🤩LLaMA-Factory GLM LoRA 微调
安装llama-factory包
git clone --depth 1 https://github.com/hiyouga/LLaMA-Factory.git
进入下载好的llama-factory,安装依赖包
cd LLaMA-Factory
pip install -e ".[torch,metrics]"
#上面这步操作会完成torch、transformers、datasets等相关依赖包的安装
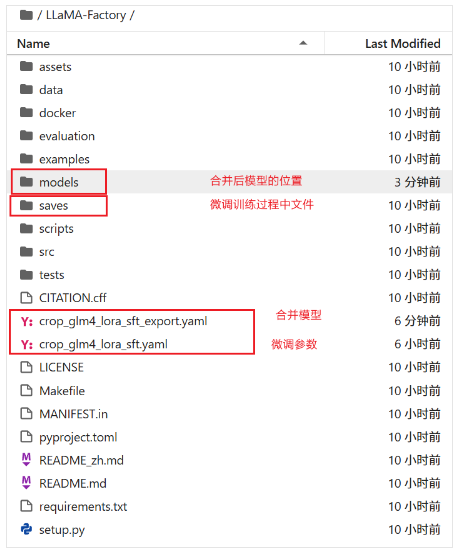
大模型下载
modelscope代码方式下载
py
import torch
from modelscope import snapshot_download, AutoModel, AutoTokenizer
import os
model_dir = snapshot_download('ZhipuAI/glm-4-9b-chat', cache_dir='/root/autodl-tmp', revision='master')
git clone方式下载
git lfs install # 大文件传输
sudo apt-get install git-lfs
git clone https://www.modelscope.cn/ZhipuAI/glm-4-9b-chat.git
指令数据集构建-Alpaca 格式
Alpaca 格式是一种用于训练自然语言处理(NLP)模型的数据集格式,特别是在对话系统和问答系统中。这种格式通常包含指令(instruction)、输入(input)和输出(output)三个部分,它们分别对应模型的提示、模型的输入和模型的预期输出。三者的数据都是字符串形式
Alpaca 格式训练数据集
json
[
{
"instruction": "Answer the following question about the movie 'Inception'.",
"input": "What is the main theme of the movie?",
"output": "The main theme of the movie 'Inception' is the exploration of dreams and reality."
},
{
"instruction": "Provide a summary of the book '1984' by George Orwell.",
"input": "What is the book '1984' about?",
"output": "The book '1984' is a dystopian novel that tells the story of a totalitarian regime and the protagonist's struggle against it."
}
]
我的数据集构建如下
crop_train.json
json
[
{
"instruction": "你是农作物领域专门进行关系抽取的专家。请从给定的文本中抽取出关系三元组,不存在的关系返回空列表。请按照JSON字符串的格式回答。",
"input": "煤是一种常见的化石燃料,家庭用煤经过了从\"煤球\"到\"蜂窝煤\"的演变。",
"output": "[{\"head\": \"煤\", \"relation\": \"use\", \"tail\": \"燃料\"}]"
},
{
"instruction": "你是农作物领域专门进行关系抽取的专家。请从给定的文本中抽取出关系三元组,不存在的关系返回空列表。请按照JSON字符串的格式回答。",
"input": "内分泌疾病是指内分泌腺或内分泌组织本身的分泌功能和(或)结构异常时发生的症候群。",
"output": "[{\"head\": \"腺\", \"relation\": \"use\", \"tail\": \"分泌\"}]"
},
]
我的数据格式转换代码:
py
import json
import re
# 选择要格式转换的数据集
file_name = "merged_trainProcess.json"
# 读取原始数据
with open(f'./{file_name}', 'r', encoding='utf-8') as file:
data = json.load(file)
# 转换数据格式
converted_data = [
{
"instruction": item["instruction"],
"input": item["text"],
"output": json.dumps(item["triplets"], ensure_ascii=False),
} for item in data]
# 将转换后的数据写入新文件
output_file_name = f'processed_{file_name}'
with open(output_file_name, 'w', encoding='utf-8') as file:
json.dump(converted_data, file, ensure_ascii=False, indent=4)
print(f'{output_file_name} Done')
构建好后保存到llama-factory目录中某文件下
修改 LLaMa-Factory 目录中的 data/dataset_info.json 文件,在其中添加:
"crop_merged": {
"file_name": "/home/featurize/data/crop_train.json" #自己的训练数据集.json文件的绝对路径
}
微调模型代码
在 LLaMA-Factory 目录中**新建配置文件 crop_glm4_lora_sft.yaml :**
yaml
### model:glm-4-9b-chat模型地址的绝对路径
model_name_or_path: /home/featurize/glm-4-9b-chat
### method
stage: sft # supervised fine-tuning(监督式微调)
do_train: true # 是否执行训练过程
finetuning_type: lora # 微调技术的类型
lora_target: all # all 表示对模型的所有参数进行 LoRA 微调
### dataset
# dataset 要和 data/dataset_info.json 中添加的信息保持一致
dataset: crop_merged # 数据集的名称
template: glm4 # 数据集的模板类型
cutoff_len: 2048 # 输入序列的最大长度
max_samples: 100000 # 最大样本数量, 代表在训练过程中最多只会用到训练集的1000条数据;-1代表训练所有训练数据集
overwrite_cache: true
preprocessing_num_workers: 16 # 数据预处理时使用的进程数
### output
# output_dir是模型训练过程中的checkpoint,训练日志等的保存目录
output_dir: saves/crop-glm4-epoch10/lora/sft
logging_steps: 10 # 日志记录的频率
#save_steps: 500
plot_loss: true # 绘制损失曲线
overwrite_output_dir: true # 是否覆盖输出目录中的现有内容
save_strategy: epoch # 保存策略,epoch 表示每个 epoch 结束时保存。
### train
per_device_train_batch_size: 1 # 每个设备上的批次大小,每增加几乎显存翻倍
gradient_accumulation_steps: 8 # 梯度累积的步数,这里设置为 8,意味着每 8 步执行一次梯度更新。
learning_rate: 1.0e-4 # 学习率
num_train_epochs: 3 # 训练的 epoch 数
lr_scheduler_type: cosine #学习率调度器的类型
warmup_ratio: 0.1
fp16: true # 更适合模型推理,混合精度训练,在训练中同时使用FP16和FP32
#bf16: true # 更适合训练阶段
gradient_checkpointing: true # 启用梯度检查点(gradient checkpointing),减少中间激活值的显存占用
### eval
do_eval: false # 是否进行评估
val_size: 0.1 # 验证集的大小比例,这里设置为 0.1,即 10%
per_device_eval_batch_size: 1 # 每个设备上的评估批次大小
eval_strategy: steps # 评估策略,steps 表示根据步数进行评估
eval_steps: 1000 # 评估的步数间隔
yaml
### model
model_name_or_path: /home/featurize/glm-4-9b-chat
### method
stage: sft
do_train: true
finetuning_type: lora
lora_target: all
### dataset
dataset: crop_merged
template: glm4
cutoff_len: 512
overwrite_cache: true
preprocessing_num_workers: 16
### output
output_dir: saves/crop-glm4-epoch10/lora/sft
logging_steps: 100
save_steps: 1000
plot_loss: true
overwrite_output_dir: true
### train
per_device_train_batch_size: 1
gradient_accumulation_steps: 8
learning_rate: 1.0e-4
num_train_epochs: 1
lr_scheduler_type: cosine
warmup_ratio: 0.1
bf16: true
gradient_checkpointing: true
### eval
val_size: 0.1
per_device_eval_batch_size: 1
eval_strategy: epoch
执行以下命令开始微调:
cd LLaMA-Factory
llamafactory-cli train crop_glm4_lora_sft.yaml
合并模型代码
训练完成后,在 LLaMA-Factory 目录中**新建配置文件 crop_glm4_lora_sft_export.yaml:**
导出的模型将被保存在models/CropLLM-glm-4-9b-chat
目录下。
yaml
### model
model_name_or_path: /home/featurize/glm-4-9b-chat
# 刚才crop_glm4_lora_sft.yaml文件中的 output_dir
adapter_name_or_path: saves/crop-glm4-epoch10/lora/sft
template: glm4
finetuning_type: lora
### export
export_dir: models/CropLLM-glm-4-9b-chat
export_size: 2
export_device: cpu
export_legacy_format: false
半精度(FP16、BF16)
执行以下命令开始合并模型:
cd LLaMA-Factory
llamafactory-cli export crop_glm4_lora_sft_export.yaml
在 models/CropLLM-glm-4-9b-chat 目录中就可以获得经过Lora微调后的完整模型。
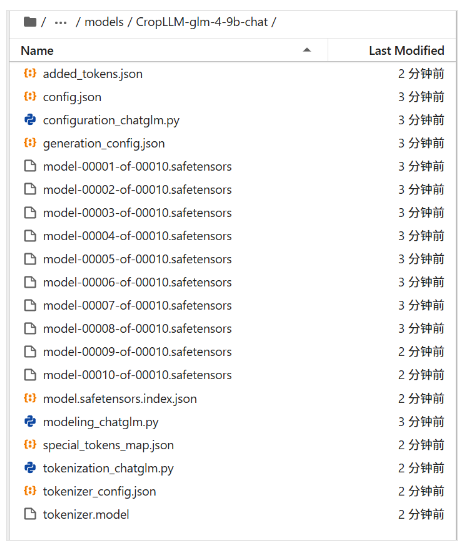
推理预测
py
import torch
from transformers import AutoModelForCausalLM, AutoTokenizer
model_path = "/home/featurize/LLaMA-Factory/models/CropLLM-glm-4-9b-chat"
device = "cuda"
tokenizer = AutoTokenizer.from_pretrained(model_path, trust_remote_code=True)
system_prompt = "你是农作物领域专门进行关系抽取的专家。请从给定的文本中抽取出关系三元组,不存在的关系返回空列表。请按照JSON字符串的格式回答。"
input = "玉米黑粉病又名"乌霉"和"瘤黑粉病",病原菌是真菌,:担孢子菌,是由于玉米黑粉菌所引起的一种局部浸染性病害。病瘤内的黑粉是病菌的"
inputs = tokenizer.apply_chat_template([{"role": "system", "content": system_prompt},
{"role": "user", "content": input}],
add_generation_prompt=True,
tokenize=True,
return_tensors="pt",
return_dict=True
)
inputs = inputs.to(device)
model = AutoModelForCausalLM.from_pretrained(
model_path,
torch_dtype=torch.bfloat16,
low_cpu_mem_usage=True,
trust_remote_code=True
).to(device).eval()
gen_kwargs = {"max_length": 2500, "do_sample": True, "top_k": 1}
with torch.no_grad():
outputs = model.generate(**inputs, **gen_kwargs)
outputs = outputs[:, inputs['input_ids'].shape[1]:]
print(f"model: {model_path}")
print(f"task: {system_prompt}")
print(f"input: {input}")
output = tokenizer.decode(outputs[0], skip_special_tokens=True)
print(f"output: {output}")