一、上下文
《Spark-SparkSubmit详细过程》详细分析了从脚本提交任务后driver是如何调用到自己编写的Spark代码的,而我们的Spark代码在运行前必须准备好分布式资源,接下来我们就分析下资源是如何分配的
二、Spark代码示例
我们以一个简单的WordCount程序为例,来分析Spark后端是如何为这个程序分配资源的
Scala
object WordCount {
def main(args: Array[String]): Unit = {
//可以通过 SparkConf 为 Spark 绝大多数配置设置参数,且这些参数的优先级要高于系统属性
//注意:一旦 SparkConf 传递给 Spark 后,就无法再对其进行修改,因为Spark不支持运行时修改
val conf = new SparkConf().setAppName("WordCount")
//Spark 的主要入口点 SparkContext 表示到Spark集群的连接,用于在该集群上创建RDD、累加器、广播变量
//每个JVM只能有一个 SparkContext 处于活动状态
val sc = new SparkContext(conf)
//从HDFS、本地文件系统(在所有节点上都可用)或任何Hadoop支持的文件系统URI读取文本文件,并将其作为字符串的RDD返回。
val sourceRdd = sc.textFile("file/word_count_data.txt")
//原始一行数据:js,c,vba,json,xml
//flatMap将每行数据按照逗号分割,得到每个单词 形成 (单词1) (单词2) (单词1) ... 的格式
//map将每个单词的次数都赋值成1形成 (单词1,1) (单词2,1) (单词1,次数) ... 的格式
//reduceByKey将相同单词中的次数进行累加
val resultRdd = sourceRdd.flatMap(_.split(",")).map(x=>{(x,1)}).reduceByKey(_+_)
//打印结果
resultRdd.foreach(println)
//停止SparkContext
sc.stop()
}
SparkConf 是对该程序的一个属性设置,且支持链式设置,会覆盖默认的系统属性。一旦程序开始运行就不可以对其再修改了。
SparkContext是程序与Spark集群连接的入口,可以用于在该集群上创建RDD、广播变量、累加器等等,那么RDD运行所需的资源肯定是在创建SparkContext时就已经具备好的。下面我们看看SparkContext中是如何结合spark-submit参数来协调资源的
三、SparkContext
Scala
class SparkContext(config: SparkConf) extends Logging {
//创建一个从系统属性加载设置的SparkContext(例如,使用./bin/spark-submit启动时)
def this() = this(new SparkConf())
//......省略.......
//私有变量。这些变量保留了上下文的内部状态,外部世界无法访问。它们是可变的
//因为我们想提前将它们初始化为一个中性值,这样在构造函数仍在运行时调用"stop()"是安全的。
//只列举重要的一些属性
//支持应用的个性化配置
private var _conf: SparkConf = _
//一个正在运行的Spark实例的所有运行时环境对象(无论是master还是worker),包括序列化器、RpcEnv、块管理器、映射输出跟踪器等。目前,Spark代码通过全局变量查找SparkEnv,因此所有线程都可以访问相同的SparkEnv。它可以通过SparkEnv.get访问(例如在创建SparkContext之后)
//我们后面单独分析下它
private var _env: SparkEnv = _
//用于调度系统的后端接口,允许在TaskSchedulerImpl下插入不同的系统。我们假设一个类似Mesos的模型,当机器可用时,应用程序会获得资源供应,并可以在机器上启动任务。
private var _schedulerBackend: SchedulerBackend = _
//低级任务调度程序接口,目前由[[org.apache.sspark.scheduler.TaskSchedulerImpl]]专门实现。
//此接口允许插入不同的任务调度器。每个TaskScheduler都为单个SparkContext安排任务。
//这些调度器从DAGScheduler获取每个阶段提交给它们的任务集,并负责将任务发送到集群、运行它们、在出现故障时重试,以及缓解延迟。他们将事件返回给DAGScheduler。
private var _taskScheduler: TaskScheduler = _
//一个常住在 Driver端的 HeartbeatReceiver 通信端点 ,用来接收所有 executors 的心跳
private var _heartbeatReceiver: RpcEndpointRef = _
//实现面向 stage 调度的高级调度层。它为每个作业计算一个 stage 的DAG,
//跟踪哪些RDD和stage输出被物化,并找到运行作业的最小时间表。
//然后,它将stage作为TaskSet提交给在集群上运行它们的底层TaskScheduler实现。
//TaskSet包含完全独立的任务,这些任务可以根据集群上已有的数据
@volatile private var _dagScheduler: DAGScheduler = _
try {
//......省略.......
//创建SparkEnv
_env = createSparkEnv(_conf, isLocal, listenerBus)
//设置 常住driver端的 _heartbeatReceiver 的 Endpoint
_heartbeatReceiver = env.rpcEnv.setupEndpoint(
HeartbeatReceiver.ENDPOINT_NAME, new HeartbeatReceiver(this))
// 创建并启动调度程序
val (sched, ts) = SparkContext.createTaskScheduler(this, master, deployMode)
_schedulerBackend = sched
_taskScheduler = ts
_dagScheduler = new DAGScheduler(this)
_heartbeatReceiver.ask[Boolean](TaskSchedulerIsSet)
//启动任务调度器
_taskScheduler.start()
//......省略.......
//基于给定的主URL创建任务调度器。返回调度器后端和任务调度器的 2-tuple
private def createTaskScheduler(...){
//......省略.......
master match {
case "local" =>
case LOCAL_N_REGEX(threads) =>
case LOCAL_N_FAILURES_REGEX(threads, maxFailures) =>
case SPARK_REGEX(sparkUrl) =>
//创建一个 任务调度实现类 TaskSchedulerImpl
val scheduler = new TaskSchedulerImpl(sc)
val masterUrls = sparkUrl.split(",").map("spark://" + _)
//创建一个 StandaloneSchedulerBackend 是 SchedulerBackend 的子类
val backend = new StandaloneSchedulerBackend(scheduler, sc, masterUrls)
//初始化 任务调度器
scheduler.initialize(backend)
(backend, scheduler)
case LOCAL_CLUSTER_REGEX(numWorkers, coresPerWorker, memoryPerWorker) =>
case masterUrl =>
}
}
四、TaskSchedulerImpl
Scala
private[spark] class TaskSchedulerImpl(
val sc: SparkContext,
val maxTaskFailures: Int,
isLocal: Boolean = false,
clock: Clock = new SystemClock)
extends TaskScheduler with Logging {
//初始化
def initialize(backend: SchedulerBackend): Unit = {
this.backend = backend
//构建调度器 默认是 FIFO 调度 可以通过 spark.scheduler.mode 进行配置
schedulableBuilder = {
schedulingMode match {
case SchedulingMode.FIFO =>
new FIFOSchedulableBuilder(rootPool)
case SchedulingMode.FAIR =>
new FairSchedulableBuilder(rootPool, sc)
case _ =>
throw new IllegalArgumentException(s"Unsupported $SCHEDULER_MODE_PROPERTY: " +
s"$schedulingMode")
}
}
schedulableBuilder.buildPools()
}
//启动调度程序
override def start(): Unit = {
//SparkContext中创建的是 StandaloneSchedulerBackend 因此会调用 它的 start()
//StandaloneSchedulerBackend 又会调用其父类CoarseGrainedSchedulerBackend 的 start()
backend.start()
if (!isLocal && conf.get(SPECULATION_ENABLED)) {
logInfo("Starting speculative execution thread")
speculationScheduler.scheduleWithFixedDelay(
() => Utils.tryOrStopSparkContext(sc) { checkSpeculatableTasks() },
SPECULATION_INTERVAL_MS, SPECULATION_INTERVAL_MS, TimeUnit.MILLISECONDS)
}
}
}
五、CoarseGrainedSchedulerBackend
Scala
//等待粗粒度执行器连接的调度程序后端。此后端在Spark作业期间保留每个执行器,而不是在任务完成时放弃执行器,
//并要求调度器为每个新任务启动一个新的执行器。执行器可以通过多种方式启动,例如粗粒度Mesos模式的Mesos任务或Spark独立部署模式(Spark.deploy.*)的独立进程。
class CoarseGrainedSchedulerBackend(scheduler: TaskSchedulerImpl, val rpcEnv: RpcEnv)
extends ExecutorAllocationClient with SchedulerBackend with Logging {
//这里会创建并注册一个 DriverEndpoint ,且 DriverEndpoint的 onStart() 方法会执行
val driverEndpoint = rpcEnv.setupEndpoint(ENDPOINT_NAME, createDriverEndpoint())
protected def createDriverEndpoint(): DriverEndpoint = new DriverEndpoint()
override def start(): Unit = {
}
class DriverEndpoint extends IsolatedRpcEndpoint with Logging {
override def onStart(): Unit = {
// 定期恢复下 以允许延迟调度工作
val reviveIntervalMs = conf.get(SCHEDULER_REVIVE_INTERVAL).getOrElse(1000L)
reviveThread.scheduleAtFixedRate(() => Utils.tryLogNonFatalError {
Option(self).foreach(_.send(ReviveOffers))
}, 0, reviveIntervalMs, TimeUnit.MILLISECONDS)
}
override def receiveAndReply(context: RpcCallContext): PartialFunction[Any, Unit] = {
case RegisterExecutor(executorId, executorRef, hostname, cores, logUrls,
attributes, resources, resourceProfileId) =>
if (executorDataMap.contains(executorId)) {
context.sendFailure(new IllegalStateException(s"Duplicate executor ID: $executorId"))
} else if (scheduler.excludedNodes.contains(hostname) ||
isExecutorExcluded(executorId, hostname)) {
// 如果集群管理器在被排除的节点上为我们提供了一个Executor(因为在我们通知它我们的排除之前,它已经开始分配这些资源,或者如果它忽略了我们的排除),那么我们会立即拒绝该Executor
logInfo(s"Rejecting $executorId as it has been excluded.")
context.sendFailure(
new IllegalStateException(s"Executor is excluded due to failures: $executorId"))
} else {
//如果Executor 的rpc-env没有监听传入连接,则"hostPort"将为null,应使用客户端连接联系Executor 。
val executorAddress = if (executorRef.address != null) {
executorRef.address
} else {
context.senderAddress
}
logInfo(s"Registered executor $executorRef ($executorAddress) with ID $executorId, " +
s" ResourceProfileId $resourceProfileId")
addressToExecutorId(executorAddress) = executorId
totalCoreCount.addAndGet(cores)
totalRegisteredExecutors.addAndGet(1)
val resourcesInfo = resources.map { case (rName, info) =>
// 这必须同步,因为在请求Executor时会读取此块中突变的变量
val numParts = scheduler.sc.resourceProfileManager
.resourceProfileFromId(resourceProfileId).getNumSlotsPerAddress(rName, conf)
(info.name, new ExecutorResourceInfo(info.name, info.addresses, numParts))
}
val data = new ExecutorData(executorRef, executorAddress, hostname,
0, cores, logUrlHandler.applyPattern(logUrls, attributes), attributes,
resourcesInfo, resourceProfileId, registrationTs = System.currentTimeMillis())
// This must be synchronized because variables mutated
// in this block are read when requesting executors
CoarseGrainedSchedulerBackend.this.synchronized {
executorDataMap.put(executorId, data)
if (currentExecutorIdCounter < executorId.toInt) {
currentExecutorIdCounter = executorId.toInt
}
}
listenerBus.post(
SparkListenerExecutorAdded(System.currentTimeMillis(), executorId, data))
// Note: some tests expect the reply to come after we put the executor in the map
context.reply(true)
}
}
override def receive: PartialFunction[Any, Unit] = {
//启动 executor
case LaunchedExecutor(executorId) =>
executorDataMap.get(executorId).foreach { data =>
data.freeCores = data.totalCores
}
//仅仅为一个 executor 提供虚假资源 offer
makeOffers(executorId)
}
}
}
六、StandaloneSchedulerBackend
Scala
//Spark独立集群管理器的[[SchedulerBackend]]实现。
private[spark] class StandaloneSchedulerBackend(
scheduler: TaskSchedulerImpl,
sc: SparkContext,
masters: Array[String])
extends CoarseGrainedSchedulerBackend(scheduler, sc.env.rpcEnv)
with StandaloneAppClientListener
with Logging {
override def start(): Unit = {
super.start()
//调度器后端应仅在客户端模式下尝试连接到启动器。
//在集群模式下,将应用程序提交给Master的代码需要连接到启动器。
if (sc.deployMode == "client") {
launcherBackend.connect()
}
//executors 中的 endpoint 需要持有 driver的地址 用于和 driver通信
val driverUrl = RpcEndpointAddress(
sc.conf.get(config.DRIVER_HOST_ADDRESS),
sc.conf.get(config.DRIVER_PORT),
CoarseGrainedSchedulerBackend.ENDPOINT_NAME).toString
val args = Seq(
"--driver-url", driverUrl,
"--executor-id", "{{EXECUTOR_ID}}",
"--hostname", "{{HOSTNAME}}",
"--cores", "{{CORES}}",
"--app-id", "{{APP_ID}}",
"--worker-url", "{{WORKER_URL}}")
val extraJavaOpts = sc.conf.get(config.EXECUTOR_JAVA_OPTIONS)
.map(Utils.splitCommandString).getOrElse(Seq.empty)
val classPathEntries = sc.conf.get(config.EXECUTOR_CLASS_PATH)
.map(_.split(java.io.File.pathSeparator).toSeq).getOrElse(Nil)
val libraryPathEntries = sc.conf.get(config.EXECUTOR_LIBRARY_PATH)
.map(_.split(java.io.File.pathSeparator).toSeq).getOrElse(Nil)
//使用一些必要的配置启动 executors ,以便在调度程序中注册
val sparkJavaOpts = Utils.sparkJavaOpts(conf, SparkConf.isExecutorStartupConf)
val javaOpts = sparkJavaOpts ++ extraJavaOpts
// executors 端的主类 CoarseGrainedExecutorBackend
val command = Command("org.apache.spark.executor.CoarseGrainedExecutorBackend",
args, sc.executorEnvs, classPathEntries ++ testingClassPath, libraryPathEntries, javaOpts)
val webUrl = sc.ui.map(_.webUrl).getOrElse("")
val coresPerExecutor = conf.getOption(config.EXECUTOR_CORES.key).map(_.toInt)
// 如果我们使用动态分配,现在将初始执行器限制设置为0。ExecutorDallocationManager稍后会将实际的初始限额发送给Master。
val initialExecutorLimit =
if (Utils.isDynamicAllocationEnabled(conf)) {
Some(0)
} else {
None
}
val executorResourceReqs = ResourceUtils.parseResourceRequirements(conf,
config.SPARK_EXECUTOR_PREFIX)
//这里有一个 ApplicationDescription 之前有要给 DriverDescription
//可以想到 它是用来启动一个 Application 用的
val appDesc = ApplicationDescription(sc.appName, maxCores, sc.executorMemory, command,
webUrl, sc.eventLogDir, sc.eventLogCodec, coresPerExecutor, initialExecutorLimit,
resourceReqsPerExecutor = executorResourceReqs)
//创建一个 StandaloneAppClient
//允许应用程序与Spark独立集群管理器对话。获取集群事件的主URL、应用程序描述和侦听器,并在发生各种事件时回调侦听器。
client = new StandaloneAppClient(sc.env.rpcEnv, masters, appDesc, this, conf)
//启动它
client.start()
launcherBackend.setState(SparkAppHandle.State.SUBMITTED)
waitForRegistration()
launcherBackend.setState(SparkAppHandle.State.RUNNING)
}
}
七、StandaloneAppClient
Scala
private[spark] class StandaloneAppClient(...){
private val masterRpcAddresses = masterUrls.map(RpcAddress.fromSparkURL(_))
private val REGISTRATION_TIMEOUT_SECONDS = 20
private val REGISTRATION_RETRIES = 3
private class ClientEndpoint(override val rpcEnv: RpcEnv) extends ThreadSafeRpcEndpoint
with Logging {
override def onStart(): Unit = {
//向Master注册一个app
registerWithMaster(1)
}
//异步向所有 Master 注册。它将每隔 20 秒调用"registerWithMaster",直到超过 3 次数。一旦我们成功连接到主机,所有调度工作和期货都将被取消。
//thRetry表示这是第n次尝试向master注册。
private def registerWithMaster(nthRetry: Int): Unit = {
registerMasterFutures.set(tryRegisterAllMasters())
registrationRetryTimer.set(registrationRetryThread.schedule(new Runnable {
override def run(): Unit = {
if (registered.get) {
registerMasterFutures.get.foreach(_.cancel(true))
registerMasterThreadPool.shutdownNow()
} else if (nthRetry >= REGISTRATION_RETRIES) {
markDead("All masters are unresponsive! Giving up.")
} else {
registerMasterFutures.get.foreach(_.cancel(true))
registerWithMaster(nthRetry + 1)
}
}
}, REGISTRATION_TIMEOUT_SECONDS, TimeUnit.SECONDS))
}
}
private def tryRegisterAllMasters(): Array[JFuture[_]] = {
for (masterAddress <- masterRpcAddresses) yield {
registerMasterThreadPool.submit(new Runnable {
override def run(): Unit = try {
if (registered.get) {
return
}
logInfo("Connecting to master " + masterAddress.toSparkURL + "...")
val masterRef = rpcEnv.setupEndpointRef(masterAddress, Master.ENDPOINT_NAME)
//向Master 发送 RegisterApplication 消息
masterRef.send(RegisterApplication(appDescription, self))
} catch {
case ie: InterruptedException => // Cancelled
case NonFatal(e) => logWarning(s"Failed to connect to master $masterAddress", e)
}
})
}
}
def start(): Unit = {
// 只需启动一个rpcEndpoint;它将呼叫回听众
//设置一个 AppClient 端点 ClientEndpoint 的 onstart 的方法会调起
endpoint.set(rpcEnv.setupEndpoint("AppClient", new ClientEndpoint(rpcEnv)))
}
}
八、Master
Scala
private[deploy] class Master(...){
//处理其他端点的消息
override def receive: PartialFunction[Any, Unit] = {
case RegisterApplication(description, driver) =>
// TODO Prevent repeated registrations from some driver
if (state == RecoveryState.STANDBY) {
// ignore, don't send response
} else {
logInfo("Registering app " + description.name)
//创建一个 app
val app = createApplication(description, driver)
//driver端添加app的持有 比如 在waitingApps 中添加 这个 app 为后续调度做准备
registerApplication(app)
logInfo("Registered app " + description.name + " with ID " + app.id)
//持久化这个app
persistenceEngine.addApplication(app)
//给driver返回一个已经注册的响应
driver.send(RegisteredApplication(app.id, self))
//调度,开始在 Worker 上 分配 executors
schedule()
}
}
//创建一个app
private def createApplication(desc: ApplicationDescription, driver: RpcEndpointRef):
ApplicationInfo = {
val now = System.currentTimeMillis()
val date = new Date(now)
val appId = newApplicationId(date)
//且会自己执行 init()
new ApplicationInfo(now, appId, desc, date, driver, defaultCores)
}
private def schedule(): Unit = {
if (state != RecoveryState.ALIVE) {
return
}
val shuffledAliveWorkers = Random.shuffle(workers.toSeq.filter(_.state == WorkerState.ALIVE))
val numWorkersAlive = shuffledAliveWorkers.size
var curPos = 0
//启动等待的所有 driver 目前 driver 已经启动了,跳过这一步
for (driver <- waitingDrivers.toList) {
...
}
//在Workers上启动Executors
startExecutorsOnWorkers()
}
//调度并在 workers 上 启动 Executors
private def startExecutorsOnWorkers(): Unit = {
//现在这是一个非常简单的FIFO调度器。依次对等待的 app 进行调度
for (app <- waitingApps) {
val coresPerExecutor = app.desc.coresPerExecutor.getOrElse(1)
// 如果剩余的内核小于coresPerExecutor,则不会分配剩余的内核
// 简单理解就是 app剩下的核 要满足最少是一个 executor 所需的核数 ,也就是以 executor 所需的核数为单位 进行分配 executor 最少的 核数为 1
if (app.coresLeft >= coresPerExecutor) {
//过滤掉哪些 没有足够资源 的 worker 并按照剩余的核数倒序排序 来依次启动 executors
val usableWorkers = workers.toArray.filter(_.state == WorkerState.ALIVE)
.filter(canLaunchExecutor(_, app.desc))
.sortBy(_.coresFree).reverse
val appMayHang = waitingApps.length == 1 &&
waitingApps.head.executors.isEmpty && usableWorkers.isEmpty
if (appMayHang) {
logWarning(s"App ${app.id} requires more resource than any of Workers could have.")
}
//真正去计算资源分配 返回要给 数组 里面有分配好的核数
val assignedCores = scheduleExecutorsOnWorkers(app, usableWorkers, spreadOutApps)
//现在我们已经决定了在每个worker上分配多少个内核,让我们分配它们
for (pos <- 0 until usableWorkers.length if assignedCores(pos) > 0) {
allocateWorkerResourceToExecutors(
app, assignedCores(pos), app.desc.coresPerExecutor, usableWorkers(pos))
}
}
}
}
//调度 executors 去 workers 上启动。返回一个数组,其中包含分配给每个worker的核心数。
//有两种启动executor的模式。
// 第一种方法试图将应用程序的executor分散到尽可能多的worker进程上,默认设置,更适合数据局部性
// 第二种方法则相反(即在尽可能少的worker进程中启动它们)。
//分配给每个executor的核心数量是可配置的。当显式设置此选项时,如果worker进程有足够的内核和内存,
//则可以在同一worker进程上启动来自同一应用程序的多个executor。
//否则,默认情况下,每个executor都会抓取worker上可用的所有内核,
// 在这种情况下,在一次单独的计划迭代中,每个应用程序只能在每个worker上启动一个executor。
//请注意,当未设置"spark.executor.cores"时,
// 我们仍然可以在同一个worker上从同一个应用程序启动多个executor。
// 假设appA和appB都有一个executor在worker1上运行,并且appA.coresLef>0,则appB完成并释放worker1上的所有内核,
// 因此对于下一个计划迭代,appA启动一个新的executor,抓取worker1上所有空闲的内核,因此我们从运行在worker1的appA中获得多个executor。
private def scheduleExecutorsOnWorkers(
app: ApplicationInfo,
usableWorkers: Array[WorkerInfo],
spreadOutApps: Boolean): Array[Int] = {
//每个Executor所需的核数
val coresPerExecutor = app.desc.coresPerExecutor
//每个Executor所需的最小核数
val minCoresPerExecutor = coresPerExecutor.getOrElse(1)
//每个worker一个Executor
val oneExecutorPerWorker = coresPerExecutor.isEmpty
//每个Executor所需的内存
val memoryPerExecutor = app.desc.memoryPerExecutorMB
//每个Executor所需的资源
val resourceReqsPerExecutor = app.desc.resourceReqsPerExecutor
//可用的worker数量
val numUsable = usableWorkers.length
val assignedCores = new Array[Int](numUsable) // 去每个worker上需要申请的核数
val assignedExecutors = new Array[Int](numUsable) // 去每个worker上需要申请的executor数量
var coresToAssign = math.min(app.coresLeft, usableWorkers.map(_.coresFree).sum)
/**返回指定的worker是否可以为此app启动Executor */
def canLaunchExecutorForApp(pos: Int): Boolean = {
val keepScheduling = coresToAssign >= minCoresPerExecutor
val enoughCores = usableWorkers(pos).coresFree - assignedCores(pos) >= minCoresPerExecutor
val assignedExecutorNum = assignedExecutors(pos)
//如果我们允许每个worker有多个executor,那么我们总是可以启动新的executor。
//否则,如果这个worker上已经有一个executor,只需给它更多的内核。
val launchingNewExecutor = !oneExecutorPerWorker || assignedExecutorNum == 0
if (launchingNewExecutor) {
val assignedMemory = assignedExecutorNum * memoryPerExecutor
val enoughMemory = usableWorkers(pos).memoryFree - assignedMemory >= memoryPerExecutor
val assignedResources = resourceReqsPerExecutor.map {
req => req.resourceName -> req.amount * assignedExecutorNum
}.toMap
val resourcesFree = usableWorkers(pos).resourcesAmountFree.map {
case (rName, free) => rName -> (free - assignedResources.getOrElse(rName, 0))
}
val enoughResources = ResourceUtils.resourcesMeetRequirements(
resourcesFree, resourceReqsPerExecutor)
val underLimit = assignedExecutors.sum + app.executors.size < app.executorLimit
keepScheduling && enoughCores && enoughMemory && enoughResources && underLimit
} else {
//我们正在为现有的执行器添加内核,因此无需检查内存和执行器限制
keepScheduling && enoughCores
}
}
//过滤可以启动executor 的worker
var freeWorkers = (0 until numUsable).filter(canLaunchExecutorForApp)
//对可以启动executor 的worker循环进行 executors 的分配
while (freeWorkers.nonEmpty) {
freeWorkers.foreach { pos =>
var keepScheduling = true
while (keepScheduling && canLaunchExecutorForApp(pos)) {
coresToAssign -= minCoresPerExecutor
assignedCores(pos) += minCoresPerExecutor
if (oneExecutorPerWorker) {
assignedExecutors(pos) = 1
} else {
assignedExecutors(pos) += 1
}
//分散app意味着将其executor分散到尽可能多的worker中。如果我们不分散,
//那么我们应该继续在这个worker上调度executor,直到我们使用了它的所有资源。
//否则,请转到下一个worker。 默认 keepScheduling = true
if (spreadOutApps) {
keepScheduling = false
}
}
}
freeWorkers = freeWorkers.filter(canLaunchExecutorForApp)
}
assignedCores
}
//将Worke的资源分配给一个或多个Executor。
private def allocateWorkerResourceToExecutors(
app: ApplicationInfo,
assignedCores: Int,
coresPerExecutor: Option[Int],
worker: WorkerInfo): Unit = {
//如果指定了每个Executor的Cores,我们将分配给此worker的核心平均分配给Executor 没有余数。
//否则,我们将启动一个Executor,获取此worker上所有分配的Cores。
val numExecutors = coresPerExecutor.map { assignedCores / _ }.getOrElse(1)
val coresToAssign = coresPerExecutor.getOrElse(assignedCores)
//一个一个executor去worker上启动
for (i <- 1 to numExecutors) {
val allocated = worker.acquireResources(app.desc.resourceReqsPerExecutor)
//这里面会创建一个 ExecutorDesc 作为 Executor 启动的描述 像之前的app、driver都有这个描述
val exec = app.addExecutor(worker, coresToAssign, allocated)
//启动Executor
launchExecutor(worker, exec)
app.state = ApplicationState.RUNNING
}
}
private def launchExecutor(worker: WorkerInfo, exec: ExecutorDesc): Unit = {
//在那个 worker 上启动 executor
logInfo("Launching executor " + exec.fullId + " on worker " + worker.id)
worker.addExecutor(exec)
//Master endpoint 向 worker endpoint 发送 LaunchExecutor 消息
worker.endpoint.send(LaunchExecutor(masterUrl, exec.application.id, exec.id,
exec.application.desc, exec.cores, exec.memory, exec.resources))
//Master endpoint 向 driverendpoint 发送 ExecutorAdded消息
exec.application.driver.send(
ExecutorAdded(exec.id, worker.id, worker.hostPort, exec.cores, exec.memory))
}
}
九、Worker
Scala
private[deploy] class Worker(......
extends ThreadSafeRpcEndpoint with Logging {
override def receive: PartialFunction[Any, Unit] = synchronized {
case LaunchExecutor(masterUrl, appId, execId, appDesc, cores_, memory_, resources_) =>
if (masterUrl != activeMasterUrl) {
logWarning("Invalid Master (" + masterUrl + ") attempted to launch executor.")
} else if (decommissioned) {
logWarning("Asked to launch an executor while decommissioned. Not launching executor.")
} else {
try {
logInfo("Asked to launch executor %s/%d for %s".format(appId, execId, appDesc.name))
// 创建 executor 的 本地工作目录
val executorDir = new File(workDir, appId + "/" + execId)
if (!executorDir.mkdirs()) {
throw new IOException("Failed to create directory " + executorDir)
}
// 为执行者创建本地目录。这些通过SPARK_EXECUTOR_DIRS环境变量传递给executor,并在应用程序完成时由Worker删除。
val appLocalDirs = appDirectories.getOrElse(appId, {
val localRootDirs = Utils.getOrCreateLocalRootDirs(conf)
val dirs = localRootDirs.flatMap { dir =>
try {
val appDir = Utils.createDirectory(dir, namePrefix = "executor")
Utils.chmod700(appDir)
Some(appDir.getAbsolutePath())
} catch {
...
}
}.toSeq
dirs
})
appDirectories(appId) = appLocalDirs
//管理一个executor流程的执行。这目前仅在standalone模式下使用。
val manager = new ExecutorRunner(
appId,
execId,
appDesc.copy(command = Worker.maybeUpdateSSLSettings(appDesc.command, conf)),
cores_,
memory_,
self,
workerId,
webUi.scheme,
host,
webUi.boundPort,
publicAddress,
sparkHome,
executorDir,
workerUri,
conf,
appLocalDirs,
ExecutorState.LAUNCHING,
resources_)
executors(appId + "/" + execId) = manager
manager.start()
coresUsed += cores_
memoryUsed += memory_
addResourcesUsed(resources_)
} catch {
......
}
}
}
}
十、ExecutorRunner
Scala
private[deploy] class ExecutorRunner(...){
private[worker] def start(): Unit = {
//准备一个线程启动 executor
workerThread = new Thread("ExecutorRunner for " + fullId) {
override def run(): Unit = { fetchAndRunExecutor() }
}
//线程启动 fetchAndRunExecutor() 执行 :下载并运行应用程序描述中描述的executor
//第6步中封装的启动类为 org.apache.spark.executor.CoarseGrainedExecutorBacken
//下面我们看看 executor 中做了什么
workerThread.start()
......
}
}
十一、CoarseGrainedExecutorBacken(executor进程主类)
Scala
private[spark] object CoarseGrainedExecutorBackend extends Logging {
def main(args: Array[String]): Unit = {
val createFn: (RpcEnv, Arguments, SparkEnv, ResourceProfile) =>
CoarseGrainedExecutorBackend = { case (rpcEnv, arguments, env, resourceProfile) =>
new CoarseGrainedExecutorBackend(rpcEnv, arguments.driverUrl, arguments.executorId,
arguments.bindAddress, arguments.hostname, arguments.cores, arguments.userClassPath.toSeq,
env, arguments.resourcesFileOpt, resourceProfile)
}
//解析参数并运行
run(parseArguments(args, this.getClass.getCanonicalName.stripSuffix("$")), createFn)
System.exit(0)
}
def run(
arguments: Arguments,
backendCreateFn: (RpcEnv, Arguments, SparkEnv, ResourceProfile) =>
CoarseGrainedExecutorBackend): Unit = {
//......
// 创建 RpcEnv 获取 driver端的 Spark properties.
val executorConf = new SparkConf
val fetcher = RpcEnv.create(
"driverPropsFetcher",
arguments.bindAddress,
arguments.hostname,
-1,
executorConf,
new SecurityManager(executorConf),
numUsableCores = 0,
clientMode = true)
//尝试3次获取 driver Endpoint 引用
var driver: RpcEndpointRef = null
val nTries = 3
for (i <- 0 until nTries if driver == null) {
try {
driver = fetcher.setupEndpointRefByURI(arguments.driverUrl)
} catch {
case e: Throwable => if (i == nTries - 1) {
throw e
}
}
}
// 向driver发送 RetrieveSparkAppConfig 消息
val cfg = driver.askSync[SparkAppConfig](RetrieveSparkAppConfig(arguments.resourceProfileId))
val props = cfg.sparkProperties ++ Seq[(String, String)](("spark.app.id", arguments.appId))
fetcher.shutdown()
//根据从driver端获取的属性创建SparkEnv
val driverConf = new SparkConf()
for ((key, value) <- props) {
// this is required for SSL in standalone mode
if (SparkConf.isExecutorStartupConf(key)) {
driverConf.setIfMissing(key, value)
} else {
driverConf.set(key, value)
}
}
cfg.hadoopDelegationCreds.foreach { tokens =>
SparkHadoopUtil.get.addDelegationTokens(tokens, driverConf)
}
driverConf.set(EXECUTOR_ID, arguments.executorId)
//为executor创建SparkEnv
val env = SparkEnv.createExecutorEnv(driverConf, arguments.executorId, arguments.bindAddress,
arguments.hostname, arguments.cores, cfg.ioEncryptionKey, isLocal = false)
// 在BlockStoreClient中设置应用程序尝试ID(如果可用)
val appAttemptId = env.conf.get(APP_ATTEMPT_ID)
appAttemptId.foreach(attemptId =>
env.blockManager.blockStoreClient.setAppAttemptId(attemptId)
)
//创建CoarseGrainedExecutorBackend
val backend = backendCreateFn(env.rpcEnv, arguments, env, cfg.resourceProfile)
//并将CoarseGrainedExecutorBackend设置为 Executor Endpoint 其上的 onStart() 方法执行
env.rpcEnv.setupEndpoint("Executor", backend)
arguments.workerUrl.foreach { url =>
env.rpcEnv.setupEndpoint("WorkerWatcher",
new WorkerWatcher(env.rpcEnv, url, isChildProcessStopping = backend.stopping))
}
env.rpcEnv.awaitTermination()
}
}
}
十二、CoarseGrainedExecutorBackend(executor中的Rpc端点)
Scala
private[spark] class CoarseGrainedExecutorBackend(...)
extends IsolatedRpcEndpoint with ExecutorBackend with Logging {
//当driver成功接受注册请求时,内部用于启动executor的消息。
case object RegisteredExecutor
override def onStart(): Unit = {
//......
logInfo("Connecting to driver: " + driverUrl)
try {
//提供了一个实用程序,用于将Spark JVM内的SparkConf(例如,执行器、驱动程序或独立的shuffle服务)转换为TransportConf,其中包含有关我们环境的详细信息,如分配给此JVM的内核数量。
val shuffleClientTransportConf = SparkTransportConf.fromSparkConf(env.conf, "shuffle")
//判断Netty是否可以直接使用 off-heap 内存 且 操作系统能分配的最大 off-heap < 200M 抛出异常
//涉及配置
// spark.network.sharedByteBufAllocators.enabled 默认true 是否在不同Netty通道之间共享池化ByteBuf分配器的标志。如果启用,则只创建两个池化ByteBuf分配器:一个允许缓存(用于传输服务器),另一个不允许缓存(对于传输客户端)。禁用后,将为每个传输服务器和客户端创建一个新的分配器。
// spark.io.preferDirectBufs 默认true 共享ByteBuf分配器将首选堆外字节缓冲区
// spark.network.io.preferDirectBufs 默认true 在Netty中分配堆外字节缓冲区
if (NettyUtils.preferDirectBufs(shuffleClientTransportConf) &&
PlatformDependent.maxDirectMemory() < env.conf.get(MAX_REMOTE_BLOCK_SIZE_FETCH_TO_MEM)) {
throw new SparkException(s"Netty direct memory should at least be bigger than " +
s"'${MAX_REMOTE_BLOCK_SIZE_FETCH_TO_MEM.key}', but got " +
s"${PlatformDependent.maxDirectMemory()} bytes < " +
s"${env.conf.get(MAX_REMOTE_BLOCK_SIZE_FETCH_TO_MEM)}")
}
_resources = parseOrFindResources(resourcesFileOpt)
} catch {
case NonFatal(e) =>
exitExecutor(1, "Unable to create executor due to " + e.getMessage, e)
}
rpcEnv.asyncSetupEndpointRefByURI(driverUrl).flatMap { ref =>
//这是一个非常快速的操作,因此我们可以使用"ThreadUtils.sameThread"
driver = Some(ref)
//向driver 发送 RegisterExecutor 消息 ,我们看第五步中driver的处理
ref.ask[Boolean](RegisterExecutor(executorId, self, hostname, cores, extractLogUrls,
extractAttributes, _resources, resourceProfile.id))
}(ThreadUtils.sameThread).onComplete {
case Success(_) =>
self.send(RegisteredExecutor)
case Failure(e) =>
exitExecutor(1, s"Cannot register with driver: $driverUrl", e, notifyDriver = false)
}(ThreadUtils.sameThread)
}
override def receive: PartialFunction[Any, Unit] = {
//driver收到注册 executor 并返回 true 执行该段逻辑
case RegisteredExecutor =>
logInfo("Successfully registered with driver")
try {
//创建一个 Executor
executor = new Executor(executorId, hostname, env, userClassPath, isLocal = false,
resources = _resources)
//再向driver发送 LaunchedExecutor 消息,可以看 第五步 driver端的处理
driver.get.send(LaunchedExecutor(executorId))
} catch {
case NonFatal(e) =>
exitExecutor(1, "Unable to create executor due to " + e.getMessage, e)
}
}
}
十三、Executor
Scala
//Spark执行器,由线程池支持运行任务。
//这可以与Mesos、YARN、kubernetes和独立调度器一起使用。内部RPC接口用于与driver通信,Mesos细粒度模式除外。
private[spark] class Executor(...){
// 维护正在运行的任务列表
private val runningTasks = new ConcurrentHashMap[Long, TaskRunner]
//当Executor无法向driver程序发送心跳超过"HEARTBEAT_MAX_FAILURES"次数时,它应该自行终止。默认值为60。例如,如果最大失败次数为60次,心跳间隔为10秒,则它将尝试发送长达600秒(10分钟)的心跳。
private val HEARTBEAT_MAX_FAILURES = conf.get(EXECUTOR_HEARTBEAT_MAX_FAILURES)
//发送心跳的间隔(毫秒) spark.executor.heartbeatInterval 默认 10s
private val HEARTBEAT_INTERVAL_MS = conf.get(EXECUTOR_HEARTBEAT_INTERVAL)
//heartbeat 任务
private val heartbeater = new Heartbeater(
() => Executor.this.reportHeartBeat(),
"executor-heartbeater",
HEARTBEAT_INTERVAL_MS)
//启动工作线程池
private val threadPool = {
val threadFactory = new ThreadFactoryBuilder()
.setDaemon(true)
.setNameFormat("Executor task launch worker-%d")
.setThreadFactory((r: Runnable) => new UninterruptibleThread(r, "unused"))
.build()
Executors.newCachedThreadPool(threadFactory).asInstanceOf[ThreadPoolExecutor]
}
//启动任务
def launchTask(context: ExecutorBackend, taskDescription: TaskDescription): Unit = {
val tr = new TaskRunner(context, taskDescription, plugins)
runningTasks.put(taskDescription.taskId, tr)
threadPool.execute(tr)
if (decommissioned) {
log.error(s"Launching a task while in decommissioned state.")
}
}
}
十四、总结
1、代码中根据SparkConf构建SparkContext
2、创建任务调度器并启用
3、StandaloneSchedulerBackend 和 CoarseGrainedSchedulerBackend 的 start() 启动
4、DriverEndpoint 创建 等待其他Endpoint发送消息 (比如Master 和 Executur Endpoint)
5、构建Executor的启动参数,主类为CoarseGrainedExecutorBackend
6、创建StandaloneAppClient并启动
7、Driver端创建ClientEndpoint并向Master注册
8、创建app描述信息向Master发送RegisterApplication 消息
9、Master 根据app描述信息开始调度资源,决策在哪些Worker上启动多少个Executor
10、Master端以Executor为单位依次向划分好的Worker发送LaunchExecutor消息,向Driver发送ExecutorAdded消息
11、Worker 创建一个线程启动封装好的Executor进程(主类为CoarseGrainedExecutorBackend)
12、Executor中会创建Executor Endpoint,并向Driver进行注册,如果注册成功会向自己发送RegisteredExecutor消息
13、Executor处理给自己发的RegisteredExecutor消息,其中会创建一个Executor对象并向Driver发送LaunchedExecutor消息
14、Executor对象由线程池支持运行任务,且并默认每隔10s发送依次心跳给Driver
为了方便理解和记忆,我们也画下流程图,下载放大就会清晰哟
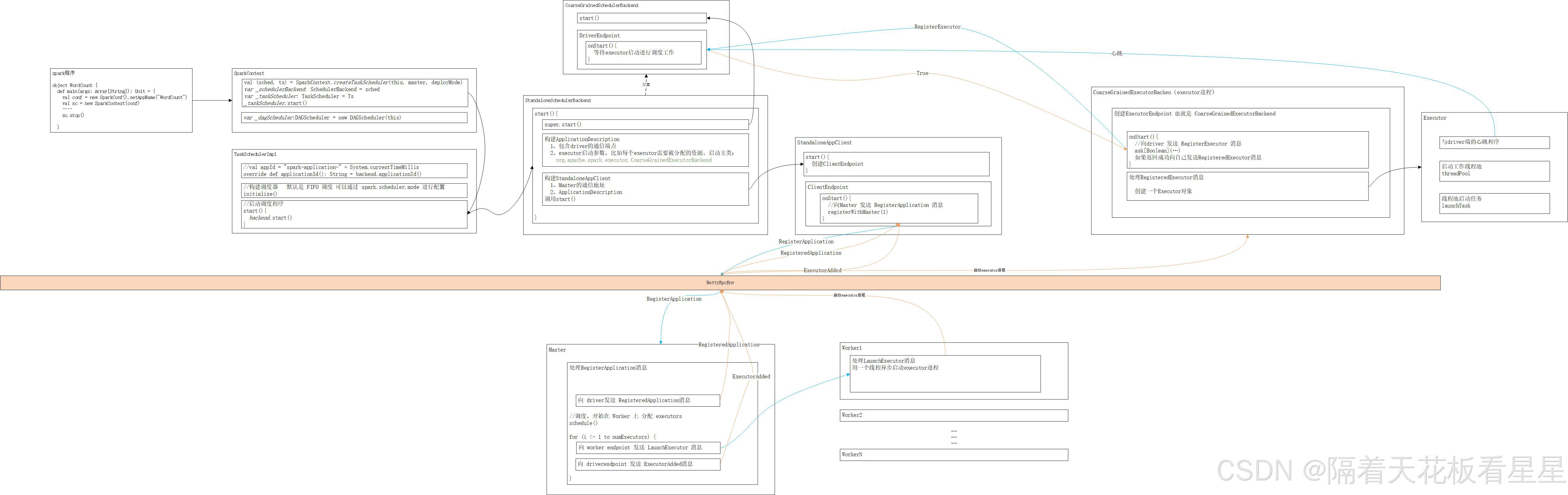