- 🍨 本文为🔗365天深度学习训练营 中的学习记录博客
- 🍖 原作者:K同学啊# 前言
前言
这周学习训练一个Word2Vec模型,并进行一些基本的词向量操作。
Word2Vec 模型
Word2Vec 是一种基于神经网络的词向量表示方法,通过从大规模文本语料中学习到的词向量,捕捉词汇之间的语义关系。
训练一个Word2Vec模型
1. 导入所需的库
python
import jieba
import jieba.analyse
import chardet
jieba
用于中文分词。chardet
用于检测文件编码。jieba.analyse
用于关键词提取(未在本代码中使用)。
2. 添加自定义词频
python
jieba.suggest_freq('沙瑞金', True)
# ... (其他类似的词)
jieba.suggest_freq('赵德汉', True)
suggest_freq
方法用于调整词频,使得分词器能够更好地识别这些特定词汇。
3. 读取和分词处理文本文件
python
result_cut = []
with open('./in_the_name_of_people.txt', 'rb') as f:
raw_data = f.read()
encoding = chardet.detect(raw_data)['encoding']
lines = raw_data.decode(encoding).splitlines()
for line in lines:
result_cut.append(list(jieba.cut(line)))
- 以二进制方式读取文本文件内容。
- 使用
chardet
检测文件编码,并进行解码。 - 将文本按行分割,并对每行使用
jieba.cut
进行分词,结果存入result_cut
列表。
4. 去除停用词
python
stopwords_list = [",", "。", "\n", "\u3000", " ", ":", "!", "?", "..."]
def remove_stopwords(ls):
return [word for word in ls if word not in stopwords_list]
result_stop = [remove_stopwords(x) for x in result_cut if remove_stopwords(x)]
- 定义一个停用词列表,包括常见的标点符号和空格。
remove_stopwords
函数用于从分词结果中去除停用词。- 对分词结果
result_cut
应用remove_stopwords
函数,得到result_stop
。
5. 训练Word2Vec模型
python
from gensim.models import Word2Vec
model = Word2Vec(result_stop,
vector_size=100,
window=5,
min_count=1)
- 使用
gensim
库的Word2Vec
模型训练词向量。 vector_size
设置词向量的维度为100。window
设置上下文窗口大小为5。min_count
设置为1,即出现次数少于1次的词语将被忽略。
6. 计算词语相似度
python
print(model.wv.similarity('沙瑞金', '季昌明'))
print(model.wv.similarity('沙瑞金', '田国富'))
- 使用
similarity
方法计算两个词语之间的相似度。
7. 找出最相似的词
python
for e in model.wv.most_similar(positive=['沙瑞金'], topn=5):
print(e[0], e[1])
- 使用
most_similar
方法找出与'沙瑞金'最相似的5个词语及其相似度。
8. 找出不匹配的词
python
odd_word = model.wv.doesnt_match(["苹果", "香蕉", "橙子", "书"])
print(f"在这组词汇中不匹配的词汇:{odd_word}")
- 使用
doesnt_match
方法找出列表中最不符合其余词语的词语。
9. 获取词频
python
word_frequency = model.wv.get_vecattr("沙瑞金", "count")
print(f"沙瑞金:{word_frequency}")
- 使用
get_vecattr
方法获取词语'沙瑞金'在语料中的出现次数。
结果
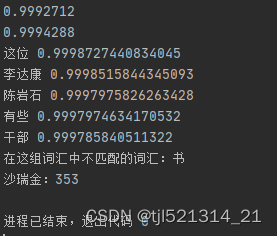
总结
通过Word2Vec模型,我们可以有效地捕捉词汇之间的语义关系,应用在自然语言处理任务中如文本分类、聚类和推荐系统等。