本文中的例子来源于:
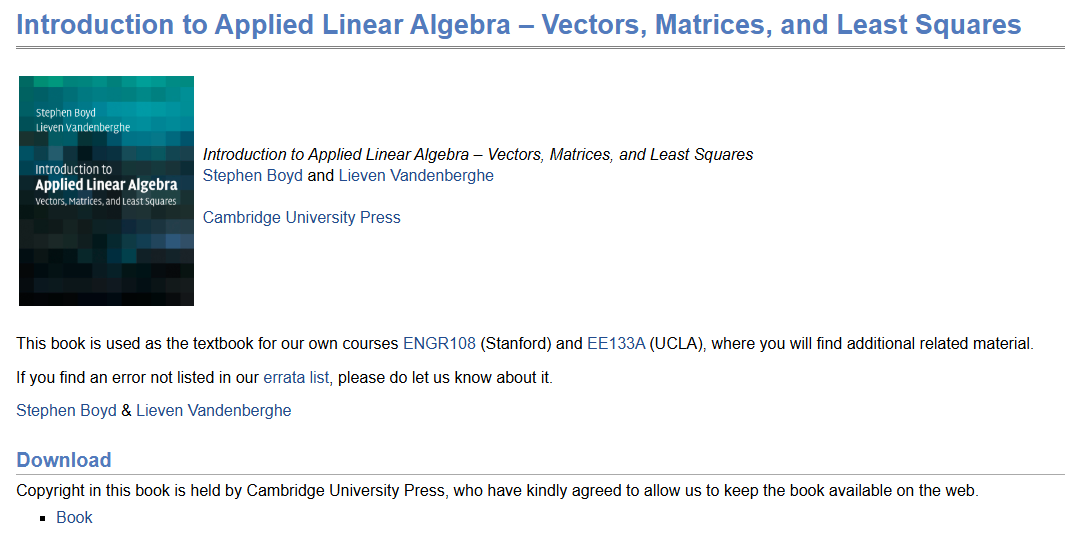
这本书,网址为:https://web.stanford.edu/~boyd/vmls/
矩阵在资产收益(Asset Returns)中的应用:以资产回报矩阵为例
在量化金融中,矩阵作为一种重要的数学工具,被广泛用于描述和分析金融市场中的资产收益。本文将结合一个具体的资产回报矩阵案例,介绍矩阵在资产收益分析中的基本概念及其实际应用。
1. 什么是资产回报矩阵?
资产回报矩阵(Asset Return Matrix)是一个二维数组,用于描述一组资产在多个时间段内的回报率(returns)。假设我们有 (T) 个时间段和 (n) 个资产,资产回报矩阵 (R) 的形式为:
R = [ R 11 R 12 ⋯ R 1 n R 21 R 22 ⋯ R 2 n ⋮ ⋮ ⋱ ⋮ R T 1 R T 2 ⋯ R T n ] . R = \begin{bmatrix} R_{11} & R_{12} & \cdots & R_{1n} \\ R_{21} & R_{22} & \cdots & R_{2n} \\ \vdots & \vdots & \ddots & \vdots \\ R_{T1} & R_{T2} & \cdots & R_{Tn} \end{bmatrix}. R= R11R21⋮RT1R12R22⋮RT2⋯⋯⋱⋯R1nR2n⋮RTn .
- 矩阵的行(row): 每一行代表某个时间段(如某一天)内所有资产的收益。
- 矩阵的列(column): 每一列表示某个资产在所有时间段的收益序列。
- 矩阵中的元素 ( R i j R_{ij} Rij): 表示第 ( i i i) 个时间段内,第 ( j j j) 个资产的回报率。例如,( R 12 , 7 = − 0.03 R_{12,7} = -0.03 R12,7=−0.03) 表示第 12 个时间段内,第 7 个资产的回报率为 -3%(即亏损 3%)。
2. 一个简单的案例
下面的表格显示了一个资产回报矩阵的例子,其中包含 ( n = 4 n = 4 n=4) 个资产(Apple, Google, 3M 和 Amazon)在 ( T = 3 T = 3 T=3) 个时间段(2016 年 3 月 1 日、2 日和 3 日)内的日收益率:
Date | AAPL | GOOG | MMM | AMZN |
---|---|---|---|---|
March 1, 2016 | 0.00219 | 0.00006 | -0.00113 | 0.00202 |
March 2, 2016 | 0.00744 | -0.00894 | -0.00019 | -0.00468 |
March 3, 2016 | 0.01488 | -0.00215 | 0.00433 | -0.00407 |
我们可以将该数据表示为矩阵 ( R R R):
R = [ 0.00219 0.00006 − 0.00113 0.00202 0.00744 − 0.00894 − 0.00019 − 0.00468 0.01488 − 0.00215 0.00433 − 0.00407 ] . R = \begin{bmatrix} 0.00219 & 0.00006 & -0.00113 & 0.00202 \\ 0.00744 & -0.00894 & -0.00019 & -0.00468 \\ 0.01488 & -0.00215 & 0.00433 & -0.00407 \end{bmatrix}. R= 0.002190.007440.014880.00006−0.00894−0.00215−0.00113−0.000190.004330.00202−0.00468−0.00407 .
3. 矩阵的解释
-
单个元素:
矩阵中的每个元素 ( R i j R_{ij} Rij) 表示第 ( i i i) 个时间段内,第 ( j j j) 个资产的收益。例如:
- ( R 1 , 1 = 0.00219 R_{1,1} = 0.00219 R1,1=0.00219):表示 2016 年 3 月 1 日 Apple 的日收益率为 0.219%。
- ( R 2 , 2 = − 0.00894 R_{2,2} = -0.00894 R2,2=−0.00894):表示 2016 年 3 月 2 日 Google 的日收益率为 -0.894%(即亏损 0.894%)。
-
矩阵的行:
矩阵的每一行表示所有资产在某个时间段内的收益。例如:
- 矩阵的第 3 行 ( [ 0.01488 , − 0.00215 , 0.00433 , − 0.00407 ] [0.01488, -0.00215, 0.00433, -0.00407] [0.01488,−0.00215,0.00433,−0.00407]) 表示 2016 年 3 月 3 日 Apple、Google、3M 和 Amazon 的收益率。
-
矩阵的列:
矩阵的每一列表示某个资产在所有时间段的收益。例如:
- 矩阵的第 2 列 ( [ 0.00006 , − 0.00894 , − 0.00215 ] [0.00006, -0.00894, -0.00215] [0.00006,−0.00894,−0.00215]) 表示 Google 在 2016 年 3 月 1 日、2 日和 3 日的日收益率。
4. 资产回报矩阵的实际应用
4.1 收益时间序列分析
对于任一资产 ( j j j),其回报的时间序列可以表示为矩阵 ( R R R) 的第 ( j j j) 列。通过分析该列,我们可以:
- 绘制收益时间序列的趋势图。
- 计算该资产的均值收益、标准差等统计指标,以衡量其长期表现和风险。
4.2 投资组合收益计算
假设投资者的投资组合权重为一个列向量 ( w = [ w 1 , w 2 , ... , w n ] T w = [w_1, w_2, \dots, w_n]^T w=[w1,w2,...,wn]T),其中 ( w j w_j wj) 表示第 (j) 个资产的权重(投资比例)。投资组合在第 ( i i i) 个时间段的收益可以通过矩阵向量乘法计算:
r i portfolio = R i , : ⋅ w , r_i^{\text{portfolio}} = R_{i,:} \cdot w, riportfolio=Ri,:⋅w,
其中 ( R i , : R_{i,:} Ri,:) 表示第 ( i i i) 行(即所有资产在第 ( i i i) 个时间段的收益)。
例如,假设投资组合权重为 ( w = [ 0.3 , 0.3 , 0.2 , 0.2 ] T w = [0.3, 0.3, 0.2, 0.2]^T w=[0.3,0.3,0.2,0.2]T),在 2016 年 3 月 1 日的收益为:
r 1 portfolio = [ 0.00219 , 0.00006 , − 0.00113 , 0.00202 ] ⋅ [ 0.3 , 0.3 , 0.2 , 0.2 ] T = 0.000939. r_1^{\text{portfolio}} = [0.00219, 0.00006, -0.00113, 0.00202] \cdot [0.3, 0.3, 0.2, 0.2]^T = 0.000939. r1portfolio=[0.00219,0.00006,−0.00113,0.00202]⋅[0.3,0.3,0.2,0.2]T=0.000939.
这表明投资组合在这一天的回报率为 0.0939%。
4.3 风险分析与协方差矩阵
矩阵 ( R R R) 的列可以用来计算资产之间的协方差矩阵 ( C C C),以评估资产间的相关性:
C = 1 T R T R . C = \frac{1}{T} R^T R. C=T1RTR.
协方差矩阵 ( C C C) 的对角线元素表示单个资产的方差,其余元素表示资产间的协方差。
5. 总结
资产回报矩阵是量化金融中的重要工具,能够有效地表示和分析资产的收益数据。通过矩阵操作,我们可以轻松完成收益时间序列分析、投资组合优化、风险评估等任务。在实际应用中,掌握矩阵的基本操作和金融意义,能够帮助我们更好地理解和分析金融市场中的数据。
Application of Matrices in Asset Returns: An Example with an Asset Return Matrix
In quantitative finance, matrices are a fundamental mathematical tool used to describe and analyze the returns of financial assets. This blog post introduces the concept of the asset return matrix and explains its significance and applications, using a simple example.
1. What is an Asset Return Matrix?
An asset return matrix represents the returns of a collection of assets over a set of time periods. If we have ( T T T) time periods and ( n n n) assets, the matrix ( R R R) is defined as:
R = [ R 11 R 12 ⋯ R 1 n R 21 R 22 ⋯ R 2 n ⋮ ⋮ ⋱ ⋮ R T 1 R T 2 ⋯ R T n ] . R = \begin{bmatrix} R_{11} & R_{12} & \cdots & R_{1n} \\ R_{21} & R_{22} & \cdots & R_{2n} \\ \vdots & \vdots & \ddots & \vdots \\ R_{T1} & R_{T2} & \cdots & R_{Tn} \end{bmatrix}. R= R11R21⋮RT1R12R22⋮RT2⋯⋯⋱⋯R1nR2n⋮RTn .
- Rows of the matrix represent the returns of all assets for a specific time period.
- Columns of the matrix represent the return series for a specific asset over all time periods.
- Elements ( R i j R_{ij} Rij) represent the return of the ( j j j)-th asset in the ( i i i)-th time period. For example, ( R 12 , 7 = − 0.03 R_{12,7} = -0.03 R12,7=−0.03) means that asset 7 had a return of ( − 3 % -3\% −3%) in time period 12.
2. A Simple Example
Table shows an example of an asset return matrix for ( n = 4 n = 4 n=4) assets (Apple, Google, 3M, and Amazon) over ( T = 3 T = 3 T=3) time periods (March 1, 2, and 3, 2016):
Date | AAPL | GOOG | MMM | AMZN |
---|---|---|---|---|
March 1, 2016 | 0.00219 | 0.00006 | -0.00113 | 0.00202 |
March 2, 2016 | 0.00744 | -0.00894 | -0.00019 | -0.00468 |
March 3, 2016 | 0.01488 | -0.00215 | 0.00433 | -0.00407 |
This table can be written as the matrix ( R R R):
R = [ 0.00219 0.00006 − 0.00113 0.00202 0.00744 − 0.00894 − 0.00019 − 0.00468 0.01488 − 0.00215 0.00433 − 0.00407 ] . R = \begin{bmatrix} 0.00219 & 0.00006 & -0.00113 & 0.00202 \\ 0.00744 & -0.00894 & -0.00019 & -0.00468 \\ 0.01488 & -0.00215 & 0.00433 & -0.00407 \end{bmatrix}. R= 0.002190.007440.014880.00006−0.00894−0.00215−0.00113−0.000190.004330.00202−0.00468−0.00407 .
3. Interpreting the Matrix
-
Individual elements:
Each element ( R i j R_{ij} Rij) represents the return of asset ( j j j) in time period ( i i i). For example:
- ( R 1 , 1 = 0.00219 R_{1,1} = 0.00219 R1,1=0.00219): Apple's return on March 1, 2016, was 0.219%.
- ( R 2 , 2 = − 0.00894 R_{2,2} = -0.00894 R2,2=−0.00894): Google's return on March 2, 2016, was ( − 0.894 % -0.894\% −0.894%) (a loss of 0.894%).
-
Rows:
Each row gives the returns of all assets in a specific time period. For example:
- The 3rd row ( [ 0.01488 , − 0.00215 , 0.00433 , − 0.00407 ] [0.01488, -0.00215, 0.00433, -0.00407] [0.01488,−0.00215,0.00433,−0.00407]) shows the returns of Apple, Google, 3M, and Amazon on March 3, 2016.
-
Columns:
Each column gives the time series of returns for a specific asset. For example:
- The 2nd column ( [ 0.00006 , − 0.00894 , − 0.00215 ] [0.00006, -0.00894, -0.00215] [0.00006,−0.00894,−0.00215]) shows Google's returns on March 1, 2, and 3, 2016.
4. Practical Applications of the Asset Return Matrix
4.1 Return Time Series Analysis
For any specific asset ( j j j), the return time series is given by the (j)-th column of (R). This can be used to:
- Plot the return trend over time.
- Compute statistics such as average return, standard deviation, or volatility to assess the asset's performance and risk.
4.2 Portfolio Return Calculation
The asset return matrix can be used to compute portfolio returns. Suppose the portfolio weights are represented as a column vector ( w = [ w 1 , w 2 , ... , w n ] T w = [w_1, w_2, \dots, w_n]^T w=[w1,w2,...,wn]T), where ( w j w_j wj) is the proportion of the portfolio invested in asset ( j j j). The portfolio return for time period ( i i i) is:
r i portfolio = R i , : ⋅ w , r_i^{\text{portfolio}} = R_{i,:} \cdot w, riportfolio=Ri,:⋅w,
where ( R i , : R_{i,:} Ri,:) is the ( i i i)-th row of ( R R R) (the returns of all assets in time period ( i i i)).
For example, if the portfolio weights are ( w = [ 0.3 , 0.3 , 0.2 , 0.2 ] T w = [0.3, 0.3, 0.2, 0.2]^T w=[0.3,0.3,0.2,0.2]T), the portfolio return on March 1, 2016, is:
r 1 portfolio = [ 0.00219 , 0.00006 , − 0.00113 , 0.00202 ] ⋅ [ 0.3 , 0.3 , 0.2 , 0.2 ] T = 0.000939. r_1^{\text{portfolio}} = [0.00219, 0.00006, -0.00113, 0.00202] \cdot [0.3, 0.3, 0.2, 0.2]^T = 0.000939. r1portfolio=[0.00219,0.00006,−0.00113,0.00202]⋅[0.3,0.3,0.2,0.2]T=0.000939.
This means the portfolio return on March 1, 2016, was 0.0939%.
4.3 Risk Analysis and Covariance Matrix
The columns of ( R R R) can be used to calculate the covariance matrix ( C C C) of asset returns, which helps assess the risk and correlation between assets:
C = 1 T R T R . C = \frac{1}{T} R^T R. C=T1RTR.
- The diagonal elements of ( C C C) represent the variance of individual assets.
- The off-diagonal elements represent the covariance between pairs of assets.
5. Conclusion
The asset return matrix is a powerful tool in quantitative finance, providing a structured way to represent and analyze asset returns. By leveraging matrix operations, we can easily perform tasks such as return time series analysis, portfolio optimization, and risk assessment. Understanding this framework is essential for anyone looking to analyze financial markets with mathematical precision.
后记
2024年12月19日21点33分于上海,在GPT4o大模型辅助下完成。