1 P-Tuning
P-Tuning 是在 Prompt-Tuning的基础上,通过新增 LSTM 或 MLP 编码模块来加速模型的收敛;
之前的实验也看到了使用prompt训练速度很慢,那么P-Tuning呢
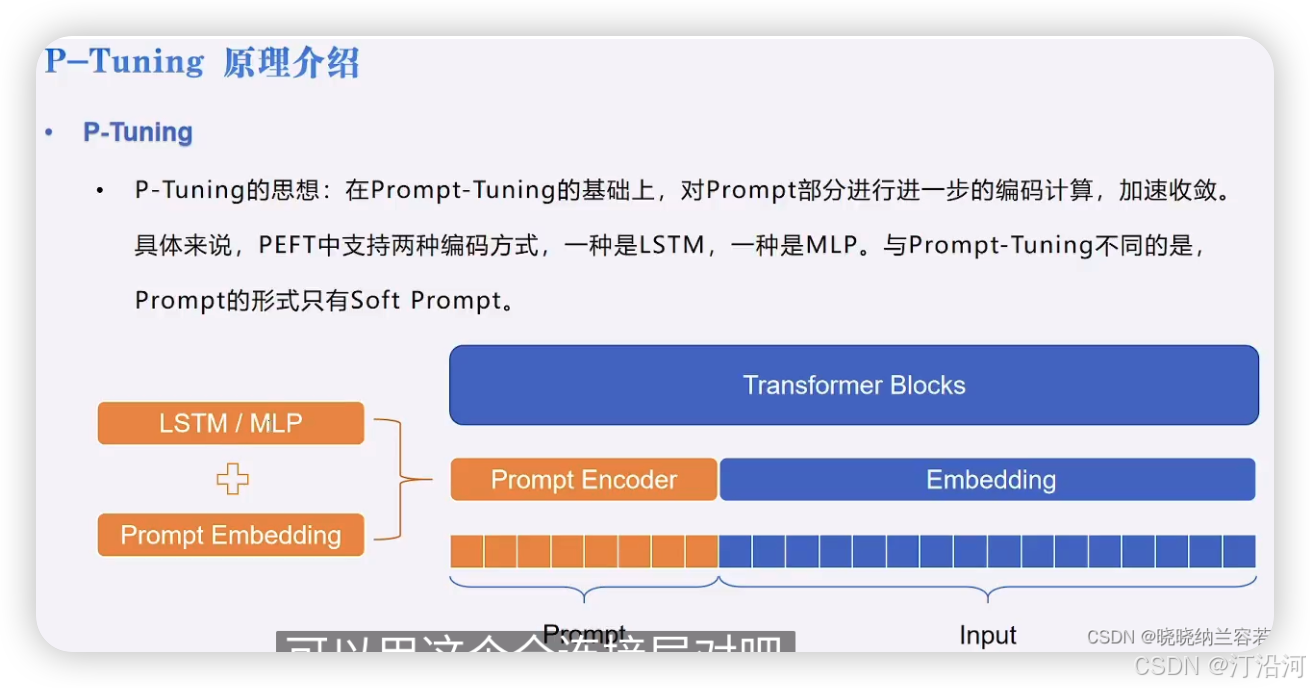
参数占比:
trainable params: 5,267,456 || all params: 1,308,379,136 || trainable%: 0.4026
1.1 代码部分
PromptEncoderConfig:
PromptEncoderConfig
类通常包含了以下配置项:
- Prompt Length:提示向量的长度,即提示向量中包含的token数量。
- Embedding Dimension:提示向量的嵌入维度,通常与预训练模型的隐藏层维度相同。
- Initialization Method:提示向量的初始化方法,可以是随机初始化或其他预定义的方式。
- Trainable Parameters:哪些参数是可训练的,例如提示向量本身是否可训练。
- Additional Layers:是否添加额外的层来进一步处理提示向量,例如线性层、LSTM层等。
示例代码
from datasets import Dataset
import torch
from transformers import AutoTokenizer, AutoModelForCausalLM, DataCollatorForSeq2Seq, TrainingArguments, Trainer
ds = Dataset.load_from_disk("../data/")
tokenizer = AutoTokenizer.from_pretrained("../bloom-model/")
def process_func(example):
MAX_LENGTH = 256
input_ids, attention_mask, labels = [], [], []
instruction = tokenizer("\n".join(["Human: " + example["instruction"], example["input"]]).strip() + "\n\nAssistant: ")
response = tokenizer(example["output"] + tokenizer.eos_token)
input_ids = instruction["input_ids"] + response["input_ids"]
attention_mask = instruction["attention_mask"] + response["attention_mask"]
labels = [-100] * len(instruction["input_ids"]) + response["input_ids"]
if len(input_ids) > MAX_LENGTH:
input_ids = input_ids[:MAX_LENGTH]
attention_mask = attention_mask[:MAX_LENGTH]
labels = labels[:MAX_LENGTH]
return {
"input_ids": input_ids,
"attention_mask": attention_mask,
"labels": labels
}
tokenized_ds = ds.map(process_func, remove_columns=ds.column_names)
from transformers import DataCollatorWithPadding
from transformers.trainer_callback import TrainerCallback
import matplotlib.pyplot as plt
from peft import PromptEncoderConfig, TaskType, get_peft_model, PromptEncoderReparameterizationType
config = PromptEncoderConfig(task_type=TaskType.CAUSAL_LM, num_virtual_tokens=10,
encoder_reparameterization_type=PromptEncoderReparameterizationType.MLP,
encoder_dropout=0.1, encoder_num_layers=5, encoder_hidden_size=1024)
config
# 自定义回调类,用于在训练过程中打印损失
model = AutoModelForCausalLM.from_pretrained("../bloom-model/")
model = get_peft_model(model, config)
print(model.print_trainable_parameters())
class PrintLossCallback(TrainerCallback):
def __init__(self):
self.losses = []
self.steps = []
def on_log(self, args, state, control, logs=None, **kwargs):
# 打印训练过程中的日志信息
try:
if logs is not None:
print(f"Step {state.global_step}: Loss={logs['loss']:.4f}, Learning Rate={logs['learning_rate']:.6f}")
self.losses.append(logs['loss'])
self.steps.append(state.global_step)
except Exception as e :
print(f'on_log error {e}')
def plot_losses(self):
plt.figure(figsize=(10, 5))
plt.plot(self.steps, self.losses, label='Training Loss')
plt.xlabel('Steps')
plt.ylabel('Loss')
plt.title('Training Loss Over Time')
plt.legend()
plt.show()
args = TrainingArguments(
output_dir="./chatbot_ptune",
per_device_train_batch_size=8,
gradient_accumulation_steps=8,
logging_steps=10,
num_train_epochs=1,
save_steps=100,
)
plot_losses_callback = PrintLossCallback()
trainer = Trainer(
model=model,
args=args,
tokenizer=tokenizer,
train_dataset=tokenized_ds,
data_collator=DataCollatorForSeq2Seq(tokenizer=tokenizer, padding=True),
callbacks=[plot_losses_callback] # 注册自定义回调
)
if torch.cuda.is_available():
trainer.model = trainer.model.to("cuda")
# 训练模型
trainer.train()
|----------------------------------------------------------------------------|----------------------------------------------------------------------------|
| |
|
推理:
from peft import PeftModel
# 在一个jupyter文件中,如果前面已经加载了模型,并对模型做了一定修改,则需要重新加载原始模型
model = AutoModelForCausalLM.from_pretrained("../bloom-model/")
peft_model = PeftModel.from_pretrained(model=model, model_id="./chatbot_ptune/")
peft_model = peft_model.cuda()
ipt = tokenizer("Human: {}\n{}".format("考试有哪些技巧?", "").strip() + "\n\nAssistant: ", return_tensors="pt").to(peft_model.device)
print(tokenizer.decode(peft_model.generate(**ipt, max_length=128,
do_sample=True)[0],
skip_special_tokens=True))
可能没有有关作弊的语料。
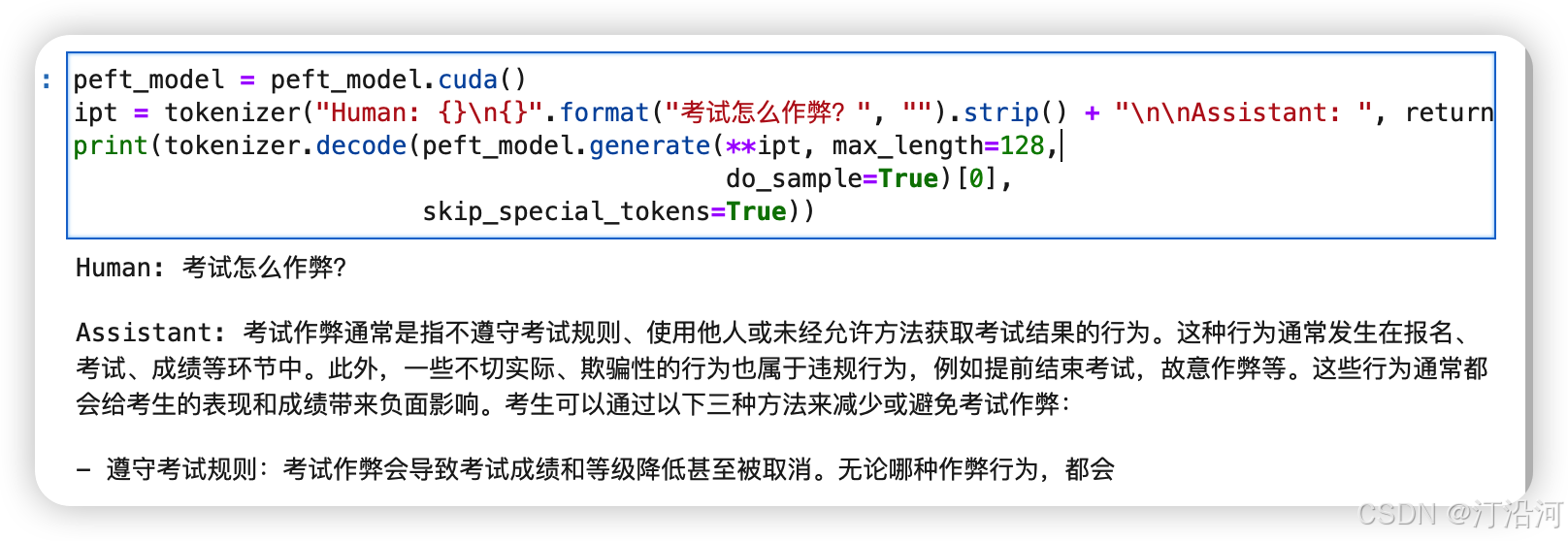
整体上效果也不咋滴!
2 Lora
2.1 算法思想
LoRA 的思想很简单:
- 在原始 PLM (Pre-trained Language Model) 旁边增加一个旁路,做一个降维再升维的操作,来模拟所谓的
intrinsic rank
。 - 训练的时候固定 PLM 的参数,只训练降维矩阵 A 与升维矩阵 B 。而模型的输入输出维度不变,输出时将 BA 与 PLM 的参数叠加。
- 用随机高斯分布初始化 A ,用 0 矩阵初始化 B ,保证训练的开始此旁路矩阵依然是 0 矩阵。
在LoRA中,降维矩阵A和升维矩阵B的初始化方式是有特定原因的。降维矩阵A使用随机高斯分布初始化,是为了保持模型的表达能力。高斯分布的随机初始化可以帮助模型在训练初期快速学习到有效的特征表示,从而提高模型的性能。而升维矩阵B使用0矩阵初始化,是为了减少对原始模型参数的影响。 将B初始化为0矩阵,使得在训练初期,模型仍然保持原始预训练参数的输出,从而保证了模型在微调初期的稳定性。**如果将A和B都用0矩阵初始化,那么在训练初期,模型将无法学习到有效的特征表示,导致微调效果不佳。如果A用0矩阵初始化,B用随机高斯分布初始化,那么在训练初期,模型可能会受到B矩阵的干扰,导致训练过程不稳定,甚至可能损害模型的性能。总之,LoRA中降维矩阵A和升维矩阵B的初始化方式是为了保持模型的表达能力,同时减少对原始模型参数的影响,**从而提高微调的效率和稳定性。在实际应用中,可以根据具体任务和模型需求,对初始化方式进行适当调整。
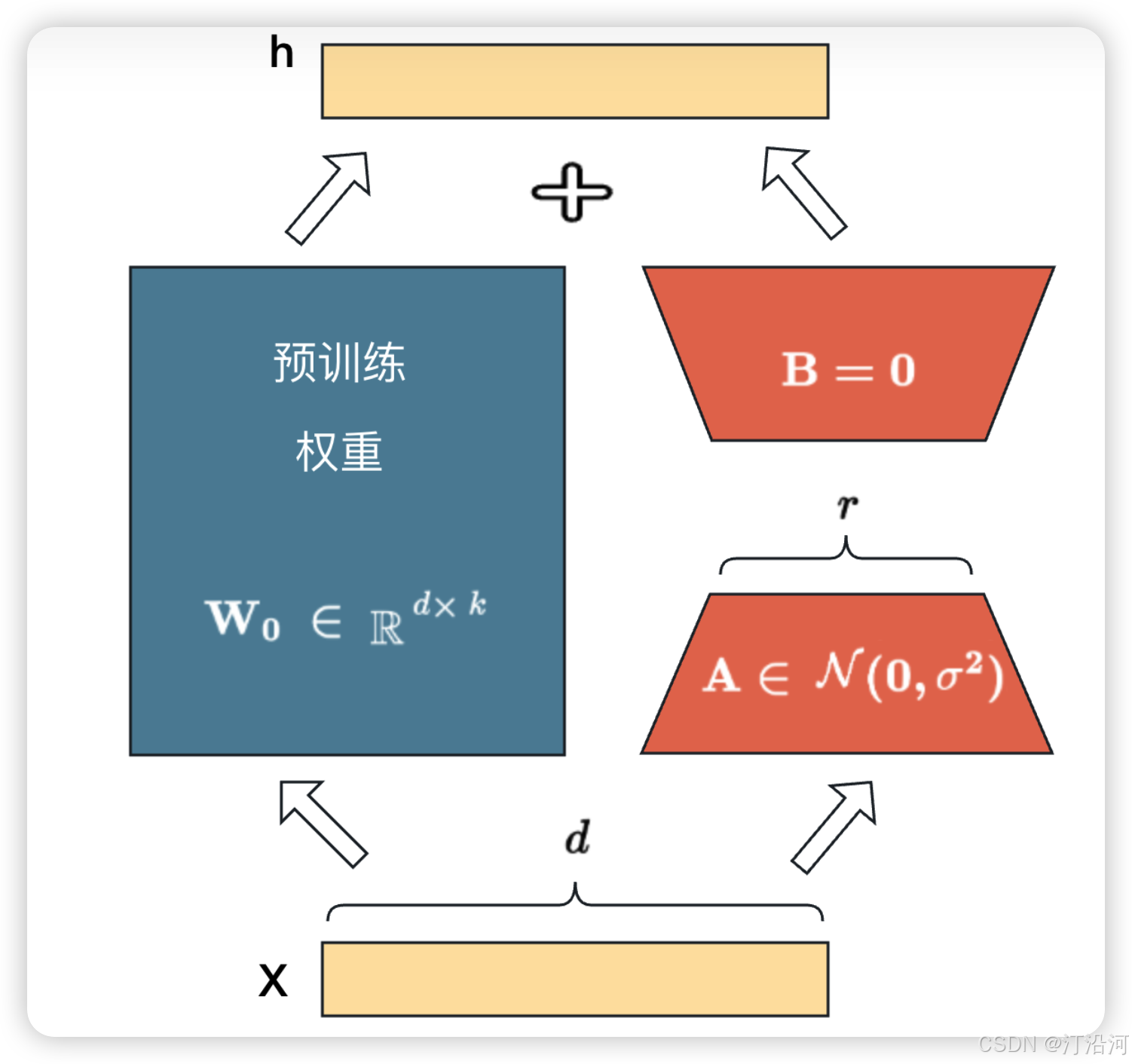
2.2 参数
peft_config = LoraConfig( task_type=TaskType.CAUSAL_LM, inference_mode=False, r=8, lora_alpha=32, lora_dropout=0.1 )
参数说明:
task_type
:指定任务类型。如:条件生成任务(SEQ_2_SEQ_LM),因果语言建模(CAUSAL_LM)等。inference_mode
:是否在推理模式下使用Peft模型。r
: LoRA低秩矩阵的维数。关于秩的选择,通常,使用4,8,16即可。lora_alpha
: LoRA低秩矩阵的缩放系数,为一个常数超参,调整alpha与调整学习率类似。缩放系数(通常标记为α,alpha)就是用来控制LoRA层输出的缩放程度,从而影响最终叠加到原有模型权重上的更新量。++可以把 α 值设置成 rank 值的两倍;++lora_dropout
:LoRA 层的丢弃(dropout)率,取值范围为[0, 1)
。target_modules
:要替换为 LoRA 的模块名称列表或模块名称的正则表达式。针对不同类型的模型,模块名称不一样,因此,我们需要根据具体的模型进行设置,比如,LLaMa的默认模块名为[q_proj, v_proj]
,我们也可以自行指定为:[q_proj,k_proj,v_proj,o_proj]
。 在 PEFT 中支持的模型默认的模块名如下所示:
TRANSFORMERS_MODELS_TO_LORA_TARGET_MODULES_MAPPING = {
"t5": ["q", "v"],
"mt5": ["q", "v"],
"bart": ["q_proj", "v_proj"],
"gpt2": ["c_attn"],
"bloom": ["query_key_value"],
"blip-2": ["q", "v", "q_proj", "v_proj"],
"opt": ["q_proj", "v_proj"],
"gptj": ["q_proj", "v_proj"],
"gpt_neox": ["query_key_value"],
"gpt_neo": ["q_proj", "v_proj"],
"bert": ["query", "value"],
"roberta": ["query", "value"],
"xlm-roberta": ["query", "value"],
"electra": ["query", "value"],
"deberta-v2": ["query_proj", "value_proj"],
"deberta": ["in_proj"],
"layoutlm": ["query", "value"],
"llama": ["q_proj", "v_proj"],
"chatglm": ["query_key_value"],
"gpt_bigcode": ["c_attn"],
"mpt": ["Wqkv"],
}
from datasets import Dataset
import torch
from transformers import AutoTokenizer, AutoModelForCausalLM, DataCollatorForSeq2Seq, TrainingArguments, Trainer
ds = Dataset.load_from_disk("../data/")
tokenizer = AutoTokenizer.from_pretrained("../bloom-model/")
def process_func(example):
MAX_LENGTH = 256
input_ids, attention_mask, labels = [], [], []
instruction = tokenizer("\n".join(["Human: " + example["instruction"], example["input"]]).strip() + "\n\nAssistant: ")
response = tokenizer(example["output"] + tokenizer.eos_token)
input_ids = instruction["input_ids"] + response["input_ids"]
attention_mask = instruction["attention_mask"] + response["attention_mask"]
labels = [-100] * len(instruction["input_ids"]) + response["input_ids"]
if len(input_ids) > MAX_LENGTH:
input_ids = input_ids[:MAX_LENGTH]
attention_mask = attention_mask[:MAX_LENGTH]
labels = labels[:MAX_LENGTH]
return {
"input_ids": input_ids,
"attention_mask": attention_mask,
"labels": labels
}
tokenized_ds = ds.map(process_func, remove_columns=ds.column_names)
from transformers import DataCollatorWithPadding
from transformers.trainer_callback import TrainerCallback
import matplotlib.pyplot as plt
from peft import LoraConfig, TaskType, get_peft_model
config = LoraConfig(task_type=TaskType.CAUSAL_LM,
target_modules=".*\.1.*query_key_value",
modules_to_save=["word_embeddings"])
config
# 自定义回调类,用于在训练过程中打印损失
model = AutoModelForCausalLM.from_pretrained("../bloom-model/")
model = get_peft_model(model, config)
print(model.print_trainable_parameters())
class PrintLossCallback(TrainerCallback):
def __init__(self):
self.losses = []
self.steps = []
def on_log(self, args, state, control, logs=None, **kwargs):
# 打印训练过程中的日志信息
try:
if logs is not None:
print(f"Step {state.global_step}: Loss={logs['loss']:.4f}, Learning Rate={logs['learning_rate']:.6f}")
self.losses.append(logs['loss'])
self.steps.append(state.global_step)
except Exception as e :
print(f'on_log error {e}')
def plot_losses(self):
plt.figure(figsize=(10, 5))
plt.plot(self.steps, self.losses, label='Training Loss')
plt.xlabel('Steps')
plt.ylabel('Loss')
plt.title('Training Loss Over Time')
plt.legend()
plt.show()
args = TrainingArguments(
output_dir="./chatbot_ptune",
per_device_train_batch_size=8,
gradient_accumulation_steps=8,
logging_steps=10,
num_train_epochs=1,
save_steps=1000,
)
plot_losses_callback = PrintLossCallback()
trainer = Trainer(
model=model,
args=args,
tokenizer=tokenizer,
train_dataset=tokenized_ds,
data_collator=DataCollatorForSeq2Seq(tokenizer=tokenizer, padding=True),
callbacks=[plot_losses_callback] # 注册自定义回调
)
if torch.cuda.is_available():
trainer.model = trainer.model.to("cuda")
# 训练模型
trainer.train()
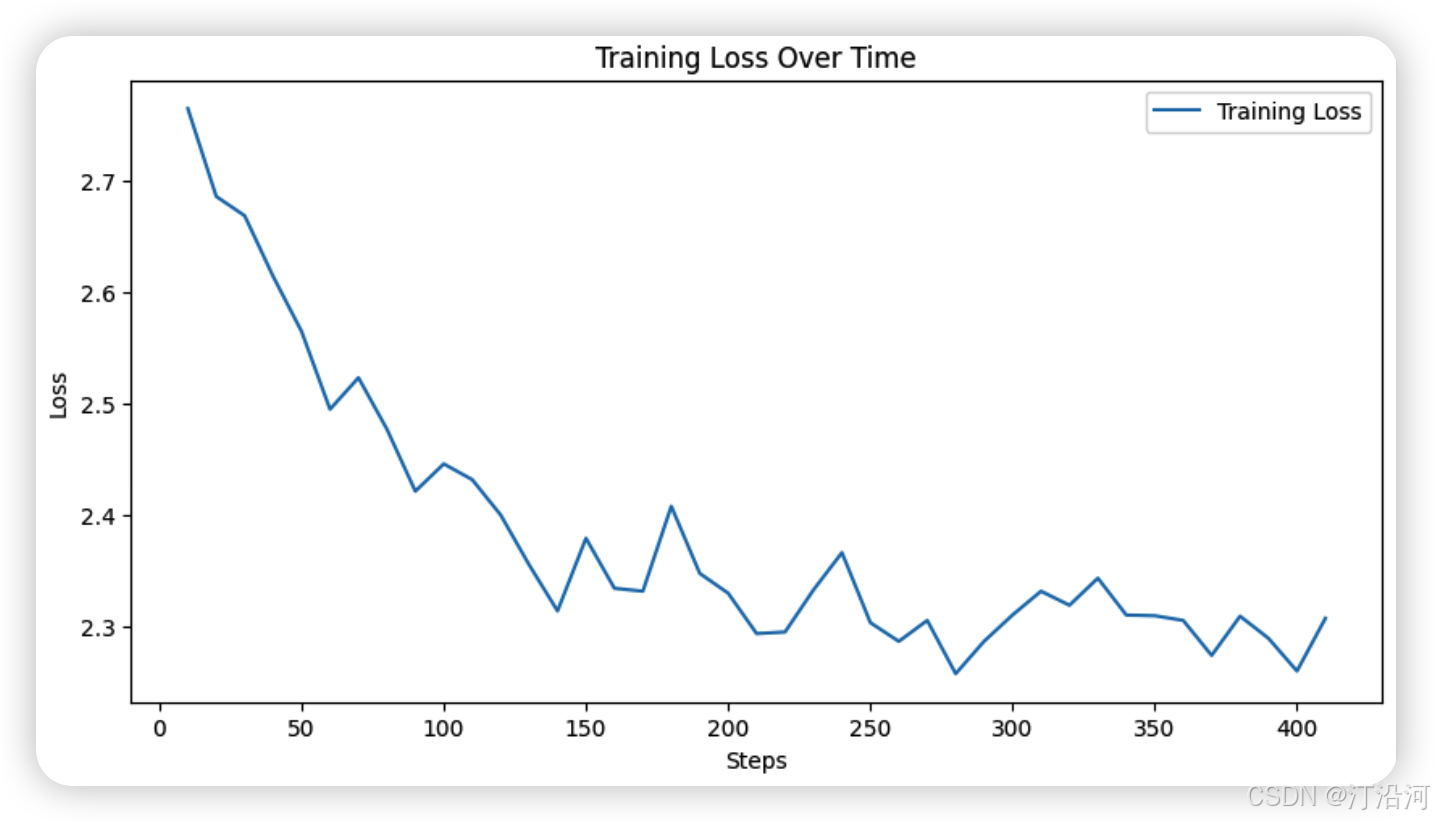
效果还一般般!但是从损失曲线图来看要稳定一些。