文章目录
针对连续动作空间和连续控制问题实现基于神经网络的强化学习策略
在动作是实值的连续动作空间 中,需要实值且连续的策略分布 。当环境的动作空间包含实数时,可以使用连续概率分布 表示强化学习智能体的策略。
前期准备
import tensorflow_probability as tfp
import seaborn as sns
实现步骤
使用TensorFlow 2.x和tensorflow_probability创建连续策略分布,并建立必要的动作采样方法
-
使用TensorFlow 2.x和tensorflow_probability创建连续策略分布。使用高斯/正态分布在连续值上创建策略分布
continuous_policy = tfp.distributions.Normal(loc=0.0, scale=1.0)
-
查看连续策略的分布
sample_actions = continuous_policy.sample(500) sns.displot(sample_actions)
-
使用高斯分布实现连续策略分布
mu = 0.0 # mean = 0.0 sigma = 1.0 # standard deviation = 1.0 continuous_policy = tfp.distributions.Normal(loc=mu, scale=sigma) for i in range(10): action = continuous_policy.sample(1) print(action)
tf.Tensor([-0.59630454], shape=(1,), dtype=float32)
tf.Tensor([0.4783637], shape=(1,), dtype=float32)
tf.Tensor([0.24406941], shape=(1,), dtype=float32)
tf.Tensor([1.6531773], shape=(1,), dtype=float32)
tf.Tensor([0.72150385], shape=(1,), dtype=float32)
tf.Tensor([-1.0535003], shape=(1,), dtype=float32)
tf.Tensor([-0.19095069], shape=(1,), dtype=float32)
tf.Tensor([-0.21695465], shape=(1,), dtype=float32)
tf.Tensor([0.8305619], shape=(1,), dtype=float32)
tf.Tensor([-0.3052128], shape=(1,), dtype=float32)
-
实现一个多维连续策略。多元高斯分布可以用来表示多维连续策略。当智能体在具有多维、连续实值动作空间的环境中行执行动作时,此类策略非常有用。
print(action) #%% mu = [0.0, 0.0] covariance_diag = [3.0, 3.0] continuous_multidim_policy = tfp.distributions.MultivariateNormalDiag(loc=mu, scale_diag=covariance_diag) for i in range(10): action = continuous_multidim_policy.sample(1) print(action)
tf.Tensor([[2.8001332 2.90677 ]], shape=(1, 2), dtype=float32)
tf.Tensor([[0.662844 2.252566]], shape=(1, 2), dtype=float32)
tf.Tensor([[1.1199734 3.1654496]], shape=(1, 2), dtype=float32)
tf.Tensor([[1.6220868 1.6744044]], shape=(1, 2), dtype=float32)
tf.Tensor([[ 4.977682 -2.586114]], shape=(1, 2), dtype=float32)
tf.Tensor([[2.3649094 2.9100516]], shape=(1, 2), dtype=float32)
tf.Tensor([[ 1.5777853 -2.8606076]], shape=(1, 2), dtype=float32)
tf.Tensor([[3.766756 4.517313]], shape=(1, 2), dtype=float32)
tf.Tensor([[-5.114787 0.63716304]], shape=(1, 2), dtype=float32)
-
查看多维连续策略
sample_actions = continuous_multidim_policy.sample(500) sns.jointplot(x=sample_actions[:, 0], y=sample_actions[:, 1], kind='scatter')
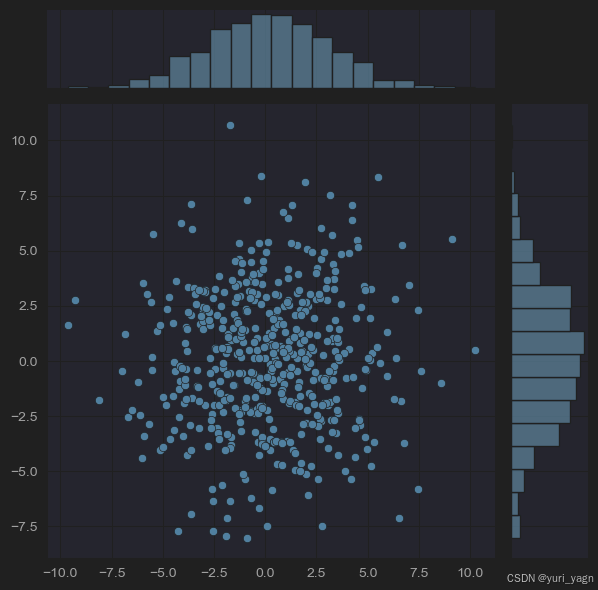
-
实现连续策略类ContinuousPolicy()
class ContinuousPolicy(object): def __init__(self, action_dim): self.action_dim = action_dim def sample(self, mu, var): self.distribution = tfp.distributions.Normal(loc=mu, scale=var) return self.distribution.sample(1) def get_action(self, mu, var): action = self.sample(mu, var) return action
-
实现一个多维连续策略类ContinuousMultiDimensionalPolicy()
import tensorflow_probability as tfp import numpy as np class ContinuousMultiDimensionalPolicy(object): def __init__(self, num_actions): self.action_dim = num_actions def sample(self, mu, covariance_diag): self.distribution = tfp.distributions.MultivariateNormalDiag(loc=mu, scale_diag=covariance_diag) return self.distribution.sample(1) def get_action(self, mu, covariance_diag): action = self.sample(mu, covariance_diag) return action
-
实现evaluate()函数
def evaluate(agent, env, render=True): obs, episode_reward, done, step_num = env.reset(), 0.0, False, 0 if isinstance(obs, tuple): obs, _ = obs # 假设观察数据是元组的第一个元素 print(obs) while not done: action = agent.get_action(obs) obs, reward, done, info = env.step(action) episode_reward += reward step_num += 1 if render: env.render() return step_num, episode_reward,done, info
-
准备在连续动作环境中测试智能体
import tensorflow as tf from tensorflow import keras from tensorflow.keras import layers class Brain(keras.Model): def __init__(self, action_dim=5, input_shape=(1, 8 * 8)): super(Brain, self).__init__() self.dense1 = layers.Dense(32, input_shape=input_shape, activation='relu') self.logits = layers.Dense(action_dim) def call(self, inputs): x = tf.convert_to_tensor(inputs) if len(x.shape) >= 2 and x.shape[0] != 1: x = tf.reshape(x, (1, -1)) return self.logits(self.dense1(x)) def process(self, observations): action_logits = self.predict_on_batch(observations) return action_logits
-
利用ContinuousPolicy对象实现一个简单的Agent()类,使其在连续动作空间环境中执行动作
class Agent(object): def __init__(self, action_dim, input_dim=(1, 8 * 8)): self.brain = Brain(action_dim, input_dim) self.policy = ContinuousPolicy(action_dim) def get_action(self, obs): action_logits = self.brain.process(obs) action = self.policy.get_action(*np.squeeze(action_logits,0)) return action
-
测试性能
import gym env = gym.make('MountainCarContinuous-v0') action_dim = 2 * env.action_space.shape[0] # 2 values (mu & sigma) for one dim agent = Agent(action_dim, env.observation_space.shape) steps, reward, done, info = evaluate(agent, env) print(f"steps: {steps}, reward: {reward}, done: {done}, info: {info}") env.close()
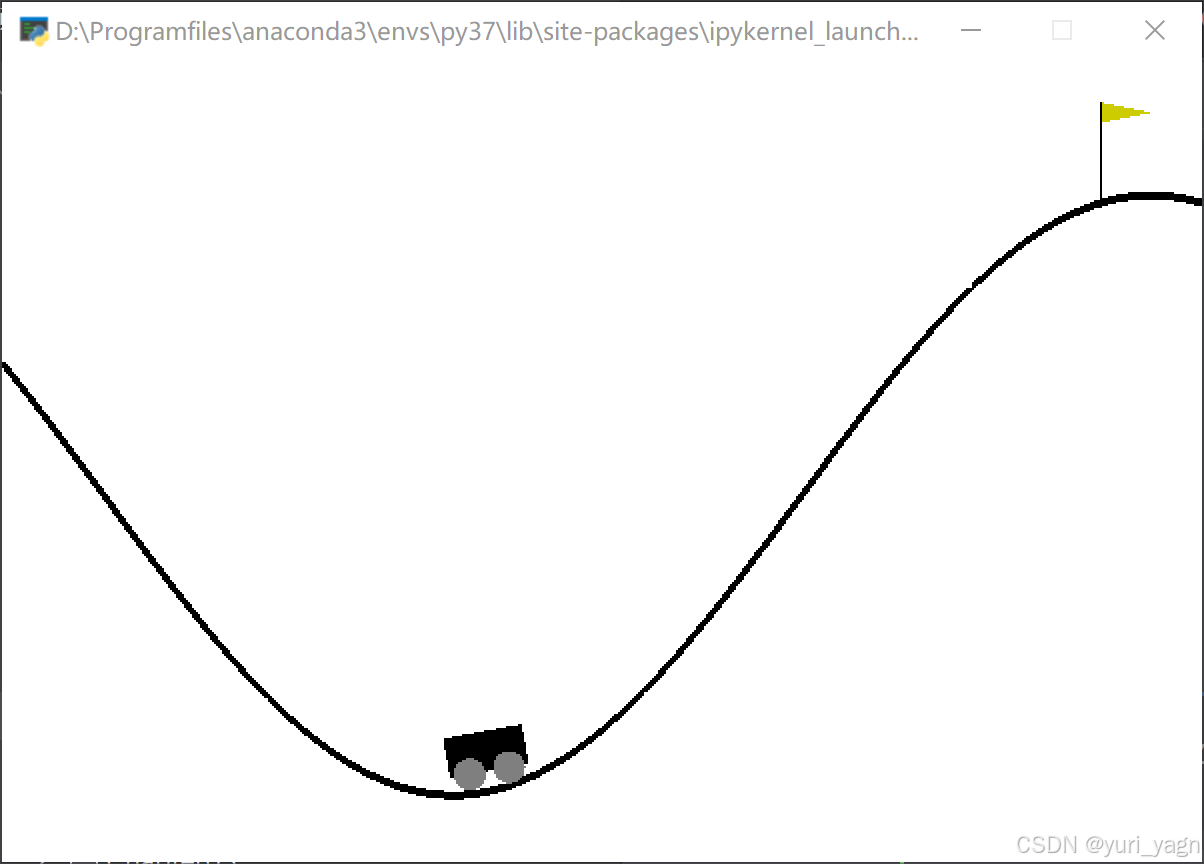
工作原理
使用高斯分布实现了强化学习智能体的连续策略。通过在其分布中采样生成连续值动作
N ( x ; μ , σ 2 ) = 1 2 π σ 2 exp ( − 1 ( 2 σ 2 ) ( x − μ ) 2 ) \mathrm{N}\left(\mathrm{x};\mu,\sigma^2\right)=\sqrt{\frac1{2\pi\sigma^2}\exp(-\frac1{(2\sigma^2)(\mathrm{x}-\mu)^2})} N(x;μ,σ2)=2πσ21exp(−(2σ2)(x−μ)21)
多元正态分布将正态分布推广到多变量,使其可以生成多为连续策略
将OpenAI Gym作为强化学习的训练环境
gym环境和接口提供了训练强化学习智能体的平台。它是目前应用最广发,接受度最高的强化学习环境接口
前期准备
安装Gym
pip install gym[atari]
实现步骤
-
查看Gym的环境列表
from gym import envs env_names = [spec.id for spec in envs.registry.values()] for name in sorted(env_names): print(name)
-
环境名称
-
使用一个gym 环境
import gym import sys def run_gym_env(argv): env = gym.make(argv[1]) # name of the environment env.reset() for _ in range(int(argv[2])): # number of steps env.render() env.step(env.action_space.sample()) env.close() if __name__ == "__main__": run_gym_env(sys.argv)
-
将脚本保存为run_gym_env.py,按下面方式运行脚本
shellpython run_gym_env.py Alien-v4 1000
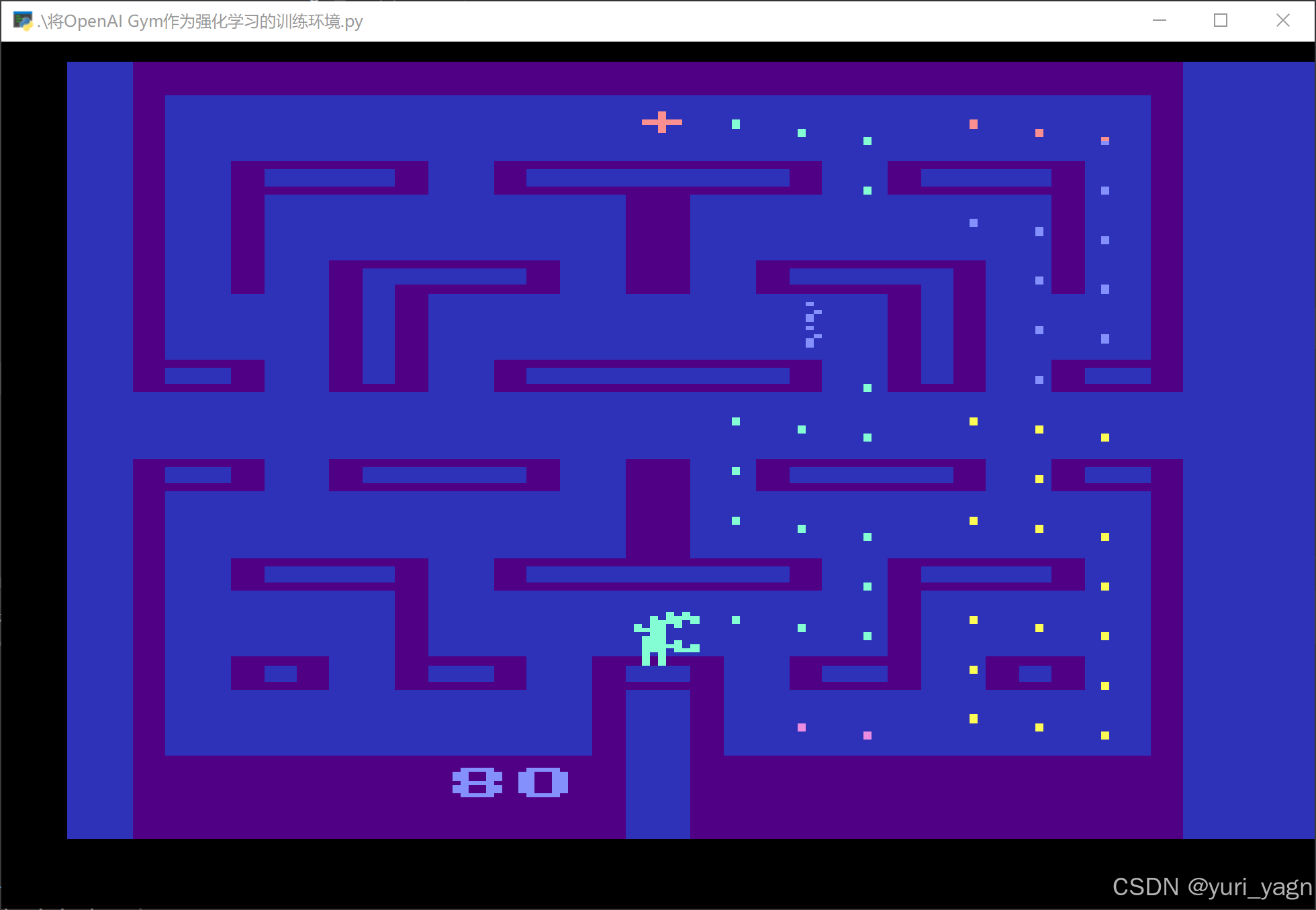
工作原理
返回值 | 类型 | 描述 |
---|---|---|
next_observation | 对象 | 环境返回的观察结果,对象可以是屏幕或相机的RGB像素数据 ,RAM内容或机器人的关节接角度和摇摆速度等,具体取决于环境 |
Reward | 浮点 | 对前一行动作的奖励,为浮点数表示,其范围随环境的不同而变化。由于环境的影响,较高的奖励总是更好的,智能体的目标是最大化总体奖励 |
Done | 布尔 | 指示环境是否在下一步重置。当布尔值为true时,意味着该回合已结束(原因包括智能体生命损失,超时或其他总之条件) |
Info | 字典 | 附加信息可由环境以任意键值组成的字典形式给出。 |
构建神经网络智能体
构建完整的智能体和环境的交互循环,这是强化学习应用的主要组成部分
前期准备
from collections import namedtuple
import gym
import matplotlib.pyplot as plt
import numpy as np
import tensorflow as tf
from tensorflow import keras
from tensorflow.keras import layers
from tqdm import tqdm
实现步骤
-
使用TensorFlow 2.x 和 Keras函数API初始化一个神经网络模型
class Brain(keras.Model): def __init__(self, action_dim=5, input_shape=(1, 8 * 8)): """Initialize the Agent's Brain model Args: action_dim (int): Number of actions """ super(Brain, self).__init__() self.dense1 = layers.Dense(32, input_shape=input_shape, activation="relu") self.logits = layers.Dense(action_dim)
-
实现Brain()类的call()函数
def call(self, inputs): x = tf.convert_to_tensor(inputs) # if len(x.shape) >= 2 and x.shape[0] != 1: # x = tf.reshape(x, (1, -1)) logits = self.logits(self.dense1(x)) return logits
-
实现Brain()类的process()函数,以方便地对一批输入/观测进行预测
def process(self, observations): # Process batch observations using `call(inputs)` behind-the-scenes action_logits = self.predict_on_batch(observations) return action_logits
-
实现Agent()类的 init ()函数
class Agent(object): def __init__(self, action_dim=5, input_shape=(1, 8 * 8)): """Agent with a neural-network brain powered policy Args: brain (keras.Model): Neural Network based model """ self.brain = Brain(action_dim, input_shape) self.brain.compile( loss="categorical_crossentropy", optimizer="adam", metrics=["accuracy"] ) self.policy = self.policy_mlp
-
为智能体定义一个简单的policy_mlp()函数
def policy_mlp(self, observations): observations = observations.reshape(1, -1) action_logits = self.brain.process(observations) action = tf.random.categorical(tf.math.log(action_logits), num_samples=1) return action # tf.squeeze(action, axis=0)
-
为智能体实现一个简便的get_action()函数
def get_action(self, observations): return self.policy(observations)
-
为learn()创建一个占位函数
def learn(self, obs, actions, **kwargs): self.brain.fit(obs, actions, **kwargs)
-
在给定的环境中评估智能体在一个回合中的表现
def evaluate(agent, env, render=True): obs, episode_reward, done, step_num, info = env.reset(), 0.0, False, 0, None while not done: action = agent.get_action(obs) obs, reward, done, info = env.step(action) episode_reward += reward step_num += 1 if render: env.render() return step_num, episode_reward, done, info
-
实现main()函数
if __name__ == "__main__": # train(num_epochs=2) # Increase value of num_epochs env = gym.make("Gridworld-v0") agent = Agent(env.action_space.n, env.observation_space.shape) for episode in tqdm(range(10)): steps, episode_reward, done, info = evaluate(agent, env) print(f"steps: {steps}, reward: {episode_reward}, done: {done}, info: {info}") env.close()
-
执行脚本
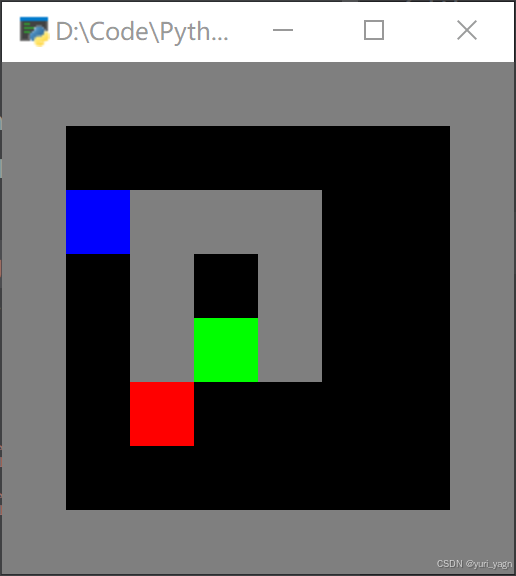
工作原理
Brain类实现了作为智能体处理单元的神经网络
Agent类利用Brain类和一个简单的策略,其中神经网络对从环境获得的观测进行处理,而策略将根据神经网络的输出选择一个动作
Brain类作为keras.Model类的一个子类进行实现,这允许为智能体的大脑定义一个自定义的神经网络模型。
在__init()__函数中,使用keras定义必要的神经网络层,创建了两个全连接层。
call函数是作为keras.Model的子类必须实现的方法,先将输入装换为TensorFlow2.x张量,再展平为1×total_number_of_elements形状的张量。比如输入是8*8,展平后就是1 * 64.然后再经过第一个全连接层(32个神经元和一个Relu激活函数),最后是logits 层处理,得到与动作维度n对应的n个输出
predict_on_batch()函数对一批作为参数的输入进行预测。与Keras的predict()函数不同,不需要对输入数据做进一步拆分。
接下来实现Agent类
self.brain = Brain(action_dim, input_shape)
input_shape是Brain类的对象实例预期处理的输入的形状,action_dim是其预期输出的形状。
智能体的策略定义为基于Brain类的对象实例神经网络架构的自定义 **多层感知机(MLP)**策略
智能体的策略函数 policy_mlp()将输入的观测展平,并发送给智能体的大脑进行处理,以获得action_logits,即动作的非归一化概率。使用TensorFlow 2.x的random模块的categorical()函数获得最终采取的动作。