文章目录
- [1 预备项目](#1 预备项目)
-
- 关联规则分析实践---------购物车分析
-
- [1 产生频繁集](#1 产生频繁集)
- [2 产生关联规则](#2 产生关联规则)
1 预备项目
关联规则分析实践---------购物车分析
python
import warnings
import numpy as np
import pandas as pd
from mlxtend.frequent_patterns import apriori
from mlxtend.frequent_patterns import association_rules
warnings.filterwarnings("ignore", category=DeprecationWarning)
# 读入数据
df_Retails = pd.read_excel('input/Online Retail.xlsx')
df_Retails.head()
python
# 数据理解
print(df_Retails.shape)
df_Retails.columns
df_Retails.describe()
python
#查看国家一列的取值
df_Retails.Country.unique()
#各国家的购物数量
df_Retails["Country"].value_counts()
#查看InvoiceNo一列中是否有重复的值
df_Retails.duplicated(subset=["InvoiceNo"]).any()
#是否有缺失值
df_Retails.isna().sum()
df_Retails['InvoiceNo'].isnull().sum(axis = 0)
python
python
#空格处理
df_Retails['Description'] = df_Retails['Description'].str.strip()
# Description: Product (item) name. Nominal.
#查看是否有缺失值
df_Retails['Description'].isna().sum()
#缺失值处理
df_Retails.dropna(axis=0
, subset=['Description']
, inplace=True)
print(df_Retails.shape)
#查看是否有缺失值
print(df_Retails['Description'].isna().sum())
#删除含有C字母的已取消订单
df_Retails['InvoiceNo'] = df_Retails['InvoiceNo'].astype('str')
df_Retails = df_Retails[~df_Retails['InvoiceNo'].str.contains('C')]
df_Retails.shape
python
#将数据改为每一行一条购物记录
#考虑到内存限制只计算Germany,全部计算则计算量太大
df_ShoppingCarts = (df_Retails[df_Retails['Country'] =="Germany"]
.groupby(['InvoiceNo', 'Description'])['Quantity']
.sum()
.unstack()
.reset_index()
.fillna(0)
.set_index('InvoiceNo'))
print(df_ShoppingCarts.shape)
df_ShoppingCarts.head()
python
#查看InvoiceNo一列中是否有重复的值
df_Retails.duplicated(subset=["InvoiceNo"]).any()
def encode_units(x):
if x <= 0:
return 0
if x >= 1:
return 1
df_ShoppingCarts_sets = df_ShoppingCarts.map(encode_units)
df_ShoppingCarts_sets.head()
1 产生频繁集
python
# 产生频繁集 最小支持度为0.07, 在输出中使用原始列名
df_Frequent_Itemsets = apriori(df_ShoppingCarts_sets
, min_support=0.07
, use_colnames=True)
df_Frequent_Itemsets
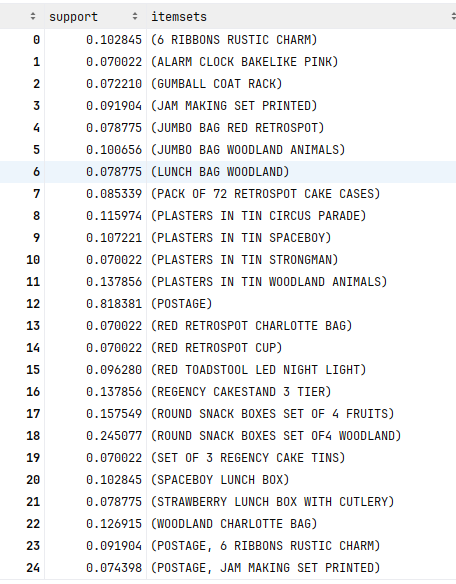
2 产生关联规则
python
# 生成关联规则,使用提升度(lift)作为度量 置提升度的最小阈值为 1,表示无正相关关系的规则也会被计算
df_AssociationRules = association_rules(df_Frequent_Itemsets
, metric="lift"
, min_threshold=1)
#输出结果的解读:https://rasbt.github.io/mlxtend/user_guide/frequent_patterns/association_rules/
df_AssociationRules.head()

python
# 筛选关联规则 筛选提升度不小于 2 置信度不小于 0.8 的关联规则
df_A= df_AssociationRules[(df_AssociationRules['lift'] >= 2) &
(df_AssociationRules['confidence'] >= 0.8) ]
df_A

python
# 可视化结果
import seaborn as sns
import matplotlib.pyplot as plt
sns.scatterplot(x = "support"
, y = "confidence"
, size = "lift"
, data = df_AssociationRules)
plt.show()
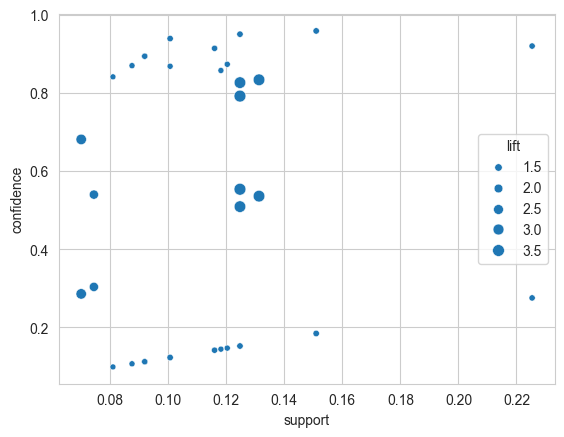