Title
题目
Intraindividual Comparison of Different Methods for Automated BPE Assessment at Breast MRI:
个体内比较不同自动化背景增强(BPE)评估方法在乳腺MRI中的效果:
Background
背景
The level of background parenchymal enhancement (BPE) at breast MRI provides predictive and prognostic information and can have diagnostic implications. However, there is a lack of standardization regarding BPE assessment.
乳腺MRI中的背景实质增强(BPE)水平提供了预测和预后信息,并可能具有诊断意义。然而,目前关于BPE评估的标准化仍然缺乏。
Method
方法
In this pseudoprospective analysis of 5773 breast MRI examinations from 3207 patients (mean age, 60 years ± 10 [SD]), the level of BPE was prospectively categorized according to the Breast Imaging Reporting and Data System by radiologists experienced in breast MRI. For automated extraction of BPE, fibroglandular tissue (FGT) was segmented in an automated pipeline. Four different published methods for automated quantitative BPE extractions were used: two methods (A and B) based on enhancement intensity and two methods (C and D) based on the volume of enhanced FGT. The results from all methods were correlated, and agreement was investigated in comparison with the respective radiologist-based categorization. For surrogate validation of BPE assessment, how accurately the methods distinguished premenopausal women with (n = 50) versus without (n = 896) antihormonal treatment was determined.
在对3207名患者(平均年龄60岁 ± 10 [SD])的5773例乳腺MRI检查进行的伪前瞻性分析中,背景实质增强(BPE)的水平由经验丰富的乳腺MRI放射科医师按照乳腺影像报告和数据系统(BI-RADS)进行前瞻性分类。为了自动提取BPE,纤维腺体组织(FGT)在自动化流程中被分割。使用了四种不同的已发布的自动化定量BPE提取方法:两种方法(A和B)基于增强强度,两种方法(C和D)基于增强FGT的体积。对所有方法的结果进行了相关性分析,并与放射科医师基于分类的结果进行了比较。为了验证BPE评估的替代准确性,评估了这些方法区分接受(n = 50)与未接受(n = 896)抗激素治疗的围绝经期女性的准确性。
Conclusion
结论
Results of different methods for quantitative BPE assessment agree only moderately among themselves or with visual categories reported by experienced radiologists; intensity-based methods correlate more closely with radiologists' ratings than volumebased methods.
不同的定量背景实质增强(BPE)评估方法之间的结果仅有中等程度的一致性,与经验丰富的放射科医师报告的视觉分类也只有适度的一致性。基于强度的方法与放射科医师的评分的相关性比基于体积的方法更高。
Results
结果
Intensity-based methods (A and B) exhibited a correlation with radiologist-based categorization of 0.56 ± 0.01 and 0.55 ± 0.01, respectively, and volume-based methods (C and D) had a correlation of 0.52 ± 0.01 and 0.50 ± 0.01 (P < .001). There were notable correlation differences (P < .001) between the BPE determined with the four methods. Among the four quantitation methods, method D offered the highest accuracy for distinguishing women with versus without antihormonal therapy (P = .01).
基于强度的方法(A和B)与放射科医师分类的相关性分别为0.56 ± 0.01和0.55 ± 0.01,而基于体积的方法(C和D)的相关性分别为0.52 ± 0.01和0.50 ± 0.01(P < .001)。四种方法之间的BPE结果存在显著的相关性差异(P < .001)。在这四种定量方法中,方法D在区分接受与未接受抗激素治疗的女性方面提供了最高的准确性(P = .01)。
Figure
图
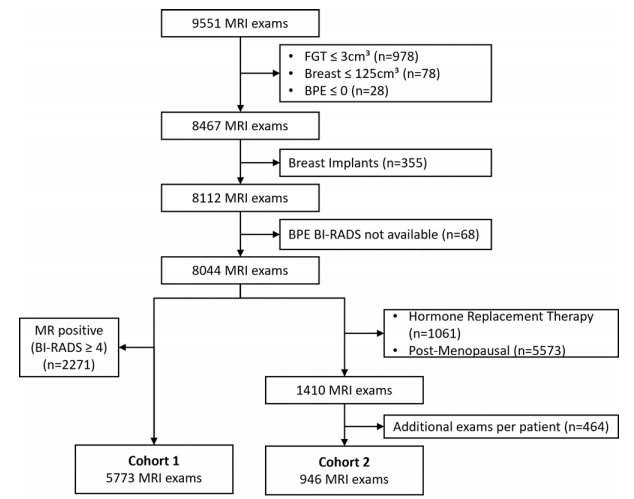
Figure 1: Study flow diagram. BI-RADS = Breast Imaging Reporting and Data System, BPE = background parenchymal enhancement, FGT = fibroglandular tissue
图1: 研究流程图。BI-RADS = 乳腺影像报告和数据系统,BPE = 背景实质增强,FGT = 纤维腺体组织
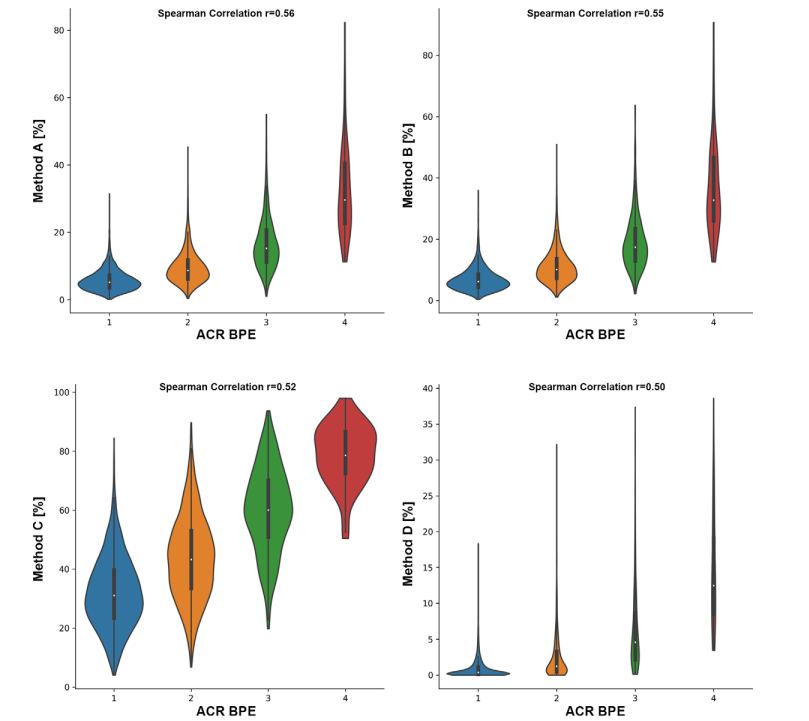
Figure 2: Violin plots show quantitative versus qualitative assessment of background parenchymal enhancement (BPE). The x-axis denotes the American College of Radiology (ACR) Breast Imaging Reporting and Data System category as determined by radiologists experienced in breast MRI. The y-axis denotes the quantitative value determined with methods A, B, C, and D. The correlation coefficient between qualitative and quantitative assessment was 0.56 for method A, 0.55 for method B, 0.52 for method C, and 0.50 for method D.
图2: 小提琴图显示了背景实质增强(BPE)的定量评估与定性评估的对比。x轴表示由经验丰富的乳腺MRI放射科医师确定的美国放射学会(ACR)乳腺影像报告和数据系统(BI-RADS)分类。y轴表示使用方法A、B、C和D确定的定量值。定性评估与定量评估之间的相关系数分别为方法A的0.56,方法B的0.55,方法C的0.52,以及方法D的0.50。
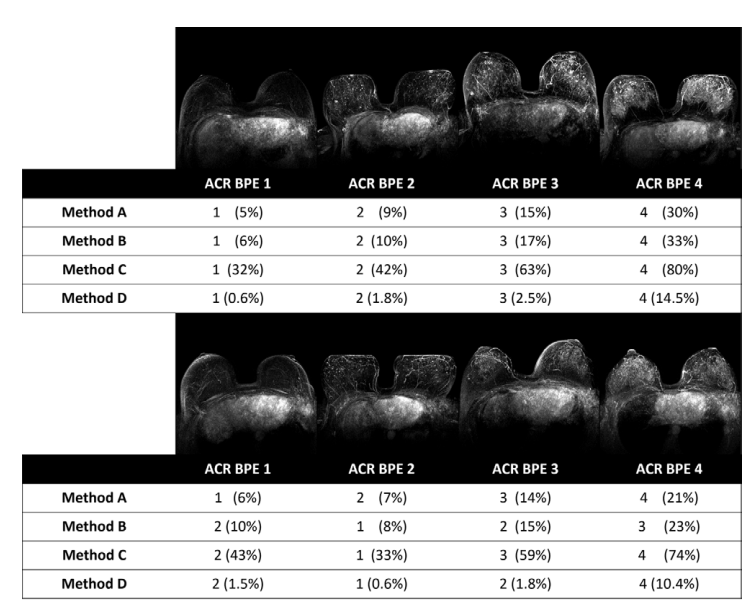
Figure 3: Maximum intensity projections of the subtraction between the first postcontrast and precontrast sequences of illustrative axial MRI examinations along with the American College of Radiology (ACR) Breast Imaging Reporting and Data System categories assigned by the radiologist and the automated methods. The upper part of the figure displays illustrative examinations that exhibit agreement among all methods and with the radiologists' assessments. In the lower part of the figure, method A is in agreement with the qualitative rating by the radiologists, while methods B, C, and D exhibit deviations from the radiologists' rating. Note that the projections may show subtraction artifacts due to motion between the pre- and postcontrast T1-weighted images, but this does not influence method A, as it determines the intensity on the pre- and postcontrast images independently. BPE = background parenchymal enhancement.
图3: 轴位MRI检查中,最大强度投影显示了首次对比增强与前对比序列之间的减法图像,以及由放射科医师和自动化方法分配的美国放射学会(ACR)乳腺影像报告和数据系统(BI-RADS)分类。图的上部展示了所有方法与放射科医师评估结果一致的示例检查。在图的下部,方法A与放射科医师的定性评级一致,而方法B、C和D则显示出与放射科医师评级的偏差。请注意,由于预对比和后对比T1加权图像之间的运动,投影可能会出现减法伪影,但这不会影响方法A,因为方法A独立于前后对比图像确定强度。BPE = 胶原质背景增强。
Table
表
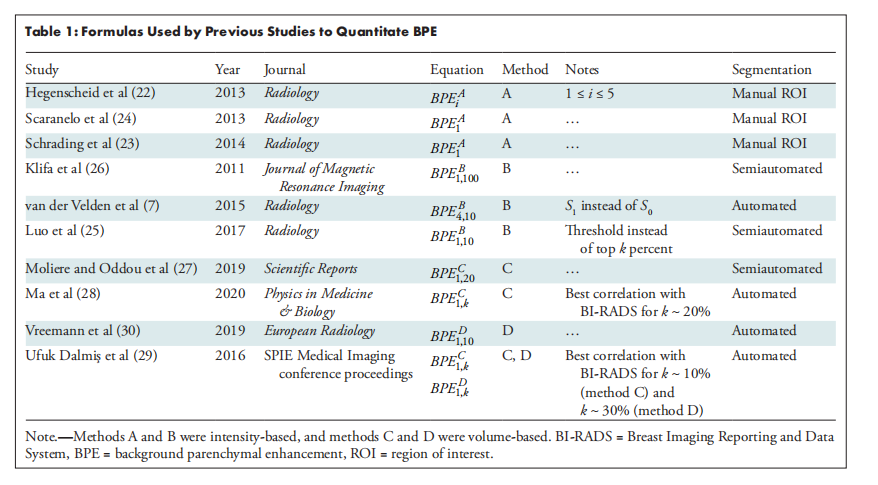
Table 1: Formulas Used by Previous Studies to Quantitate BPE
表1: 以往研究中用于量化背景实质增强(BPE)的公式
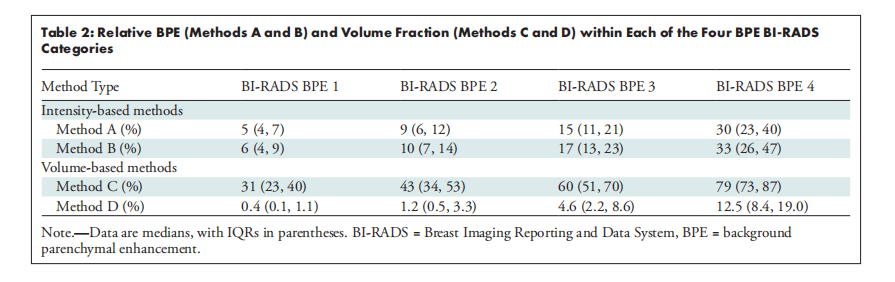
Table 2: Relative BPE (Methods A and B) and Volume Fraction (Methods C and D) within Each of the Four BPE BI-RADS Categoris
表2: 四种背景实质增强(BPE)BI-RADS分类中相对BPE(方法A和B)与体积分数(方法C和D)的比较
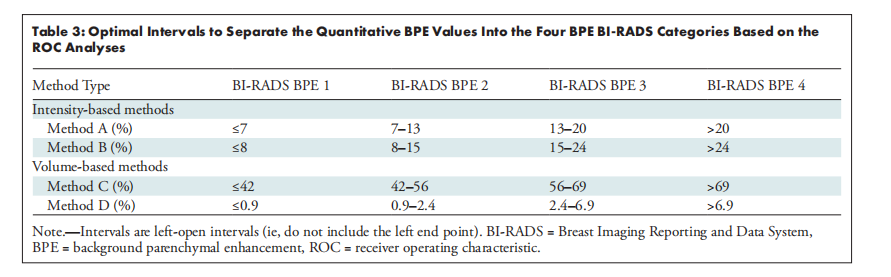
Table 3: Optimal Intervals to Separate the Quantitative BPE Values Into the Four BPE BI-RADS Categories Based on the ROC Analyses
表3: 基于ROC分析将定量BPE值划分为四个BPE BI-RADS类别的最佳区间
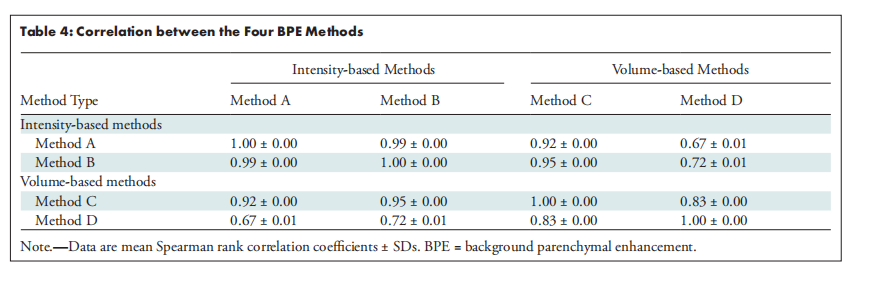
Table 4: Correlation between the Four BPE Methods
表4: 四种BPE方法之间的相关