Title
题目
AI-based histopathology image analysisreveals a distinct subset of endometrialcancers
AI 基于病理图像分析揭示了一种不同类型的子宫内膜癌。
01
文献速递介绍
子宫内膜癌(EC)有四种分子亚型,具有很强的预后价值和治疗意义。最常见的亚型是NSMP(无特定分子特征),是在排除其他三种分子亚型的特征后分配的,包括具有异质性临床结果的患者。在本研究中,我们利用人工智能(AI)驱动的病理图像分析来区分p53abn和NSMP EC亚型,从而识别出一组NSMP EC患者,该组患者的无进展生存期和疾病特异性生存期显著较差(称为"p53abn-like NSMP")。这一发现基于368名患者的发现队列以及来自其他中心的290名和614名患者的两个独立验证队列。浅层全基因组测序显示,"p53abn-like NSMP"组比NSMP组具有更高的拷贝数异常负担,表明该组在生物学上与其他NSMP ECs不同。我们的研究展示了AI在检测预后不同且用常规和标准分子或病理学标准无法识别的EC亚群方面的强大能力,改进了基于图像的肿瘤分类。本研究结果仅适用于女性。
Method
方法
The Declaration of Helsinki and the International Ethical Guidelines forBiomedical Research Involving Human Subjects were strictly adheredto throughout this study. All protocols for this study, including thewaiver of consent, have been approved by the University of BritishColumbia/BC Cancer Research Ethics Board. Participants did notreceive compensation.
在整个研究过程中,我们严格遵守《赫尔辛基宣言》和《涉及人类受试者的生物医学研究国际伦理指南》。本研究的所有协议,包括同意书豁免,均已获得不列颠哥伦比亚大学/BC癌症研究伦理委员会的批准。参与者未获得报酬。
Results
结果
Patient cohort selection and description2318 H&E-stained hysterectomy tissue sections from 1272 patients withhistologically confirmed endometrial carcinoma of NSMP or p53abnsubtypes were included in this study3--5 . Our discovery cohort included155 whole-section slides (WSI) from 146 patients from TCGA3 and 431WSIs (222 patients) from Tubingen University5 . Two separate validation Canadian cohorts were included in this study: (1) the BritishColumbia (BC) cohort, a tissue microarray (TMA) dataset corresponding to 290 patients from our own center4 , and (2) Cross Canada(CC) cohort, 640 WSIs (614 patients) collected from 26 hospitalsacross Canada31. Tables 1--3 show the clinicopathological features ofthe discovery and validation cohorts. Overview of the cohorts, outcome information, treatment information, and distribution of samplesin the CC cohort across different centers can be found in Supplementary Tables 1--4.
患者队列选择和描述
本研究包括来自1272名患者的2318张H&E染色的子宫切除术组织切片,这些患者的子宫内膜癌经组织学确认为NSMP或p53abn亚型。我们的发现队列包括来自TCGA的146名患者的155张全切片(WSI)和来自图宾根大学的222名患者的431张WSI。此外,本研究还包括两个独立的加拿大验证队列:(1)不列颠哥伦比亚(BC)队列,一个包含我们中心290名患者的组织微阵列(TMA)数据集;(2)跨加拿大(CC)队列,来自加拿大26家医院收集的640张WSI(614名患者)。表1-3展示了发现和验证队列的临床病理特征。有关各队列的概述、结果信息、治疗信息以及CC队列中不同中心样本分布的信息,请参见补充表1-4。
Figure
图
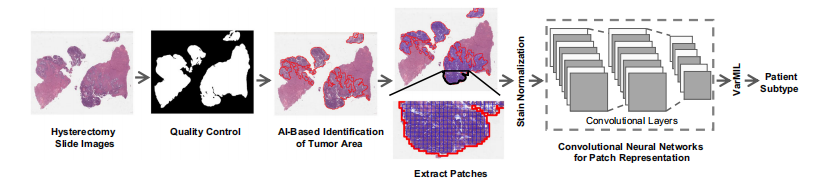
Fig. 1 | Workflow of the AI-based histopathology image analysis. First, the
quality control framework, HistoQC81, generates a mask that comprises tissueregions exclusively and removes artifacts. Then, an AI model to identify tumorregions within histopathology slides is trained. Next, images are tessellated intosmall patches and normalized to remove color variations. The normalized patchesare fed to a deep-learning model to derive patch-level representations. Finally, amodel based on multiple instance learning (VarMIL) was utilized to predict thepatient subtype.
图1 | 基于AI的病理图像分析工作流程。首先,质量控制框架HistoQC81生成仅包含组织区域的掩膜并去除伪影。然后,训练AI模型以识别病理切片中的肿瘤区域。接下来,将图像分割成小块,并进行颜色标准化以消除颜色差异。标准化后的小块被输入到深度学习模型中,以获得小块级别的表示。最后,基于多实例学习(VarMIL)的模型用于预测患者亚型。
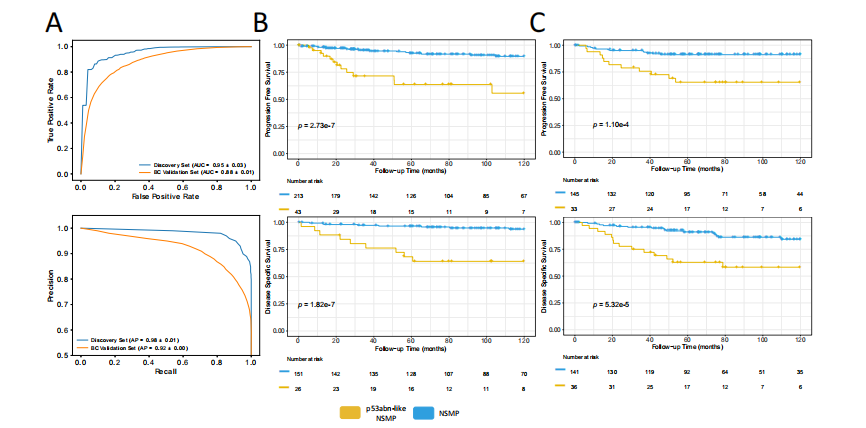
Fig. 2 | Performance statistics and Kaplan Meier (KM) survival curves for AIidentified EC subtypes. A AUROC and precision-recall plots of average of 10 splitsfor p53abn vs. NSMP classifier for discovery and validation sets, (B) KM curvesassociated with PFS and DSS (where available) for the discovery set, using a twosided log-rank statistical test, (C) KM curves associated with PFS and DSS (whereavailable) in the BC validation set, using a two-sided log-rank statistical test (Sourcedata are provided as a Source Data file).
图2 | AI识别的子宫内膜癌(EC)亚型的性能统计和Kaplan-Meier(KM)生存曲线。A. p53abn与NSMP分类器在发现组和验证组的10次分割平均的AUROC和精确率-召回图,B. 使用双侧log-rank统计检验分析的发现组的无进展生存期(PFS)和疾病特异性生存期(DSS,若有)相关的KM曲线,C. 使用双侧log-rank统计检验分析的BC验证组的PFS和DSS(若有)相关的KM曲线(源数据作为源数据文件提供)。
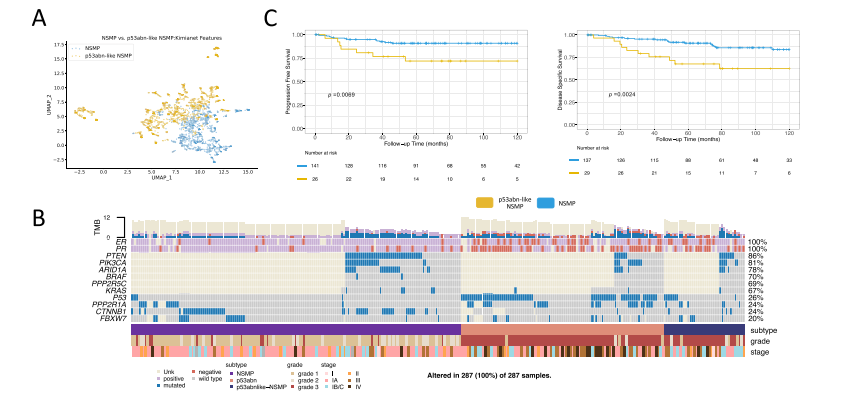
Fig. 3 | KimiaNet features, clinicopathological features, point mutations, andKM curves of the validation cohort. A Histopathological features from the slidesin the validation cohort utilizing KimiaNet feature representation from the slides inthe validation cohort demonstrate that p53abn-like NSMP and the rest of the NSMPcases constitute two separate clusters, (B) Clinicopathological features and pointmutation data for the BC validation cohort, (C) KM curves associated with PFS andDSS for the BC validation cohort after removing TP53 mutant cases, using a twosided log-rank statistical test (Source data are provided as a Source Data file)
图3 | KimiaNet特征、临床病理特征、点突变及验证队列的KM曲线。A. 使用KimiaNet特征表示的验证队列切片的病理特征展示出p53abn-like NSMP和其余NSMP病例构成了两个独立的集群,B. BC验证队列的临床病理特征和点突变数据,C. 在移除TP53突变病例后,使用双侧log-rank统计检验分析的BC验证队列的无进展生存期(PFS)和疾病特异性生存期(DSS)相关的KM曲线(源数据作为源数据文件提供)。
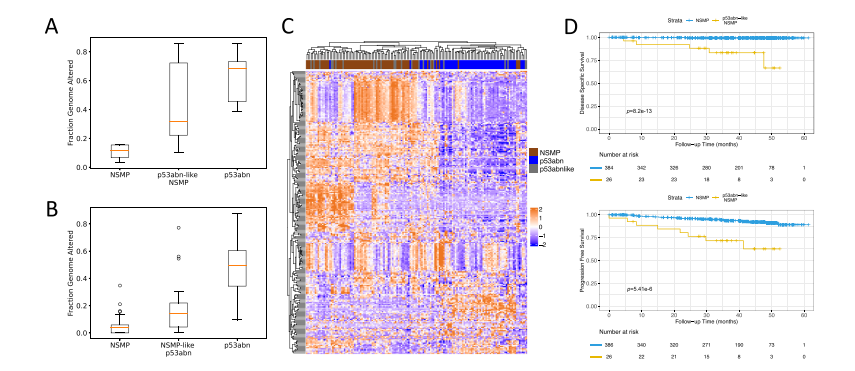
Fig. 4 | Molecular profiling of p53abn-like NSMP cases. Boxplots of copy numberburden (i.e., fraction genome altered) in NSMP, p53abn-like NSMP, and p53abncases in the (A) BC validation cohort (6 NSMP, 7 p53abn-like NSMP, 5 p53abn) and(B) TCGA (69 NSMP, 21 p53abn-like NSMP, 56 p53abn) cohorts. In box plots in A andB, the central line represents the median, while the bottom and top edges of thebox correspond to the 25th and 75th percentiles, respectively. The whiskers extendto the most extreme data points that are not identified as outliers. Any data pointsbeyond the lower and upper whiskers are considered outliers. C Gene expressionprofiles associated with the p53abn-like NSMP (n = 21), NSMP (n = 69), and p53abn(n = 56) tumors in the TCGA cohort. D KM curves associated with PFS and DSS(where available) in the CC validation set (Source data are provided as a SourceData file).
图4 | p53abn-like NSMP病例的分子特征。NSMP、p53abn-like NSMP和p53abn病例在(A)BC验证队列(6个NSMP,7个p53abn-like NSMP,5个p53abn)和(B)TCGA(69个NSMP,21个p53abn-like NSMP,56个p53abn)队列中的拷贝数负担(即基因组变异部分)箱线图。在A和B中的箱线图中,中线表示中位数,而箱体的底部和顶部边缘分别对应于第25百分位数和第75百分位数。胡须延伸到未被识别为异常值的最极端数据点。任何超出下胡须和上胡须的数据点都被认为是异常值。C. TCGA队列中与p53abn-like NSMP(n = 21)、NSMP(n = 69)和p53abn(n = 56)肿瘤相关的基因表达谱。D. 在CC验证组中与无进展生存期(PFS)和疾病特异性生存期(DSS,若有)相关的KM曲线(源数据作为源数据文件提供)。
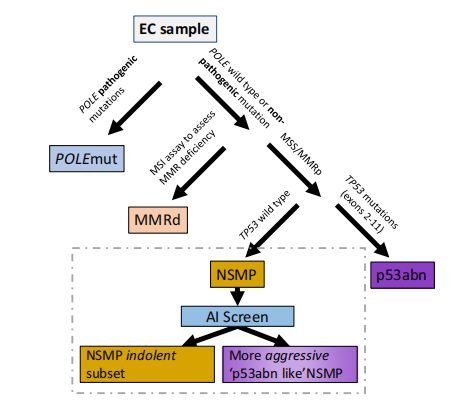
Fig. 5 | AI-enhanced NSMP stratification. AI-enhanced NSMP stratification. Therefined classification scheme that leverages AI screening as a supplementary stratification mechanism within the NSMP molecular subtype
图5 | AI增强的NSMP分层。AI增强的NSMP分层。利用AI筛查作为NSMP分子亚型中的补充分层机制的精细分类方案。
Table
表
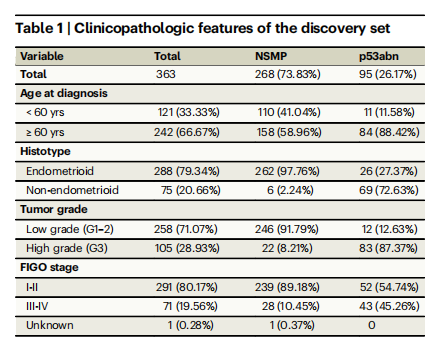
Table 1 | Clinicopathologic features of the discovery set
表1 | 发现组的临床病理特征
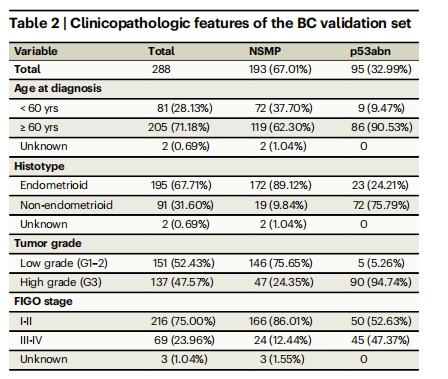
Table 2 | Clinicopathologic features of the BC validation set
表2 | 不列颠哥伦比亚(BC)验证组的临床病理特征
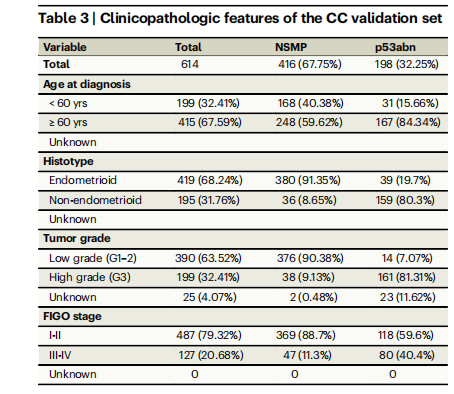
Table 3 | Clinicopathologic features of the CC validation set
表3 | 跨加拿大(CC)验证组的临床病理特征