AI - 如何构建一个大模型中的Tool
大家好!今天我们聊聊一个有趣的技术问题:什么是工具(Tool),如何使用聊天模型调用工具,以及如何将工具的输出传递给聊天模型。我们还是基于LangChain来进行讨论,希望大家能更好地理解这个概念,并能够在自己的项目中应用它们。
什么是LangChain?
再温习一下,LangChain是一个框架,旨在帮助开发者用大语言模型(例如GPT-4)构建智能应用。通过LangChain,你可以将不同的数据源、模型和工具整合到一起,从而构建出复杂、功能强大的应用程序。
什么是工具?
在自然语言处理和大语言模型的领域,"工具"实际上指的就是一些可以执行特定任务的外部函数、API或者服务。比如说,你可以有一个工具来查询天气,一个工具来翻译文本,或者一个工具来进行复杂的数学计算。
简单来说,工具就是那些帮助我们扩展大语言模型功能的小插件。
举个例子,我们可以有一个计算两个数字之和的工具:
python
def add_numbers(a, b):
return a + b
这个add_numbers
函数就是一个简单的工具。
如何使用聊天模型调用工具?
在现代的自然语言处理系统中,我们希望大语言模型(例如GPT-3)能够智能地调用这些工具来获取所需的信息。那么我们如何实现这一点呢?我们可以使用LangChain,一个强大的框架,它可以帮助我们整合这些工具。
举一个例子,现在我们想要让LLM计算一个算术表达式的值,用户输入的问题是"What is 3 * 12? Also, what is 11 + 49?",我们该怎么定义工具来实现呢?
示例代码
啥也不说了,直接上代码,以下是完整的代码实现,其中用到了ChatGroq这个LLM。
备注:对于本文中的代码片段,主体来源于LangChain官网,有兴趣的读者可以去官网查看。
python
import os
from langchain_core.messages import HumanMessage, SystemMessage
from langchain_groq import ChatGroq
from langchain_core.output_parsers import PydanticToolsParser
# 设置环境变量以配置不同的API密钥和LangChain相关配置
os.environ["GROQ_API_KEY"] = '************'
# 定义一个工具函数,用于将两个整数相加。函数名、类型提示和文档字符串
# 都构成了工具的schema,这部分信息会被传递给模型,帮助其更好地理解工具功能。
def add(a: int, b: int) -> int:
"""Add two integers.
Args:
a: First integer
b: Second integer
"""
return a + b
# 定义第二个工具函数,用于将两个整数相乘
def multiply(a: int, b: int) -> int:
"""Multiply two integers.
Args:
a: First integer
b: Second integer
"""
return a * b
# 将上述两个函数添加到工具列表中
tools = [add, multiply]
# 定义查询,这里包含了两个计算任务,一个乘法和一个加法
query = "What is 3 * 12? Also, what is 11 + 49?"
# 初始化ChatGroq模型,这里使用的模型是"llama3-8b-8192"
llm = ChatGroq(model="llama3-8b-8192")
# 绑定工具到语言模型中,使其能够调用这些工具
llm_with_tools = llm.bind_tools(tools)
# 调用绑定了工具的模型,执行查询
output = llm_with_tools.invoke(query)
print(output) # 打印语言模型返回的初始输出
# 创建一个链式调用,将语言模型输出通过Pydantic工具解析器进行进一步处理
chain = llm_with_tools | PydanticToolsParser(tools=[add, multiply])
# 执行链式调用,得到最终结果
result = chain.invoke(query)
print(result) # 打印最终处理后的结果
最后的输出结果为:
[36, 60]
代码详细说明
- 导入必要的模块和库:首先,我们导入了一些用于配置环境变量、消息处理、调用模型和解析输出的库。
- 设置环境变量 :通过
os.environ
设置了一些配置和 API 密钥,确保我们可以正确调用所需的服务。 - 定义工具函数 :我们定义了两个工具函数
add
和multiply
,分别用于加法和乘法运算。每个工具函数都有详细的类型提示和文档字符串,帮助模型更好地理解它们的功能。 - 创建工具列表 :将定义好的工具函数添加到工具列表
tools
中,可以方便地进行批量操作和调用。 - 定义查询 :这里的查询包含了两个计算任务,一个是
3 * 12
,另一个是11 + 49
。 - 初始化语言模型 :使用
ChatGroq
初始化语言模型,并指定模型类型为llama3-8b-8192
。 - 绑定工具到模型 :通过
bind_tools
方法将工具绑定到语言模型中,使模型能够在回答问题时调用这些工具来辅助完成任务。 - 调用绑定了工具的模型 :使用
invoke
方法执行查询,获取模型的初始输出并打印。 - 创建链式调用 :通过
PydanticToolsParser
进一步解析模型输出,使得工具的输出能够被正确处理和呈现。 - 执行链式调用 :使用
invoke
方法,执行链式调用得到最终结果,并打印出经过解析器处理后的结果。
如何将工具的输出传递给聊天模型?
当工具执行完任务并返回结果时,上面的例子只是简单的输出了答案,并不是一个完整的回答,这并不是我们期望的。我们需要将这些结果传递回聊天模型,以便生成最终的回复。让我们继续看另外一份示例代码。
示例代码
python
import os
from langchain_core.messages import HumanMessage, SystemMessage
from langchain_groq import ChatGroq
from langchain_core.output_parsers import PydanticToolsParser
from langchain_core.tools import tool
# 设置环境变量以配置不同的API密钥和LangChain相关配置
os.environ["GROQ_API_KEY"] = '********'
# 使用@tool装饰器定义一个工具函数,用于将两个整数相加
@tool
def add(a: int, b: int) -> int:
"""Adds a and b."""
return a + b
# 使用@tool装饰器定义另外一个工具函数,用于将两个整数相乘
@tool
def multiply(a: int, b: int) -> int:
"""Multiplies a and b."""
return a * b
# 将所有定义好的工具函数添加到工具列表中
tools = [add, multiply]
# 定义一个查询,包含了两个计算任务
query = "What is 3 * 12? Also, what is 11 + 49?"
# 创建一个消息列表,其中包含了人的消息
messages = [HumanMessage(query)]
# 初始化ChatGroq模型,这里使用的模型是"llama3-8b-8192"
llm = ChatGroq(model="llama3-8b-8192")
# 绑定工具到语言模型中,使其能够调用这些工具
llm_with_tools = llm.bind_tools(tools)
# 使用绑定了工具的模型处理消息,生成AI回复
ai_msg = llm_with_tools.invoke(messages)
# 将AI生成的回复消息添加到消息列表中
messages.append(ai_msg)
# 遍历AI回复中的工具调用部分
for tool_call in ai_msg.tool_calls:
# 根据工具调用的名称选择相应的工具
selected_tool = {"add": add, "multiply": multiply}[tool_call["name"].lower()]
# 调用选定的工具并获取工具的回复消息
tool_msg = selected_tool.invoke(tool_call)
# 将工具的回复消息添加到消息列表中
messages.append(tool_msg)
# 再次调用绑定了工具的模型,处理新的消息列表,生成最终输出
output = llm_with_tools.invoke(messages)
# 打印出最终的内容输出
print(output.content)
最后的输出结果如下,符合期望。
36 * 12 = 432. 11 + 49 = 60. The answer to both questions is: 432 and 60.
代码详细说明
-
导入必要的模块和库:首先,我们导入了一些用于环境配置、消息处理、调用模型和工具定义的库。
-
设置环境变量 :通过
os.environ
设置了一些配置和 API 密钥,确保我们可以正确调用所需的服务。 -
定义工具函数:
- 使用
@tool
装饰器定义两个工具函数:add
用于加法运算,multiply
用于乘法运算。 - 这些函数的目标是提供可复用的逻辑,供语言模型在处理查询时调用。
- 使用
-
创建工具列表 :将定义好的工具函数添加到工具列表
tools
中,以方便进行批量操作和调用。 -
定义查询和消息:
- 定义一个查询,包含了加法和乘法两个计算任务。
- 使用
HumanMessage
创建一个初始消息,并将其添加到消息列表messages
中。
-
初始化语言模型 :使用
ChatGroq
初始化语言模型,并指定模型类型为llama3-8b-8192
。 -
绑定工具到模型 :通过
bind_tools
方法,将工具绑定到语言模型中,使模型能够在回答问题时调用这些工具来辅助完成任务。 -
处理初始消息 :使用绑定了工具的模型调用
invoke
方法处理初始消息,生成AI回复并添加到消息列表中。 -
处理工具调用:
- 遍历AI回复中的工具调用部分,识别需要调用的工具名称。
- 根据工具调用的名称选择相应的工具,并调用该工具。
- 将工具的回复消息添加到消息列表中。
-
生成最终输出:第二次调用绑定了工具的模型,处理更新后的消息列表,生成最终的内容输出并打印。
其他案例
如果只是计算一个加减乘除,那么对于工具来说,现实意义不大,现在我们来看一个查询实时天气的案例,你可以询问某一个城市的天气。
python
import os
from langchain_core.messages import HumanMessage, SystemMessage
from langchain_groq import ChatGroq # 导入聊天模型
from langchain_core.tools import tool # 导入工具装饰器
import requests # 导入requests库,用于进行HTTP请求
# 设置环境变量以配置不同的API密钥和LangChain相关配置
os.environ["GROQ_API_KEY"] = '**************'
os.environ["WEATHER_API_KEY"] = '************'
# 使用@tool装饰器定义一个工具函数,用于查询某个城市的天气
@tool
def get_weather(city: str) -> str:
"""Get the weather of a city.
Args:
city: the city to query weather
"""
api_key = os.environ["WEATHER_API_KEY"] # 获取API密钥
url = f"http://api.weatherapi.com/v1/current.json?key={api_key}&q={city}" # 构建API请求URL
response = requests.get(url) # 发送HTTP GET请求
return response.json() # 返回API响应的JSON数据
# 将定义好的工具函数添加到工具列表中
tools = [get_weather]
# 定义一个查询,用于获取上海市的天气
query = "What is weather of Shanghai?"
# 初始化ChatGroq模型,这里使用的模型是"llama3-8b-8192"
llm = ChatGroq(model="llama3-8b-8192")
# 绑定工具到语言模型中,使其能够调用这些工具
llm_with_tools = llm.bind_tools(tools)
# 创建一个消息列表,其中包含了人的消息
messages = [HumanMessage(query)]
# 使用绑定了工具的模型处理消息,生成AI回复
ai_msg = llm_with_tools.invoke(messages)
# 将AI生成的回复消息添加到消息列表中
messages.append(ai_msg)
# 调用get_weather工具,并将查询结果添加到消息列表中
tool_msg = get_weather.invoke(ai_msg.tool_calls[0])
messages.append(tool_msg)
# 再次调用绑定了工具的模型,处理更新后的消息列表,生成最终输出
output = llm_with_tools.invoke(messages)
# 打印出最终的内容输出
print(output.content)
你可以问:
"What is the weather of Shanghai?"
回答是:
The weather in Shanghai is currently Clear with a temperature of 10.1°C (50.2°F) and a wind speed of 7.6 km/h (4.7 mph) from the Southeast. The humidity is at 62% and the atmospheric pressure is at 1018.0 millibars (30.06 inches).
与LLM的交互过程描述如下:
详细描述:
- 用户发送查询: 用户向ChatGroq模型发送查询,内容为"上海的天气是什么?"
- ChatGroq模型处理查询 : ChatGroq模型接收到用户的查询后,识别出该查询需要调用工具
get_weather
。 - 调用工具
get_weather
: ChatGroq模型调用绑定的工具get_weather
,传递参数"上海"。注意:这里其实是ChatGroq告知客户端,让客户端代码来调用工具。 - 工具
get_weather
请求Weather API : 工具get_weather
使用HTTP GET请求Weather API,查询上海的天气信息。 - Weather API返回数据: Weather API返回包含上海天气信息的JSON数据。
- 工具
get_weather
返回天气信息 : 工具get_weather
解析JSON数据并将天气信息返回给ChatGroq模型。 - ChatGroq模型返回结果: ChatGroq模型将解析后的天气信息发送给用户。
代码详细说明
- 导入必要的模块和库 :
- 导入处理环境变量的
os
模块。 - 导入消息处理类
HumanMessage
和SystemMessage
。 - 导入
ChatGroq
用于初始化聊天模型。 - 导入
tool
装饰器用于定义工具函数。 - 导入
requests
库用于进行HTTP请求。
- 导入处理环境变量的
- 设置环境变量 :
- 通过
os.environ
设置各类API密钥和LangChain相关配置,确保代码能够正确调用数据和服务。
- 通过
- 定义工具函数 :
- 使用
@tool
装饰器定义get_weather
函数,该函数用于获取指定城市的天气。 - 构建API请求URL并使用
requests.get
方法发送HTTP GET请求,从而获取数据并返回JSON格式的响应。
- 使用
- 创建工具列表 :
- 将
get_weather
函数添加到工具列表tools
中,以便后续模型调用。
- 将
- 定义查询和消息 :
- 定义查询字符串,表示用户想知道上海的天气。
- 使用
HumanMessage
创建初始消息,并将其添加到消息列表messages
中。
- 初始化语言模型 :
- 使用
ChatGroq
初始化语言模型,并指定模型类型为llama3-8b-8192
。
- 使用
- 绑定工具到模型 :
- 通过
bind_tools
方法将工具绑定到语言模型中,使模型在处理消息时能够调用这些工具。
- 通过
- 处理初始消息 :
- 使用绑定了工具的模型调用
invoke
方法处理初始消息,生成AI回复,并将其添加到消息列表中。
- 使用绑定了工具的模型调用
- 处理工具调用 :
- 调用
get_weather
工具函数处理AI回复中的工具调用部分,将得到的工具消息添加到消息列表中。
- 调用
- 生成最终输出 :
- 再次使用绑定了工具的模型调用
invoke
方法处理更新后的消息列表,生成最终内容输出,并打印结果。
- 再次使用绑定了工具的模型调用
消息抓取
以上整个过程中,我们都是在调用LangChain API与LLM在进行交互,至于底层发送的请求细节,一无所知。在某些场景下面,我们还是需要去探究一下这些具体的细节,这样可以有一个全面的了解。下面我们看一下具体的发送内容。
LLM请求1
json
{
"messages": [
[
{
"lc": 1,
"type": "constructor",
"id": [
"langchain",
"schema",
"messages",
"HumanMessage"
],
"kwargs": {
"content": "What is weather of Shanghai?",
"type": "human"
}
}
]
]
}
LLM回答1
json
{
"generations": [
[
{
"text": "",
"generation_info": {
"finish_reason": "tool_calls",
"logprobs": null
},
"type": "ChatGeneration",
"message": {
"lc": 1,
"type": "constructor",
"id": [
"langchain",
"schema",
"messages",
"AIMessage"
],
"kwargs": {
"content": "",
"additional_kwargs": {
"tool_calls": [
{
"id": "call_2fdg",
"function": {
"arguments": "{\"city\":\"Shanghai\"}",
"name": "get_weather"
},
"type": "function"
}
]
},
"response_metadata": {
"token_usage": {
"completion_tokens": 74,
"prompt_tokens": 919,
"total_tokens": 993,
"completion_time": 0.061666667,
"prompt_time": 0.105363553,
"queue_time": 0.0005093089999999995,
"total_time": 0.16703022
},
"model_name": "llama3-8b-8192",
"system_fingerprint": "fp_a97cfe35ae",
"finish_reason": "tool_calls",
"logprobs": null
},
"type": "ai",
"id": "run-1f49da98-4cc5-42ad-aa68-1962e447dbfa-0",
"tool_calls": [
{
"name": "get_weather",
"args": {
"city": "Shanghai"
},
"id": "call_2fdg",
"type": "tool_call"
}
],
"usage_metadata": {
"input_tokens": 919,
"output_tokens": 74,
"total_tokens": 993
},
"invalid_tool_calls": []
}
}
}
]
],
"llm_output": {
"token_usage": {
"completion_tokens": 74,
"prompt_tokens": 919,
"total_tokens": 993,
"completion_time": 0.061666667,
"prompt_time": 0.105363553,
"queue_time": 0.0005093089999999995,
"total_time": 0.16703022
},
"model_name": "llama3-8b-8192",
"system_fingerprint": "fp_a97cfe35ae"
},
"run": null,
"type": "LLMResult"
}
LLM请求2
json
{
"messages": [
[
{
"lc": 1,
"type": "constructor",
"id": [
"langchain",
"schema",
"messages",
"HumanMessage"
],
"kwargs": {
"content": "What is weather of Shanghai?",
"type": "human"
}
},
{
"lc": 1,
"type": "constructor",
"id": [
"langchain",
"schema",
"messages",
"AIMessage"
],
"kwargs": {
"content": "",
"additional_kwargs": {
"tool_calls": [
{
"id": "call_2fdg",
"function": {
"arguments": "{\"city\":\"Shanghai\"}",
"name": "get_weather"
},
"type": "function"
}
]
},
"response_metadata": {
"token_usage": {
"completion_tokens": 74,
"prompt_tokens": 919,
"total_tokens": 993,
"completion_time": 0.061666667,
"prompt_time": 0.105363553,
"queue_time": 0.0005093089999999995,
"total_time": 0.16703022
},
"model_name": "llama3-8b-8192",
"system_fingerprint": "fp_a97cfe35ae",
"finish_reason": "tool_calls",
"logprobs": null
},
"type": "ai",
"id": "run-1f49da98-4cc5-42ad-aa68-1962e447dbfa-0",
"tool_calls": [
{
"name": "get_weather",
"args": {
"city": "Shanghai"
},
"id": "call_2fdg",
"type": "tool_call"
}
],
"usage_metadata": {
"input_tokens": 919,
"output_tokens": 74,
"total_tokens": 993
},
"invalid_tool_calls": []
}
},
{
"lc": 1,
"type": "constructor",
"id": [
"langchain",
"schema",
"messages",
"ToolMessage"
],
"kwargs": {
"content": "{\"location\": {\"name\": \"Shanghai\", \"region\": \"Shanghai\", \"country\": \"China\", \"lat\": 31.005, \"lon\": 121.4086, \"tz_id\": \"Asia/Shanghai\", \"localtime_epoch\": 1733010903, \"localtime\": \"2024-12-01 07:55\"}, \"current\": {\"last_updated_epoch\": 1733010300, \"last_updated\": \"2024-12-01 07:45\", \"temp_c\": 9.2, \"temp_f\": 48.6, \"is_day\": 1, \"condition\": {\"text\": \"Sunny\", \"icon\": \"//cdn.weatherapi.com/weather/64x64/day/113.png\", \"code\": 1000}, \"wind_mph\": 4.7, \"wind_kph\": 7.6, \"wind_degree\": 229, \"wind_dir\": \"SW\", \"pressure_mb\": 1016.0, \"pressure_in\": 30.0, \"precip_mm\": 0.0, \"precip_in\": 0.0, \"humidity\": 76, \"cloud\": 0, \"feelslike_c\": 8.2, \"feelslike_f\": 46.7, \"windchill_c\": 9.6, \"windchill_f\": 49.3, \"heatindex_c\": 10.4, \"heatindex_f\": 50.8, \"dewpoint_c\": 4.4, \"dewpoint_f\": 40.0, \"vis_km\": 10.0, \"vis_miles\": 6.0, \"uv\": 0.0, \"gust_mph\": 8.6, \"gust_kph\": 13.8}}",
"type": "tool",
"name": "get_weather",
"tool_call_id": "call_2fdg",
"status": "success"
}
}
]
]
}
LLM回答2
json
{
"generations": [
[
{
"text": "According to the tool, the weather in Shanghai is currently Sunny with a temperature of 9.2°C (48.6°F) and a wind speed of 7.6 km/h (4.7 mph).",
"generation_info": {
"finish_reason": "stop",
"logprobs": null
},
"type": "ChatGeneration",
"message": {
"lc": 1,
"type": "constructor",
"id": [
"langchain",
"schema",
"messages",
"AIMessage"
],
"kwargs": {
"content": "According to the tool, the weather in Shanghai is currently Sunny with a temperature of 9.2°C (48.6°F) and a wind speed of 7.6 km/h (4.7 mph).",
"response_metadata": {
"token_usage": {
"completion_tokens": 45,
"prompt_tokens": 1370,
"total_tokens": 1415,
"completion_time": 0.0375,
"prompt_time": 0.062532845,
"queue_time": 0.0015657239999999906,
"total_time": 0.100032845
},
"model_name": "llama3-8b-8192",
"system_fingerprint": "fp_179b0f92c9",
"finish_reason": "stop",
"logprobs": null
},
"type": "ai",
"id": "run-1b04ed85-1696-4574-be5a-dd7a37989fdf-0",
"usage_metadata": {
"input_tokens": 1370,
"output_tokens": 45,
"total_tokens": 1415
},
"tool_calls": [],
"invalid_tool_calls": []
}
}
}
]
],
"llm_output": {
"token_usage": {
"completion_tokens": 45,
"prompt_tokens": 1370,
"total_tokens": 1415,
"completion_time": 0.0375,
"prompt_time": 0.062532845,
"queue_time": 0.0015657239999999906,
"total_time": 0.100032845
},
"model_name": "llama3-8b-8192",
"system_fingerprint": "fp_179b0f92c9"
},
"run": null,
"type": "LLMResult"
}
在这次的回答中,我们可以看到这样的天气状况。
According to the tool, the weather in Shanghai is currently Sunny with a temperature of 9.2°C (48.6°F) and a wind speed of 7.6 km/h (4.7 mph)."
结论
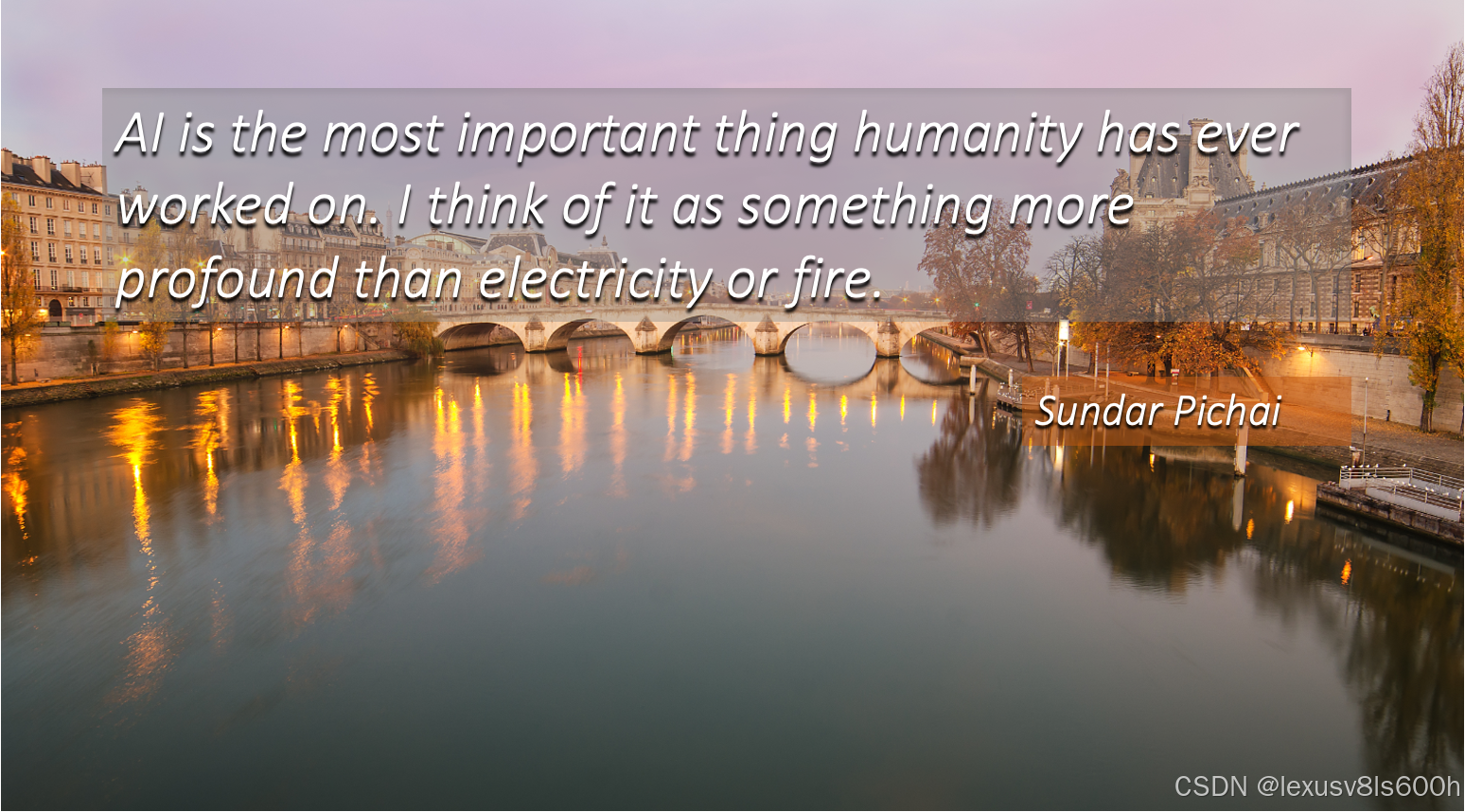
今天,我们探讨了什么是工具,如何使用聊天模型调用工具,以及如何将工具的输出传递回聊天模型。通过LangChain,我们可以轻松地将这些工具集成到大语言模型中,使其功能更为强大。
希望大家通过这篇文章能够掌握这些基本概念和操作,并应用到自己的项目中。如果有任何问题或建议,欢迎在评论区分享!谢谢大家的关注!