航拍工程车识别检测数据集 yolo数据集 共650张
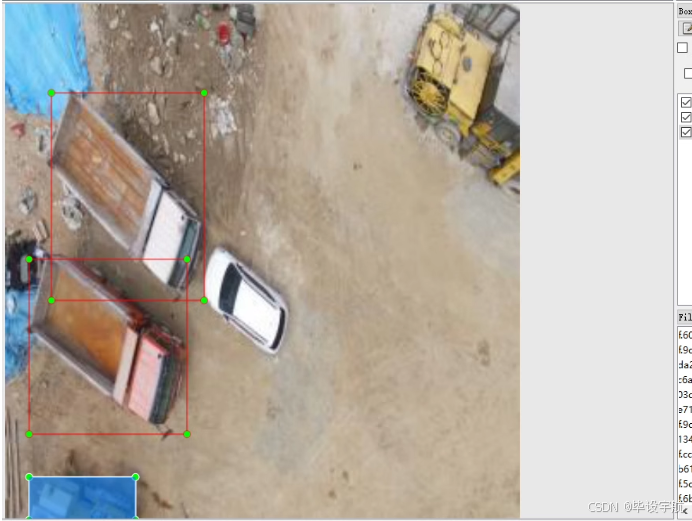
2
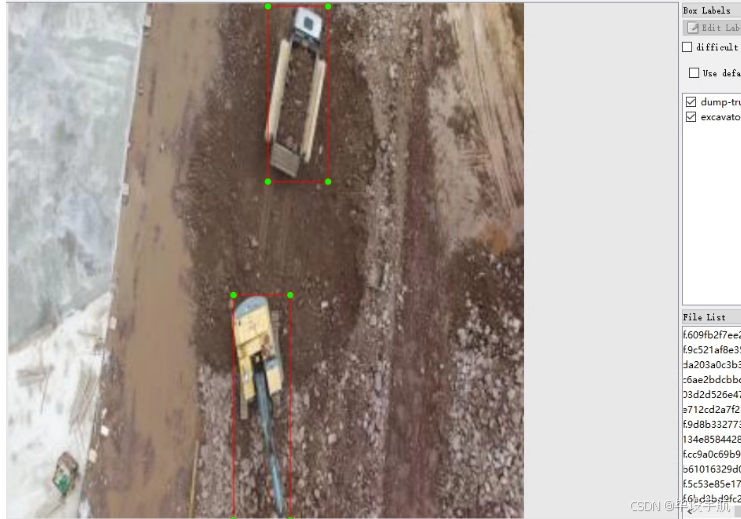
工程车辆识别数据集(Engineering Vehicle Recognition Dataset, EVRD)
摘要
EVRD 是一个专门针对航拍视角下的工程车辆识别而设计的数据集,旨在提供一种标准的训练和评估平台,用于开发和比较各种物体检测算法在工程车辆识别方面的性能。该数据集包含650张航拍图像,这些图像主要来源于建筑工地、道路施工区域和其他类似场景。每个图像都经过精心标注,标记出图像中存在的各种工程车辆的位置和类别。数据集总共覆盖了9个不同的工程车辆类别,包括推土机、混凝土搅拌车、混凝土泵车、自卸卡车、挖掘机、叉车、起重机、打桩机和压路机。数据集的目的是为了促进无人机自动识别和跟踪工程车辆的应用发展,例如在建筑工地的安全监管、设备调度等方面。
数据集特点
- 多样化的工程车辆类别:数据集包含9种常见工程车辆,包括推土机、混凝土搅拌车、混凝土泵车、自卸卡车、挖掘机、叉车、起重机、打桩机和压路机。
- 航拍视角:所有的图像都是从空中拍摄,模拟无人机视角,更符合实际应用场景的需求。
- 详尽的标注:每张图像都进行了精确的边界框标注,同时指明了车辆的具体类别。
- 合理的数据分布:各个类别的样本数量相对均衡,有利于训练和评估模型的泛化能力。
- 易于使用:数据集已经按照YOLO格式整理,可以直接用于训练和评估YOLO系列的目标检测模型。
- 真实场景:图像来自于真实的建筑工地和道路施工现场,具有较高的实用价值。
数据集构成
- 图像数量:总共有650张航拍图像。
- 类别数:9类
- 类别名称及对应数量 :
bulldozer
: 推土机 (65张)concrete-mixer
: 混凝土搅拌车 (136张)concrete-pump
: 混凝土泵车 (12张)dump-truck
: 自卸卡车 (291张)excavator
: 挖掘机 (690张)forklift
: 叉车 (10张)lifting-equipment
: 起重机 (32张)piling-machine
: 打桩机 (56张)roller
: 压路机 (6张)tower-crane
: 塔式起重机 (206张)
示例代码
以下是一个简单的Python脚本示例,用于加载数据集中的一对图像-标签对,并可视化其中的标注信息:
import os
import cv2
import numpy as np
import matplotlib.pyplot as plt
from matplotlib.patches import Rectangle
# 数据集目录路径
data_dir = 'path/to/evrd_dataset'
train_image_dir = os.path.join(data_dir, 'images/train')
train_label_dir = os.path.join(data_dir, 'labels/train')
# 选取一张训练图像及其对应标签
image_files = os.listdir(train_image_dir)
image_file = image_files[0] # 假设取第一张图
label_file = os.path.splitext(image_file)[0] + '.txt'
image_path = os.path.join(train_image_dir, image_file)
label_path = os.path.join(train_label_dir, label_file)
# 加载图像
image = cv2.imread(image_path, cv2.IMREAD_COLOR)
height, width, _ = image.shape
# 解析YOLO格式标签
def parse_yolo_label(label_path, image_width, image_height):
bboxes = []
with open(label_path, 'r') as f:
lines = f.readlines()
for line in lines:
class_id, x_center, y_center, box_width, box_height = map(float, line.strip().split())
x_min = int((x_center - box_width / 2) * image_width)
y_min = int((y_center - box_height / 2) * image_height)
box_width = int(box_width * image_width)
box_height = int(box_height * image_height)
bboxes.append((class_id, x_min, y_min, box_width, box_height))
return bboxes
# 解析标签
bboxes = parse_yolo_label(label_path, width, height)
# 可视化标注
fig, ax = plt.subplots(figsize=(10, 10))
ax.imshow(cv2.cvtColor(image, cv2.COLOR_BGR2RGB))
colors = ['red', 'blue', 'green', 'orange', 'purple', 'yellow', 'pink', 'brown', 'gray']
names = ['bulldozer', 'concrete-mixer', 'concrete-pump', 'dump-truck', 'excavator', 'forklift', 'lifting-equipment', 'piling-machine', 'roller', 'tower-crane']
for bbox, color_name in zip(bboxes, colors):
class_id, x, y, w, h = bbox
rect = Rectangle((x, y), w, h, linewidth=2, edgecolor=color_name, facecolor='none')
ax.add_patch(rect)
ax.text(x, y - 10, names[int(class_id)], color=color_name, fontsize=8)
plt.title('Engineering Vehicle Recognition Dataset')
plt.axis('off')
plt.show()
数据集使用指南
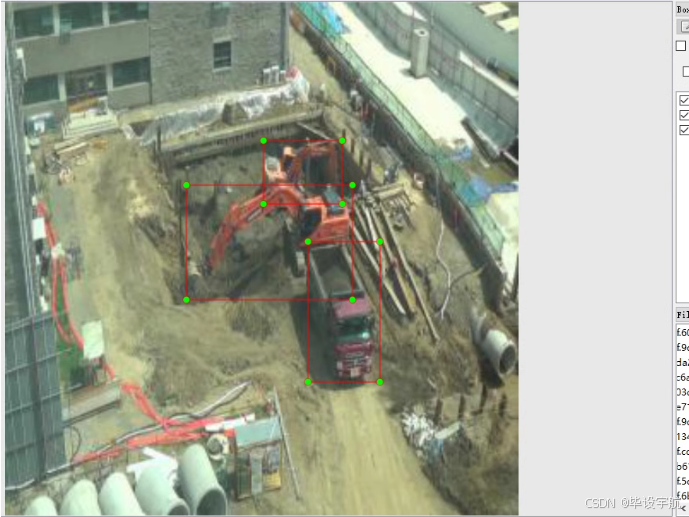
-
数据准备:
- 确认数据集路径是否正确,并且图像和标签文件均存在指定的目录下。
- 检查数据集是否有损坏或缺失的文件,确保所有图像和对应的标注文件都是完整的。
-
数据集划分:
- 数据集已经划分为训练集、验证集和测试集,可以直接使用。
-
配置文件:
-
根据所使用的深度学习框架创建相应的配置文件。对于YOLOv5等模型,通常需要一个
data.yaml
文件来描述数据集路径和类别信息。 -
data.yaml
示例内容如下:train: path/to/evrd_dataset/images/train val: path/to/evrd_dataset/images/validation test: path/to/evrd_dataset/images/test nc: 9 names: ['bulldozer', 'concrete-mixer', 'concrete-pump', 'dump-truck', 'excavator', 'forklift', 'lifting-equipment', 'piling-machine', 'roller', 'tower-crane']
-
-
模型训练:
- 选择适合任务的深度学习框架(如YOLOv5, YOLOv7, Detectron2等)。
- 配置训练参数,包括学习率、批次大小、迭代次数等。
- 使用提供的数据集开始训练模型。
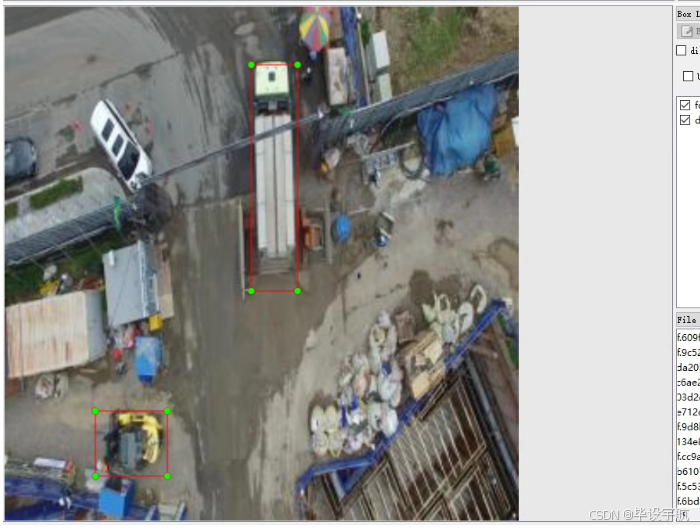