目录
[第9章 广告数仓DIM层](#第9章 广告数仓DIM层)
[9.1 广告信息维度表](#9.1 广告信息维度表)
[9.2 平台信息维度表](#9.2 平台信息维度表)
[9.3 数据装载脚本](#9.3 数据装载脚本)
[第10章 广告数仓DWD层](#第10章 广告数仓DWD层)
[10.1 广告事件事实表](#10.1 广告事件事实表)
[10.1.1 建表语句](#10.1.1 建表语句)
[10.1.2 数据装载](#10.1.2 数据装载)
[10.1.2.1 初步解析日志](#10.1.2.1 初步解析日志)
[10.1.2.2 解析IP和UA](#10.1.2.2 解析IP和UA)
[10.1.2.3 标注无效流量](#10.1.2.3 标注无效流量)
[10.2 数据装载脚本](#10.2 数据装载脚本)
第9章 广告数仓DIM层
DIM层设计要点:
(1)DIM层的设计依据是维度建模理论,该层存储维度模型的维度表。
(2)DIM层的数据存储格式为orc列式存储+snappy压缩。
(3)DIM层表名的命名规范为dim_表名_全量表或者拉链表标识(full/zip)。
9.1 广告信息维度表
1 )建表语句
sql
复制代码
drop table if exists dim_ads_info_full;
create external table if not exists dim_ads_info_full
(
ad_id string comment '广告id',
ad_name string comment '广告名称',
product_id string comment '广告产品id',
product_name string comment '广告产品名称',
product_price decimal(16, 2) comment '广告产品价格',
material_id string comment '素材id',
material_url string comment '物料地址',
group_id string comment '广告组id'
) PARTITIONED BY (`dt` STRING)
STORED AS ORC
LOCATION '/warehouse/ad/dim/dim_ads_info_full'
TBLPROPERTIES ('orc.compress' = 'snappy');
2 )加载数据
sql
复制代码
insert overwrite table dim_ads_info_full partition (dt='2023-01-07')
select
ad.id,
ad_name,
product_id,
name,
price,
material_id,
material_url,
group_id
from
(
select
id,
ad_name,
product_id,
material_id,
group_id,
material_url
from ods_ads_info_full
where dt = '2023-01-07'
) ad
left join
(
select
id,
name,
price
from ods_product_info_full
where dt = '2023-01-07'
) pro
on ad.product_id = pro.id;
9.2 平台信息维度表
1 )建表语句
sql
复制代码
drop table if exists dim_platform_info_full;
create external table if not exists dim_platform_info_full
(
id STRING comment '平台id',
platform_name_en STRING comment '平台名称(英文)',
platform_name_zh STRING comment '平台名称(中文)'
) PARTITIONED BY (`dt` STRING)
STORED AS ORC
LOCATION '/warehouse/ad/dim/dim_platform_info_full'
TBLPROPERTIES ('orc.compress' = 'snappy');
2 )加载数据
sql
复制代码
insert overwrite table dim_platform_info_full partition (dt = '2023-01-07')
select
id,
platform_name_en,
platform_name_zh
from ods_platform_info_full
where dt = '2023-01-07';
9.3 数据装载脚本
1 ) 在 hadoop102 的 /home/atguigu/bin 目录下创建 ad_ods_to_dim.sh
atguigu@hadoop102 bin\]$ vim ad_ods_to_dim.sh
**2** **)** **编写如下内容**
```bash
#!/bin/bash
APP=ad
# 如果是输入的日期按照取输入日期;如果没输入日期取当前时间的前一天
if [ -n "$2" ] ;then
do_date=$2
else
do_date=`date -d "-1 day" +%F`
fi
dim_platform_info_full="
insert overwrite table ${APP}.dim_platform_info_full partition (dt='$do_date')
select
id,
platform_name_en,
platform_name_zh
from ${APP}.ods_platform_info_full
where dt = '$do_date';
"
dim_ads_info_full="
insert overwrite table ${APP}.dim_ads_info_full partition (dt='$do_date')
select
ad.id,
ad_name,
product_id,
name,
price,
material_id,
material_url,
group_id
from
(
select
id,
ad_name,
product_id,
material_id,
group_id,
material_url
from ${APP}.ods_ads_info_full
where dt = '$do_date'
) ad
left join
(
select
id,
name,
price
from ${APP}.ods_product_info_full
where dt = '$do_date'
) pro
on ad.product_id = pro.id;
"
case $1 in
"dim_ads_info_full")
hive -e "$dim_ads_info_full"
;;
"dim_platform_info_full")
hive -e "$dim_platform_info_full"
;;
"all")
hive -e "$dim_ads_info_full$dim_platform_info_full"
;;
esac
```
**3** **)增加脚本执行权限**
\[atguigu@hadoop102 bin\]$ chmod +x ad_ods_to_dim.sh
**4** **)** **脚本用法**
\[atguigu@hadoop102 bin\]$ ad_ods_to_dim.sh all 2023-01-07
## 第10章 广告数仓DWD层
DWD层设计要点:
(1)DWD层的设计依据是维度建模理论,该层存储维度模型的事实表。
(2)DWD层的数据存储格式为orc列式存储+snappy压缩。
(3)DWD层表名的命名规范为dwd_数据域_表名_单分区增量全量标识(inc/full)
### 10.1 广告事件事实表
#### 10.1.1 建表语句
```sql
drop table if exists dwd_ad_event_inc;
create external table if not exists dwd_ad_event_inc
(
event_time bigint comment '事件时间',
event_type string comment '事件类型',
ad_id string comment '广告id',
ad_name string comment '广告名称',
ad_product_id string comment '广告商品id',
ad_product_name string comment '广告商品名称',
ad_product_price decimal(16, 2) comment '广告商品价格',
ad_material_id string comment '广告素材id',
ad_material_url string comment '广告素材地址',
ad_group_id string comment '广告组id',
platform_id string comment '推广平台id',
platform_name_en string comment '推广平台名称(英文)',
platform_name_zh string comment '推广平台名称(中文)',
client_country string comment '客户端所处国家',
client_area string comment '客户端所处地区',
client_province string comment '客户端所处省份',
client_city string comment '客户端所处城市',
client_ip string comment '客户端ip地址',
client_device_id string comment '客户端设备id',
client_os_type string comment '客户端操作系统类型',
client_os_version string comment '客户端操作系统版本',
client_browser_type string comment '客户端浏览器类型',
client_browser_version string comment '客户端浏览器版本',
client_user_agent string comment '客户端UA',
is_invalid_traffic boolean comment '是否是异常流量'
) PARTITIONED BY (`dt` STRING)
STORED AS ORC
LOCATION '/warehouse/ad/dwd/dwd_ad_event_inc/'
TBLPROPERTIES ('orc.compress' = 'snappy');
```
#### 10.1.2 数据装载
该表的数据装载逻辑相对复杂,所以我们分步完成,其包含的步骤如下所示:
**1** **)初步解析日志**
解析出日志中的事件类型、广告平台、广告id、客户端ip及ua等信息。
**2** **)解析** **ip** **和** **ua**
进一步对**ip**和**ua**信息进行解析,得到ip对应的地理位置信息以及ua对应得浏览器和操作系统等信息。
**3** **)标注异常流量**
异常流量分为**GIVT** (General Invalid Traffic 的缩写,即常规无效流量)和**SIVT**(Sophisticated Invalid Traffic,即复杂无效流量)。这两类流量分别具有如下特点:
常规无效流量可根据已知"蜘蛛"程序和漫游器列表或通过其他例行检查识别出来。
复杂无效流量往往难以识别,这种类型的流量无法通过简单的规则识别,需要通过更深入的分析才能识别出来。
常规无效流量一般包括:来自数据中心的流量(通过IP识别),来自已知抓取工具的流量(通过UA识别)等等。
复杂无效流量一般包括:高度模拟真人访客的机器人和爬虫流量,虚拟化设备中产生的流量,被劫持的设备产生的流量等等。
以下是本课程包含的异常流量识别逻辑:
(1)根据已知的爬虫UA列表进行判断
(2)根据异常访问行为进行判断,具体异常行为如下:
* 同一ip访问过快
* 同一设备id访问过快
* 同一ip固定周期访问
* 同一设备id固定周期访问
##### **10.1.2.1 初步解析日志**
该步骤,只需将日志中的所有信息解析为单独字段,并将结果保存至临时表即可。
使用parse_url()方法进行处理,通过desc function extended parse_url;查看方法用法
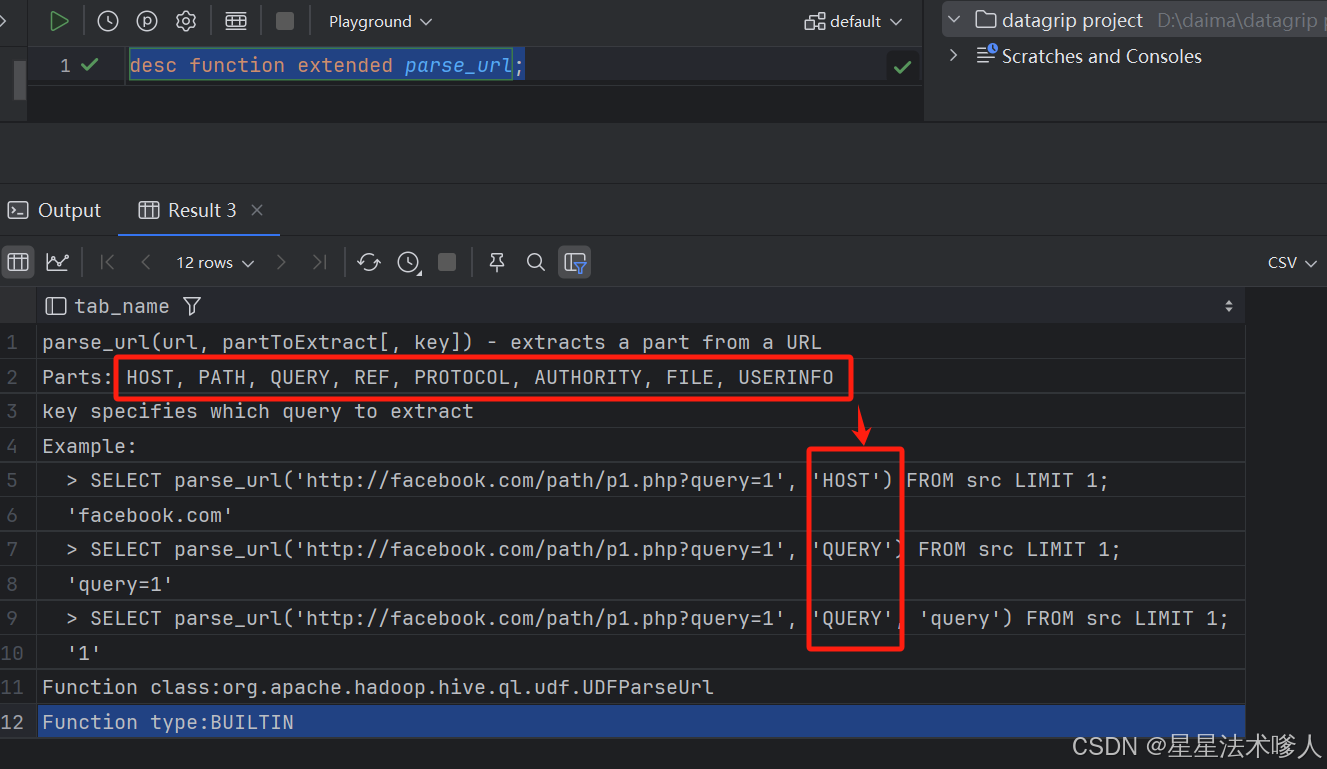
使用reflect()方法进行反射,对ua进行解码
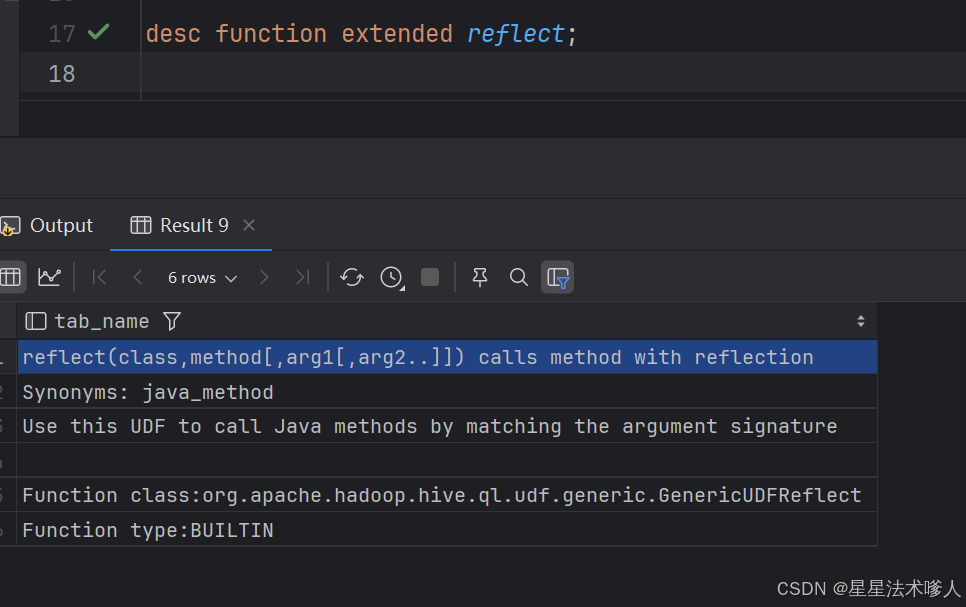
使用示例
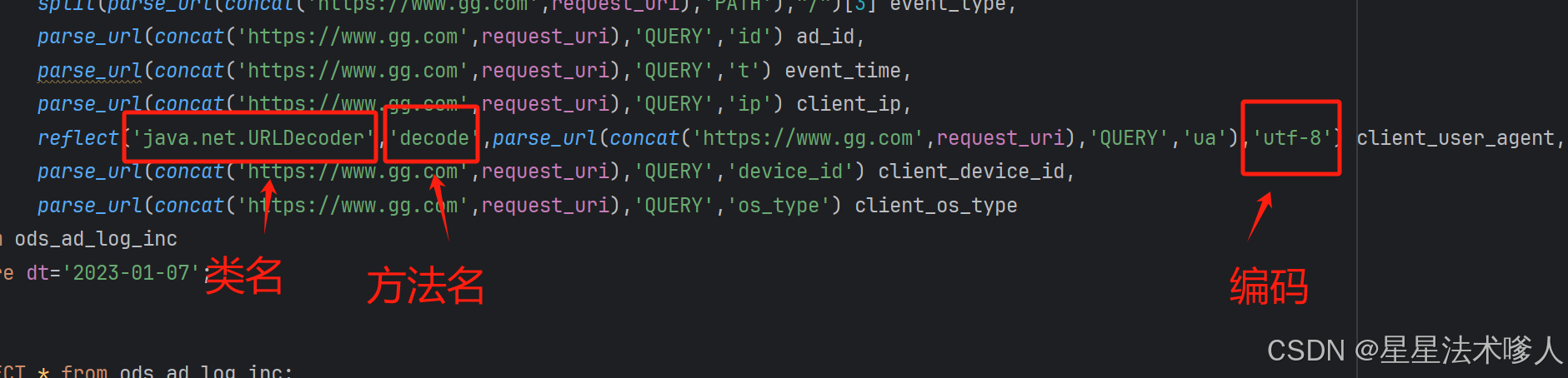
```sql
create temporary table coarse_parsed_log
as
select split(parse_url(concat('https://www.gg.com',request_uri),'PATH'),"/")[2] platform_name_en,
split(parse_url(concat('https://www.gg.com',request_uri),'PATH'),"/")[3] event_type,
parse_url(concat('https://www.gg.com',request_uri),'QUERY','id') ad_id,
parse_url(concat('https://www.gg.com',request_uri),'QUERY','t') event_time,
parse_url(concat('https://www.gg.com',request_uri),'QUERY','ip') client_ip,
reflect('java.net.URLDecoder','decode',parse_url(concat('https://www.gg.com',request_uri),'QUERY','ua'),'utf-8') client_user_agent,
parse_url(concat('https://www.gg.com',request_uri),'QUERY','device_id') client_device_id,
parse_url(concat('https://www.gg.com',request_uri),'QUERY','os_type') client_os_type
from ods_ad_log_inc
where dt='2023-01-07';
```
注:
(1)临时表(temporary table)只在当前会话有效,使用时需注意
(2)parse_url函数和reflect函数的用法可使用以下命令查看
```sql
hive>
desc function extended parse_url;
desc function extended reflect;
```
##### **10.1.2.2 解析IP和UA**
该步骤,需要根据IP得到地理位置信息(例如省份、城市等),并根据UA得到客户端端操作系统及浏览器等信息。需要注意的是,Hive并未提供用于解析IP地址和User Agent的函数,故我们需要先自定义函数。
**1** **)自定义** **IP** **解析函数**
该函数的主要功能是根据IP地址得到其所属的地区、省份、城市等信息。
上述功能一般可通过以下方案实现:
方案一:请求某些**第三方**提供的的IP定位接口(例如:高德开放平台),该方案的优点是IP定位准确、数据可靠性高;缺点是,一般有请求次数和QPS(Queries Per Second)限制,若超过限制需付费使用。
方案二:使用**免费的离线IP**数据库进行查询,该方案的优点是**无任何限制**,缺点是数据的准确率、可靠性略差。
在当前的场景下,我们更适合选择方案二。主要原因是,方案一的效率较低,因为这些IP定位接口,一般是每次请求定位一个IP,若采用该方案,则处理每条数据都要请求一次接口,在加上这些API的QPS限制,就会导致我们的SQL运行效率极低。
**(** **1** **)免费** **IP** **地址库介绍**
我们采用的免费IP地址库为ip2region v2.0,其地址如下:
[https://github.com/lionsoul2014/ip2region.git](https://github.com/lionsoul2014/ip2region.git "https://github.com/lionsoul2014/ip2region.git")
ip2region是一个离线IP地址定位库和IP定位数据管理框架,有着10微秒级别的查询效率,并提供了众多主流编程语言的客户端实现。
**(** **2** **)使用说明**
其官方案例如下:
```java
public class TestIP2Region {
public static void main(String[] args) throws Exception {
//1.ip2region.xdb是其ip地址库文件,下载地址如为: https://github.com/lionsoul2014/ip2region/raw/master/data/ip2region.xdb
byte[] bytes;
Searcher searcher =null;
try {
// 读取本地磁盘的ip解析库进入到内存当中
bytes = Searcher.loadContentFromFile("src\\main\\resources\\ip2region.xdb");
// 创建解析对象
searcher = Searcher.newWithBuffer(bytes);
// 解析ip
String search = searcher.search("223.223.182.174");
// 打印结果
System.out.println(search);
searcher.close();
} catch (Exception e) {
e.printStackTrace();
}finally {
if (searcher!=null){
try {
searcher.close();
} catch (IOException e) {
e.printStackTrace();
}
}
}
}
}
```
**(** **3** **)自定义函数实现**
①函数功能定义
* **函数名:** **parse_ip**
* **参数:**
|--------------|--------|------------------------------------------------------------------|
| **参数名** | **类型** | **说明** |
| **filepath** | string | ip2region.xdb文件路径。 注:该路径要求为HDFS路径,也就是我们需将ip2region.xdb文件上传至hdfs。 |
| **ipv4** | string | 需要解析的ipv4地址 |
* **输出:**
**输出类型为结构体,具体定义如下:**
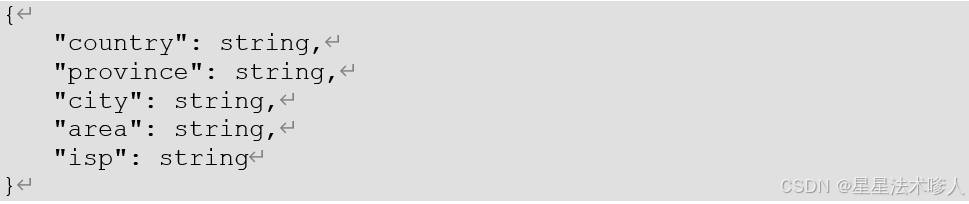
②创建一个maven项目,pom文件内容如下
```XML
4.0.0
com.gg
ad_hive_udf
1.0-SNAPSHOT
8
8
org.apache.hive
hive-exec
3.1.3
provided
org.lionsoul
ip2region
2.7.0
org.apache.maven.plugins
maven-assembly-plugin
3.0.0
jar-with-dependencies
make-assembly
package
single
```
③创建com.gg.ad.hive.udf.ParseIP类,并编辑如下内容:
```java
package com.gg.ad.hive.udf;
import org.apache.hadoop.conf.Configuration;
import org.apache.hadoop.fs.FSDataInputStream;
import org.apache.hadoop.fs.FileSystem;
import org.apache.hadoop.fs.Path;
import org.apache.hadoop.hive.ql.exec.UDFArgumentException;
import org.apache.hadoop.hive.ql.metadata.HiveException;
import org.apache.hadoop.hive.ql.udf.generic.GenericUDF;
import org.apache.hadoop.hive.serde2.objectinspector.ConstantObjectInspector;
import org.apache.hadoop.hive.serde2.objectinspector.ObjectInspector;
import org.apache.hadoop.hive.serde2.objectinspector.ObjectInspectorFactory;
import org.apache.hadoop.hive.serde2.objectinspector.PrimitiveObjectInspector;
import org.apache.hadoop.hive.serde2.objectinspector.primitive.PrimitiveObjectInspectorFactory;
import org.apache.hadoop.io.IOUtils;
import org.lionsoul.ip2region.xdb.Searcher;
import java.io.ByteArrayInputStream;
import java.io.ByteArrayOutputStream;
import java.io.OutputStream;
import java.util.ArrayList;
public class ParseIP extends GenericUDF{
Searcher searcher = null;
/**
* 判断函数传入的参数个数以及类型 同时确定返回值类型
* @param arguments
* @return
* @throws UDFArgumentException
*/
@Override
public ObjectInspector initialize(ObjectInspector[] arguments) throws UDFArgumentException {
// 传入参数的个数
if (arguments.length != 2){
throw new UDFArgumentException("parseIP必须填写2个参数");
}
// 校验参数的类型
ObjectInspector hdfsPathOI = arguments[0];
if (hdfsPathOI.getCategory() != ObjectInspector.Category.PRIMITIVE) {
throw new UDFArgumentException("parseIP第一个参数必须是基本数据类型");
}
PrimitiveObjectInspector hdfsPathOI1 = (PrimitiveObjectInspector) hdfsPathOI;
if (hdfsPathOI1.getPrimitiveCategory() != PrimitiveObjectInspector.PrimitiveCategory.STRING) {
throw new UDFArgumentException("parseIP第一个参数必须是string类型");
}
// 校验参数的类型
ObjectInspector ipOI = arguments[1];
if (ipOI.getCategory() != ObjectInspector.Category.PRIMITIVE) {
throw new UDFArgumentException("parseIP第二个参数必须是基本数据类型");
}
PrimitiveObjectInspector ipOI1 = (PrimitiveObjectInspector) ipOI;
if (ipOI1.getPrimitiveCategory() != PrimitiveObjectInspector.PrimitiveCategory.STRING) {
throw new UDFArgumentException("parseIP第二个参数必须是string类型");
}
// 读取ip静态库进入内存中
// 获取hdfsPath地址
if (hdfsPathOI instanceof ConstantObjectInspector){
String hdfsPath = ((ConstantObjectInspector) hdfsPathOI).getWritableConstantValue().toString();
// 从hdfs读取静态库
Path path = new Path(hdfsPath);
try {
FileSystem fileSystem = FileSystem.get( new Configuration());
FSDataInputStream inputStream = fileSystem.open(path);
ByteArrayOutputStream byteArrayOutputStream = new ByteArrayOutputStream();
IOUtils.copyBytes(inputStream,byteArrayOutputStream,1024);
byte[] bytes = byteArrayOutputStream.toByteArray();
//创建静态库,解析IP对象
searcher = Searcher.newWithBuffer(bytes);
}catch (Exception e){
e.printStackTrace();
}
}
// 确定函数返回值的类型
ArrayList structFieldNames = new ArrayList<>();
structFieldNames.add("country");
structFieldNames.add("area");
structFieldNames.add("province");
structFieldNames.add("city");
structFieldNames.add("isp");
ArrayList structFieldObjectInspectors = new ArrayList<>();
structFieldObjectInspectors.add(PrimitiveObjectInspectorFactory.javaStringObjectInspector);
structFieldObjectInspectors.add(PrimitiveObjectInspectorFactory.javaStringObjectInspector);
structFieldObjectInspectors.add(PrimitiveObjectInspectorFactory.javaStringObjectInspector);
structFieldObjectInspectors.add(PrimitiveObjectInspectorFactory.javaStringObjectInspector);
structFieldObjectInspectors.add(PrimitiveObjectInspectorFactory.javaStringObjectInspector);
return ObjectInspectorFactory.getStandardStructObjectInspector(structFieldNames, structFieldObjectInspectors);
}
/**
* 处理数据
* @param arguments
* @return
* @throws HiveException
*/
@Override
public Object evaluate(DeferredObject[] arguments) throws HiveException {
String ip = arguments[1].get().toString();
ArrayList