🍨 本文为🔗365天深度学习训练营 中的学习记录博客
🍖 原作者:K同学啊
文章目录
电脑环境:
语言环境:Python 3.8.0
编译器:Jupyter Notebook
深度学习环境:tensorflow 2.15.0
一、前期工作
1.设置GPU(如果使用的是CPU可以忽略这步)
python
from tensorflow import keras
from keras import layers, models
import os, PIL, pathlib
import matplotlib.pyplot as plt
import tensorflow as tf
gpus = tf.config.list_physical_devices("GPU")
if gpus:
gpu0 = gpus[0] #如果有多个GPU,仅使用第0个GPU
tf.config.experimental.set_memory_growth(gpu0, True) #设置GPU显存用量按需使用
tf.config.set_visible_devices([gpu0],"GPU")
2. 导入数据
python
data_dir = "./data/"
data_dir = pathlib.Path(data_dir)
3. 查看数据
python
image_count = len(list(data_dir.glob('*/*/*.jpg')))
print("图片总数为:",image_count)
输出:图片总数为: 578
打开一张图片:
python
Monkeypox = list(data_dir.glob('train/nike/*.jpg'))
PIL.Image.open(str(Monkeypox[1]))
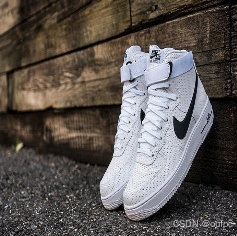
二、数据预处理
1、加载数据
使用image_dataset_from_directory方法将磁盘中的数据加载到tf.data.Dataset中。
python
batch_size = 32
img_height = 224
img_width = 224
train_ds = tf.keras.preprocessing.image_dataset_from_directory(
"./data/train/",
seed=123,
image_size=(img_height, img_width),
batch_size=batch_size)
val_ds = tf.keras.preprocessing.image_dataset_from_directory(
"./data/test/",
seed=123,
image_size=(img_height, img_width),
batch_size=batch_size)
我们可以通过class_names输出数据集的标签。标签将按字母顺序对应于目录名称。
python
class_names = train_ds.class_names
print(class_names)
输出:
'adidas', 'nike'
2、数据可视化
python
plt.figure(figsize=(20, 10))
for images, labels in train_ds.take(1):
for i in range(20):
ax = plt.subplot(5, 10, i + 1)
plt.imshow(images[i].numpy().astype("uint8"))
plt.title(class_names[labels[i]])
plt.axis("off")
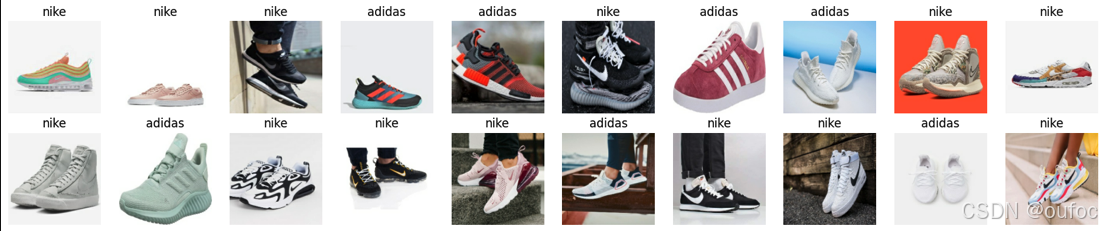
3、再次检查数据
python
for image_batch, labels_batch in train_ds:
print(image_batch.shape)
print(labels_batch.shape)
break
输出:
(32, 224, 224, 3)
(32,)
4、配置数据集
python
AUTOTUNE = tf.data.AUTOTUNE
train_ds = train_ds.cache().shuffle(1000).prefetch(buffer_size=AUTOTUNE)
val_ds = val_ds.cache().prefetch(buffer_size=AUTOTUNE)
三、构建CNN网络
python
"""
关于卷积核的计算不懂的可以参考文章:https://blog.csdn.net/qq_38251616/article/details/114278995
layers.Dropout(0.4) 作用是防止过拟合,提高模型的泛化能力。
关于Dropout层的更多介绍可以参考文章:https://mtyjkh.blog.csdn.net/article/details/115826689
"""
model = models.Sequential([
layers.experimental.preprocessing.Rescaling(1./255, input_shape=(img_height, img_width, 3)),
layers.Conv2D(16, (3, 3), activation='relu', input_shape=(img_height, img_width, 3)), # 卷积层1,卷积核3*3
layers.AveragePooling2D((2, 2)), # 池化层1,2*2采样
layers.Conv2D(32, (3, 3), activation='relu'), # 卷积层2,卷积核3*3
layers.AveragePooling2D((2, 2)), # 池化层2,2*2采样
layers.Dropout(0.3),
layers.Conv2D(64, (3, 3), activation='relu'), # 卷积层3,卷积核3*3
layers.Dropout(0.3),
layers.Flatten(), # Flatten层,连接卷积层与全连接层
layers.Dense(128, activation='relu'), # 全连接层,特征进一步提取
layers.Dense(len(class_names)) # 输出层,输出预期结果
])
model.summary() # 打印网络结构
四、训练模型
1、设置动态学习率
ExponentialDecay函数:
tf.keras.optimizers.schedules.ExponentialDecay是 TensorFlow 中的一个学习率衰减策略,用于在训练神经网络时动态地降低学习率。学习率衰减是一种常用的技巧,可以帮助优化算法更有效地收敛到全局最小值,从而提高模型的性能。
主要参数:
- initial_learning_rate(初始学习率):初始学习率大小。
- decay_steps(衰减步数):学习率衰减的步数。在经过 decay_steps 步后,学习率将按照指数函数衰减。例如,如果 decay_steps 设置为 10,则每10步衰减一次。
- decay_rate(衰减率):学习率的衰减率。它决定了学习率如何衰减。通常,取值在 0 到 1 之间。
- staircase(阶梯式衰减):一个布尔值,控制学习率的衰减方式。如果设置为 True,则学习率在每个 decay_steps 步之后直接减小,形成阶梯状下降。如果设置为 False,则学习率将连续衰减。
python
# 设置初始学习率
initial_learning_rate = 0.1
lr_schedule = tf.keras.optimizers.schedules.ExponentialDecay(
initial_learning_rate,
decay_steps=10, # 敲黑板!!!这里是指 steps,不是指epochs
decay_rate=0.92, # lr经过一次衰减就会变成 decay_rate*lr
staircase=True)
# 将指数衰减学习率送入优化器
optimizer = tf.keras.optimizers.Adam(learning_rate=lr_schedule)
model.compile(optimizer=optimizer,
loss=tf.keras.losses.SparseCategoricalCrossentropy(from_logits=True),
metrics=['accuracy'])
注:这里设置的动态学习率为:指数衰减型(ExponentialDecay)。在每一个epoch开始前,学习率(learning_rate)都将会重置为初始学习率(initial_learning_rate),然后再重新开始衰减。计算公式如下:
learning_rate = initial_learning_rate * decay_rate ^ (step / decay_steps)
2、早停与保存最佳模型参数
EarlyStopping()参数说明:
- monitor: 被监测的数据。
- min_delta: 在被监测的数据中被认为是提升的最小变化, 例如,小于 min_delta 的绝对变化会被认为没有提升。
- patience: 没有进步的训练轮数,在这之后训练就会被停止。
- verbose: 详细信息模式。
- mode: {auto, min, max} 其中之一。 在 min 模式中, 当被监测的数据停止下降,训练就会停止;在 max 模式中,当被监测的数据停止上升,训练就会停止;在 auto 模式中,方向会自动从被监测的数据的名字中判断出来。
- baseline: 要监控的数量的基准值。 如果模型没有显示基准的改善,训练将停止。
- estore_best_weights: 是否从具有监测数量的最佳值的时期恢复模型权重。 如果为 False,则使用在训练的最后一步获得的模型权重。
python
from tensorflow.keras.callbacks import ModelCheckpoint, EarlyStopping
epochs = 50
# 保存最佳模型参数
checkpointer = ModelCheckpoint('best_model.h5',
monitor='val_accuracy',
verbose=1,
save_best_only=True,
save_weights_only=True)
# 设置早停
earlystopper = EarlyStopping(monitor='val_accuracy',
min_delta=0.001,
patience=20,
verbose=1)
3、模型训练
python
history = model.fit(train_ds,
validation_data=val_ds,
epochs=epochs,
callbacks=[checkpointer, earlystopper])
python
Epoch 1/50
15/16 [===========================>..] - ETA: 0s - loss: 0.7137 - accuracy: 0.5021
Epoch 1: val_accuracy improved from -inf to 0.50000, saving model to best_model.h5
16/16 [==============================] - 3s 73ms/step - loss: 0.7141 - accuracy: 0.4920 - val_loss: 0.6969 - val_accuracy: 0.5000
Epoch 2/50
15/16 [===========================>..] - ETA: 0s - loss: 0.6964 - accuracy: 0.4681
Epoch 2: val_accuracy did not improve from 0.50000
16/16 [==============================] - 1s 42ms/step - loss: 0.6967 - accuracy: 0.4602 - val_loss: 0.6935 - val_accuracy: 0.5000
。。。。。。。。。。。。。。。。。。。。。。。。。。。。。。。。。。。。。。。。。
16/16 [==============================] - 1s 45ms/step - loss: 0.6936 - accuracy: 0.5000 - val_loss: 0.6932 - val_accuracy: 0.5000
Epoch 21/50
15/16 [===========================>..] - ETA: 0s - loss: 0.6957 - accuracy: 0.4532
Epoch 21: val_accuracy did not improve from 0.50000
16/16 [==============================] - 1s 44ms/step - loss: 0.6955 - accuracy: 0.4562 - val_loss: 0.6935 - val_accuracy: 0.5000
Epoch 21: early stopping
从输出结果看到val_accuracy一直=0.5000,那么肯定是哪里不对了。
五、模型评估
1、Loss与Accuracy图
python
acc = history.history['accuracy']
val_acc = history.history['val_accuracy']
loss = history.history['loss']
val_loss = history.history['val_loss']
epochs_range = range(len(loss))
plt.figure(figsize=(12, 4))
plt.subplot(1, 2, 1)
plt.plot(epochs_range, acc, label='Training Accuracy')
plt.plot(epochs_range, val_acc, label='Validation Accuracy')
plt.legend(loc='lower right')
plt.title('Training and Validation Accuracy')
plt.subplot(1, 2, 2)
plt.plot(epochs_range, loss, label='Training Loss')
plt.plot(epochs_range, val_loss, label='Validation Loss')
plt.legend(loc='upper right')
plt.title('Training and Validation Loss')
plt.show()
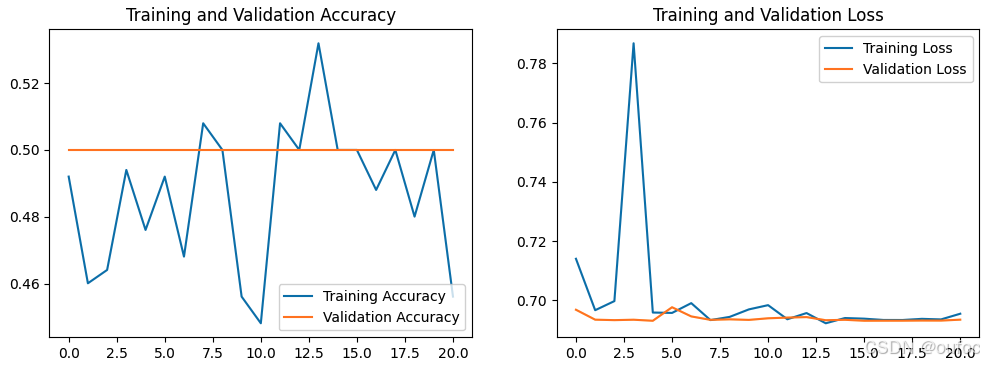
2、尝试更改initial_learning_rate=0.001
再次训练输出结果为:
python
Epoch 1/50
16/16 [==============================] - ETA: 0s - loss: 5.3369 - accuracy: 0.4940
Epoch 1: val_accuracy improved from -inf to 0.52632, saving model to best_model.h5
16/16 [==============================] - 128s 1s/step - loss: 5.3369 - accuracy: 0.4940 - val_loss: 0.6984 - val_accuracy: 0.5263
Epoch 2/50
15/16 [===========================>..] - ETA: 0s - loss: 0.6932 - accuracy: 0.5021
Epoch 2: val_accuracy improved from 0.52632 to 0.61842, saving model to best_model.h5
16/16 [==============================] - 1s 65ms/step - loss: 0.6924 - accuracy: 0.5080 - val_loss: 0.6893 - val_accuracy: 0.6184
。。。。。。。。。。。。。。。。。。。。。。。。。。。。。。。。。。。
Epoch 43/50
15/16 [===========================>..] - ETA: 0s - loss: 0.1565 - accuracy: 0.9596
Epoch 43: val_accuracy did not improve from 0.85526
16/16 [==============================] - 1s 45ms/step - loss: 0.1579 - accuracy: 0.9582 - val_loss: 0.4551 - val_accuracy: 0.8421
Epoch 43: early stopping
这里看到减小了initial_learning_rate后效果好了很多。
3、指定图片预测
python
# 加载效果最好的模型权重
model.load_weights('best_model.h5')
python
from PIL import Image
import numpy as np
img = Image.open("./data/test/nike/1.jpg") #这里选择你需要预测的图片
image = tf.image.resize(img, [img_height, img_width])
# expand_dims函数:在索引=axis处增加一个值为1的维度,这里使得image变成一个一张图片的一个批次
img_array = tf.expand_dims(image, 0) #/255.0 # 记得做归一化处理(与训练集处理方式保持一致)
predictions = model.predict(img_array) # 这里选用你已经训练好的模型
print("预测结果为:",class_names[np.argmax(predictions)])
输出:
python
1/1 [==============================] - 0s 422ms/step
预测结果为: nike
预测正确。
六、总结
- 学习到tensorflow中的动态学习率函数:
ExponentialDecay
- 模型的初始学习率不能设置过大:过大的学习率可能导致模型不收敛且模型精度太差;当然也不能太小,太小模型收敛慢,难跳出局部最优。
- 学习到使模型因没有改善早结束训练函数:
EarlyStopping