理解TP/FP/FN
- TP: 真实关系为A,预测关系也为A。
- FP: 预测为关系A,但真实关系不为A
- FN: 真实关系为A,但预测关系为其他关系。
代码
python
import matplotlib
matplotlib.use('Agg')
import matplotlib.pyplot as plt
from sklearn.metrics import confusion_matrix, ConfusionMatrixDisplay
# 类别标签顺序
labels = ['instance of', 'has part']
# 真实关系标签与模型预测
y_true = ['instance of', 'instance of', 'instance of', 'instance of', 'has part', 'has part']
y_pred = ['instance of', 'instance of', 'has part', 'has part', 'has part', 'instance of']
# 计算混淆矩阵,显式指定标签顺序
cm = confusion_matrix(y_true, y_pred, labels=labels)
# 显示混淆矩阵
disp = ConfusionMatrixDisplay(confusion_matrix=cm, display_labels=labels)
disp.plot(cmap=plt.cm.Blues)
# 旋转x轴标签,优化显示
plt.xticks(rotation=45)
plt.yticks(rotation=0)
plt.tight_layout()
# 保存图像
plt.savefig('confusion_matrix.png')
# 每个类别的pre/recall/f1/support
precision, recall, f1, support = precision_recall_fscore_support(y_true, y_pred, labels=labels)
# 初始化字典存储 TP、FP、FN
results = {label: {'TP': 0, 'FP': 0, 'FN': 0} for label in labels}
# 通过 precision, recall 和 support 反推出每个类别的 TP、FP 和 FN
for i, label in enumerate(labels):
TP = int(support[i] * recall[i]) # recall = TP / (TP + FN)
FN = support[i] - TP # FN = support - TP
FP = int(TP / precision[i]) - TP if precision[i] > 0 else 0 # precision = TP / (TP + FP)
results[label]['TP'] = TP
results[label]['FP'] = FP
results[label]['FN'] = FN
# 输出结果
for label in labels:
print(f"类别: {label}")
print(f" TP: {results[label]['TP']}")
print(f" FP: {results[label]['FP']}")
print(f" FN: {results[label]['FN']}")
混淆矩阵
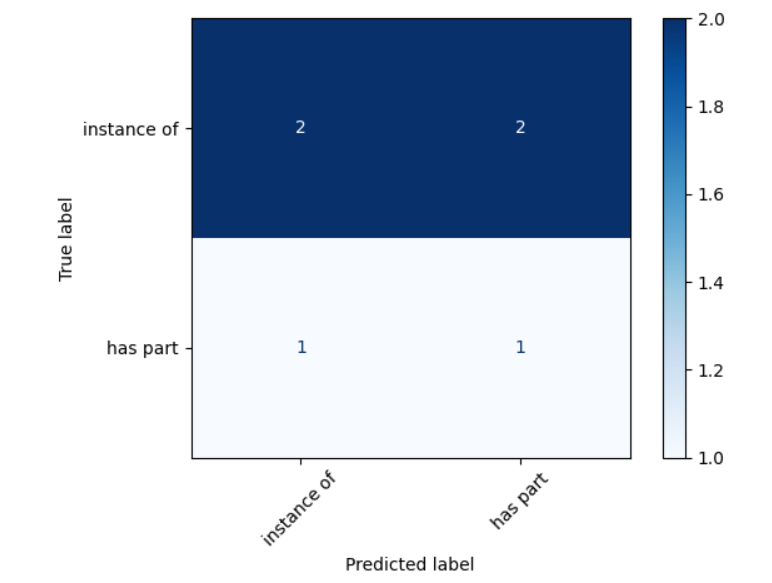
- True Positive (TP):对角线上数值(预测正确)。
- False Positive (FP):同一列中,非对角线上的数值(预测为某类但真实不是)。
- False Negative (FN):同一行中,非对角线上的数值(真实为某类但预测不是)。
演示计算 instance of 类别的TP/FP/FN:
- TP=2
- FP=1
- FN=2
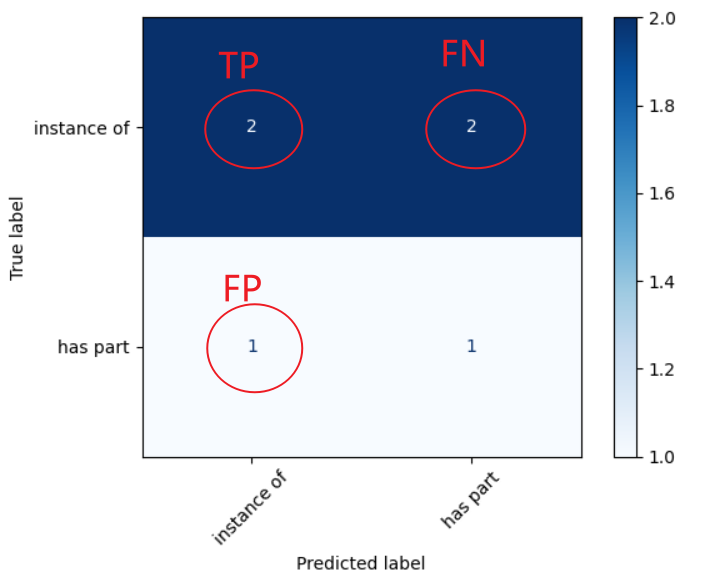