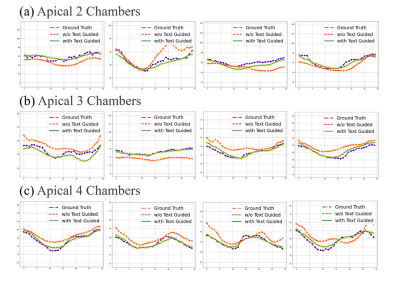
Fig. 10. The temporal trends and error of our BRC framework with or without textguided in three views, including (a) A2C, (b) A3C, and (c) A4C. The purple, red, andgreen lines indicate the ground truth, without text guidance, and with text guidance,respectively. The descriptions in the text, such as the periodicity and cardiac size in theED and ES frames, can constrain the temporal consistency of the segmentation process
图10:我们的双向循环(BRC)框架在有或无文本引导情况下,于三个视图中的时间趋势和误差情况,这三个视图包括:(a) 心尖两腔心切面(A2C),(b) 心尖三腔心切面(A3C),以及 (c) 心尖四腔心切面(A4C)。紫色、红色和绿色线条分别表示真实值、无文本引导情况和有文本引导情况。文本中的描述信息,例如舒张末期(ED)和收缩末期(ES)帧中的周期性和心脏大小等内容,可以约束分割过程中的时间一致性。
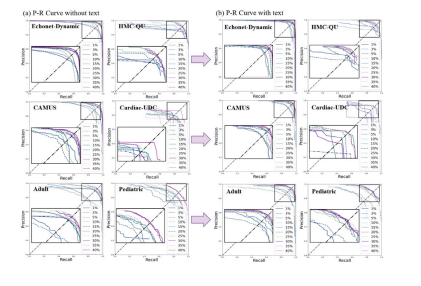
Fig. 11. Comparison of Precision--Recall (P--R) curves of different labeled proportionswith or without text in six datasets. Among them, the closer the intersection pointof the curve and the diagonal line to the upper right correspond to the better theperformance of the method.
图11:六个数据集中在有文本引导和无文本引导情况下,不同标注比例的精确率-召回率(P-R)曲线对比。其中,曲线与右上角对角线的交点越接近,对应方法的性能越好。
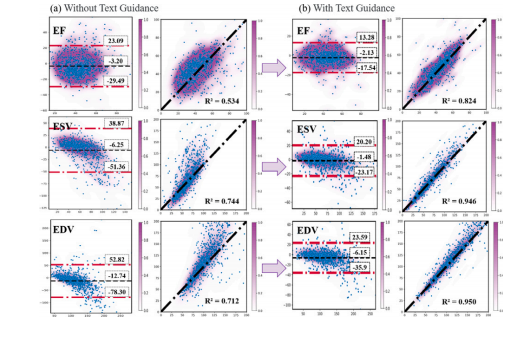
Fig. 12. Bland--Altman analysis and the linear regression analysis between our BRCpredictions and GT. The color of the colorbar indicates the density of points. TheBland--Altman analysis demonstrates the high agreement of our BRC with the GT. The𝑦*-axis represents the deviation between the value of clinical indices measured withBRC and the manual result. The black dashed line represents the mean deviation. Theblue dashed line indicates the 95% confidence interval for the deviation. The linearregression analysis demonstrates the high correlation of BRC.
图12:我们的双向循环(BRC)框架的预测结果与真实值(GT)之间的布兰德-奥特曼(Bland--Altman)分析以及线性回归分析。色条的颜色表示点的密度。布兰德-奥特曼分析表明我们的BRC框架与真实值之间具有高度的一致性。纵轴(y轴)表示使用BRC测量的临床指标值与手动测量结果之间的偏差。黑色虚线表示平均偏差。蓝色虚线表示偏差的95%置信区间。线性回归分析表明BRC框架具有高度的相关性。
Table
表
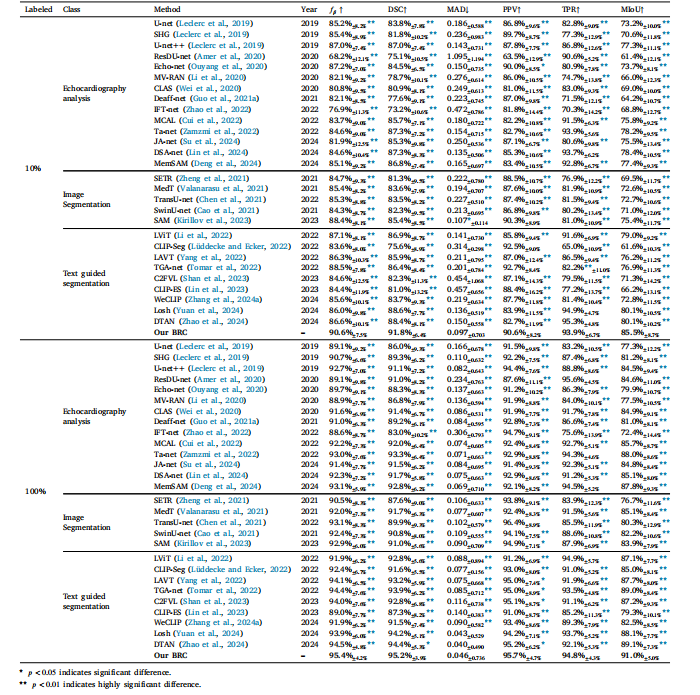
Table 1Comparison with the state-of-the-art segmentation methods in echocardiography on all datasets with different labeled proportions. This further indicates the statistical significancebetween our method and the comparison method (marked with an asterisk ∗). Statistical significance (𝑝-value) is indicated as: 𝑝 < 0.05(∗) and 𝑝 < 0.01(∗∗). Blanks indicate nosignificant difference or superiority to our method.
表1 在所有数据集上,将我们的方法与当前最先进的超声心动图分割方法,在不同标注比例下进行比较。这进一步表明了我们的方法与对比方法之间的统计学显著性差异(用星号∗标记)。统计学显著性(p值)表示为:p < 0.05(∗) 以及 p < 0.01(∗∗)。空白处表示与我们的方法相比无显著差异或未表现出优越性。

Table 2Effectiveness evaluation for different training paradigms on all datasets. 'Visual' and 'Text' debote model trained with visual input and text input.
表2 对所有数据集上不同训练范式的有效性评估。"视觉(Visual)"和"文本(Text)"分别指使用视觉输入和文本输入进行训练的模型。
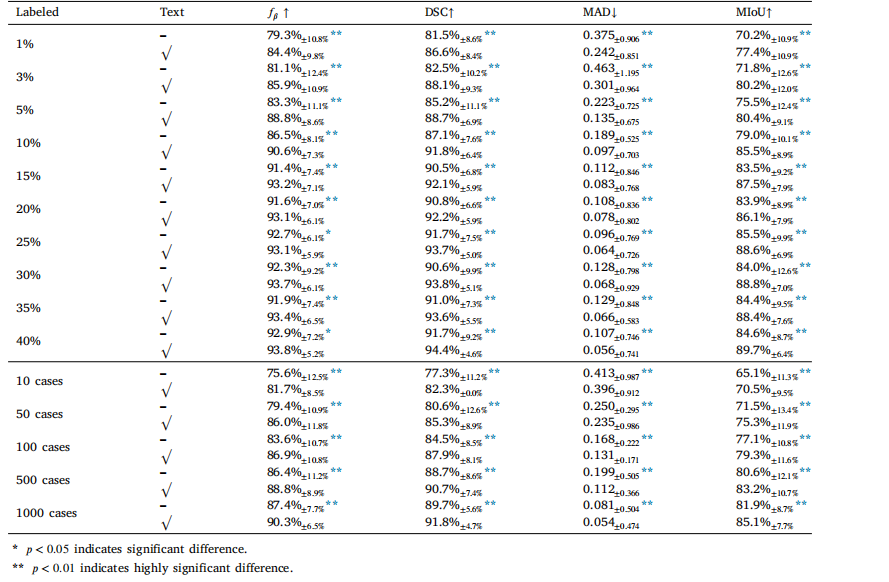
Table 3Effectiveness evaluation of text-guided segmentation on the impact of labeled data proportions and numbers. ' √ ' represents training with textguidance. This further indicates the statistical significance between text-guided and without text-guided segmentation ( marked with an asterisk∗ in the metrics of without text-guided segmentation). Statistical significance (𝑝-value) is indicated as: 𝑝 < 0.05(∗) and 𝑝 < 0.01(∗∗).
表3 对文本引导分割在标注数据比例和数量影响方面的有效性评估。"√"表示使用文本引导进行训练。这进一步表明了文本引导分割与无文本引导分割之间的统计学显著性差异(在无文本引导分割的各项指标中用星号∗标记)。统计学显著性(p值)表示为:p < 0.05(∗) 以及 p < 0.01(∗∗)。