用AlphaFold进行非常精确的蛋白质结构的预测(AlphaFold2)
- 发表于2021年07月15日 Nature
- DOI: 10.1038/s41586-021-03819-2
- 自然和科学杂志评选为2021年最重要的科学突破之一
- 2021年AI在科学界最大的突破
前言
- 2020年11月30号, deepmind博客说AlphaFold解决了50年以来生物学的大挑战
- 2021年07月15日华盛顿大学的Protein Design团队在发布在8月15日将在Science上发表了一个RoseTTAFold, 使用深度神经网络进行蛋白质结构的预测
文章结构
- 摘要
- 导论: 一页半
- alphafold2: 两页出头, 模型介绍以及训练细节
- 结果分析: 一页
- 相关工作: 非常短
- 讨论
- 附录方法的细节
- SI: 50页, 详细的解释了每个模型里面的细节
摘要
-
问题
-
蛋白质对于生命来说是必要的, 了解蛋白质的结构有助于理解蛋白质的功能
-
蛋白质是长的氨基酸序列, 不稳定, 容易卷在一起, 从而形成独特的3d结构, 从而决定了蛋白质的功能
-
预测的困难(蛋白质折叠问题), 只知道很少一部分蛋白质的结构, 实验上通过冷冻方法观察费时费力
-
现有方法
-
AlphaFold1 精度不够, 不在原子的精度
-
AlphaFold2 能够达到原子的精度
-
AlphaFold2 使用了物理和生物学的知识, 也同样使用了深度学习
-
应用型的文章
-
问题对于领域来说重不重要
-
结果的好坏, 是不是解决了这个问题
-
找新问题或者开发新模型
In this study, we develop the first, to our knowledge, computational approach capable of predicting protein structures to near experimental accuracy in a majority of cases.
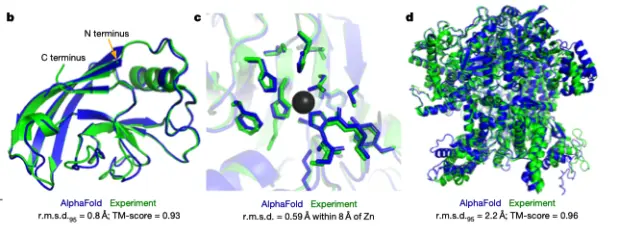
In pic, the blues are predicted from AlphaFlod, and the greens are experimental result.
The CASP assessment is carried out biennially using recently solved structures that have not been deposited in the PDB or publicly disclosed so that it is a blind test for the participating methods and has long served as the gold-standard assessment for the accuracy of structure prediction.
In CASP14, AlphaFold structures were vastly more accurate than competing methods, see pic below:
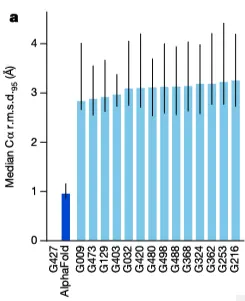
The AlphaFold network
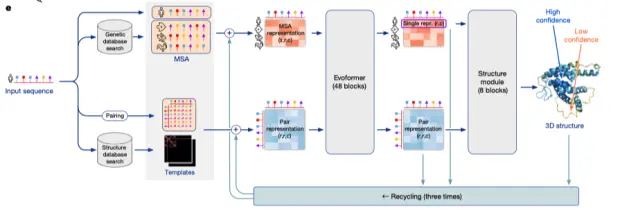
We divide the network into three parts of Feature extract、Encoder and Decoder.
Feature extract
The AlphaFold receives input features derived from the amino-acid sequence, MSA, and templates
MSA(multiple sequence alignments)
It's a common method used in bioinformatics.
The MSA is grouped by tool and ordered by the normal output of each tool, typically e-value. This means that similar sequences are more likely to be adjacent in the MSA and block deletions are more likely to generate diversity that removes whole branches of the phylogeny.
an N_{seq} × N_{res} array (N_{seq},number of sequences; N_{res} , number of residues) that represents a processed MSA.
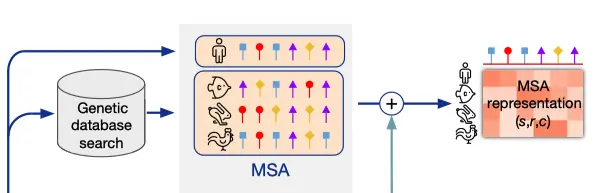
Templates
3D atom coordinates of a small number of homologous structures (templates) where available.
an N_{res} × N_{res} array that represents residue pairs.
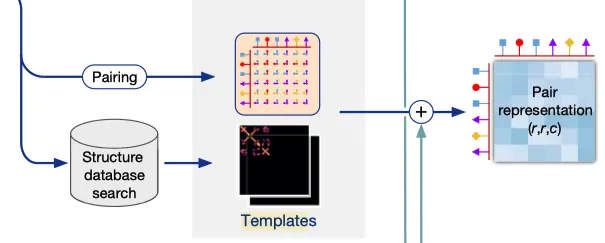
Evoformer
The Evoformer is based on transformer, the Evoformer blocks contain a number of attention-based and non-attention-based components.
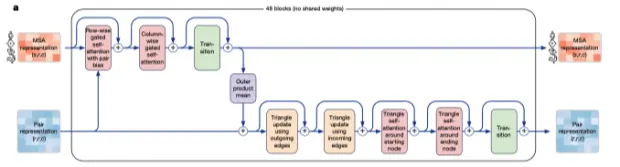
End-to-end structure prediction
The trunk of the network is followed by the structure module that introduces an explicit 3D structure in the form of a rotation and translation for each residue of the protein.
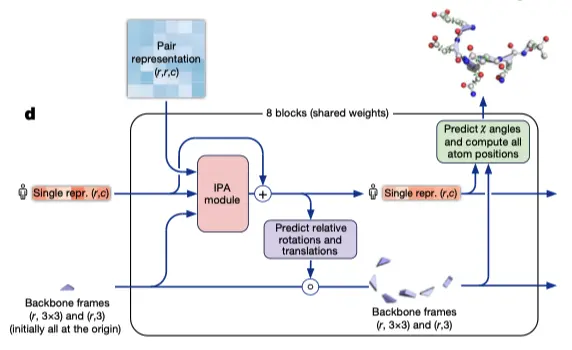
Prominent Work
Model Design
- Evoformer中输入的不是一个序列而是一个矩阵,通过组合Row-wise selfattention 和 Columnwise selfattention实现二维Transformer
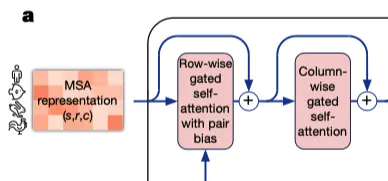
- 通过bias添加额外建模的信息
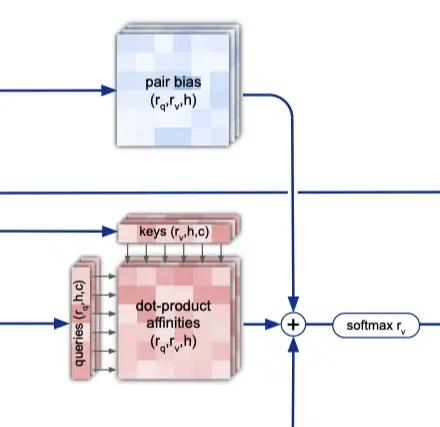
- 通过 linear + sigmod 乘矩阵实现gated控制输出权重
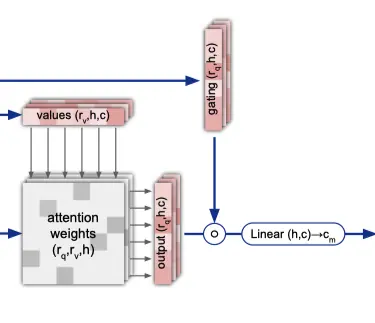
- Interpreting the neural network:循环使用模型,可以加深网络深度的同时不增大反向传播时的显存占用。使得整个模型很像RNN的结构
3d modeling
- 通过(R_k, t_k)进行刚体序列的相对位置表示,且该表示不受旋转平移等全局刚体变换的影响,同时在计算分数时显式的加入位置距离的计算。附录中含有对全局变换不变的证明。
Data augment
- Training with labelled and unlabelled data:对于无label数据集预测后选出置信度高的组成新的数据集添加噪声后与原有标号数据集组合重新用来训练,称为 noisy student self-distillation 。
- randomly mask out or mutate individual residues:类似BERT。
Train
- The initial training stage takes approximately 1 week, and the fine-tuning stage takes approximately 4 additional days.
- We train the model on Tensor Processing Unit (TPU) v3 with a batch size of 1 per TPU core, hence the model uses 128 TPU v3 cores.
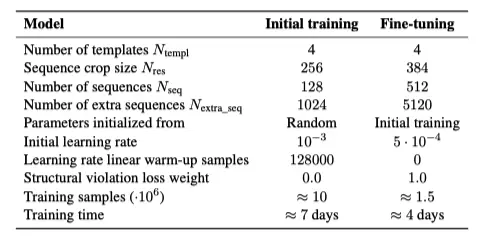
- 计算性能要求很高
The key words may help read
- residue - 氨基酸残基 这篇中可以理解为不同氨基酸,因为氨基酸差异体现在残基上
Reference
Highly accurate protein structure prediction with AlphaFold