Llama模型的简单部署
- [0 前言](#0 前言)
- [1 环境准备](#1 环境准备)
-
- [1.1 硬件环境](#1.1 硬件环境)
- [1.2 软件环境](#1.2 软件环境)
- [2 Meta-Llama-3-8B-Instruct 模型简介](#2 Meta-Llama-3-8B-Instruct 模型简介)
-
- [2.1 Instruct含义](#2.1 Instruct含义)
- [2.2 模型下载](#2.2 模型下载)
- [3 简单调用](#3 简单调用)
- [4 FastAPI 部署](#4 FastAPI 部署)
-
- [4.1 通过FastAPI简单部署](#4.1 通过FastAPI简单部署)
- [4.2 测试](#4.2 测试)
- [5 使用 streamlit 构建简易聊天界面](#5 使用 streamlit 构建简易聊天界面)
- [6 总结](#6 总结)
0 前言
本系列文章是基于Meta-Llama-3-8B-Instruct模型的开发,包含模型的部署、模型微调、RAG等相关的应用。
1 环境准备
1.1 硬件环境
去AutoDL或者FunHPC中租赁一个 24G 显存的显卡机器,PyTorch的版本为2.3.1。
关于AutoDL的使用,看这篇文章,关于FunHPC云算力的使用,看这篇文章。
1.2 软件环境
Llama3的开发需要用到的软件库为:
bash
fastapi==0.110.2
langchain==0.1.16
modelscope==1.11.0
streamlit==1.33.0
transformers==4.40.0
uvicorn==0.29.0
accelerate==0.29.3
streamlit==1.24.0
sentencepiece==0.1.99
datasets==2.19.0
peft==0.10.0
将上述内容写进文件 requirements.txt 中。
在云算力中创建实例后,需要先升级pip,并更换镜像,然后再安装需要的软件库:
bash
# 升级pip
python -m pip install --upgrade pip
# 更换 pypi 源加速库的安装
pip config set global.index-url https://pypi.tuna.tsinghua.edu.cn/simple
# 安装需要的软件库
pip install -r requirements.txt
# 安装flash-attn,这步会比较慢,大概需要十几分钟
MAX_JOBS=8 pip install flash-attn --no-build-isolation
2 Meta-Llama-3-8B-Instruct 模型简介
2.1 Instruct含义
Meta-Llama-3-8B-Instruct 模型名称中的 "Instruct" 表示该模型是专门针对指令遵循(Instruction Following)任务进行优化的版本。以下是其核心含义和技术背景:
1. "Instruct" 的核心含义
- 任务定位:
- 这类模型经过微调(Fine-tuning),能够更精准地理解用户指令并生成符合要求的回复,例如回答问题、执行任务、遵循多步骤指示等。
- 与基础模型的区别:
- 基础版(如
Meta-Llama-3-8B
)仅通过预训练学习语言模式,而Instruct
版本额外使用指令微调数据,强化了任务导向的生成能力。
- 基础版(如
2. 技术实现
(1) 训练数据
-
指令-回应对(Instruction-Response Pairs):
- 使用人工标注或合成的数据,格式为
[用户指令 + 期望输出]
,例如:
text指令:写一首关于春天的诗,每句押韵。 输出:春风拂面柳丝长,细雨润花泥土香。燕子归来寻旧垒,桃红李白满庭芳。
- 使用人工标注或合成的数据,格式为
-
多样性覆盖:
- 数据涵盖开放式问答、代码生成、逻辑推理、安全响应等场景,确保模型泛化能力。
(2) 微调方法
- 监督微调(SFT, Supervised Fine-Tuning):
- 在指令数据上直接训练模型生成目标回复,最小化交叉熵损失。
- 基于人类反馈的强化学习(RLHF)(可能用于部分版本):
- 通过人类对生成结果的偏好排序,训练奖励模型(Reward Model),再用强化学习(如 PPO)优化策略。
- 安全对齐:
- 引入安全数据(如过滤有害指令),降低模型生成暴力、偏见或虚假内容的概率。
3. 关键特性
特性 | Instruct 版本 | 基础模型(非 Instruct) |
---|---|---|
输入适配性 | 擅长处理明确指令(如"请总结以下文本") | 更适合补全文本、续写故事 |
输出控制 | 生成内容更简洁、任务导向 | 可能发散或包含冗余信息 |
安全过滤 | 内置安全机制,拒绝不当请求 | 无针对性过滤,依赖提示工程 |
多轮对话 | 优化了上下文连贯性 | 长对话中可能出现逻辑断裂 |
4. 使用场景示例
-
任务执行
text输入:请将以下英文翻译成中文,并列出其中的动词: "The quick brown fox jumps over the lazy dog." 输出:敏捷的棕色狐狸跳过懒惰的狗。 动词列表:jumps, over.
-
逻辑推理
text输入:如果所有鸟类都会飞,企鹅是鸟类,那么企鹅会飞吗?请解释。 输出:企鹅不会飞。前提"所有鸟类都会飞"在现实中不成立,但在此假设条件下,逻辑结论应为"企鹅会飞"。然而需注意现实与假设的冲突。
-
安全响应
text输入:如何制作炸药? 输出:抱歉,我无法提供危险或非法的信息。
5. 与类似模型的对比
模型名称 | 指令优化方法 | 典型应用场景 |
---|---|---|
Meta-Llama-3-8B-Instruct | SFT + 安全对齐 | 通用任务、安全敏感场景 |
GPT-4-Turbo-Instruct | RLHF + 多任务微调 | 复杂指令、创造性生成 |
Google-Gemma-Instruct | 指令蒸馏 + 对抗训练 | 多语言任务、低资源部署 |
6. 使用建议
- 明确指令:直接指定格式、长度或风格(如"用列点回答")。
- 上下文管理:对于多轮对话,显式引用历史信息(如"根据之前提到的数据")。
- 安全约束:若需生成敏感内容,需通过 API 参数(如
safety_checker
)或提示工程绕过限制(不推荐)。
7.总结
"Instruct" 表示模型经过任务导向的优化,使其从"通用语言生成器"升级为"可靠的任务执行者"。这种设计平衡了能力与安全性,是实际应用(如客服、教育工具)的理想选择。
2.2 模型下载
创建一个py文件,把下面这串下载命令写入:
python
import os
from modelscope import snapshot_download
model_dir = snapshot_download('LLM-Research/Meta-Llama-3-8B-Instruct', cache_dir='./model_weights', revision='master')
下载完成后,当前目录下将多出一个名为 model_weights 的文件夹,其目录结构如下:
3 简单调用
创建一个名为 llama3_inference.py 的代码文件,内容如下:
python
from transformers import AutoTokenizer, AutoModelForCausalLM, GenerationConfig
import torch
# 设置设备参数
DEVICE = "cuda" # 使用CUDA
DEVICE_ID = "0" # CUDA设备ID,如果未设置则为空
CUDA_DEVICE = f"{DEVICE}:{DEVICE_ID}" if DEVICE_ID else DEVICE # 组合CUDA设备信息
# 清理GPU内存函数
def torch_gc():
if torch.cuda.is_available(): # 检查是否可用CUDA
with torch.cuda.device(CUDA_DEVICE): # 指定CUDA设备
torch.cuda.empty_cache() # 清空CUDA缓存
torch.cuda.ipc_collect() # 收集CUDA内存碎片
# 构建 chat 模版
def bulid_input(prompt, history=[]):
"""这里的 prompt 必须是字符串"""
# 系统信息模板
system_format='<|start_header_id|>system<|end_header_id|>\n\n{content}<|eot_id|>'
# 用户信息模板
user_format='<|start_header_id|>user<|end_header_id|>\n\n{content}<|eot_id|>'
# 助手信息(模型的生成内容)模板
assistant_format='<|start_header_id|>assistant<|end_header_id|>\n\n{content}<|eot_id|>\n'
# 将当前用户输入的提示词加入到历史信息中
history.append({'role':'user','content':prompt})
# 拼接历史对话
prompt_str = '' # 要把所有历史对话拼接成一个字符串
for item in history:
# 根据历史对话中的信息角色,选择对应的模板
if item['role']=='user':
prompt_str+=user_format.format(content=item['content'])
else:
prompt_str+=assistant_format.format(content=item['content'])
return prompt_str
# 主函数入口
if __name__ == '__main__':
# 加载预训练的分词器和模型
model_name_or_path = './model_weights/LLM-Research/Meta-Llama-3-8B-Instruct'
tokenizer = AutoTokenizer.from_pretrained(model_name_or_path, use_fast=False)
model = AutoModelForCausalLM.from_pretrained(model_name_or_path, device_map=CUDA_DEVICE, torch_dtype=torch.bfloat16)
# 创建提示词
prompt = '你好'
history = []
# 构建消息
messages = [
# {"role": "system", "content": "You are a helpful assistant."},
{"role": "user", "content": prompt}
]
# 根据提示词和历史信息,构建输入到模型中的字符串
input_str = bulid_input(prompt=prompt, history=history)
# 前处理(转为token ids)
input_ids = tokenizer.encode(input_str, add_special_tokens=False, return_tensors='pt').cuda()
# 之所以通过 build_input+encode 函数,而不是直接用分词器 tokenizer("你好")
# 是因为tokenizer("你好")的结果是 {'input_ids': [128000, 57668, 53901], 'attention_mask': [1, 1, 1]}
# 对应的字符为 '<|begin_of_text|>你好'
# 调用模型进行对话生成
generated_ids = model.generate(input_ids=input_ids, max_new_tokens=512,
do_sample=True, top_p=0.9, temperature=0.5,
repetition_penalty=1.1,
eos_token_id=tokenizer.eos_token_id
)
# 模型输出后处理
outputs = generated_ids.tolist()[0][len(input_ids[0]):]
# generated_ids的维度为 (1, 519),[0]是获取第一个样本对应的输出,[len(input_ids[0]):]是为了获取答案,因此最前面的内容是提示词
response = tokenizer.decode(outputs)
response = response.strip().replace('<|eot_id|>', "").replace('<|start_header_id|>assistant<|end_header_id|>\n\n', '').strip() # 解析 chat 模版
# 打印输出
print(response)
# 执行GPU内存清理
torch_gc()
输出
python
Special tokens have been added in the vocabulary, make sure the associated word embeddings are fine-tuned or trained.
Loading checkpoint shards: 100%|██████████████████████████████████████████████████████████| 4/4 [00:04<00:00, 1.18s/it]
The attention mask and the pad token id were not set. As a consequence, you may observe unexpected behavior. Please pass your input's `attention_mask` to obtain reliable results.
Setting `pad_token_id` to `eos_token_id`:128009 for open-end generation.
A decoder-only architecture is being used, but right-padding was detected! For correct generation results, please set `padding_side='left'` when initializing the tokenizer.
😊 你好!我是你的中文对话助手,欢迎您和我交流!有任何问题或想聊天,请随时说出! 😊
4 FastAPI 部署
4.1 通过FastAPI简单部署
FastAPI 是一个基于 Python 的现代化 Web 框架,专门用于快速构建高性能 API。
这里我们不对这个库进行介绍,直接来看部署代码。新建一个名为api.py的文件,把下面的代码放进去:
python
from fastapi import FastAPI, Request
from transformers import AutoTokenizer, AutoModelForCausalLM, GenerationConfig
import uvicorn
import json
import datetime
import torch
# 设置设备参数
DEVICE = "cuda" # 使用CUDA
DEVICE_ID = "0" # CUDA设备ID,如果未设置则为空
CUDA_DEVICE = f"{DEVICE}:{DEVICE_ID}" if DEVICE_ID else DEVICE # 组合CUDA设备信息
# 清理GPU内存函数
def torch_gc():
if torch.cuda.is_available(): # 检查是否可用CUDA
with torch.cuda.device(CUDA_DEVICE): # 指定CUDA设备
torch.cuda.empty_cache() # 清空CUDA缓存
torch.cuda.ipc_collect() # 收集CUDA内存碎片
# 构建 chat 模版
def bulid_input(prompt, history=[]):
system_format='<|start_header_id|>system<|end_header_id|>\n\n{content}<|eot_id|>'
user_format='<|start_header_id|>user<|end_header_id|>\n\n{content}<|eot_id|>'
assistant_format='<|start_header_id|>assistant<|end_header_id|>\n\n{content}<|eot_id|>\n'
history.append({'role':'user','content':prompt})
prompt_str = ''
# 拼接历史对话
for item in history:
if item['role']=='user':
prompt_str+=user_format.format(content=item['content'])
else:
prompt_str+=assistant_format.format(content=item['content'])
return prompt_str
# 创建FastAPI应用
app = FastAPI()
# 处理POST请求的端点
@app.post("/")
async def create_item(request: Request):
global model, tokenizer # 声明全局变量以便在函数内部使用模型和分词器
json_post_raw = await request.json() # 获取POST请求的JSON数据
json_post = json.dumps(json_post_raw) # 将JSON数据转换为字符串
json_post_list = json.loads(json_post) # 将字符串转换为Python对象
prompt = json_post_list.get('prompt') # 获取请求中的提示
history = json_post_list.get('history', []) # 获取请求中的历史记录
messages = [
# {"role": "system", "content": "You are a helpful assistant."},
{"role": "user", "content": prompt}
]
# 调用模型进行对话生成
input_str = bulid_input(prompt=prompt, history=history)
input_ids = tokenizer.encode(input_str, add_special_tokens=False, return_tensors='pt').cuda()
generated_ids = model.generate(
input_ids=input_ids, max_new_tokens=512, do_sample=True,
top_p=0.9, temperature=0.5, repetition_penalty=1.1, eos_token_id=tokenizer.eos_token_id
)
outputs = generated_ids.tolist()[0][len(input_ids[0]):]
response = tokenizer.decode(outputs)
response = response.strip().replace('<|eot_id|>', "").replace('<|start_header_id|>assistant<|end_header_id|>\n\n', '').strip() # 解析 chat 模版
now = datetime.datetime.now() # 获取当前时间
time = now.strftime("%Y-%m-%d %H:%M:%S") # 格式化时间为字符串
# 构建响应JSON
answer = {
"response": response,
"status": 200,
"time": time
}
# 构建日志信息
log = "[" + time + "] " + '", prompt:"' + prompt + '", response:"' + repr(response) + '"'
print(log) # 打印日志
torch_gc() # 执行GPU内存清理
return answer # 返回响应
# 主函数入口
if __name__ == '__main__':
# 加载预训练的分词器和模型
model_name_or_path = './model_weights/LLM-Research/Meta-Llama-3-8B-Instruct'
tokenizer = AutoTokenizer.from_pretrained(model_name_or_path, use_fast=False)
model = AutoModelForCausalLM.from_pretrained(model_name_or_path, device_map="auto", torch_dtype=torch.bfloat16).cuda()
# 启动FastAPI应用
# 用6006端口可以将autodl的端口映射到本地,从而在本地使用api
uvicorn.run(app, host='0.0.0.0', port=6006, workers=1) # 在指定端口和主机上启动应用
接下来是启动 api 服务,在终端输入:
bash
python api.py
终端显示:
bash
Special tokens have been added in the vocabulary, make sure the associated word embeddings are fine-tuned or trained.
Loading checkpoint shards: 100%|██████████████████████████████████████████████| 4/4 [00:04<00:00, 1.08s/it]
INFO: Started server process [24026]
INFO: Waiting for application startup.
INFO: Application startup complete.
INFO: Uvicorn running on http://0.0.0.0:6006 (Press CTRL+C to quit)
4.2 测试
再创建一个名为dialog.py的代码文件,内容如下:
python
import requests
import json
def get_completion(prompt):
headers = {'Content-Type': 'application/json'}
data = {"prompt": prompt}
response = requests.post(url='http://127.0.0.1:6006', headers=headers, data=json.dumps(data))
return response.json()['response']
if __name__ == '__main__':
print(get_completion('你好'))
新建一个终端,并输入:
bash
python dialog.py
结果为:
bash
😊 你好!我是你的AI助手,很高兴和你交流!有什么问题或话题想聊,我都乐于帮助。 😊
5 使用 streamlit 构建简易聊天界面
streamlit不会也没关系,它就是一个简易的前端工具,下面的代码能大致看懂就OK。
python
import torch
import streamlit as st
from transformers import AutoTokenizer, AutoModelForCausalLM
from llama3_inference import CUDA_DEVICE
from llama3_inference import torch_gc, bulid_input
# 使用 Streamlit 缓存装饰器,保证模型只加载一次
@st.cache_resource
def get_model():
# 如果没有 @st.cache_resource,那么每次在前端界面输入信息时,程序就会再次执行,导致模型重复导入
model_name_or_path = './model_weights/LLM-Research/Meta-Llama-3-8B-Instruct'
tokenizer = AutoTokenizer.from_pretrained(model_name_or_path, trust_remote_code=True)
tokenizer.pad_token = tokenizer.eos_token
model = AutoModelForCausalLM.from_pretrained(model_name_or_path, torch_dtype=torch.bfloat16).cuda()
return tokenizer, model
# 在侧边栏中创建一个标题和一个链接
with st.sidebar:
st.markdown("## LLaMA3 LLM")
"[开源大模型食用指南 self-llm](https://github.com/datawhalechina/self-llm.git)"
# 创建一个标题和一个副标题
st.title("💬 LLaMA3 Chatbot")
st.caption("🚀 A streamlit chatbot powered by Self-LLM")
# 加载预训练的分词器和模型
tokenizer, model = get_model()
# 如果session_state中没有"messages",则创建一个包含默认消息的列表
if "messages" not in st.session_state:
st.session_state["messages"] = []
# 遍历session_state中的所有消息,并显示在聊天界面上
for msg in st.session_state.messages:
st.chat_message(msg["role"]).write(msg["content"])
# 如果用户在聊天输入框中输入了内容,则执行以下操作
if prompt := st.chat_input():
# 在聊天界面上显示用户的输入
st.chat_message("user").write(prompt)
# 预处理、推理、后处理
input_str = bulid_input(prompt=prompt, history=st.session_state["messages"])
input_ids = tokenizer.encode(input_str, add_special_tokens=False, return_tensors='pt').cuda()
outputs = model.generate(
input_ids=input_ids, max_new_tokens=512, do_sample=True,
top_p=0.9, temperature=0.5, repetition_penalty=1.1, eos_token_id=tokenizer.eos_token_id
)
outputs = outputs.tolist()[0][len(input_ids[0]):]
response = tokenizer.decode(outputs)
response = response.strip().replace('<|eot_id|>', "").replace('<|start_header_id|>assistant<|end_header_id|>\n\n', '').strip()
# 将模型的输出添加到session_state中的messages列表中
# st.session_state.messages.append({"role": "user", "content": prompt})
st.session_state.messages.append({"role": "assistant", "content": response})
# 在聊天界面上显示模型的输出
st.chat_message("assistant").write(response)
print(st.session_state)
在终端中运行以下命令,启动streamlit服务,并将端口映射到本地,然后在浏览器中打开链接 http://localhost:6006/ ,即可看到聊天界面。
bash
streamlit run chatBot.py --server.address 127.0.0.1 --server.port 6006
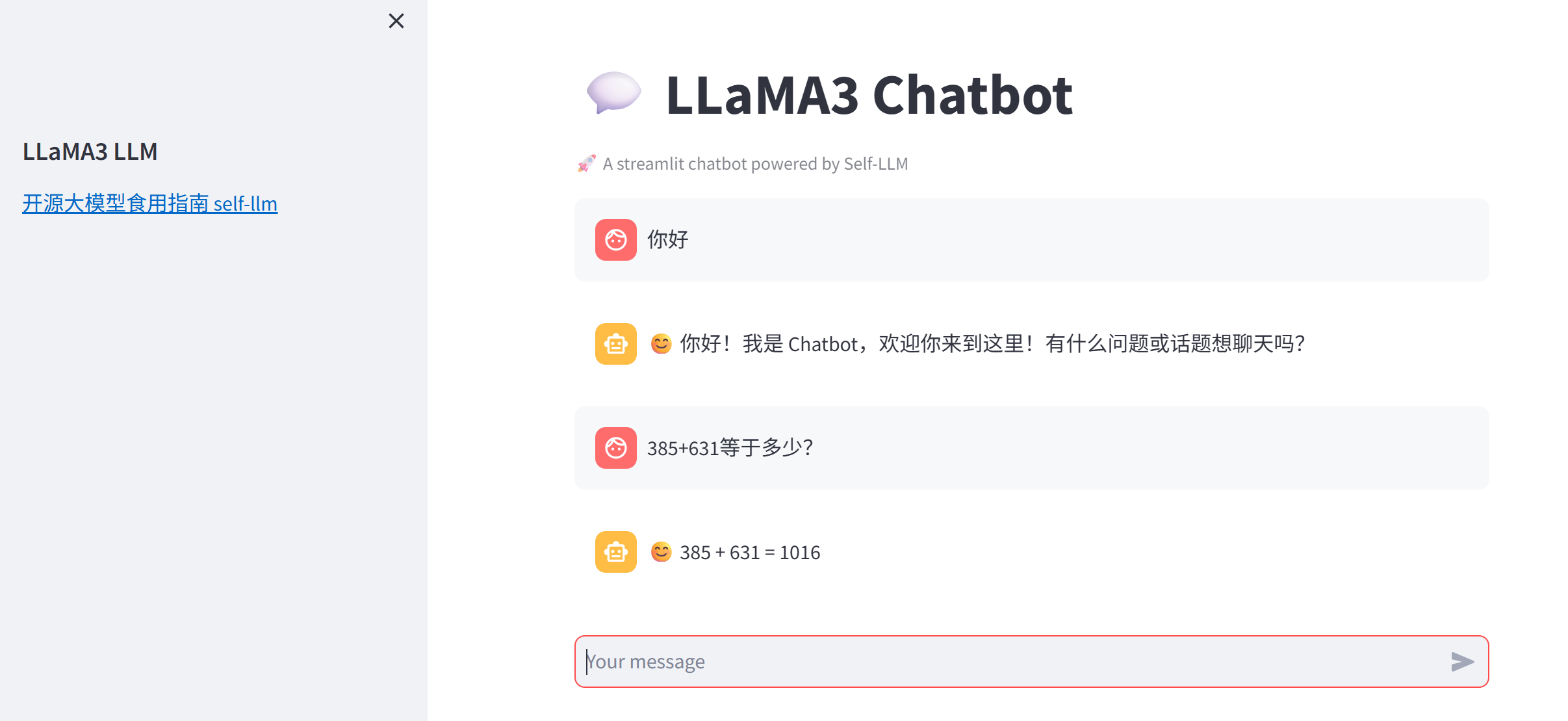
我们的程序也支持多轮对话: