目录
[1. Deterministic Image Inpainting 判别器图像修复](#1. Deterministic Image Inpainting 判别器图像修复)
[1.1. sigle-shot framework](#1.1. sigle-shot framework)
[(1) Generators](#(1) Generators)
[(2) training objects / Loss Functions](#(2) training objects / Loss Functions)
[1.2. two-stage framework](#1.2. two-stage framework)
[2. Stochastic Image Inpainting 随机图像修复](#2. Stochastic Image Inpainting 随机图像修复)
[2.1. VAE-based methods](#2.1. VAE-based methods)
[2.2. GAN-based methods](#2.2. GAN-based methods)
[2.3. Flow-based methods](#2.3. Flow-based methods)
[2.4. MLM-based methods](#2.4. MLM-based methods)
[2.5. Diffusion model-based methods](#2.5. Diffusion model-based methods)
[3. text-guided image inpainting ⽂本引导的图像修复](#3. text-guided image inpainting ⽂本引导的图像修复)
[4. Inpainting Mask 掩码机制](#4. Inpainting Mask 掩码机制)
[(1) regular mask](#(1) regular mask)
[(2) irregular mask](#(2) irregular mask)
[5. Loss Function 损失函数](#5. Loss Function 损失函数)
[6. Dataset 图像修复领域数据集](#6. Dataset 图像修复领域数据集)
[(1) faces(CelebA & CelebA-HQ)](#(1) faces(CelebA & CelebA-HQ))
[(2) real-world encountered scenes(Places2)](#(2) real-world encountered scenes(Places2))
[(3) street scenes(Paris)](#(3) street scenes(Paris))
[(4) texture(DTD)](#(4) texture(DTD))
[(5) objects (ImageNet)](#(5) objects (ImageNet))
[7. Evaluation Protocol 评估指标](#7. Evaluation Protocol 评估指标)
[7.1. pixel-aware metrics](#7.1. pixel-aware metrics)
[7.2. (human) perception-aware metriics](#7.2. (human) perception-aware metriics)
[8. Performance Evaluation 表现评估](#8. Performance Evaluation 表现评估)
[8.1 Representative Image Inpainting Methods](#8.1 Representative Image Inpainting Methods)
[8.2 Loss Functions](#8.2 Loss Functions)
[9. Inpainting-based Application 基于图像修复的领域应⽤](#9. Inpainting-based Application 基于图像修复的领域应⽤)
[(1) Object Removal](#(1) Object Removal)
[(2) Text Editing](#(2) Text Editing)
[(3) Old Photo Restoration](#(3) Old Photo Restoration)
[(4) Image Compression](#(4) Image Compression)
[(5) Text-guided image editing](#(5) Text-guided image editing)
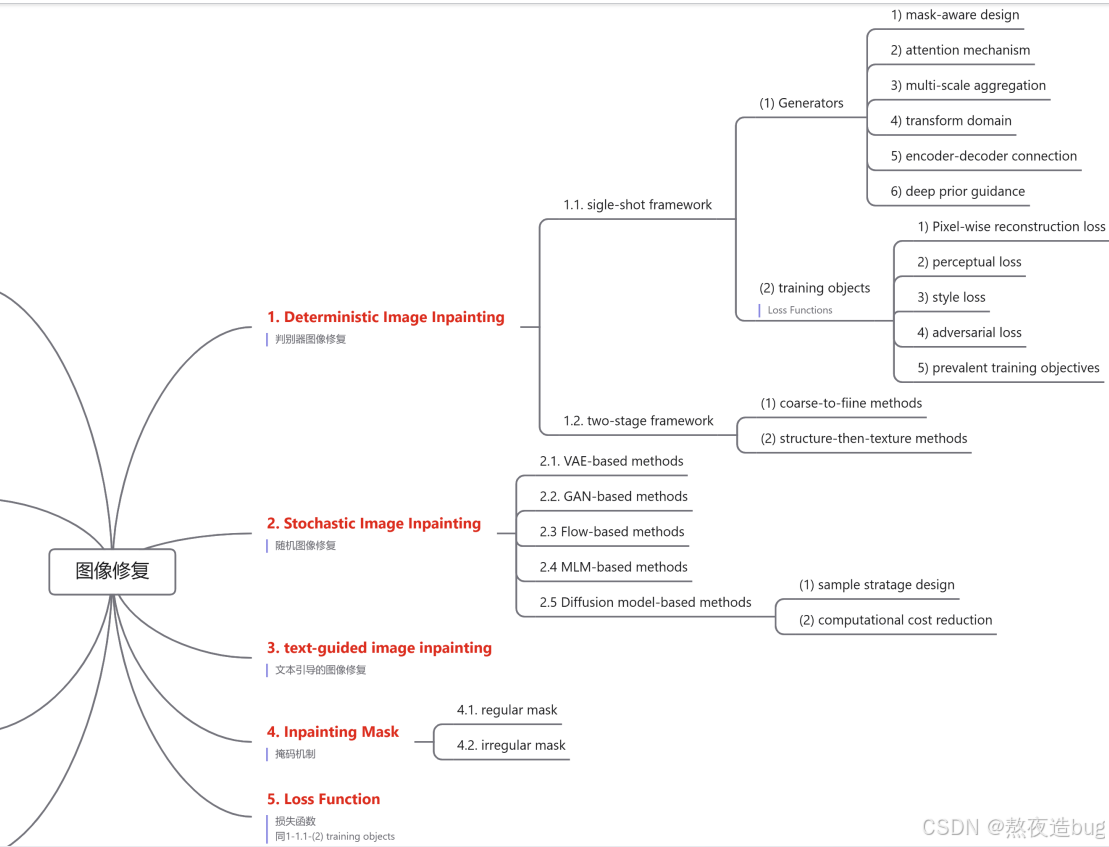
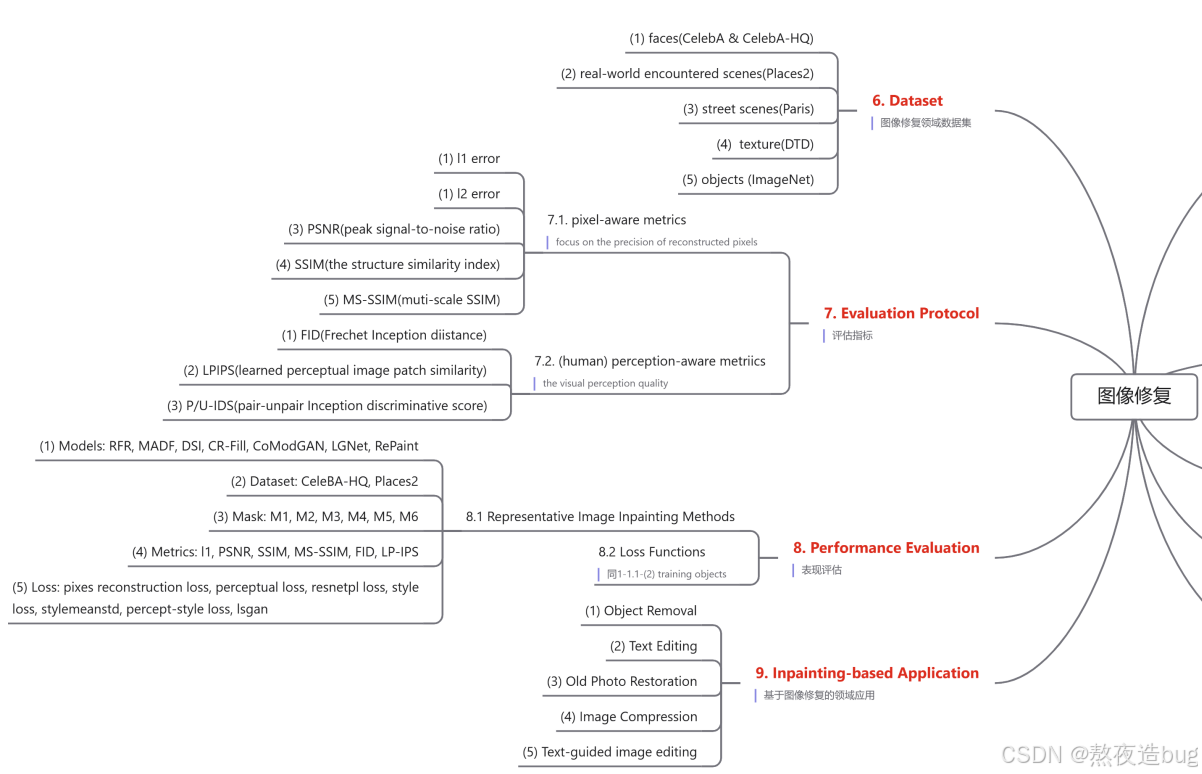
1. Deterministic Image Inpainting 判别器图像修复
1.1. sigle-shot framework
(1) Generators
- mask-aware design
- attention mechanism
- multi-scale aggregation
- transform domain
- encoder-decoder connection
- deep prior guidance
(2) training objects / Loss Functions
- Pixel-wise reconstruction loss
- perceptual loss
- style loss
- adversarial loss
- prevalent training objectives
1.2. two-stage framework
(1) coarse-to-fiine methods
(2) structure-then-texture methods
2. Stochastic Image Inpainting 随机图像修复
2.1. VAE-based methods
2.2. GAN-based methods
2.3. Flow-based methods
2.4. MLM-based methods
2.5. Diffusion model-based methods
(1) sample stratage design
(2) computational cost reduction
3. text-guided image inpainting ⽂本引导的图像修复
4. Inpainting Mask 掩码机制
(1) regular mask
(2) irregular mask
5. Loss Function 损失函数
同1-1.1-(2) training objects
6. Dataset 图像修复领域数据集
(1) faces(CelebA & CelebA-HQ)
(2) real-world encountered scenes(Places2)
(3) street scenes(Paris)
(4) texture(DTD)
(5) objects (ImageNet)
7. Evaluation Protocol 评估指标
7.1. pixel-aware metrics
focus on the precision of reconstructed pixels
(1) l1 error
(1) l2 error
(3) PSNR(peak signal-to-noise ratio)
(4) SSIM(the structure similarity index)
(5) MS-SSIM(muti-scale SSIM)
7.2. (human) perception-aware metriics
the visual perception quality
(1) FID(Frechet Inception diistance)
(2) LPIPS(learned perceptual image patch similarity)
(3) P/U-IDS(pair-unpair Inception discriminative score)
8. Performance Evaluation 表现评估
8.1 Representative Image Inpainting Methods
(1) Models: RFR, MADF, DSI, CR-Fill, CoModGAN, LGNet, RePaint
(2) Dataset: CeleBA-HQ, Places2
(3) Mask: M1, M2, M3, M4, M5, M6
(4) Metrics: l1, PSNR, SSIM, MS-SSIM, FID, LP-IPS
(5) Loss: pixes reconstruction loss, perceptual loss, resnetpl loss, style loss, stylemeanstd,
percept-style loss, lsgan
8.2 Loss Functions
同1-1.1-(2) training objects