1 自然语言处理概述
- 语料:一个样本,句子/文章
- 语料库:由语料组成
- 词表:分词之后的词语去重保存成为词表
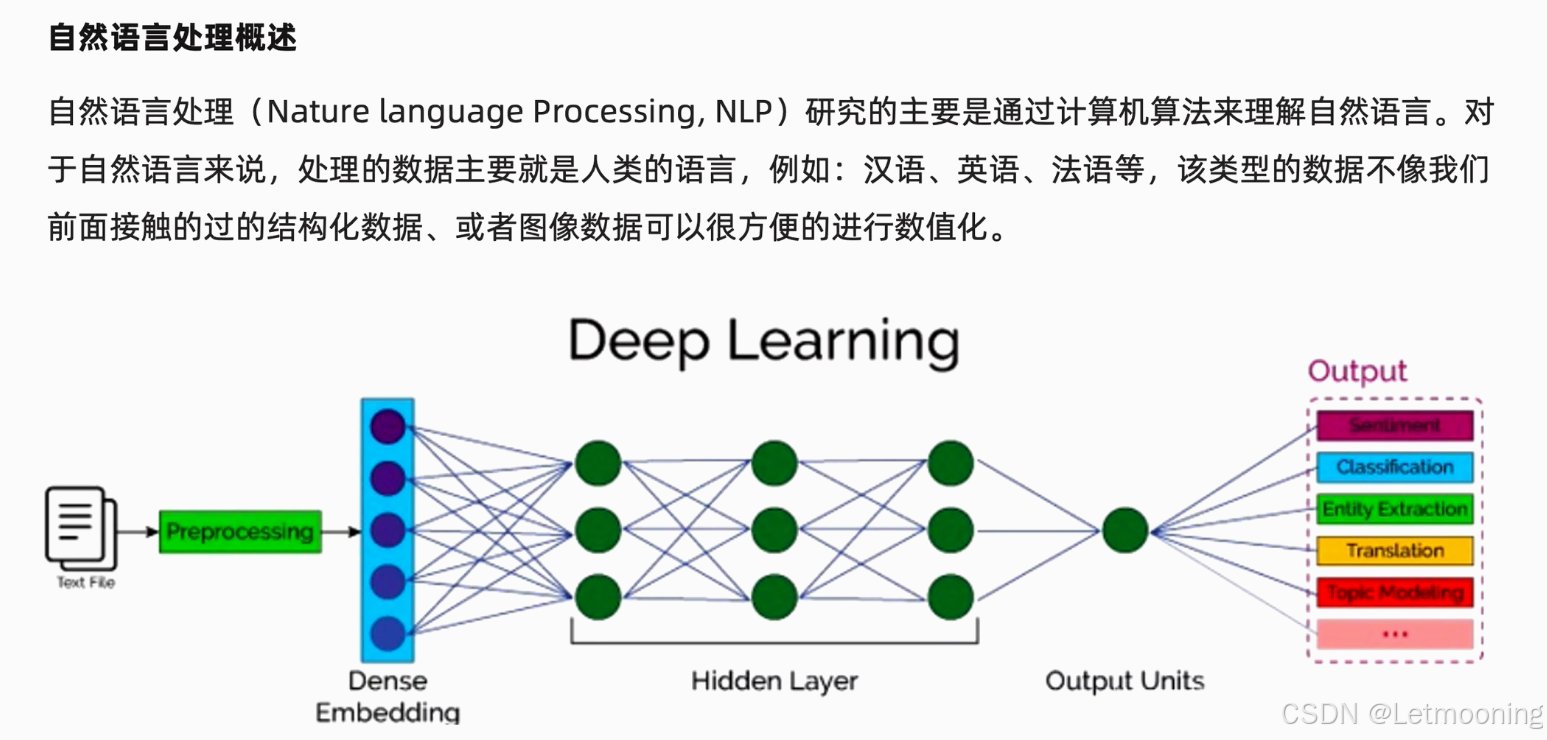
2 词嵌入层
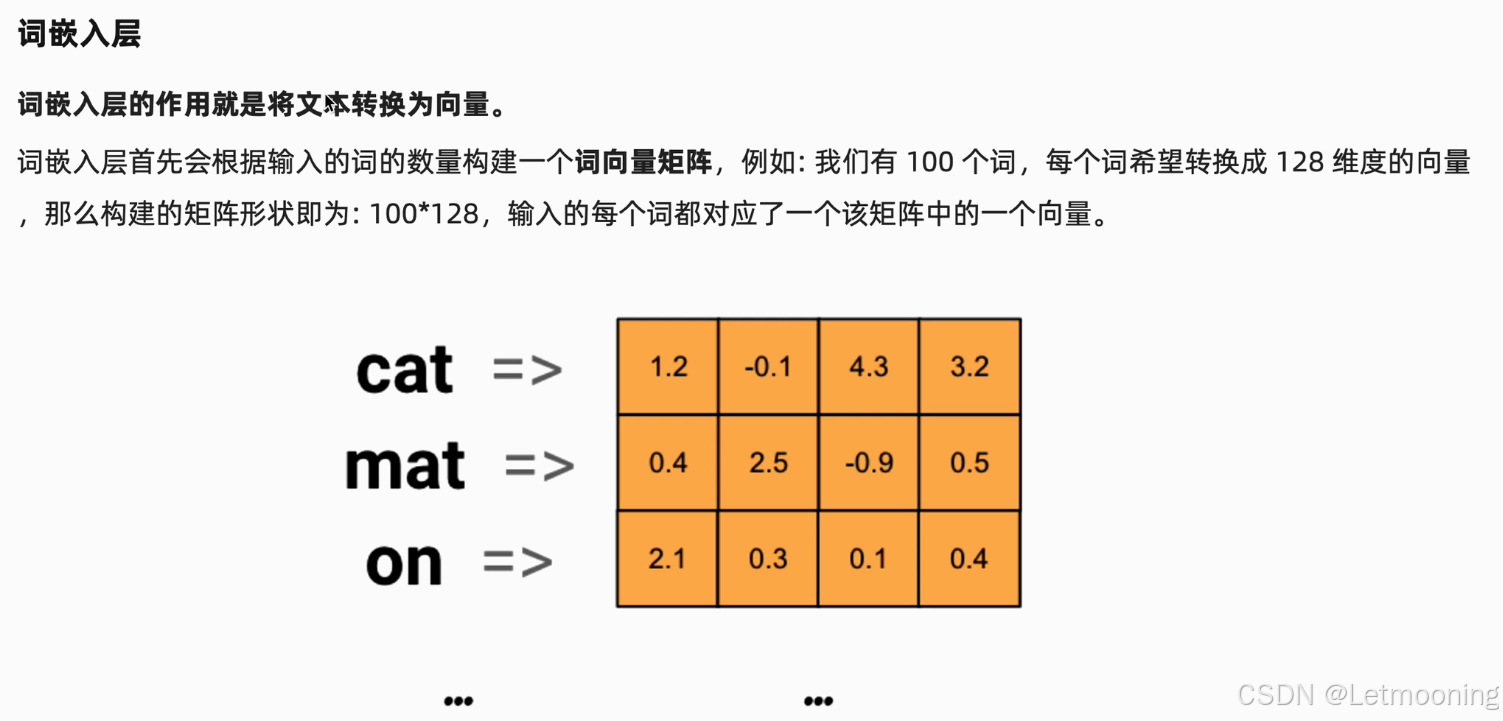
python
import jieba
import torch.nn as nn
import torch
# 文本数据
text='北京东奥的进度条已经过半,不少外国运动员在完成自己的比赛后踏上归途。'
# 分词
words=jieba.lcut(text)
print(words)
# 构建词表
uwords=list(set(words))
print(uwords)
words_num=len(uwords)
print(words_num)
# 构建词向量矩阵
embed=nn.Embedding(num_embeddings=words_num,embedding_dim=5)
print(embed(torch.tensor(1)))
# 输出结果
for i,word in enumerate(uwords):
print(word,end=' ')
print(embed(torch.tensor(i)))
bash
['北京', '东奥', '的', '进度条', '已经', '过半', ',', '不少', '外国', '运动员', '在', '完成', '自己', '的', '比赛', '后', '踏上', '归途', '。']
['自己', '运动员', '外国', '在', '后', '比赛', ',', '已经', '。', '过半', '不少', '进度条', '归途', '东奥', '踏上', '北京', '完成', '的']
18
tensor([-0.0293, -0.5446, -0.4495, -0.4013, -0.8653],
grad_fn=<EmbeddingBackward0>)
自己 tensor([-0.0907, -0.6044, 1.9097, 1.1630, -0.4595],
grad_fn=<EmbeddingBackward0>)
运动员 tensor([-0.0293, -0.5446, -0.4495, -0.4013, -0.8653],
grad_fn=<EmbeddingBackward0>)
外国 tensor([ 1.9382, -1.3591, -0.2884, -1.4880, -0.2400],
grad_fn=<EmbeddingBackward0>)
在 tensor([ 1.0954, 0.2975, -0.5151, -0.4355, 0.3870],
grad_fn=<EmbeddingBackward0>)
后 tensor([-0.1857, -0.4351, 0.3869, -0.6311, -1.5527],
grad_fn=<EmbeddingBackward0>)
比赛 tensor([-1.7570, -1.1983, -0.7864, 0.7223, -0.5285],
grad_fn=<EmbeddingBackward0>)
, tensor([-0.2706, 1.7983, 0.9599, -0.5464, 0.7365],
grad_fn=<EmbeddingBackward0>)
已经 tensor([ 1.4934, -0.7174, 1.1466, -0.3617, 0.6748],
grad_fn=<EmbeddingBackward0>)
。 tensor([ 0.7996, -0.5406, -0.6476, 0.3923, 0.5128],
grad_fn=<EmbeddingBackward0>)
过半 tensor([ 1.2070, 0.9933, 0.2634, 0.3173, -0.2273],
grad_fn=<EmbeddingBackward0>)
不少 tensor([ 0.6716, 1.6509, 0.7375, 0.7585, -0.6289],
grad_fn=<EmbeddingBackward0>)
进度条 tensor([ 0.4440, 1.9701, 0.6437, -0.2500, -0.8144],
grad_fn=<EmbeddingBackward0>)
归途 tensor([-0.5646, 0.8995, -0.5827, -1.0231, 1.3692],
grad_fn=<EmbeddingBackward0>)
东奥 tensor([-0.8312, 0.2083, 1.3728, 0.2860, 0.2762],
grad_fn=<EmbeddingBackward0>)
踏上 tensor([ 0.0955, 0.5528, -0.5286, 0.6969, -0.7469],
grad_fn=<EmbeddingBackward0>)
北京 tensor([ 0.4739, 0.6474, 0.3765, -1.9607, -1.1079],
grad_fn=<EmbeddingBackward0>)
完成 tensor([ 1.2215, -0.3468, -0.1432, 0.5908, 1.2294],
grad_fn=<EmbeddingBackward0>)
的 tensor([ 0.3083, 0.0163, 1.4923, -0.2768, 0.0904],
grad_fn=<EmbeddingBackward0>)
3 循环网络RNN
- 激活函数为tanh
- 隐藏状态:当前词前面的信息
-
batch,seqlen(句子长度),词向量维度
- pytorch框架的[seq_len,batch,input_size]
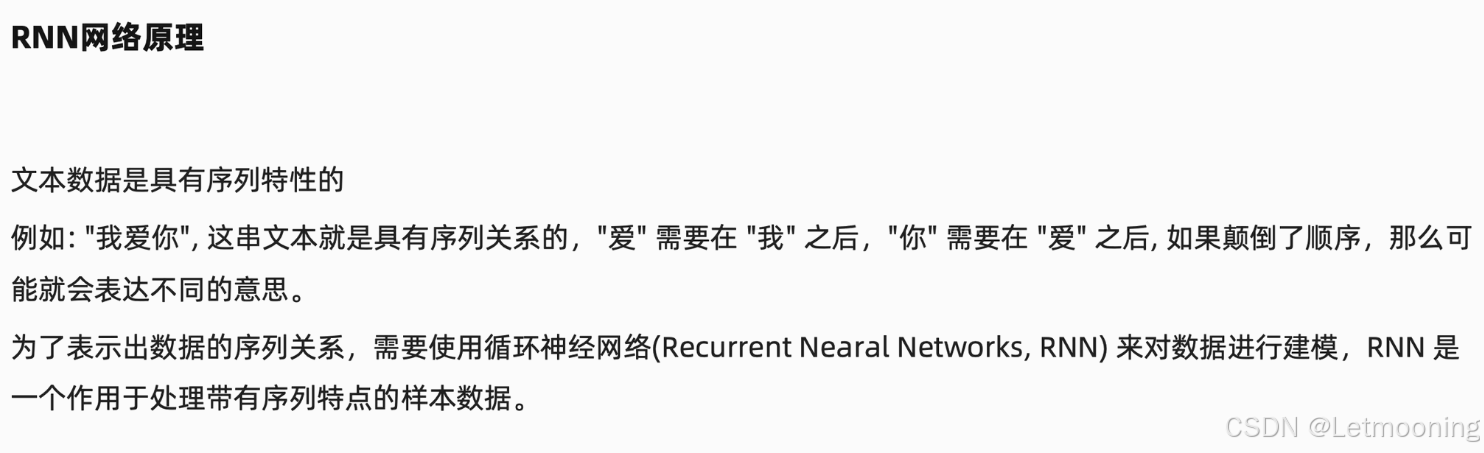
python
# RNN层API
import torch.nn as nn
import torch
# 词向量维度128,隐藏向量维度256
rnn=nn.RNN(input_size=128,hidden_size=256,num_layers=2)
# 第一个数字:seq_len,句子长度,也就是词语个数
# 第二个数字:batch,批量个数,也就是句子的个数
# 第三个数字:input_size,词向量的维度
# [seq_len,batch,input_size]
x=torch.randn([32,10,128])
# 第一个数字:num_layers,隐藏层的个数
# 第二个数字:batch,批量个数,也就是句子的个数
# 第三个数字:hidden_size,隐藏向量的维度
# [num_layers,batch,hidden_size]
h0=torch.zeros([2,10,256])
output,hn=rnn(x,h0)
# [seq_len,batch,hidden_size]
print(output.shape)
# [num_layers,batch,hidden_size]
print(hn.shape)
4 文本生成案例

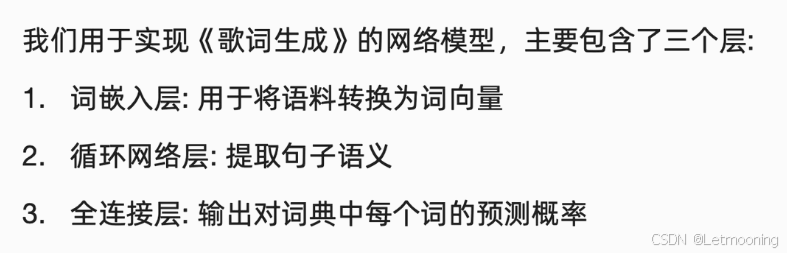
python
import jieba
# 构建词表
all_words = []
unique_words = []
for text in open('jaychou_lyrics.txt', 'r', encoding='utf8'):
words = jieba.lcut(text)
all_words.append(words)
for word in words:
if word not in unique_words:
unique_words.append(word)
word2idx = {word: idx for idx, word in enumerate(unique_words)}
# print(all_words)
# print(unique_words)
# print(word2idx)
print(len(unique_words))
corpus_ids = []
for words in all_words:
temp = []
for word in words:
temp.append(word2idx[word])
temp.append(word2idx[' '])
corpus_ids.extend(temp)
print(corpus_ids)
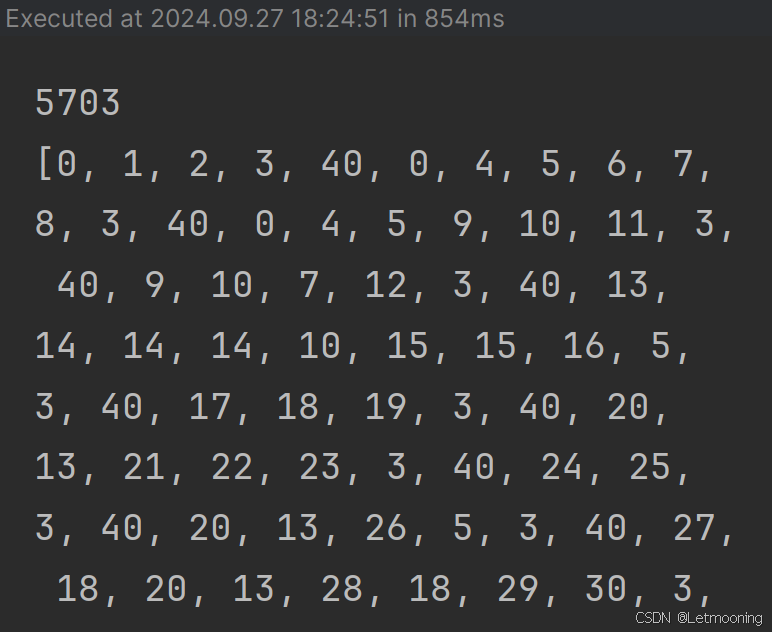
python
from torch.utils.data import Dataset
class textDataset(Dataset):
def __init__(self, corpus_ids, seq_len):
self.corpus_ids = corpus_ids
self.seq_len = seq_len
self.word_count = len(self.corpus_ids)
self.number = self.word_count // self.seq_len
def __len__(self):
return self.number
def __getitem__(self, idx):
# idx指词的索引,并将其修正索引到文档的范围里面
start = min(max(idx, 0), self.word_count - self.seq_len - 2)
x = self.corpus_ids[start:start + self.seq_len]
y = self.corpus_ids[start + 1:start + 1 + self.seq_len]
return torch.tensor(x), torch.tensor(y)
dataset = textDataset(corpus_ids, 5)
print(dataset.__getitem__(1))
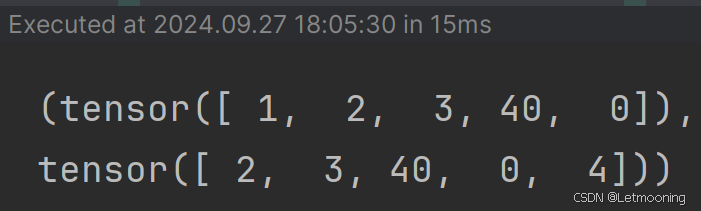
python
# 模型构建
from torch import nn
class textModel(nn.Module):
def __init__(self,word_count):
super().__init__()
self.emb=nn.Embedding(num_embeddings=word_count,embedding_dim=128)
self.rnn=nn.RNN(input_size=128,hidden_size=256,num_layers=1)
self.fc=nn.Linear(in_features=256,out_features=word_count)
def forward(self,x,h0):
# x 输入的词索引序列
embed=self.emb(x)
out,hn=self.rnn(embed.transpose(0,1),h0)
output=self.fc(out.reshape(-1,out.shape[-1]))
return output,hn
def init_hidden(self,bs):
'''
num_layer=1
bs=2
hidden_size=256
:return:
'''
return torch.zeros(1,bs,256)
python
# 模型训练
import torch.optim as optim
from torch.utils.data import DataLoader
word_count=len(unique_words)
model=textModel(word_count)
# 损失
error=nn.CrossEntropyLoss()
# 优化器
optimizer=optim.Adam(model.parameters(),lr=0.001,betas=[0.9,0.99])
epoches=2
for epoch in range(epoches):
dataloader=DataLoader(dataset=dataset,batch_size=2,shuffle=True)
loss_sum=0
num=0.1
for x,y in dataloader:
h0=model.init_hidden(len(y))
out,hn=model(x,h0)
# y=torch.transpose(y,0,1).reshape(-1)
y=torch.transpose(y,0,1).contiguous().view(-1)
loss=error(out,y)
loss_sum+=loss.item()
num+=1
optimizer.zero_grad()
loss.backward()
optimizer.step()
print(loss_sum/num)
torch.save(model.state_dict(),'model.pth')
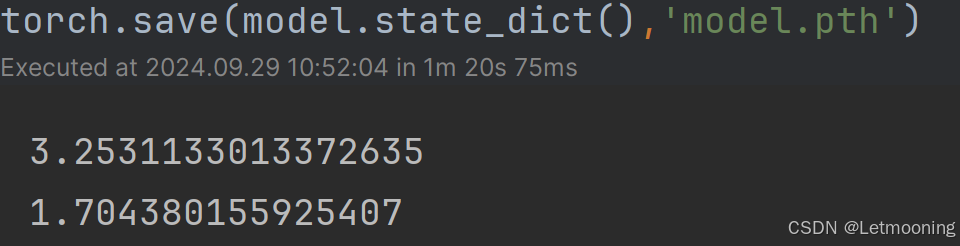
python
# 模型预测
model.load_state_dict(torch.load('model.pth',weights_only=True))
start_word='蝴蝶'
id=word2idx[start_word]
hn=model.init_hidden(bs=1)
idxs=[id]
for i in range(20):
out,hn=model(torch.tensor([[id]]),hn)
id=torch.argmax(out)
idxs.append(id.item())
print(idxs)
for idx in idxs:
print(unique_words[idx],end='')
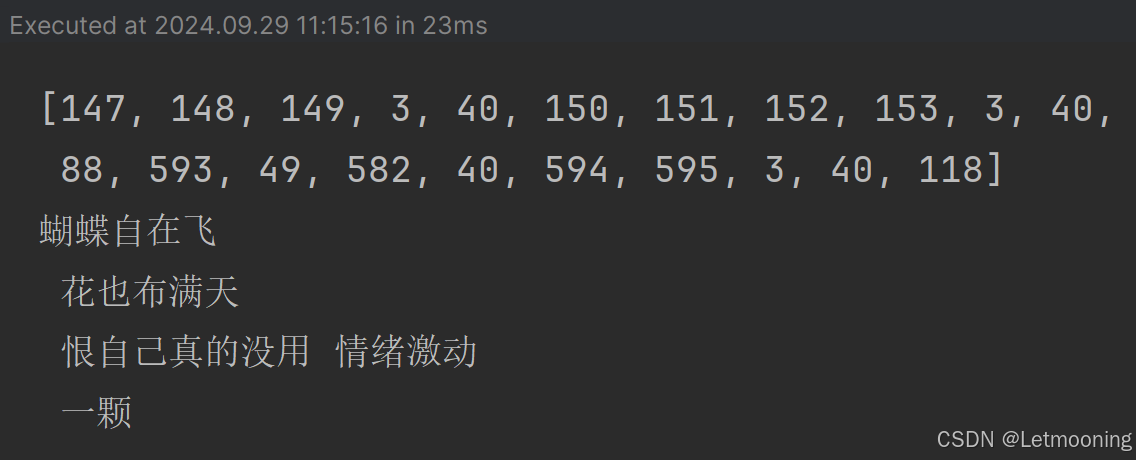