目录
[Isaac Sim介绍](#Isaac Sim介绍)
[OMNI GYM环境编写](#OMNI GYM环境编写)
Isaac Sim介绍
Isaac Sim是英伟达出的一款机器人仿真平台,适用于做机器人仿真。同类产品包括mujoco,vrep和pybullt等,它的主要优势就是可以做并行强化学习仿真,这对于提高训练效率是非常有好处的。
作者使用的版本是 :ISAAC SIM 2023.1,因为isaac sim每次版本的迭代变化都很大,而isaac 4.0 又是大更改,因此我还是在2023.1版本上进行测试的。
之前使用isaac sim没有进行并行化训练,原因就是如果想用并行化训练单纯使用isaac sim是搞不出来的,还要搭配另外的环境,例如2023.1就要使用**OmniIsaacGymEnvs,**或者ORbit。如果是4.0的用户就是使用isaac lab了。
OmniIssacGymEnvs安装
IsaacGymEnvs的安装非常简单,按照官方仓库readme安装即可
GitHub - isaac-sim/OmniIsaacGymEnvs: Reinforcement Learning Environments for Omniverse Isaac Gym
OmniIsaacGymEnvs 提供了很多经典的强化学习训练场景,最典型的就是Cartpole环境了。
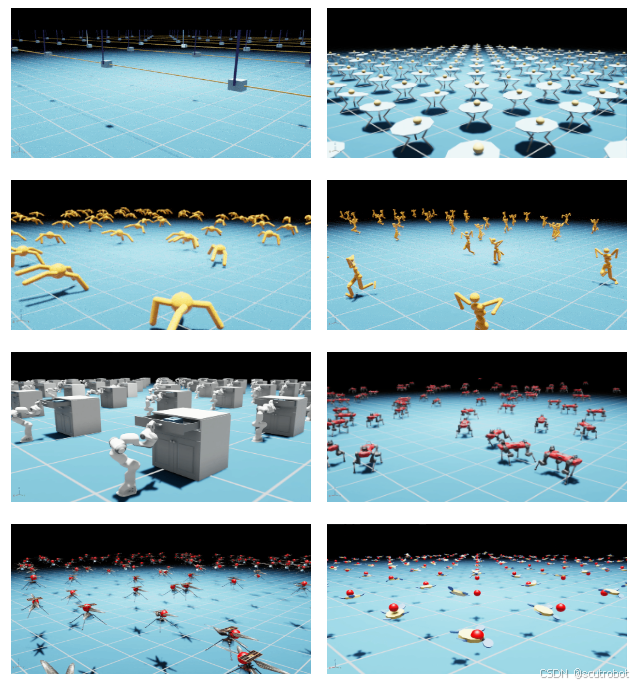
安装步骤:
-
git clone https://github.com/NVIDIA-Omniverse/OmniIsaacGymEnvs.git 下载仓库
-
将默认执行的python设置为Isaac sim环境执行的python
For Linux: alias PYTHON_PATH=~/.local/share/ov/pkg/isaac_sim-/python.sh
For Windows: doskey PYTHON_PATH=C:\Users\user\AppData\Local\ov\pkg\isaac_sim-\python.bat $*
For IsaacSim Docker: alias PYTHON_PATH=/isaac-sim/python.sh
3.安装 omniisaacgymenvs
模块
PYTHON_PATH -m pip install -e .
按照官方的指示,这样就可以把仓库安装好了,然后执行,就可以测试官方给的例程。
PYTHON_PATH scripts/rlgames_train.py task=Cartpole
但是注意到,这里用的是rlgames作为强化学习的库,这并不是一个常见的库,实际上英伟达自己在论坛上在推行一个叫做SKRL的库。
SKRL安装与测试
SKRL网址:Examples - skrl (1.3.0)
SKRL是英伟达自己推荐的一个强化学习库,它的优势在于可以无缝衔接英伟达自己的并行仿真环境,虽然说训练效果可能不如SB3好,但是它适配了啊。并且在使用多智能体的时候训练速度也是挺快的。
pip install skrl["torch"]
SKRL的安装按照官方的教程直接装就可以了。
这里需要特别注意的是OIGE的配置文件和rlgame是不一样的,具体可以参考官方给出的example,在yaml文件中要做一些修改。
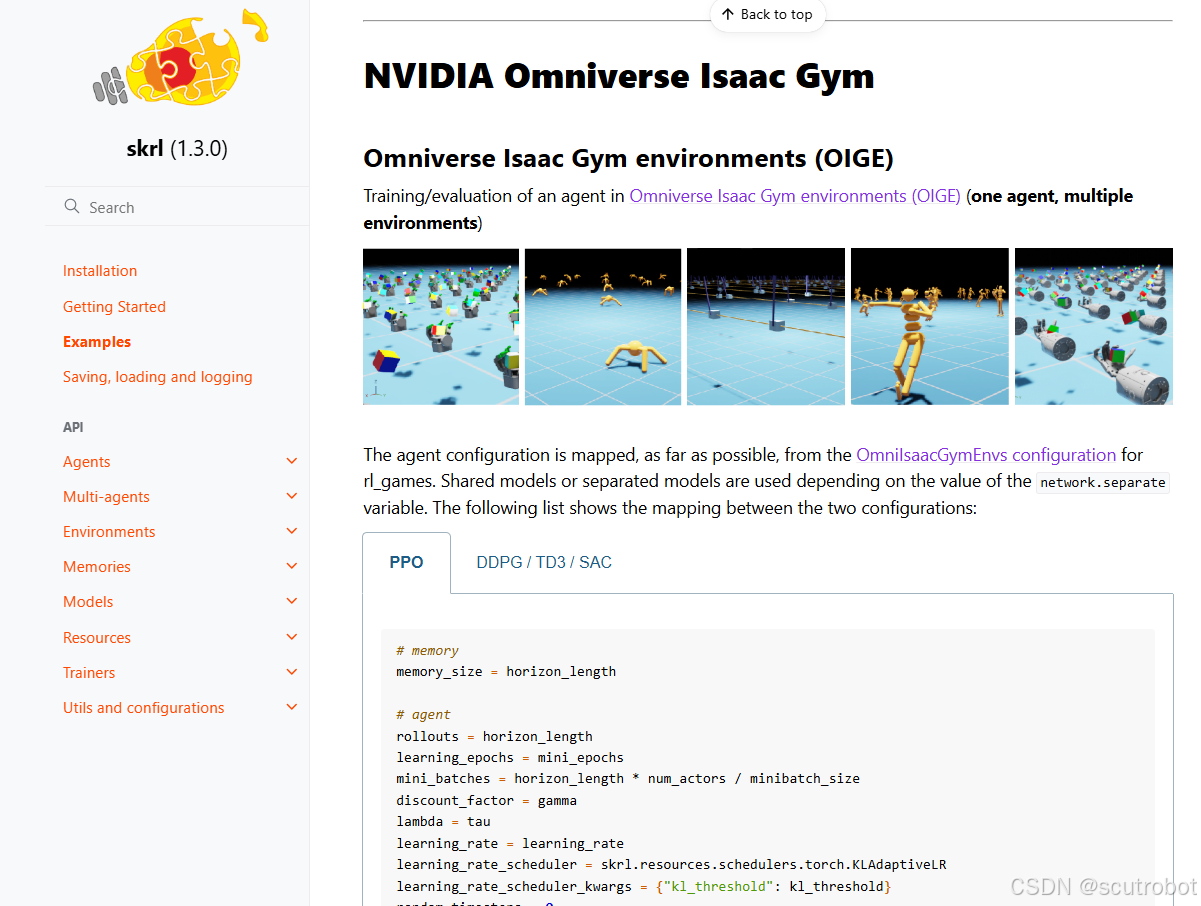
把skrl官方提供的yaml文件下载下来,并使用它给出的python文件运行,就可以将官方给的demo跑起来了。
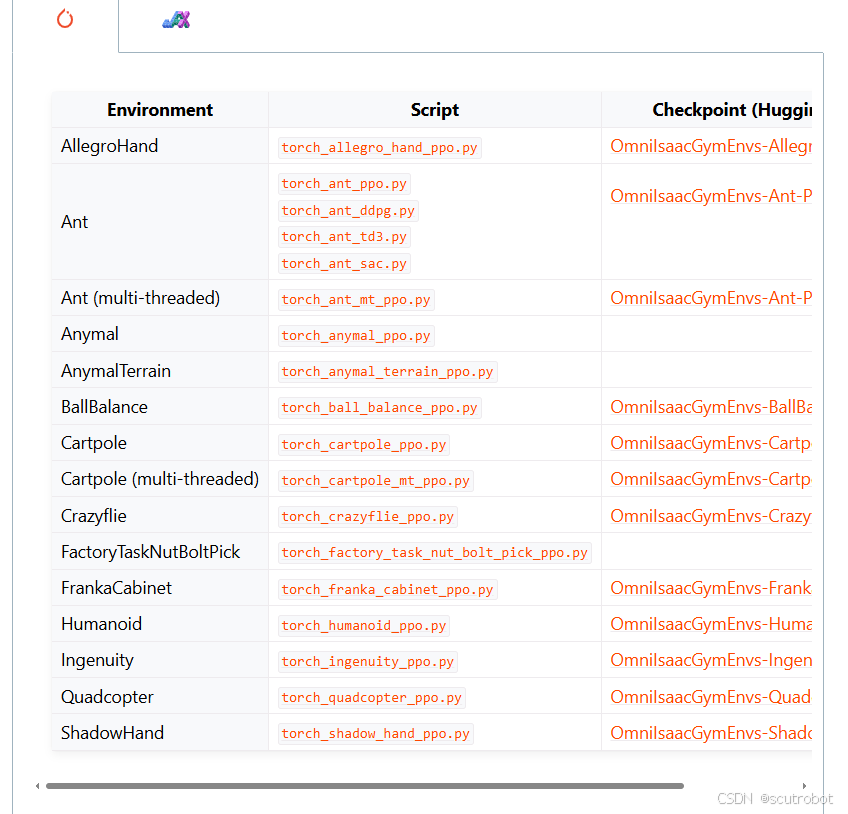
这里作者使用的GPU是4060TI 16G的版本
在环境中设置了4096个agent,运行起来还是非常顺畅的,训练了1600个回合,只花了1分钟左右

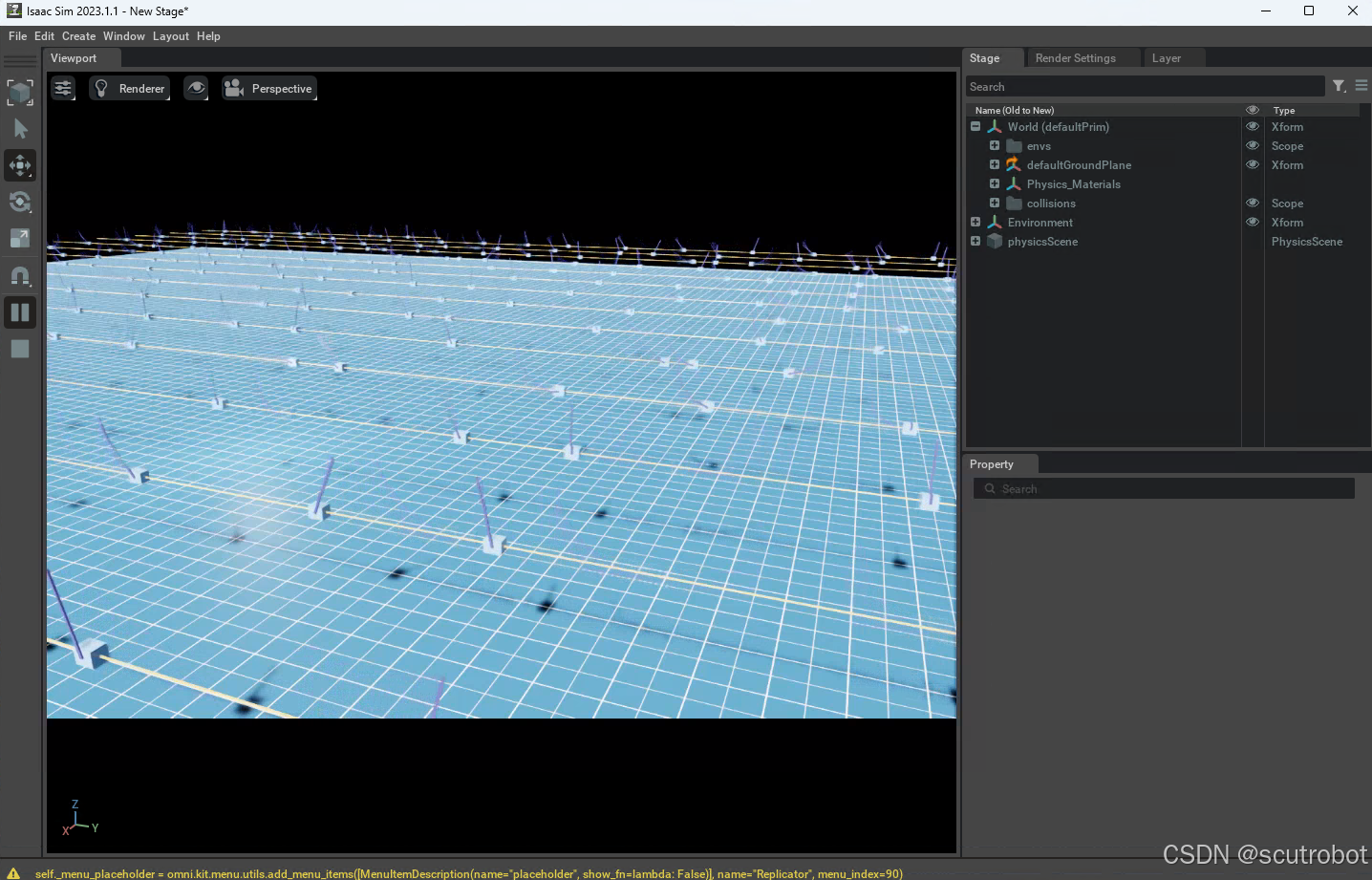
另外,官方提供了headless可选性,当headless设置成True时,就不会显示界面,这时候运行速度会更加快,1600个回合只需要15秒钟不到的时间即可完成。

可以看到,SKRL与omni isaac gym的衔接还是比较OK的,至少官方给出的例程运行起来是没什么问题的。
基于UR5的机械臂Reach强化学习测评
机器人控制
在测试完官方给出的环境后,肯定是希望可以测试下自己的环境。作者自己使用的是UR5机械臂,isaac sim中本身已经提供了这一款机械臂了,所以模型直接下载下来就可以,是usd格式的模型。
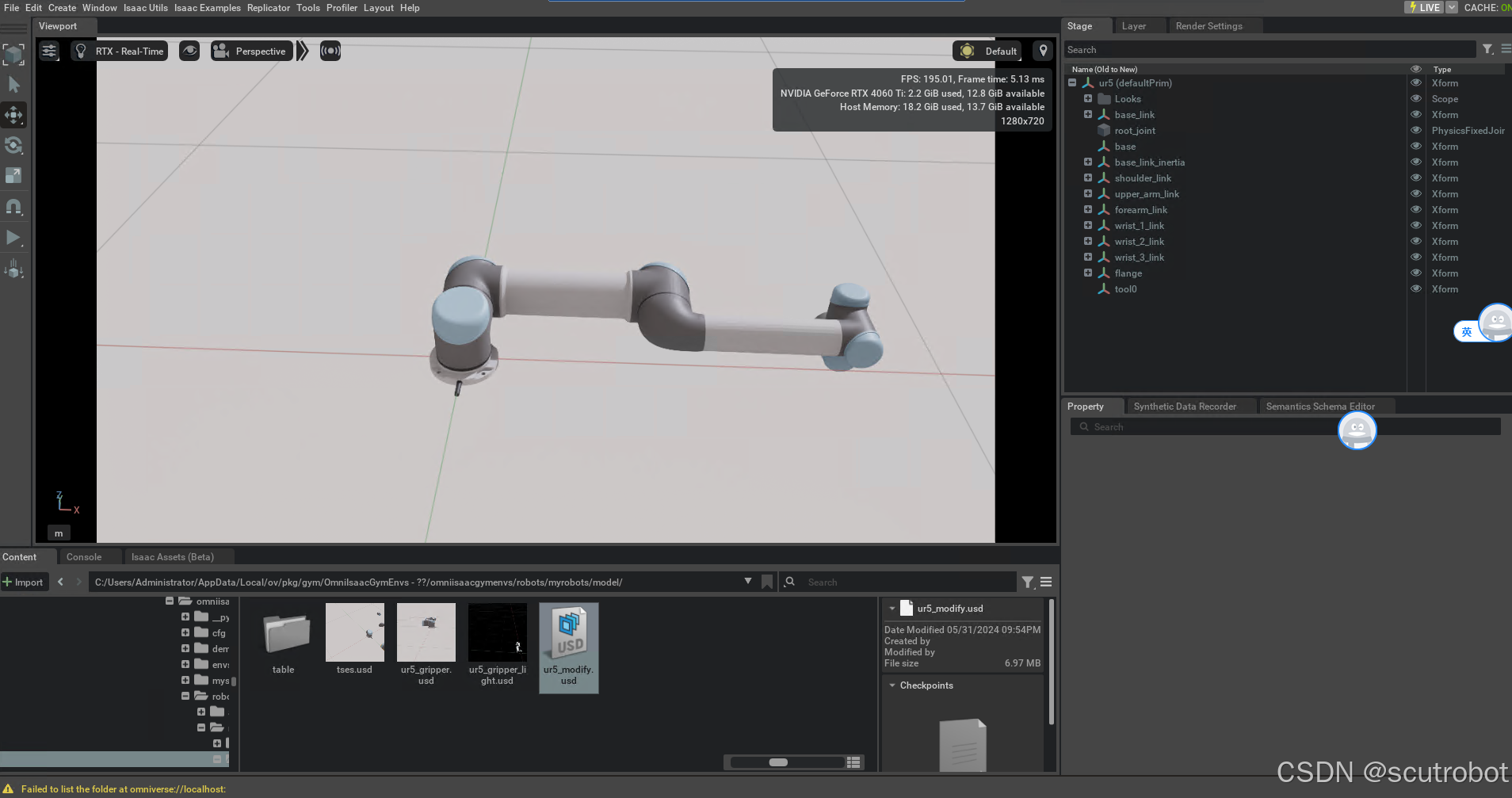
在机器人控制方面,官方提供的是RMPFLOW的轨迹规划库,但是RMPFLOW本身要配置很多东西,官方只提供了UR10的配置文件,因此这里我选用了最简单的控制方法。在网上下载了UR5的urdf文件,然后使用ikpy函数库读取urdf文件,并进行逆运动学求解,把求解出来的关节角度再下发到模型中。这里提供我写的UR5函数控制类作为参考:
python
from typing import Optional
import carb
import numpy as np
import torch
from omni.isaac.core.robots.robot import Robot
from omni.isaac.core.utils.nucleus import get_assets_root_path
from omni.isaac.core.utils.stage import add_reference_to_stage
from omni.isaac.core.articulations import ArticulationView,Articulation
from omni.isaac.core.utils.types import ArticulationAction, ArticulationActions
from common import MatrixProcess as mp
from omni.isaac.core.prims import RigidPrim, RigidPrimView,XFormPrimView
import common.robot.Urik as urik
from common.robot.Ur5ik import Kinematic
import logging
class UR5(Robot):
def __init__(
self,
prim_path: str,
ik_urdfPath:str,
name: Optional[str] = "UR5",
tcpOffset_pose=np.array([0,0,0]),
tcpOffset_ori=np.array([1,0,0,0]),
usd_path: Optional[str] = None,
translation: Optional[np.ndarray] = None,
orientation: Optional[np.ndarray] = None,
) -> None:
self._usd_path = usd_path
self._name = name
if self._usd_path is None:
self._usd_path = "C:\\Users\\Administrator\\AppData\\Local\\ov\\pkg\\gym\\OmniIsaacGymEnvs\\omniisaacgymenvs\\robots\\myrobots\\model\\ur5_modify.usd"
print("=== _usd_path=", self._usd_path)
add_reference_to_stage(self._usd_path, prim_path)
super().__init__(
prim_path=prim_path,
name=name,
translation=translation,
orientation=orientation,
articulation_controller=None,
)
self.robot_position=torch.tensor([translation[0],translation[1],translation[2]]).to("cuda")
self.tcpoffset_pose = tcpOffset_pose
self.tcpOffset_quaternion = tcpOffset_ori
self._iksolver = Kinematic()
def initView(self):
self._ur5_view=ArticulationView(
prim_paths_expr="/World/envs/.*/UR5", name="ur5_view", reset_xform_properties=False
)
self._ur5_ee_view=RigidPrimView(
prim_paths_expr="/World/envs/.*/UR5/tool0", name="ur5_ee", reset_xform_properties=False
)
def get_view(self):
return self._ur5_view
def get_joints(self):
joints=self._ur5_view.get_joint_positions()
#print("joint",np.round(joints.cpu().numpy(),2))
return joints
def get_TCP_pose(self,isworld):
pose,rot=self._ur5_ee_view.get_local_poses()#获取机器人坐标系下的坐标,xyzw?
if isworld==True:
pose=pose+self.robot_position# 加上机器人坐标系距离原点的位移
#print("pose",np.round(pose.cpu().numpy(),4))
return pose,rot
def set_joints(self,Joints6D,indices):
self._ur5_view.set_joint_positions(Joints6D, indices=indices)
def apply_joints(self,Joints6D,indices):
joints = ArticulationActions(Joints6D)
self._ur5_view.apply_action(joints,indices=indices)
def set_pose(self, position, oriention,desire_joints, apply=False, isworldpose=True):
"""
apply=True时,使用applay action
isworldpose=True时,转化至世界坐标系
"""
position=position.cpu().numpy()
oriention=oriention.cpu().numpy()
desire_joints=desire_joints.cpu().numpy()
robot_position=self.robot_position.cpu().numpy()
if (isworldpose == True):
position = position - robot_position
####根据tcp坐标,反求末端坐标,然后求解ik
Tbase_tcp = position
Qbase_tcp = mp.qua_wxyz2xyzw_array(oriention) # xyzw
Tend_tcp = self.tcpoffset_pose
Qend_tcp = mp.qua_wxyz2xyzw(self.tcpOffset_quaternion)
# 计算末端姿态
Q_TCP_inv = mp.quaternion_conjugate(Qend_tcp)
Q_end = mp.quaternion_multiply_array(Qbase_tcp, Q_TCP_inv)
# 计算末端位置
T_TCP_transformed = mp.rotate_vectors_array(Qbase_tcp, Tend_tcp)
T_end = Tbase_tcp - T_TCP_transformed
position = T_end
oriention = mp.qua_xyzw2wxyz_array(Q_end)
####
# 1,根据pose6d 计算IK joint
result=self.get_iks(position,oriention,desire_joints)
joints=torch.tensor(result).float().to("cuda")
if (apply == False):
self._ur5_view.set_joint_positions(joints)
else:
joints = ArticulationAction(joints)
self._ur5_view.apply_action(joints)
def get_iks(self, positions, orientions,q_desires):
len=positions.shape[0]
joints=[]
for i in range(len):
position=positions[i]
oriention=orientions[i]
q_desire=q_desires[i]
joint=self.get_ik(position,oriention,q_desire)
joints.append(joint)
#print(np.round(joint,2))
return np.array(joints)
def get_ik(self, pose, ori,q_desire):
"""
输入机器人末端的目标位置,计算逆运动学关节,返回计算用于apply action的ArticulationAction
Args:
pose: 目标位置
ori: 目标方向
initjoint: 用于求逆运动学的初始关节
Returns:
计算用于apply action的ArticulationAction
"""
try:
joint=self._iksolver.inverse_kinematic_Q(pose=pose,ori=ori,q_desire=q_desire)
return joint
except:
return q_desire
OMNI GYM环境编写
以及自己写的reach gym环境。这个环境编写的教程现在官方的手册是看不到的,如果你还是跟我一样使用2023.1版本,那么你可以看下旧版本的手册是如何教你写这个的。
python
import logging
import math
import random
import numpy as np
import torch
from omni.isaac.core.articulations import ArticulationView
from omni.isaac.core.utils.prims import get_prim_at_path
from omniisaacgymenvs.tasks.base.rl_task import RLTask
from omniisaacgymenvs.robots.articulations.cartpole import Cartpole
from omniisaacgymenvs.robots.myrobots.ur5 import UR5
from omni.isaac.core.utils.stage import add_reference_to_stage
from omni.isaac.core.prims import RigidPrim, RigidPrimView,XFormPrimView
class Ur5_InsertTask(RLTask):
def __init__(self, name, sim_config, env, offset=None) -> None:
self.update_config(sim_config)
self._max_episode_length = 150
self._num_observations = 3
self._num_actions = 3
self._reset_pose=torch.tensor(np.array([0, math.radians(-90), math.radians(-90), math.radians(-90), math.radians(90), math.radians(0)]), dtype=torch.float32).to("cuda")
RLTask.__init__(self, name, env)
def update_config(self, sim_config):
# extract task config from main config dictionary
self._sim_config = sim_config
self._cfg = sim_config.config
self._task_cfg = sim_config.task_config
# parse task config parameters
self._num_envs = self._task_cfg["env"]["numEnvs"]
self._env_spacing = self._task_cfg["env"]["envSpacing"]
self._cartpole_positions = torch.tensor([0.0, 0.0, 0.65])
def set_up_scene(self, scene) -> None:
# first create a single environment
self.get_ur5()
self.get_table()
self.get_cube()
super().set_up_scene(scene)
self.ur5.initView()
scene.add(self.ur5.get_view())
self._cubes = XFormPrimView(
prim_paths_expr="/World/envs/.*/prop/.*", name="prop_view", reset_xform_properties=False
)
scene.add(self._cubes)
def pre_physics_step(self, actions) -> None:
if not self.world.is_playing():
return
reset_env_ids = self.reset_buf.nonzero(as_tuple=False).squeeze(-1)#只获取没有复位的环境
if len(reset_env_ids) > 0:
self.reset_idx(reset_env_ids)
#TODO:复位的环境动作清零
#获取当前位置
joints = self.ur5.get_joints()
self.cube_pos, self.cube_rot = self._cubes.get_local_poses()
self.tcp_pos,self.tcp_rot=self.ur5.get_TCP_pose(isworld=True)
#设置动作增量
target=self.tcp_pos+actions*0.05
#执行动作
self.ur5.set_pose(target, self.cube_rot, joints, apply=True)
#self.ur5.set_pose(self.cube_pos, self.cube_rot, joints, apply=True)
def get_observations(self) -> dict:
cube_pos, cube_rot = self._cubes.get_local_poses()
self.tcp_pos,self.tcp_rot=self.ur5.get_TCP_pose(isworld=True)
#计算与目标的误差,作为关节角度
pos_error= cube_pos - self.tcp_pos
pos_error=torch.clip_(pos_error,-1,1)
self.obs_buf[:,0] = pos_error[:,0]
self.obs_buf[:,1] = pos_error[:,1]
self.obs_buf[:,2] = pos_error[:,2]
observations = {self.ur5.get_view().name: {"obs_buf": self.obs_buf}}
return observations
def calculate_metrics(self) -> None:
# assign rewards to the reward buffer
distances=torch.norm(self.cube_pos-self.tcp_pos,dim=1)
#reward=torch.where(distances<0.002,50,0)
#reward = torch.where(distances >= 0.002, -distances, reward)
#reward = torch.where(distances >0.1, -50, reward)
#reward=torch.where(distances >= 0.002, -distances, 100)
#reward=-distances
# reward = torch.where(distances < 0.01, 1, -distances)
# reward = torch.where(distances < 0.002, 100, reward)
# reward+=-0.1
reward=-distances
reward = torch.where(distances < 0.005, 50, 0)
#logging.warning(reward)
#reward=50
self.rew_buf[:] = reward
def is_done(self) -> None:
distances = torch.norm(self.cube_pos - self.tcp_pos, dim=1)
distance_x=abs(self.cube_pos[:,0]-self.tcp_pos[:,0])
distance_y=abs(self.cube_pos[:,1]-self.tcp_pos[:,1])
distance_z = abs(self.cube_pos[:, 2] - self.tcp_pos[:, 2])
resets=torch.where(distances<0.005,1,0)
reset_env_ids = resets.nonzero(as_tuple=False).squeeze(-1)#只获取没有复位的环境
if(len(reset_env_ids)>0):
logging.warning(msg=("成功个数:",len(reset_env_ids)))
resets = torch.where((distance_x > 0.11) | (distance_y > 0.11) | (distance_z > 0.11),
torch.tensor(1, dtype=resets.dtype), resets)
resets = torch.where(self.progress_buf >= self._max_episode_length, torch.tensor(1, dtype=resets.dtype), resets)
self.reset_buf[:] = resets
return
def post_reset(self):
reset_tensor = self._reset_pose.repeat(self._num_envs, 1)#(6->(envs,6))
self.ur5.set_joints(reset_tensor, indices=torch.arange(self._num_envs))
def reset_idx(self, env_ids):
self.update_cube(env_ids)
num_resets = len(env_ids)
random_array = np.random.rand(num_resets, 3)
# 将其缩放到 [-0.2, 0.2)
noise = (random_array - 0.5) * 0.2
cube_pose=self.cube_pose
goal=cube_pose+torch.tensor(noise).cuda()
goal=goal-self.ur5.robot_position
intijoint=np.repeat(self._reset_pose.cpu().numpy()[np.newaxis, :], num_resets, axis=0)
joints=self.ur5.get_iks(goal.cpu().numpy(),
self.origin_cube_orientation.cpu().numpy(),
intijoint)
joints=torch.tensor(joints, dtype=torch.float32).cuda()
indices = env_ids.to(dtype=torch.int32)
self.ur5.set_joints(joints, indices=indices)
# bookkeeping
self.reset_buf[env_ids] = 0
self.progress_buf[env_ids] = 0
################################
def get_cartpole(self):
# add a single robot to the stage
cartpole = Cartpole(
prim_path=self.default_zero_env_path + "/Cartpole", name="Cartpole", translation=self._cartpole_positions
)
# applies articulation settings from the task configuration yaml file
self._sim_config.apply_articulation_settings(
"Cartpole", get_prim_at_path(cartpole.prim_path), self._sim_config.parse_actor_config("Cartpole")
)
def get_ur5(self):
self.ur5=UR5(
prim_path=self.default_zero_env_path + "/UR5", name="UR5", translation=self._cartpole_positions,
ik_urdfPath="E:\\1_Project\\py\\paper3\\sim-force2-real\\model\\ur5new\\ur_description-main\\ur_description-main\\urdf\\ur5.urdf"
)
def get_table(self):
usdpath="C:\\Users\\Administrator\\AppData\\Local\\ov\\pkg\\gym\\OmniIsaacGymEnvs\\omniisaacgymenvs\\robots\\myrobots\\model\\table\\table.usd"
add_reference_to_stage(usdpath, prim_path=self.default_zero_env_path + "/table")
def get_cube(self):
from omni.isaac.core.objects import VisualCuboid
cube_pose=np.array([0.5, 0.3, 1.00])
cube_orientation=np.array([0.0000000000, 1, 0, 0.0000000])
self.origin_cube_pose=torch.tensor(np.tile(cube_pose, (self.num_envs, 1))).cuda()
self.origin_cube_orientation=torch.tensor(np.tile(cube_orientation, (self.num_envs, 1))).cuda()
VisualCuboid(
prim_path=self.default_zero_env_path + "/prop/prop_0",
name="fancy_cube",
position=cube_pose,
orientation=cube_orientation,
scale=np.array([0.05015, 0.05015, 0.05015]),
color=np.array([0, 0, 1.0]),
)
def update_cube(self,indices):
n = len(indices)
# Generate (n, 3) random numbers in the range (-0.3, 0.3)
random_offsets = (torch.rand((n, 3)) * 0.5 - 0.3).cuda()
self.cube_pose=self.origin_cube_pose[indices]+random_offsets
self._cubes.set_local_poses(self.cube_pose,self.origin_cube_orientation,indices)
def set_task_parameters(self):
self.init_error_xyz=0.05
SKRL运行文件
接着是SKRL运行的主程序文件:
python
import torch
import torch.nn as nn
# import the skrl components to build the RL system
from skrl.agents.torch.sac import SAC, SAC_DEFAULT_CONFIG
from skrl.envs.loaders.torch import load_omniverse_isaacgym_env
from skrl.envs.wrappers.torch import wrap_env
from skrl.memories.torch import RandomMemory
from skrl.models.torch import DeterministicMixin, GaussianMixin, Model
from skrl.resources.preprocessors.torch import RunningStandardScaler
from skrl.trainers.torch import SequentialTrainer
from skrl.utils import set_seed
# seed for reproducibility
set_seed() # e.g. `set_seed(42)` for fixed seed
# define models (stochastic and deterministic models) using mixins
class StochasticActor(GaussianMixin, Model):
def __init__(self, observation_space, action_space, device, clip_actions=False,
clip_log_std=True, min_log_std=-2, max_log_std=2):
Model.__init__(self, observation_space, action_space, device)
GaussianMixin.__init__(self, clip_actions, clip_log_std, min_log_std, max_log_std)
self.net = nn.Sequential(nn.Linear(self.num_observations, 256),
nn.ELU(),
nn.Linear(256, 256),
nn.ELU())
self.mean_layer = nn.Sequential(nn.Linear(256, self.num_actions),
nn.Tanh())
self.log_std_parameter = nn.Parameter(torch.zeros(self.num_actions))
def compute(self, inputs, role):
action=self.mean_layer(self.net(inputs["states"]))
log=self.log_std_parameter
output={}
return action,log,output
class Critic(DeterministicMixin, Model):
def __init__(self, observation_space, action_space, device, clip_actions=False):
Model.__init__(self, observation_space, action_space, device)
DeterministicMixin.__init__(self, clip_actions)
self.net = nn.Sequential(nn.Linear(self.num_observations + self.num_actions, 256),
nn.ELU(),
nn.Linear(256, 1),
nn.ELU())
def compute(self, inputs, role):
return self.net(torch.cat([inputs["states"], inputs["taken_actions"]], dim=1)), {}
# load and wrap the Omniverse Isaac Gym environment
env = load_omniverse_isaacgym_env(task_name="Ur5Insert")
env = wrap_env(env)
device = env.device
# instantiate a memory as rollout buffer (any memory can be used for this)
memory = RandomMemory(memory_size=1000000, num_envs=env.num_envs, device=device)
# instantiate the agent's models (function approximators).
# SAC requires 5 models, visit its documentation for more details
# https://skrl.readthedocs.io/en/latest/api/agents/sac.html#models
models = {}
models["policy"] = StochasticActor(env.observation_space, env.action_space, device)
models["critic_1"] = Critic(env.observation_space, env.action_space, device)
models["critic_2"] = Critic(env.observation_space, env.action_space, device)
models["target_critic_1"] = Critic(env.observation_space, env.action_space, device)
models["target_critic_2"] = Critic(env.observation_space, env.action_space, device)
# configure and instantiate the agent (visit its documentation to see all the options)
# https://skrl.readthedocs.io/en/latest/api/agents/sac.html#configuration-and-hyperparameters
cfg = SAC_DEFAULT_CONFIG.copy()
cfg["gradient_steps"] = 1
cfg["batch_size"] = 4096
cfg["discount_factor"] = 0.99
cfg["polyak"] = 0.005
cfg["actor_learning_rate"] = 5e-4
cfg["critic_learning_rate"] = 5e-4
cfg["random_timesteps"] = 80
cfg["learning_starts"] = 80
cfg["grad_norm_clip"] = 0
cfg["learn_entropy"] = True
cfg["entropy_learning_rate"] = 5e-3
cfg["initial_entropy_value"] = 1.0
cfg["state_preprocessor"] = RunningStandardScaler
cfg["state_preprocessor_kwargs"] = {"size": env.observation_space, "device": device}
# logging to TensorBoard and write checkpoints (in timesteps)
cfg["experiment"]["write_interval"] = 800
cfg["experiment"]["checkpoint_interval"] = 8000
cfg["experiment"]["directory"] = "runs/torch/Ur5InsertSAC"
agent = SAC(models=models,
memory=memory,
cfg=cfg,
observation_space=env.observation_space,
action_space=env.action_space,
device=device)
# configure and instantiate the RL trainer
cfg_trainer = {"timesteps": 160000, "headless": True}
trainer = SequentialTrainer(cfg=cfg_trainer, env=env, agents=agent)
# start training
trainer.train()
训练结果与速度对比
在这里,我使用的是SAC,并且在yaml配置文件里面改了很多参数,最终才把整个程序跑起来并成功训练。不得不吐槽一下,SKRL对于这么一个简单的任务,竟然对超参数那么敏感,我使用PPO甚至训练了5W步都不收敛,跟SB3比还是有点差距的。
这里我只设置了32个agent,SAC大概在1000步左右就学会了怎么reach。1000步的时间大概花了1分半钟。不得不说,这个速度相比官方的cartpole例程,1024个agent相比是要慢非常多的。这其中是什么原因我也不知道,速度慢了差不多30倍。
但是尽管如此,omni Isaac gym的并行强化学习还是非常强,仅仅需要1分钟就把任务学会了。
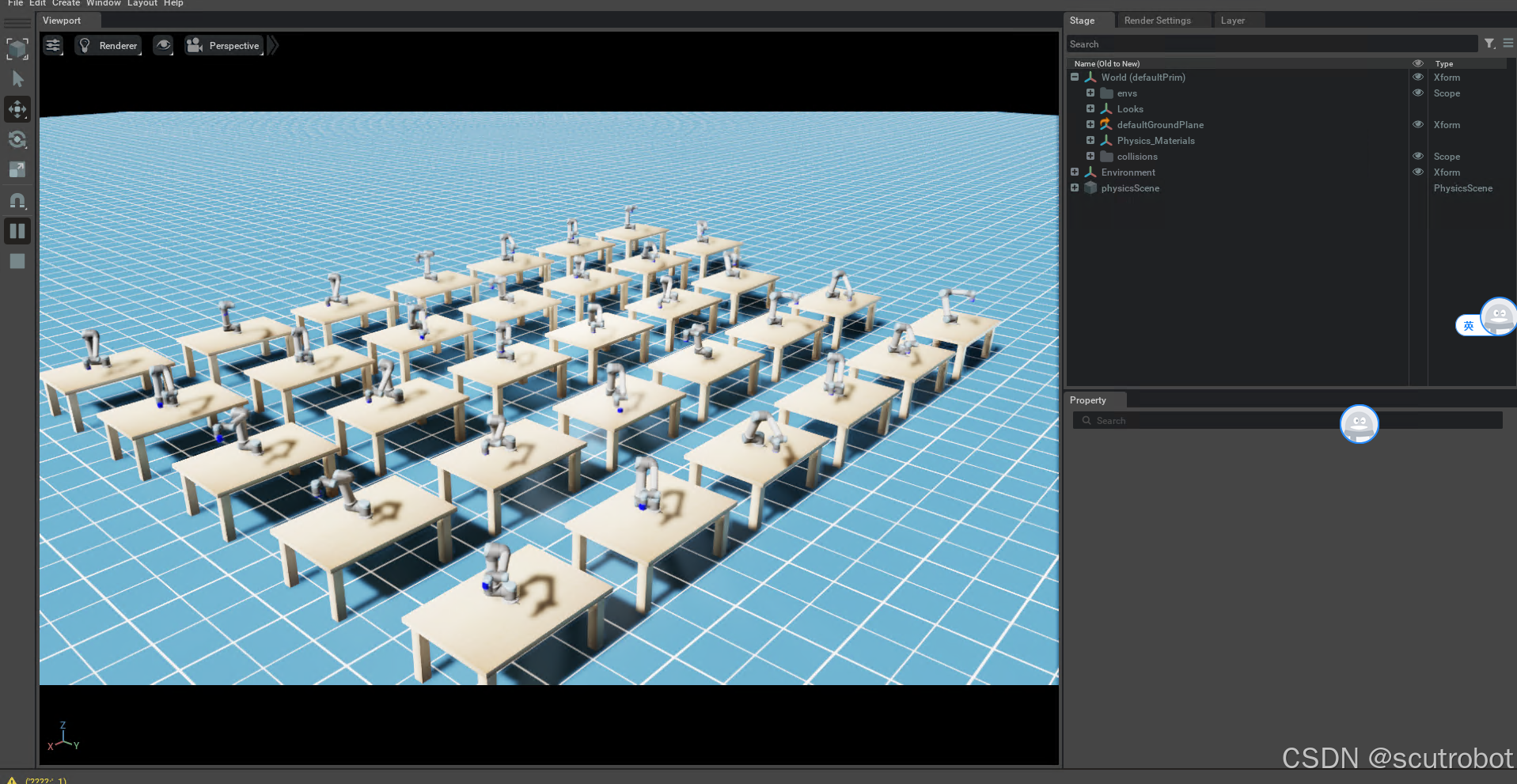
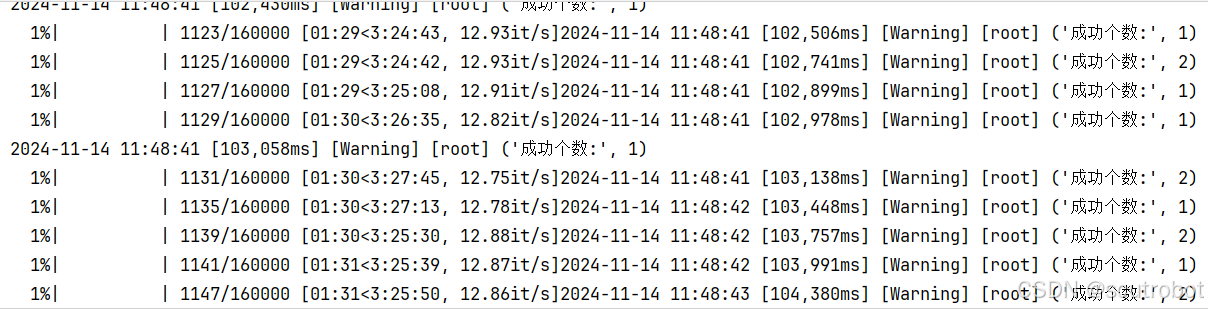
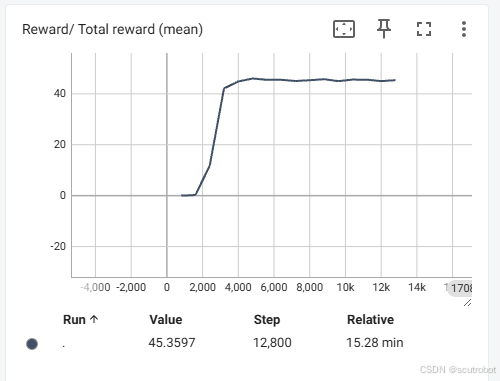
另外我也对比了只是用1个agent的效果:
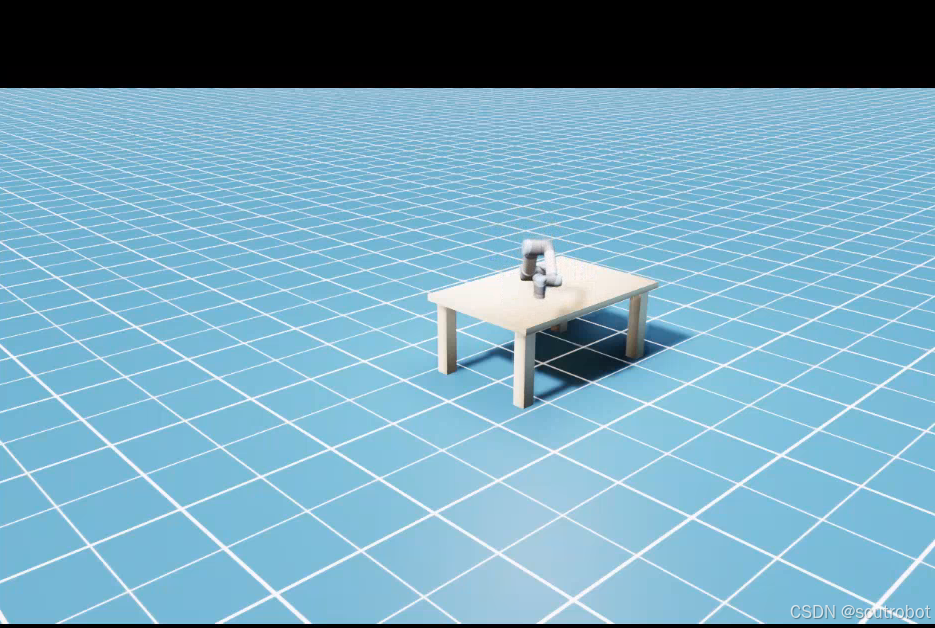
首先训练速度并没有快很多,1分钟1600步左右,其次这个训练结果跟并行训练比确实差很多。
32个agent在1000回合左右reward就已经上去了,并且有智能体已经能够陆续完成任务。但是只有一个agent的时候,甚至训练到了 5000步都还没有一次成功,更别说reward上去了。
然后,可以测试下把机器人的数量加到512是个什么情况,把机器人加到512后,软件启动有了明显的卡顿,等了1分钟界面才显示出来。
并且训练速度也是一言难尽,1分钟左右才100个回合。

综上而言,OMNI isaac gym在并行训练上确实是有很强大的效果,并且效率提升很大。但是在自己编写环境时,速度远远不及官方的例程好,甚至会有点卡顿。
结果分析
这里我分析可能存在的问题:
- 机器人模型是多关节的,而cartpole只是2关节的,关节数会对仿真速度造成影响。
2.尽管OMNI ISAAC GYM环境给你并行出来很多个机器人,但是你在做数据处理的时候,也非常考验你的编程能力。例如这里我没有使用官方的控制库rmpflow,而是选择了自己求解IK,我写的是for循环求解IK,那么每多一个机器人,就会多求一次ik,这里就会造成大量的时间消耗。目前我还没有找到可以批量求IK的库。此外不单是IK,如果涉及到图像处理,例如想使用opencv做一些边缘提取的话,那么这种for循环更是灾难。
3.但尽管如此,看到只有1个机器人的时候,运行速度也远不如官方给的carpole例程
运行体验与建议
-
Isaac sim实际上nvida很早就推出了,近些年也一直有在更新。但每次更新出来bug都很多,并且每次版本迭代API变化都很大。并行仿真环境一开始先是isaac gym,然后到了omni isaac gym,接着又是orbit。现在4.0之后,前面三个版本直接弃用,全部移植到isaac lab里面。需要仔细移植。
-
Isaac sim的优势在于视觉的仿真,正如官方给出的demo,视觉的仿真可以做到非常的逼真,这对于做视觉操作任务的研究无疑是非常好的,特别是在做视觉的sim2real以及数据合成这一块。但是力传感器的仿真一直存在问题,不知道4.0会不会好一些。
希望这些建议能帮助 NVIDIA 不断完善 Isaac Sim,使其成为更加优秀的仿真平台。