Flink Windows 操作
上篇文章介绍了Flink 几种类型 Windows 本文介绍窗口操作相关API,以及各自使用场景 。
本期Flink Windows 相关操作apply/union/join/collect/CoMap/CoFlatMap
Windows apply
通过实现WindowFunction或AllWindowFunction接口来完成的,这些接口允许你定义如何对窗口内的数据进行处理。通过使用这些函数,你可以实现复杂的窗口计算逻辑
,满足各种业务需求。
例如对窗口内数值进行求和:
java
import org.apache.flink.api.java.tuple.Tuple2;
import org.apache.flink.streaming.api.datastream.DataStream;
import org.apache.flink.streaming.api.environment.StreamExecutionEnvironment;
import org.apache.flink.streaming.api.functions.source.RichParallelSourceFunction;
import org.apache.flink.streaming.api.functions.windowing.WindowFunction;
import org.apache.flink.streaming.api.windowing.assigners.TumblingProcessingTimeWindows;
import org.apache.flink.streaming.api.windowing.time.Time;
import org.apache.flink.streaming.api.windowing.windows.TimeWindow;
import org.apache.flink.util.Collector;
import java.util.Arrays;
import java.util.List;
import java.util.Random;
public class WindowDemo {
public static void main(String[] args) throws Exception {
final StreamExecutionEnvironment env = StreamExecutionEnvironment.getExecutionEnvironment();
DataStream<Tuple2<String, Integer>> input = ...;
DataStream<Tuple2<String, Integer>> result = input
.keyBy(f->f.f0) // 按第一个字段(假设是key)进行分组
.window(TumblingProcessingTimeWindows.of(Time.seconds(5))) // 定义一个10秒的时间窗口
.apply(new WindowFunction<Tuple2<String, Integer>, Tuple2<String, Integer>, String, TimeWindow>() {
@Override
public void apply(String key, TimeWindow window, Iterable<Tuple2<String, Integer>> values, Collector<Tuple2<String, Integer>> out) throws Exception {
int sum = 0;
for (Tuple2<String, Integer> value : values) {
sum += value.f1;
}
out.collect(new Tuple2<>(key, sum)); // 输出键和窗口内值的总和
}
});
result.print(); // 打印结果
env.execute();
}
}
Union
两个或多个数据流的联合会创建一个包含所有流中所有元素的新流。
注意事项
:使用Union操作符合并的数据流,其数据类型必须相同。
Union 操作,很方便实现对"多源数据"进行分析, 在实际应用中,数据可能来自多个不同的源,不同的系统的,不同的数据库。那么Union 就有用武之地。
当然也有在一些复杂的流处理场景中,可能会先将一个大的数据流根据某些条件分流成多个小的数据流进行独立处理,然后再将这些处理后的数据流通过Union操作符合并成一个数据流,以便进行进一步的汇总或分析。
以下是一个Union 使用示例代码
java
package com.codetonight.datastream.operater;
import com.codetonight.Example;
import org.apache.flink.api.common.functions.FilterFunction;
import org.apache.flink.streaming.api.datastream.DataStream;
import org.apache.flink.streaming.api.environment.StreamExecutionEnvironment;
public class UnionExample {
public static void main(String[] args) throws Exception {
final StreamExecutionEnvironment env =
StreamExecutionEnvironment.getExecutionEnvironment();
DataStream<Example.Person> flintstones1 = env.fromElements(
new Example.Person("Fred", 35),
new Example.Person("Wilma", 35),
new Example.Person("Pebbles", 2));
DataStream<Example.Person> flintstones2 = env.fromElements(
new Example.Person("Tom", 35),
new Example.Person("java", 35),
new Example.Person("CPlus", 2));
DataStream<Example.Person> flintstones = flintstones1.union(flintstones2);
DataStream<Example.Person> adults = flintstones.filter(new FilterFunction<Example.Person>() {
@Override
public boolean filter(Example.Person person) throws Exception {
return person.age >= 18;
}
});
adults.print();
env.execute();
}
public static class Person {
public String name;
public Integer age;
public Person() {}
public Person(String name, Integer age) {
this.name = name;
this.age = age;
}
public String toString() {
return this.name.toString() + ": age " + this.age.toString();
}
}
}
Window Join
它允许开发者在两个或多个数据流之间基于时间和/或键值进行联接操作。Window Join作用于两个流中有相同key且处于相同窗口的元素上
使用方式
java
dataStream.join(otherStream)
.where(<key selector>).equalTo(<key selector>)
.window(TumblingEventTimeWindows.of(Time.seconds(3)))
.apply (new JoinFunction () {...});
Interval Join
Interval Join
允许数据流(我们称之为"左流")去Join另一条数据流(我们称之为"右流")中前后一段时间内的数据。Interval Join
处理具有时间相关性的数据流时特别有用,比如在处理用户行为分析、交易日志等场景中。
下面代码展示 Interval Join
使用方式。
满足 ** key1 == key2 && leftTs - 2 < rightTs < leftTs + 2
**,
Interval Join的使用场景包括但不限于:
用户行为分析:例如,分析用户浏览行为
与交易行为
之间关系
java
// this will join the two streams so that
// key1 == key2 && leftTs - 2 < rightTs < leftTs + 2
keyedStream.intervalJoin(otherKeyedStream)
.between(Time.milliseconds(-2), Time.milliseconds(2)) // lower and upper bound
.upperBoundExclusive(true) // optional
.lowerBoundExclusive(true) // optional
.process(new ProcessJoinFunction{...});
coGroup
Window CoGroup是将两个数据流按照指定的key和窗口进行分组,并在每个窗口结束时将两个流中相同key的数据合并在一起。这种操作通常用于需要同时对两个流中的数据进行聚合、比较或关联的场景
Window CoGroup适用于需要对两个流中的数据进行复杂关联或聚合操作的场景,如:
实时用户行为分析:将用户的浏览行为和点击行为数据合并,分析用户的兴趣偏好。
用户可以在CoGroupFunction中实现自定义的处理逻辑,如聚合、过滤、比较等操作
java
dataStream.coGroup(otherStream)
.where(0).equalTo(1)
.window(TumblingEventTimeWindows.of(Time.seconds(3)))
.apply (new CoGroupFunction () {...});
java
@Public
@FunctionalInterface
public interface CoGroupFunction<IN1, IN2, O> extends Function, Serializable {
/**
* This method must be implemented to provide a user implementation of a coGroup. It is called
* for each pair of element groups where the elements share the same key.
*
* @param first The records from the first input.
* @param second The records from the second.
* @param out A collector to return elements.
* @throws Exception The function may throw Exceptions, which will cause the program to cancel,
* and may trigger the recovery logic.
*/
void coGroup(Iterable<IN1> first, Iterable<IN2> second, Collector<O> out) throws Exception;
}
Connect
它允许开发者将两个DataStream(数据流)连接起来,以便后续可以对这两个流中的数据进行统一处理。与Union操作不同,Connect操作不要求两个流的数据类型必须相同。
两个流之间可以共享状态,这在处理复杂的数据流逻辑时非常有用
dataStream.connect 操作会返回一个 ConnectedStreams
java
ConnectedStreams collectStream = dataStream.connect(otherStream);
CoMap, CoFlatMap
CoMap
和CoFlatMap
将不同类型的数据流进行组合和转换以生成新的数据流
ConnectedStreams → DataStream
下面例子CoMap使用例子。
-
将
Interger数据流
与String 数据流
进行connect
操作 得到connectedStreams -
将connectedStreams 通过CoMapFunction 转换为一个String 数据流
-
CoMapFunction map1 对原
Interger数据流
中元素 乘2 后再转String -
CoMapFunction map2 对原
String数据流
中元素转为大写
java
public class CoMapExample {
public static void main(String[] args) throws Exception {
// 设置执行环境
StreamExecutionEnvironment env = StreamExecutionEnvironment.getExecutionEnvironment();
// 创建两个不同类型的数据流
DataStream<Integer> stream1 = env.fromElements(1, 2, 3);
DataStream<String> stream2 = env.fromElements("a", "b", "c");
// 连接两个数据流
ConnectedStreams<Integer, String> connectedStreams = stream1.connect(stream2);
// 使用 CoMap 进行转换操作
DataStream<String> resultStream = connectedStreams.map(new CoMapFunction<Integer, String, String>() {
@Override
public String map1(Integer value) {
return String.valueOf(value * 2);
}
@Override
public String map2(String value) {
return value.toUpperCase();
}
});
// 打印结果
resultStream.print();
// 执行任务
env.execute();
}
}
CoFlatMap
读者参考上篇文章FlatMap 类比学习。CoFlatMap
可以完成CoMap 功能,前面例子稍作修改。读者根据输出结果自行体会CoFlatMap 与 Map 区别。
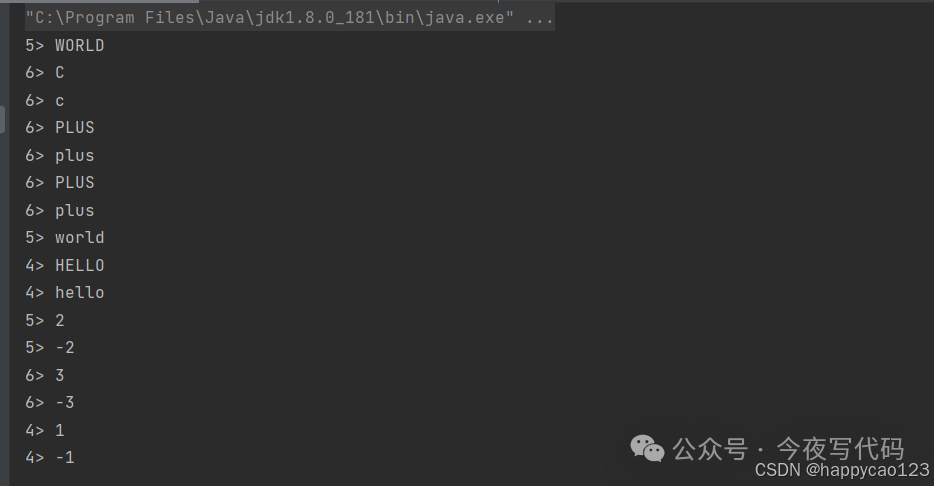
总结
本期Flink Windows 相关操作apply/union/join/collect/CoMap/CoFlatMap 。介
绍了各自的使用场景,并给出简单的例子。关于Flink 操作还有很多,期待我们一起持续学习。