llama.cpp的主要目标是在本地和云端的各种硬件上以最小的设置和最先进的性能实现LLM推理。是一个专为大型语言模型(LLM)设计的高性能推理框架,完全使用C和C++编写,没有外部依赖,这使得它可以很容易地被移植到不同的操作系统和硬件平台上。简化大型语言模型部署流程。
一、下载llama.cpp
1、访问其GitHub官方仓库:llama.cpp
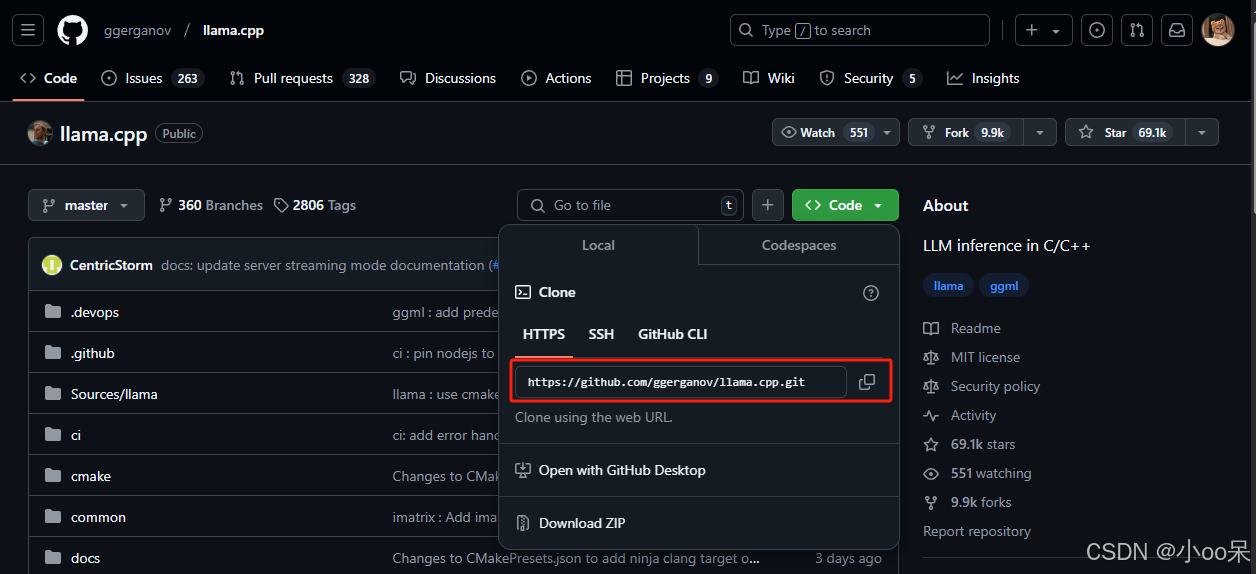
2、在你的服务器指定路径下克隆仓库
bash
git clone https://github.com/ggerganov/llama.cpp.git
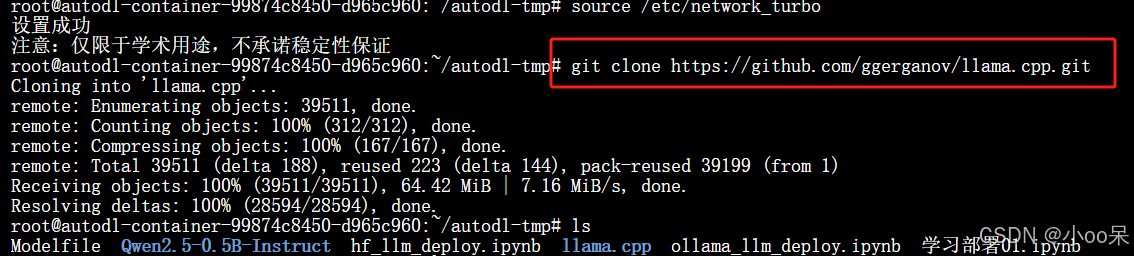
3、安装llama.cpp所需环境
bash
pip install -r requirements.txt

【注】如果你想使用其他方式安装(比如Docker),可以参考官方仓库的下面这个部分。
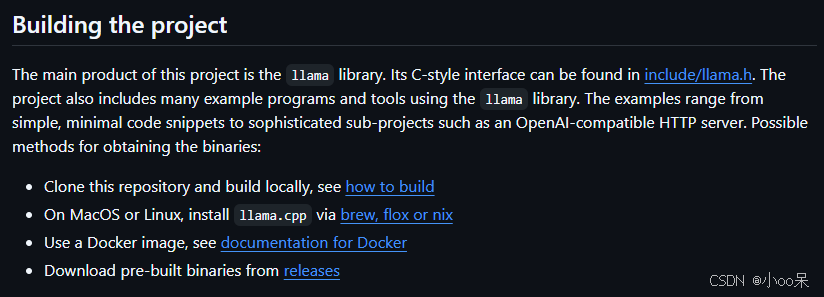
二、将大模型从HF格式转换为GGUF格式
1、在llama.cpp项目路径下会有一个convert_hf_to_gguf.py文件
2、执行命令进行格式转换(默认将模型转换为FP16的GGUF)
bash
python3 convert_hf_to_gguf.py <你模型所在路径>
3、模型格式转换成功后会提示GGUF格式的模型保存在了哪里
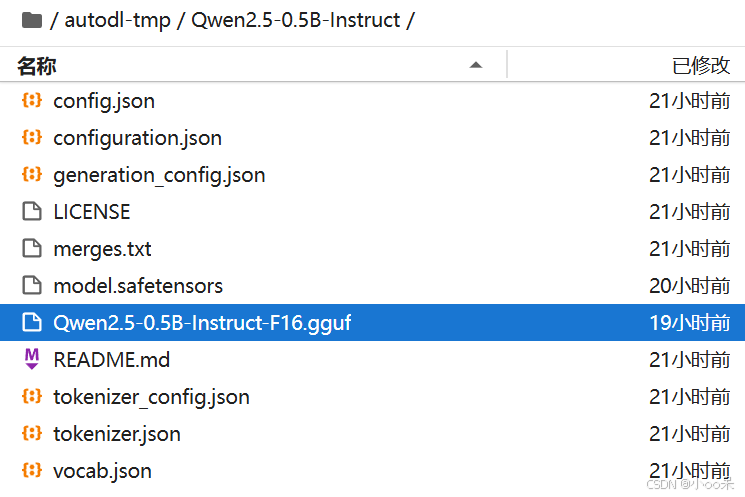
4、除了可以将大模型转换为FP16的量化精度外,还可以做Q8_0量化(等多种)并导出为GGUF格式
bash
# 将模型量化为8位(使用Q8_0方法)
python convert_hf_to_gguf.py <你的模型所在路径> --outtype q8_0 --outfile <希望保存的路径>
如果你在转换格式的时候指定了量化参数,那么llama.cpp还会帮你做量化,下面是官方给出的Q4_0量化模型对比:
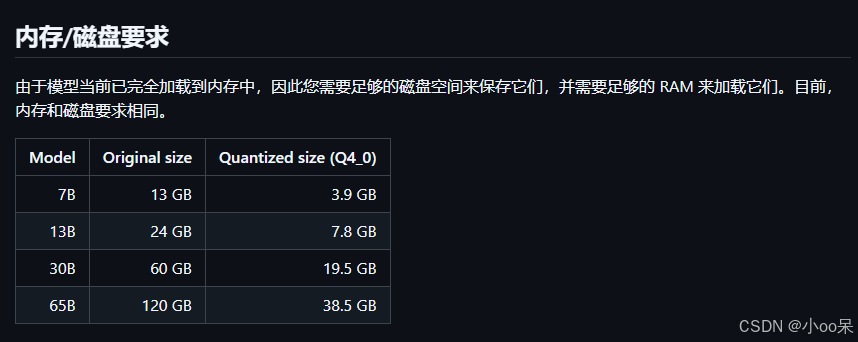
llama.cpp不光支持4位量化,还支持下面多种量化。表中列出了量化前后模型性能和参数规模的bian
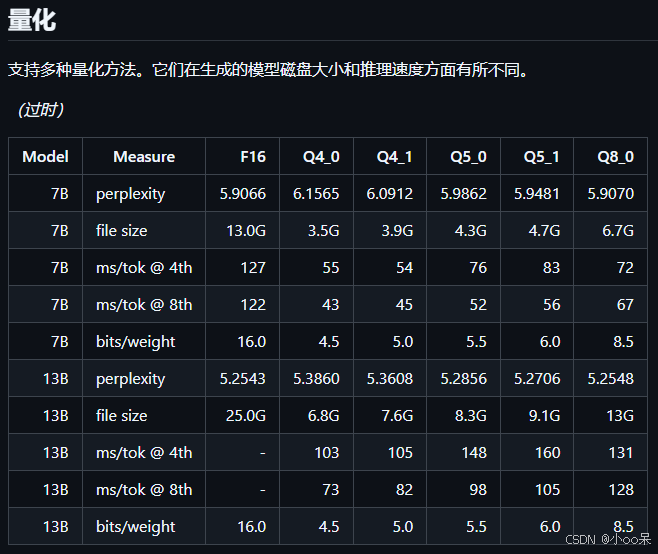
【注】 llama.cpp还提供了更多量化格式转换,说明可见下表:
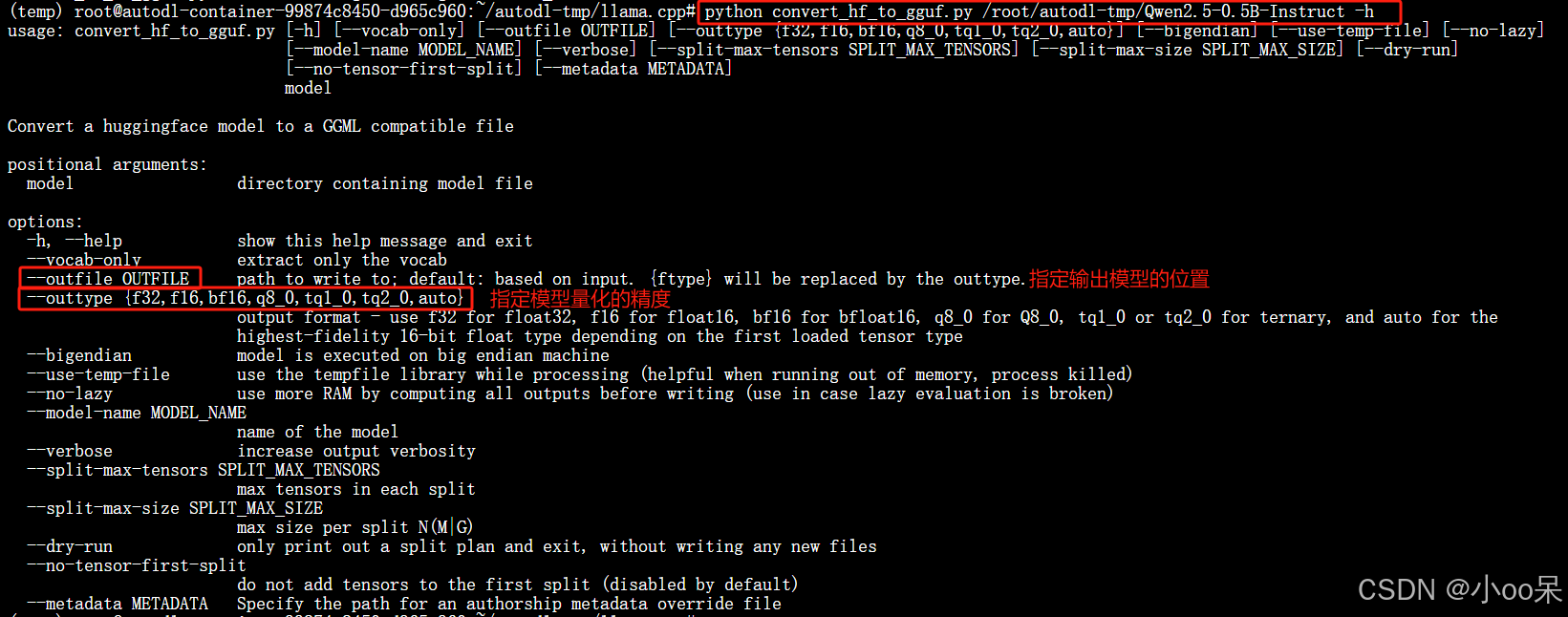