遗传算法与深度学习实战------进化优化的局限性
-
- [0. 前言](#0. 前言)
- [1. 数据集加载](#1. 数据集加载)
- [2. 模型构建](#2. 模型构建)
- 相关链接
0. 前言
深度学习 (Deep learning
, DL
) 模型的规模不断扩大,从早期只有数百个参数的模型到最新的拥有数十亿个参数的 transformer
模型。优化或训练这些网络需要大量的计算资源,为了更好的进行评估,我们从简单数据集转向更实际的进化优化应用。
1. 数据集加载
在本节中,我们将使用 MNIST
手写数字数据集执行分类分类,MNIST
通常是学习构建深度学习 (Deep learning
, DL
) 网络进行分类的第一个数据集。
(1) 导入所需库后,加载 MNIST
数据集,进行数据标准化:
python
import tensorflow as tf
import numpy as np
import sklearn
import sklearn.datasets
import sklearn.linear_model
import matplotlib.pyplot as plt
from IPython.display import clear_output
from deap import algorithms
from deap import base
from deap import benchmarks
from deap import creator
from deap import tools
import random
mnist = tf.keras.datasets.mnist
(x_train, y_train), (x_test, y_test) = mnist.load_data()
X, Y = x_train / 255.0, y_train
plt.imshow(X[0])
print(Y[0])
数据集中数字样本示例如下所示。
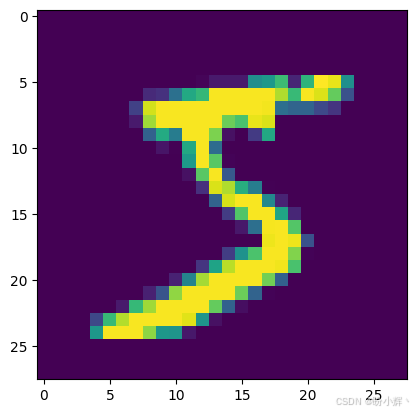
2. 模型构建
(1) 构建模型,并进行训练,使用 livelossplot
模块的 PlotLossesKeras()
函数显示实时结果。之后,显示模型准确率并生成分类报告:
python
middle_layer = 128 #@param {type:"slider", min:16, max:128, step:2}
model = tf.keras.models.Sequential([
tf.keras.layers.Flatten(input_shape=(28, 28)),
tf.keras.layers.Dense(middle_layer, activation='relu'),
tf.keras.layers.Dense(10, activation='softmax')
])
optimizer = tf.keras.optimizers.Adam(learning_rate=.001)
model.compile(optimizer=optimizer,
loss='sparse_categorical_crossentropy',
metrics=['accuracy'])
model.summary()
trainableParams = np.sum([np.prod(v.get_shape()) for v in model.trainable_weights])
print(f"Trainable parameters: {trainableParams}")
模型摘要如下所示:
shell
Model: "sequential"
_________________________________________________________________
Layer (type) Output Shape Param #
=================================================================
flatten (Flatten) (None, 784) 0
dense (Dense) (None, 128) 100480
dense_1 (Dense) (None, 10) 1290
=================================================================
Total params: 101,770
Trainable params: 101,770
Non-trainable params: 0
_________________________________________________________________
Trainable parameters: 101770
基于测试预测结果使用 sklearn
模块 classification_report()
函数生成的分类报告如下所示,可以看到,网络在分类手写数字方面表现出色:
shell
precision recall f1-score support
0 0.18 0.00 0.00 980
1 0.88 0.96 0.92 1135
2 0.65 0.80 0.72 1032
3 0.47 0.85 0.61 1010
4 0.55 0.81 0.65 982
5 0.35 0.05 0.08 892
6 0.71 0.91 0.79 958
7 0.50 0.00 0.01 1028
8 0.58 0.81 0.68 974
9 0.55 0.81 0.66 1009
accuracy 0.61 10000
macro avg 0.54 0.60 0.51 10000
weighted avg 0.55 0.61 0.52 10000
(2) 执行进化过程,随着训练的进行模型的准确率变化以及分类报告如下图所示。可以看到,当进化优化方法用于更大的模型时,它有严重的局限性:
python
def score_model():
y_hat = model.predict(x_test)
acc = [np.argmax(y)==y_test[i] for i,y in enumerate(y_hat)]
return sum(acc)/len(acc)
def print_parameters():
for layer in model.layers:
for na in layer.get_weights():
print(na)
def set_parameters(individual):
idx = 0
tensors=[]
for layer in model.layers:
for na in layer.get_weights():
size = na.size
sh = na.shape
t = individual[idx:idx+size]
t = np.array(t)
t = np.reshape(t, sh)
idx += size
tensors.append(t)
model.set_weights(tensors)
individual = np.random.rand(trainableParams)
set_parameters(individual)
print(score_model())
print_parameters()
creator.create("FitnessMax", base.Fitness, weights=(-1.0,))
creator.create("Individual", list, fitness=creator.FitnessMax)
def uniform(low, up, size=None):
try:
return [random.uniform(a, b) for a, b in zip(low, up)]
except TypeError:
return [random.uniform(a, b) for a, b in zip([low] * size, [up] * size)]
toolbox = base.Toolbox()
toolbox.register("attr_float", uniform, -1, 1, trainableParams)
toolbox.register("individual", tools.initIterate, creator.Individual, toolbox.attr_float)
toolbox.register("population", tools.initRepeat, list, toolbox.individual)
toolbox.register("select", tools.selTournament, tournsize=5)
def customBlend(ind1, ind2):
for i, (x1, x2) in enumerate(zip(ind1, ind2)):
ind1[i] = (x1 + x2) / 2
ind2[i] = (x1 + x2) / 2
return ind1, ind2
#toolbox.register("mate", tools.cxBlend, alpha=.5)
toolbox.register("mate", customBlend)
toolbox.register("mutate", tools.mutGaussian, mu=0.0, sigma=.1, indpb=.25)
def evaluate(individual):
set_parameters(individual)
print('.', end='')
return 1./score_model(),
toolbox.register("evaluate", evaluate)
MU = 25 #@param {type:"slider", min:5, max:1000, step:5}
NGEN = 1000 #@param {type:"slider", min:100, max:1000, step:10}
RGEN = 10 #@param {type:"slider", min:1, max:100, step:1}
CXPB = .6
MUTPB = .3
random.seed(64)
pop = toolbox.population(n=MU)
hof = tools.HallOfFame(1)
stats = tools.Statistics(lambda ind: ind.fitness.values)
stats.register("avg", np.mean)
stats.register("std", np.std)
stats.register("min", np.min)
stats.register("max", np.max)
from sklearn.metrics import classification_report
best = None
history = []
for g in range(NGEN):
pop, logbook = algorithms.eaSimple(pop, toolbox,
cxpb=CXPB, mutpb=MUTPB, ngen=RGEN, stats=stats, halloffame=hof, verbose=False)
best = hof[0]
clear_output()
print(f"Gen ({(g+1)*RGEN})")
history.extend([1/l["min"] for l in logbook])
plt.plot(history)
plt.show()
set_parameters(best)
accuracy = score_model()
print("Best Neural Network accuracy : ", accuracy)
if accuracy > .99999: #stop condition
break
y_pred = model.predict(x_test)
y_pred = np.argmax(y_pred, axis=1)
print(classification_report(y_test, y_pred))
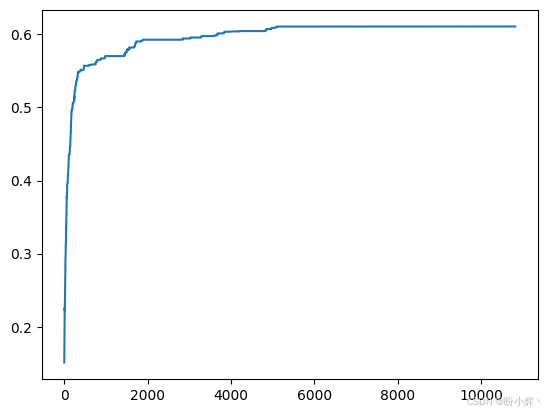
如上图所示,使用进化优化/搜索寻找最佳的网络权重/参数得到的模型性能较差,网络在几个小时的训练后的准确率仅仅只有 60%
,每个类别的准确率结果都不够良好。
可以通过完成以下问题进一步测试神经进化权重/参数优化的极限:
- 通过改变网络大小来修改基本的
Keras
模型,观察使用较小的网络是否能获得更好的结果 - 向模型添加卷积层和最大池化,可以帮助减少要演化的模型参数总数
相关链接
遗传算法与深度学习实战(1)------进化深度学习
遗传算法与深度学习实战(4)------遗传算法(Genetic Algorithm)详解与实现
遗传算法与深度学习实战(16)------神经网络超参数优化
遗传算法与深度学习实战(17)------使用随机搜索自动超参数优化
遗传算法与深度学习实战(18)------使用网格搜索自动超参数优化
遗传算法与深度学习实战(19)------使用粒子群优化自动超参数优化
遗传算法与深度学习实战(20)------使用进化策略自动超参数优化
遗传算法与深度学习实战(21)------使用差分搜索自动超参数优化
遗传算法与深度学习实战(23)------利用遗传算法优化深度学习模型
遗传算法与深度学习实战(24)------在Keras中应用神经进化优化