数据变换 Transforms
在完成数据加载后,还应该对数据进行预处理。之前在数据集篇介绍过map函数,这里的transform就是和map一起使用的。transform有针对图像、文本、音频等不同类型的,并且也支持lambda函数。
环境配置
python
import numpy as np
from PIL import Image
from download import download
from mindspore.dataset import transforms, vision, text
from mindspore.dataset import GeneratorDataset, MnistDataset
基础变换
以下由图像变换和文字变换展开。
Vision transform
Compose是接受一个数据增强操作序列,再将其组合成单个数据增强操作。(实际上就是做组合)
python
composed = transforms.Compose(
[
vision.Rescale(1.0 / 255.0, 0),
vision.Normalize(mean=(0.1307,), std=(0.3081,)),
vision.HWC2CHW()
]
)
train_dataset = train_dataset.map(composed, 'image')
之前简单介绍过上面的三个操作。
-
rescale 调整图像像素值,包括rescale 缩放因子、shift 平移因子
-
对于每个像素都根据这两个参数进行调整 o u t p u t i = i n p u t i ∗ r e s c a l e + s h i f t output_{i} = input_{i} * rescale + shift outputi=inputi∗rescale+shift。
-
normalize 输入图像归一化,包括 mean 通道均值、std通道标准差、is_hwc 输入图像格式(是bool值,True为(height, width, channel),False为(channel, height, width))
-
o u t p u t c = i n p u t c − m e a n c s t d c output_{c} = \frac{input_{c} - mean_{c}}{std_{c}} outputc=stdcinputc−meanc,其中 c c c代表通道索引。
-
HWC2CHW 转换图片格式,(height, width, channel)或(channel, height, width)互转。
Text transform
文本数据需要做分词、词表构建等操作
- PythonTokenizer
python
def my_tokenizer(content):
return content.split()
# texts 内容是 'Welcome to Beijing'
test_dataset = test_dataset.map(text.PythonTokenizer(my_tokenizer))
# 分词后变成了 'Welcome', 'to', 'Beijing'
- Lookup
词表映射变换,用来将Token转换为Index。在此之前需要先构建词表。可以使用已有的词表或者使用Vocab生成词表。
python
# 从数据集里构建词表
vocab = text.Vocab.from_dataset(test_dataset)
print(vocab.vocab())
# {'to': 2, 'Beijing': 0, 'Welcome': 1}
# 词表生成后再查询索引
test_dataset = test_dataset.map(text.Lookup(vocab))
print(next(test_dataset.create_tuple_iterator()))
# [Tensor(shape=[3], dtype=Int32, value= [1, 2, 0])]
- Lambda Transforms
lambda函数也就是内嵌在语句中的匿名函数,具体可以查看 从0到0.1学习 lambda表达式
python
test_dataset = GeneratorDataset([1, 2, 3], 'data', shuffle=False)
test_dataset = test_dataset.map(lambda x: x * 2)
print(list(test_dataset.create_tuple_iterator()))
# 输出 2 4 6
def func(x):
return x * x + 2
test_dataset = test_dataset.map(lambda x: func(x))
# 输出 6 18 38
总结
本节学习了图片和文字表的一些转换的基本操作
打卡凭证
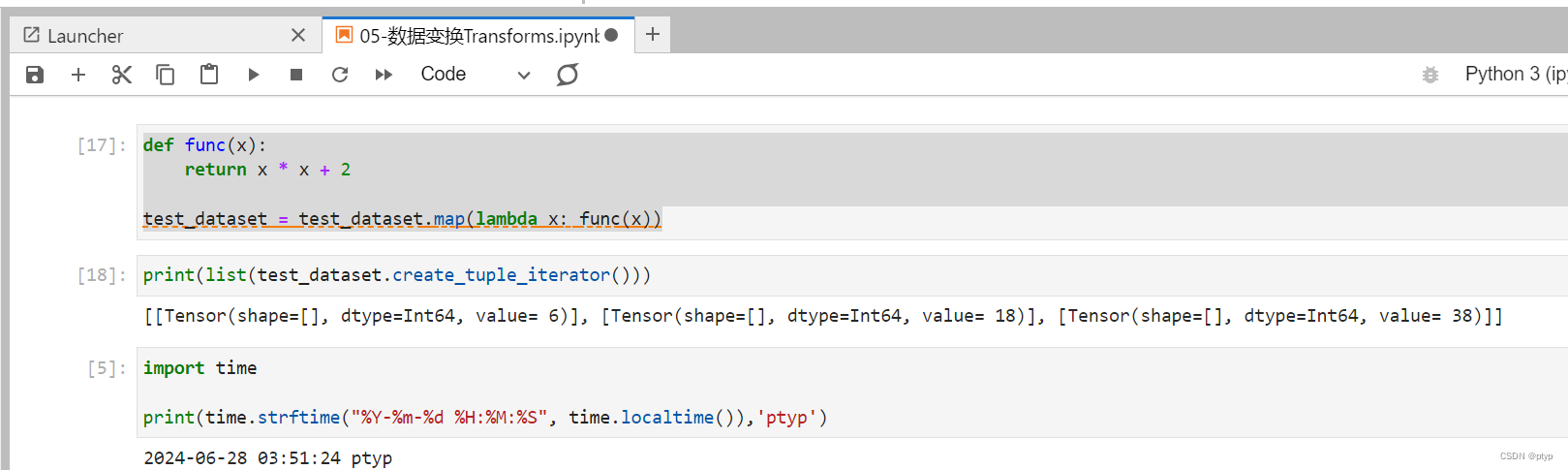