4.1由于决策树只在样本同属于一类或者所有特征值都用完或缺失时生成叶节点,同一节点的样本,在路径上的特征值都相同,而训练集中又没有冲突数据,所以必定存在训练误差为0的决策树
4.2使用最小训练误差会导致过拟合,使得学习模型泛化能力下降。
4.3
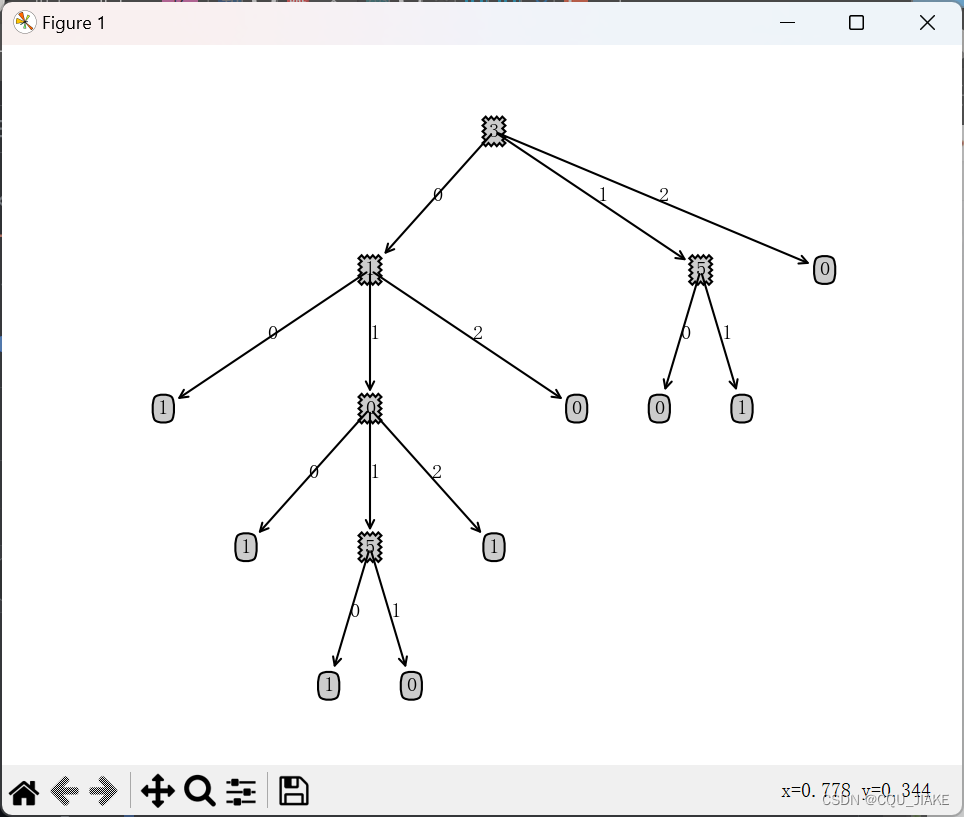
青绿0,乌黑1,浅白2
蜷缩0,稍蜷1,硬挺2
浊响0,沉闷1,清脆2
清晰0,烧糊1,模胡2
凹陷0,稍凹1,平坦2
硬滑0,软粘1
import numpy as np
import treePlotter
np.random.seed(100)
class DecisionTreeClassifier:
def __init__(self,tree_type='ID3',k_classes=2):
self.tree_type=tree_type
self.k_classes=k_classes
if tree_type=='ID3':
self.gain_func=self.Gain
elif tree_type=='CART':
self.gain_func=self.GiniIndex
elif tree_type=='C45':
self.gain_func=self.GainRatio
else:
raise ValueError('must be ID3 or CART or C45')
self.tree=None
def fit(self,X,y):
D={}
D['X']=X
D['y']=y
A=np.arange(X.shape[1])
aVs={}
for a in A:
aVs[a]=np.unique(X[:,a])
self.tree=self.TreeGenerate(D,A,aVs)
def predict(self,X):
if self.tree is None:
raise RuntimeError('cant predict before fit')
y_pred=[]
for i in range(X.shape[0]):
tree = self.tree
x=X[i]
while True:
if not isinstance(tree,dict):
y_pred.append(tree)
break
a=list(tree.keys())[0]
tree=tree[a]
if isinstance(tree,dict):
val = x[a]
tree = tree[val]
else:
y_pred.append(tree)
break
return np.array(y_pred)
def TreeGenerate(self,D,A,aVs):
X=D['X']
y=D['y']
# 情形1
unique_classes=np.unique(y)
if len(unique_classes)==1:
return unique_classes[0]
flag=True
for a in A:
if(len(np.unique(X[:,a]))>1):
flag=False
break
# 情形2
if flag:
return np.argmax(np.bincount(y))
gains=np.zeros((len(A),))
if self.tree_type=='C45':
gains=np.zeros((len(A),2))
for i in range(len(A)):
gains[i]=self.gain_func(D,A[i])
#print(gains)
subA=None
if self.tree_type=='CART':
a_best=A[np.argmin(gains)]
subA=np.delete(A,np.argmin(gains))
elif self.tree_type=='ID3':
a_best=A[np.argmax(gains)]
subA=np.delete(A,np.argmax(gains))
elif self.tree_type=='C45':
gain_mean=np.mean(gains[:,0])
higher_than_mean_indices=np.where(gains[:,0]>=gain_mean)
higher_than_mean=gains[higher_than_mean_indices,1][0]
index=higher_than_mean_indices[0][np.argmax(higher_than_mean)]
a_best=A[index]
subA=np.delete(A,index)
tree={a_best:{}}
for av in aVs[a_best]:
indices=np.where(X[:,a_best]==av)
Dv={}
Dv['X']=X[indices]
Dv['y']=y[indices]
if len(Dv['y'])==0:
tree[a_best][av]=np.argmax(np.bincount(y))
else:
tree[a_best][av]=self.TreeGenerate(Dv,subA,aVs)
return tree
@classmethod
def Ent(cls,D):
y=D['y']
bin_count=np.bincount(y)
total=len(y)
ent=0.
for k in range(len(bin_count)):
p_k=bin_count[k]/total
if p_k!=0:
ent+=p_k*np.log2(p_k)
return -ent
@classmethod
def Gain(cls,D,a):
X=D['X']
y=D['y']
aV=np.unique(X[:,a])
sum=0.
for v in range(len(aV)):
Dv={}
indices=np.where(X[:,a]==aV[v])
Dv['X']=X[indices]
Dv['y']=y[indices]
ent=cls.Ent(Dv)
sum+=(len(Dv['y'])/len(y)*ent)
gain=cls.Ent(D)-sum
return gain
@classmethod
def Gini(cls,D):
y = D['y']
bin_count = np.bincount(y)
total = len(y)
ent = 0.
for k in range(len(bin_count)):
p_k = bin_count[k] / total
ent+=p_k**2
return 1-ent
@classmethod
def GiniIndex(cls,D,a):
X = D['X']
y = D['y']
aV = np.unique(X[:, a])
sum = 0.
for v in range(len(aV)):
Dv = {}
indices = np.where(X[:, a] == aV[v])
Dv['X'] = X[indices]
Dv['y'] = y[indices]
ent = cls.Gini(Dv)
sum += (len(Dv['y']) / len(y) * ent)
gain = sum
return gain
@classmethod
def GainRatio(cls,D,a):
X = D['X']
y = D['y']
aV = np.unique(X[:, a])
sum = 0.
intrinsic_value=0.
for v in range(len(aV)):
Dv = {}
indices = np.where(X[:, a] == aV[v])
Dv['X'] = X[indices]
Dv['y'] = y[indices]
ent = cls.Ent(Dv)
sum += (len(Dv['y']) / len(y) * ent)
intrinsic_value+=(len(Dv['y'])/len(y))*np.log2(len(Dv['y'])/len(y))
gain = cls.Ent(D) - sum
intrinsic_value=-intrinsic_value
gain_ratio=gain/intrinsic_value
return np.array([gain,gain_ratio])
if __name__=='__main__':
watermelon_data = np.array([[0, 0, 0, 0, 0, 0], [1, 0, 1, 0, 0, 0],
[1, 0, 0, 0, 0, 0], [0, 0, 1, 0, 0, 0],
[2, 0, 0, 0, 0, 0], [0, 1, 0, 0, 1, 1],
[1, 1, 0, 1, 1, 1], [1, 1, 0, 0, 1, 0],
[1, 1, 1, 1, 1, 0], [0, 2, 2, 0, 2, 1],
[2, 2, 2, 2, 2, 0], [2, 0, 0, 2, 2, 1],
[0, 1, 0, 1, 0, 0], [2, 1, 1, 1, 0, 0],
[1, 1, 0, 0, 1, 1], [2, 0, 0, 2, 2, 0],
[0, 0, 1, 1, 1, 0]])
label = np.array([1, 1, 1, 1, 1, 1, 1, 1, 0, 0, 0, 0, 0, 0, 0, 0, 0])
X_test=np.array([[0, 0, 1, 0, 0, 0], [1, 0, 1, 0, 0, 0],
[1, 1, 0, 1, 1, 0], [1, 0, 1, 1, 1, 0],
[1, 1, 0, 0, 1, 1], [2, 0, 0, 2, 2, 0],
[0, 0, 1, 1, 1, 0]])
decision_clf=DecisionTreeClassifier(tree_type='ID3')
decision_clf.fit(watermelon_data,label)
print(decision_clf.tree)
treePlotter.createPlot(decision_clf.tree)
y_pred=decision_clf.predict(X_test)
print('y_pred:',y_pred)
import matplotlib.pyplot as plt
from pylab import mpl
mpl.rcParams['font.sans-serif'] = ['FangSong']
mpl.rcParams['axes.unicode_minus'] = False
decisionNode = dict(boxstyle="sawtooth", fc="0.8")
leafNode = dict(boxstyle="round4", fc="0.8")
arrow_args = dict(arrowstyle="<-")
def plotNode(nodeTxt, centerPt, parentPt, nodeType):
createPlot.ax1.annotate(nodeTxt, xy=parentPt, xycoords='axes fraction', \
xytext=centerPt, textcoords='axes fraction', \
va="center", ha="center", bbox=nodeType, arrowprops=arrow_args)
def getNumLeafs(myTree):
numLeafs = 0
firstStr = list(myTree.keys())[0]
secondDict = myTree[firstStr]
for key in secondDict.keys():
if type(secondDict[key]).__name__ == 'dict':
numLeafs += getNumLeafs(secondDict[key])
else:
numLeafs += 1
return numLeafs
def getTreeDepth(myTree):
maxDepth = 0
firstStr = list(myTree.keys())[0]
secondDict = myTree[firstStr]
for key in secondDict.keys():
if type(secondDict[key]).__name__ == 'dict':
thisDepth = getTreeDepth(secondDict[key]) + 1
else:
thisDepth = 1
if thisDepth > maxDepth:
maxDepth = thisDepth
return maxDepth
def plotMidText(cntrPt, parentPt, txtString):
xMid = (parentPt[0] - cntrPt[0]) / 2.0 + cntrPt[0]
yMid = (parentPt[1] - cntrPt[1]) / 2.0 + cntrPt[1]
createPlot.ax1.text(xMid, yMid, txtString)
def plotTree(myTree, parentPt, nodeTxt):
numLeafs = getNumLeafs(myTree)
depth = getTreeDepth(myTree)
firstStr = list(myTree.keys())[0]
cntrPt = (plotTree.xOff + (1.0 + float(numLeafs)) / 2.0 / plotTree.totalw, plotTree.yOff)
plotMidText(cntrPt, parentPt, nodeTxt)
plotNode(firstStr, cntrPt, parentPt, decisionNode)
secondDict = myTree[firstStr]
plotTree.yOff = plotTree.yOff - 1.0 / plotTree.totalD
for key in secondDict.keys():
if type(secondDict[key]).__name__ == 'dict':
plotTree(secondDict[key], cntrPt, str(key))
else:
plotTree.xOff = plotTree.xOff + 1.0 / plotTree.totalw
plotNode(secondDict[key], (plotTree.xOff, plotTree.yOff), cntrPt, leafNode)
plotMidText((plotTree.xOff, plotTree.yOff), cntrPt, str(key))
plotTree.yOff = plotTree.yOff + 1.0 / plotTree.totalD
def createPlot(inTree):
fig = plt.figure(1, facecolor='white')
fig.clf()
axprops = dict(xticks=[], yticks=[])
createPlot.ax1 = plt.subplot(111, frameon=False, **axprops)
plotTree.totalw = float(getNumLeafs(inTree))
plotTree.totalD = float(getTreeDepth(inTree))
plotTree.xOff = -0.5 / plotTree.totalw
plotTree.yOff = 1.0
plotTree(inTree, (0.5, 1.0), '')
plt.show()
4.5
import numpy as np
import treePlotter
import sklearn.datasets as datasets
from sklearn.metrics import mean_squared_error
import sklearn.tree as tree
import graphviz
class DecisionTreeRegressor:
def __init__(self, min_samples_split=3,min_samples_leaf=1,random_state=False):
self.min_samples_split=min_samples_split
self.min_samples_leaf=min_samples_leaf
self.random=random_state
self.tree = None
def fit(self, X, y):
D = {}
D['X'] = X
D['y'] = y
A = np.arange(X.shape[1])
self.tree = self.TreeGenerate(D, A)
def predict(self, X):
if self.tree is None:
raise RuntimeError('cant predict before fit')
y_pred = []
for i in range(X.shape[0]):
tree = self.tree
x = X[i]
while True:
if not isinstance(tree, dict):
y_pred.append(tree)
break
a = list(tree.keys())[0]
tree = tree[a]
if isinstance(tree, dict):
val = x[a]
split_val=float(list(tree.keys())[0][1:])
if val<=split_val:
tree=tree[list(tree.keys())[0]]
else:
tree=tree[list(tree.keys())[1]]
else:
y_pred.append(tree)
break
return np.array(y_pred)
def TreeGenerate(self, D, A):
X = D['X']
y = D['y']
if len(y)<=self.min_samples_split:
return np.mean(y)
split_j=None
split_s=None
min_val=1.e10
select_A=A
if self.random is True:
d=len(A)
select_A=np.random.choice(A,size=int(d//2),replace=False)
for j in select_A:
for s in np.unique(X[:,j]):
left_indices=np.where(X[:,j]<=s)[0]
right_indices=np.where(X[:,j]>s)[0]
if len(left_indices)<self.min_samples_leaf or len(right_indices)<self.min_samples_leaf:
continue
val=np.sum((y[left_indices]-np.mean(y[left_indices]))**2)+np.sum((y[right_indices]-np.mean(y[right_indices]))**2)
if val<min_val:
split_j=j
split_s=s
min_val=val
if split_j is None:
return np.mean(y)
tree = {split_j: {}}
left_indices=np.where(X[:,split_j]<=split_s)[0]
right_indices=np.where(X[:,split_j]>split_s)[0]
D_left, D_right = {},{}
D_left['X'],D_left['y'] = X[left_indices],y[left_indices]
D_right['X'],D_right['y']=X[right_indices],y[right_indices]
tree[split_j]['l'+str(split_s)]=self.TreeGenerate(D_left,A)
tree[split_j]['r'+str(split_s)]=self.TreeGenerate(D_right,A)
# 当前节点值
tree[split_j]['val']=np.mean(y)
return tree
if __name__=='__main__':
breast_data = datasets.load_boston()
X, y = breast_data.data, breast_data.target
X_train, y_train = X[:200], y[:200]
X_test, y_test = X[200:], y[200:]
decisiontree_reg=DecisionTreeRegressor(min_samples_split=20,min_samples_leaf=5)
decisiontree_reg.fit(X_train,y_train)
print(decisiontree_reg.tree)
treePlotter.createPlot(decisiontree_reg.tree)
y_pred=decisiontree_reg.predict(X_test)
print('tinyml mse:',mean_squared_error(y_test,y_pred))
sklearn_reg=tree.DecisionTreeRegressor(min_samples_split=20,min_samples_leaf=5,random_state=False)
sklearn_reg.fit(X_train,y_train)
print(sklearn_reg.feature_importances_)
sklearn_pred=sklearn_reg.predict(X_test)
print('sklearn mse:',mean_squared_error(y_test,sklearn_pred))
dot_data=tree.export_graphviz(sklearn_reg,out_file=None)
graph=graphviz.Source(dot_data)
4.9