昇思25天学习打卡营第16天 | Vision Transformer图像分类
文章目录
- [昇思25天学习打卡营第16天 | Vision Transformer图像分类](#昇思25天学习打卡营第16天 | Vision Transformer图像分类)
Vision Transform(ViT)模型
ViT是NLP和CV领域的融合,可以在不依赖于卷积操作的情况下在图像分类任务上达到很好的效果。
ViT模型的主体结构是基于Transformer的Encoder部分。
Transformer
Transformer由很多Encoder和Decoder模块构成,包括多头注意力(Multi-Head Attention)层,Feed Forward层,Normalization层和残差连接(Residual Connection)。
多头注意力结构基于自注意力机制(Self-Attention),是多个Self-Attention的并行组成。
Attention模块
Attention的核心在于为输入向量的每个单词学习一个权重。
- 最初的输入向量首先经过Embedding层映射为Q(Query),K(Key),V(Value)三个向量。
- 通过将Q和所有K进行点乘初一维度平方根,得到向量间的相似度,通过softmax获取每词向量之间的关系权重。
- 利用关系权重对词向量的V加权求和,得到自注意力值。
多头注意力机制只是对self-attention的并行化:
Encoder模块
ViT中的Encoder相对于标准Transformer,主要在于将Normolization放在self-attention和Feed Forward之前,其他结构与标准Transformer相同。
ViT模型输入
传统Transformer主要应用于自然语言处理的一维词向量,而图像时二维矩阵的堆叠。
在ViT中:
- 通过卷积将输入图像在每个channel上划分为 16 × 16 16\times 16 16×16个patch。如果输入 224 × 224 224\times224 224×224的图像,则每一个patch的大小为 14 × 14 14\times 14 14×14。
- 将每一个patch拉伸为一个一维向量,得到近似词向量堆叠的效果。如将 14 × 14 14\times14 14×14展开为 196 196 196的向量。
这一部分Patch Embedding用来替换Transformer中Word Embedding,用作网络中的图像输入。
模型构建
Multi-Head Attention模块
python
from mindspore import nn, ops
class Attention(nn.Cell):
def __init__(self,
dim: int,
num_heads: int = 8,
keep_prob: float = 1.0,
attention_keep_prob: float = 1.0):
super(Attention, self).__init__()
self.num_heads = num_heads
head_dim = dim // num_heads
self.scale = ms.Tensor(head_dim ** -0.5)
self.qkv = nn.Dense(dim, dim * 3)
self.attn_drop = nn.Dropout(p=1.0-attention_keep_prob)
self.out = nn.Dense(dim, dim)
self.out_drop = nn.Dropout(p=1.0-keep_prob)
self.attn_matmul_v = ops.BatchMatMul()
self.q_matmul_k = ops.BatchMatMul(transpose_b=True)
self.softmax = nn.Softmax(axis=-1)
def construct(self, x):
"""Attention construct."""
b, n, c = x.shape
qkv = self.qkv(x)
qkv = ops.reshape(qkv, (b, n, 3, self.num_heads, c // self.num_heads))
qkv = ops.transpose(qkv, (2, 0, 3, 1, 4))
q, k, v = ops.unstack(qkv, axis=0)
attn = self.q_matmul_k(q, k)
attn = ops.mul(attn, self.scale)
attn = self.softmax(attn)
attn = self.attn_drop(attn)
out = self.attn_matmul_v(attn, v)
out = ops.transpose(out, (0, 2, 1, 3))
out = ops.reshape(out, (b, n, c))
out = self.out(out)
out = self.out_drop(out)
return out
Encoder模块
python
from typing import Optional, Dict
class FeedForward(nn.Cell):
def __init__(self,
in_features: int,
hidden_features: Optional[int] = None,
out_features: Optional[int] = None,
activation: nn.Cell = nn.GELU,
keep_prob: float = 1.0):
super(FeedForward, self).__init__()
out_features = out_features or in_features
hidden_features = hidden_features or in_features
self.dense1 = nn.Dense(in_features, hidden_features)
self.activation = activation()
self.dense2 = nn.Dense(hidden_features, out_features)
self.dropout = nn.Dropout(p=1.0-keep_prob)
def construct(self, x):
"""Feed Forward construct."""
x = self.dense1(x)
x = self.activation(x)
x = self.dropout(x)
x = self.dense2(x)
x = self.dropout(x)
return x
class ResidualCell(nn.Cell):
def __init__(self, cell):
super(ResidualCell, self).__init__()
self.cell = cell
def construct(self, x):
"""ResidualCell construct."""
return self.cell(x) + x
class TransformerEncoder(nn.Cell):
def __init__(self,
dim: int,
num_layers: int,
num_heads: int,
mlp_dim: int,
keep_prob: float = 1.,
attention_keep_prob: float = 1.0,
drop_path_keep_prob: float = 1.0,
activation: nn.Cell = nn.GELU,
norm: nn.Cell = nn.LayerNorm):
super(TransformerEncoder, self).__init__()
layers = []
for _ in range(num_layers):
normalization1 = norm((dim,))
normalization2 = norm((dim,))
attention = Attention(dim=dim,
num_heads=num_heads,
keep_prob=keep_prob,
attention_keep_prob=attention_keep_prob)
feedforward = FeedForward(in_features=dim,
hidden_features=mlp_dim,
activation=activation,
keep_prob=keep_prob)
layers.append(
nn.SequentialCell([
ResidualCell(nn.SequentialCell([normalization1, attention])),
ResidualCell(nn.SequentialCell([normalization2, feedforward]))
])
)
self.layers = nn.SequentialCell(layers)
def construct(self, x):
"""Transformer construct."""
return self.layers(x)
Patch Embedding模块
python
class PatchEmbedding(nn.Cell):
MIN_NUM_PATCHES = 4
def __init__(self,
image_size: int = 224,
patch_size: int = 16,
embed_dim: int = 768,
input_channels: int = 3):
super(PatchEmbedding, self).__init__()
self.image_size = image_size
self.patch_size = patch_size
self.num_patches = (image_size // patch_size) ** 2
self.conv = nn.Conv2d(input_channels, embed_dim, kernel_size=patch_size, stride=patch_size, has_bias=True)
def construct(self, x):
"""Path Embedding construct."""
x = self.conv(x)
b, c, h, w = x.shape
x = ops.reshape(x, (b, c, h * w))
x = ops.transpose(x, (0, 2, 1))
return x
ViT网络
python
from mindspore.common.initializer import Normal
from mindspore.common.initializer import initializer
from mindspore import Parameter
def init(init_type, shape, dtype, name, requires_grad):
"""Init."""
initial = initializer(init_type, shape, dtype).init_data()
return Parameter(initial, name=name, requires_grad=requires_grad)
class ViT(nn.Cell):
def __init__(self,
image_size: int = 224,
input_channels: int = 3,
patch_size: int = 16,
embed_dim: int = 768,
num_layers: int = 12,
num_heads: int = 12,
mlp_dim: int = 3072,
keep_prob: float = 1.0,
attention_keep_prob: float = 1.0,
drop_path_keep_prob: float = 1.0,
activation: nn.Cell = nn.GELU,
norm: Optional[nn.Cell] = nn.LayerNorm,
pool: str = 'cls') -> None:
super(ViT, self).__init__()
self.patch_embedding = PatchEmbedding(image_size=image_size,
patch_size=patch_size,
embed_dim=embed_dim,
input_channels=input_channels)
num_patches = self.patch_embedding.num_patches
self.cls_token = init(init_type=Normal(sigma=1.0),
shape=(1, 1, embed_dim),
dtype=ms.float32,
name='cls',
requires_grad=True)
self.pos_embedding = init(init_type=Normal(sigma=1.0),
shape=(1, num_patches + 1, embed_dim),
dtype=ms.float32,
name='pos_embedding',
requires_grad=True)
self.pool = pool
self.pos_dropout = nn.Dropout(p=1.0-keep_prob)
self.norm = norm((embed_dim,))
self.transformer = TransformerEncoder(dim=embed_dim,
num_layers=num_layers,
num_heads=num_heads,
mlp_dim=mlp_dim,
keep_prob=keep_prob,
attention_keep_prob=attention_keep_prob,
drop_path_keep_prob=drop_path_keep_prob,
activation=activation,
norm=norm)
self.dropout = nn.Dropout(p=1.0-keep_prob)
self.dense = nn.Dense(embed_dim, num_classes)
def construct(self, x):
"""ViT construct."""
x = self.patch_embedding(x)
cls_tokens = ops.tile(self.cls_token.astype(x.dtype), (x.shape[0], 1, 1))
x = ops.concat((cls_tokens, x), axis=1)
x += self.pos_embedding
x = self.pos_dropout(x)
x = self.transformer(x)
x = self.norm(x)
x = x[:, 0]
if self.training:
x = self.dropout(x)
x = self.dense(x)
return x
总结
这一节对Transformer进行介绍,包括Attention机制、并行化的Attention以及Encoder模块。由于传统Transformer主要作用于一维的词向量,因此二维图像需要被转换为类似的一维词向量堆叠,在ViT中通过将Patch Embedding解决这一问题,并用来代替传统Transformer中的Word Embedding作为网络的输入。
打卡
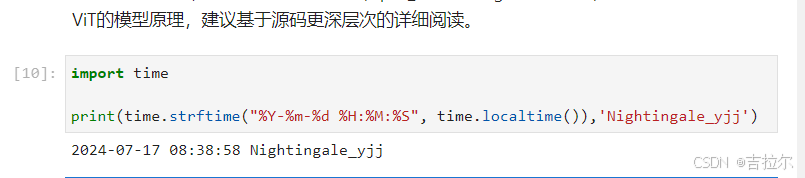