Title
题目
Abductive multi-instance multi-label learning for periodontal diseaseclassification with prior domain knowledge
基于先验领域知识的归纳式多实例多标签学习用于牙周病分类
01
文献速递介绍
牙科疾病对口腔健康构成了重大挑战,影响了全球大量人口。根据世界卫生组织(WHO)2022年发布的《全球口腔健康状况报告》,估计全球约有35亿人受到口腔疾病的影响。其中,牙周炎引起了极大的关注,据报告约40%的30岁以上个体患有牙周炎(Eke等人,2016年),并且与糖尿病(Winning等人,2017年)等多种医疗状况相关联。因此,牙周炎的预防和诊断已成为现代医学研究中的重要课题,影响着数十亿人的健康。
根据不同的临床表现,牙周疾病可以分为多种类型,如牙龈炎和牙周炎。传统的牙周疾病诊断通常包括病史采集和临床检查,如测量牙周袋深度和评估牙槽骨丧失(Årtun和Urbye,1988年;Seabra等人,2008年;Ramachandra等人,2009年;Preshaw,2015年)。然而,这些方法通常具有侵入性,而且不同检查者的测量结果可能有所不同,取决于使用的探针(Osbom等人,1992年;Hefti,1997年)。因此,开发一种新方法以帮助标准化诊断过程是至关重要的。人工智能(AI)的出现彻底改变了牙科疾病的诊断。大量研究证明,人工智能在牙周疾病管理的多个方面具有潜力(Muresan等人,2020年;Farook等人,2023年;Cholan等人,2023年)。其他研究也探讨了机器学习在牙周诊断中的应用(Özden等人,2015年;Chen等人,2018年;Feres等人,2018年;Hung等人,2019年;Na等人,2020年;Farhadian等人,2020年)。尽管这些方法在早期的AI辅助牙周诊断中表现出了巨大潜力,但在处理高分辨率图像和现实医学场景时,它们的效果似乎有限。随着深度学习的兴起,已经开发了许多基于模型的方法,建立了一个端到端的诊断循环。口内彩色图像或X光片是通过深度学习模型进行准确评估的基本数据来源。然而,由于人类牙齿的固有结构,只有少数区域可能表现出明显的变化,指示炎症或疾病。因此,通常采用基于有效目标检测方法(如RCNN(Girshick等人,2014年;Girshick,2015年;Ren等人,2015年)和YOLO(Redmon等人,2016年;Redmon和Farhadi,2017年,2018年;Bochkovskiy等人,2020年;Wang等人,2023年))的二阶段过程。这种方法通常遵循以下框架:首先,检测模型从训练数据中学习提取并分类潜在受影响的区域;随后,这些区域(或其嵌入)作为输入,用于确定整个图像的标签(Chang等人,2020年;Li等人,2021年;Ding等人,2021年;ÖZİÇ等人,2023年)。利用深度学习模型的强大能力,这些方法在任务中取得了显著成果,其准确性和召回率与医生相当。尽管在牙周疾病分类方面表现出色,上述方法仍存在一些局限性。在疾病建模方面,缺乏整合牙周疾病类型和严重程度进行综合分析的研究。大多数研究将所有类型和程度的牙周疾病视为平行关系,忽略了分类标准中可能存在的层次性和序数关系。考虑到医生认可的四个主要类别:牙周健康(PH)、牙龈炎(GI)、轻度至中度牙周炎(MMP)和重度牙周炎(SP)。图1显示了四个类别的一些示例。通过专家知识,我们可以安全地将这些标签归结为两个维度:牙龈肿胀的程度和牙龈退缩的程度。原始标签与减少后的维度之间的关系如下:牙龈炎组表现为牙龈肿胀而没有牙龈退缩;轻度至中度牙周炎组表现为牙龈肿胀和轻度至中度牙龈退缩,而重度牙周炎组则表现为严重的牙龈退缩和牙龈肿胀。因此,很明显这些类别不能被视为完全独立的,而是需要新的建模方法来捕捉输入与类别之间复杂的关系。此外,牙周疾病的诊断通常需要全面考虑怀疑区域和邻近区域的牙周状况,而基于检测的方法往往未能完成这一任务。此外,基于检测的方法需要大量精细标注的数据,以准确定位可能的感兴趣区域(ROI)。这种细致的标注要求医生仔细而精确地检查每一张图像,识别所有潜在的受影响区域且不遗漏,这既困难又费时。此外,由于牙周结构在个体之间保持一致,受影响区域通常出现在特定区域,这对医生的专业知识依赖很大。不幸的是,现有方法未能有效地将这一关键的先验信息纳入训练过程中。为了解决这些问题,本文提出了一种新颖的推理多实例多标签学习(AB-MIML)方法,用于基于粗粒度标注数据进行牙周疾病的分类和诊断。在这一任务中,我们主要处理四种标签:牙周健康(PH)、牙龈炎(GI)、轻度至中度牙周炎(MMP)和重度牙周炎(SP)。为了建模输入与结果之间的复杂关系,我们采用了改进的多实例多标签方法。具体而言,我们将图像视为一个"袋",其中包含多个对应于较小补丁的"实例"。通过将输入图像划分为这些补丁,我们使用基于注意力的技术聚合补丁级预测,并将其映射到减少后的标签,以揭示细粒度的连接。此外,我们还将先验领域知识集成到分类过程中。这些相关的先验知识通过逻辑公式表达,形成一个知识库。随后,采用归纳推理推导出最可能的结果,纠正分类错误,并根据隐含的逻辑关系生成补丁级标签。由于在这一领域缺乏公开可用的数据集,我们收集并注释了来自南京口腔医院的数据集,以验证我们提出方法的有效性。实验结果表明,我们的方法在牙周疾病诊断中的表现优于现有方法。
Abatract
摘要
Machine learning is widely used in dentistry nowadays, offering efficient solutions for diagnosing dentaldiseases, such as periodontitis and gingivitis. Most existing methods for diagnosing periodontal diseases followa two-stage process. Initially, they detect and classify potential Regions of Interest (ROIs) and subsequentlydetermine the labels of the whole images. However, unlike the recognition of natural images, the diagnosisof periodontal diseases relies significantly on pinpointing specific affected regions, which requires professionalexpertise that is not fully captured by existing models. To bridge this gap, we propose a novel ABductive MultiInstance Multi-Label learning (AB-MIML) approach. In our approach, we treat entire intraoral images as ''bags''and local patches as ''instances''. By improving current multi-instance multi-label methods, AB-MIML seeks toestablish a comprehensive many-to-many relationship to model the intricate correspondence among images,patches, and corresponding labels. Moreover, to harness the power of prior domain knowledge, AB-MIMLconverts the expertise of doctors and the structural information of images into a knowledge base and performsabductive reasoning to assist the classification and diagnosis process. Experiments unequivocally confirm thesuperior performance of our proposed method in diagnosing periodontal diseases compared to state-of-theart approaches across various metrics. Moreover, our method proves invaluable in identifying critical areascorrelated with the diagnosis process, aligning closely with determinations made by human doctors.
如今,机器学习在牙科领域得到广泛应用,为牙科疾病的诊断提供了高效的解决方案,如牙周炎和牙龈炎。现有的大多数牙周疾病诊断方法遵循两阶段过程。首先,它们检测和分类潜在的兴趣区域(ROIs),然后确定整个图像的标签。然而,与自然图像的识别不同,牙周疾病的诊断在很大程度上依赖于精确定位受影响的特定区域,这需要专业的知识,而现有的模型并未完全捕捉到这一点。为弥补这一差距,我们提出了一种新颖的ABductive MultiInstance MultiLabel学习(AB-MIML)方法。在我们的方法中,我们将整个口腔图像视为"袋",局部图像块视为"实例"。通过改进现有的多实例多标签方法,AB-MIML旨在建立一个全面的多对多关系,以模拟图像、图像块和相应标签之间的复杂对应关系。此外,为了充分利用先验领域知识,AB-MIML将医生的专业知识和图像的结构信息转换为知识库,并执行归纳推理来辅助分类和诊断过程。实验结果明确确认了我们提出的方法在诊断牙周疾病方面,相较于最先进的方法,在多个指标上的优越表现。此外,我们的方法在识别与诊断过程相关的关键区域方面也证明了其不可或缺的价值,并且与医生的判断高度一致。
Method
方法
3.1. Problem formulation
Before diving into the details of our proposed method, we first provide a formalization of the problem. To classify and diagnose common{( periodontal 𝑋1 , 𝑌1 ) , ... diseases, , ( 𝑋𝑚, 𝑌𝑚 )} we . collect 𝑋𝑖 ⊆ a corresponds dataset in the to following the intraoral form: images =of patients, and 𝑌**𝑖 ⊆ ∈ [𝐶] = {1, ...,𝐶} are the associated labels.In periodontal disease detection, we have 𝐶 = 4 for the four classes,namely, periodontal health (PH), gingivitis (GI), mild to moderate periodontitis (MMP), and severe periodontitis (SP). The goal is to learn amapping 𝑓 ∶ → from , which is able to make accurate predictionsfor a new intraoral image sampled from the same distribution of .
3.1 问题表述
在深入讨论我们提出的方法之前,我们首先对问题进行形式化描述。为了分类和诊断常见的牙周疾病,假设我们收集到一组数据集,其中每个数据点包含一张患者的口腔图像和相应的标签。我们用 𝑋 表示患者的 intraoral 图像数据,𝑌 表示与之相关联的标签。在牙周疾病检测中,标签 𝑌 共有 4 类,分别为:牙周健康 (PH)、牙龈炎 (GI)、轻度到中度牙周炎 (MMP)、以及重度牙周炎 (SP)。目标是学习一个映射函数 𝑓 ∶ → ,使其能够对从同一分布中采样的新的口腔图像进行准确的预测。
Conclusion
结论
In this paper, we propose a novel ABductive Multi-Instance MultiLabel learning framework (AB-MIML) to tackle the complex issue ofperiodontal disease classification. By leveraging the MIML paradigm,we model the intricate correspondence between images and their corresponding labels, which allows for a many-to-many mapping relationship. Meanwhile, the abduction component integrates expert knowledge expressed by logical formulas into the training and reasoningprocess, in order to help machine learning make better perceptions.Experimental results demonstrate that our framework achieves SOTAperformance in our problem setting, and can be beneficial in numerouspractical applications.
在本文中,我们提出了一种新颖的归纳多实例多标签学习框架(AB-MIML),旨在解决牙周疾病分类中的复杂问题。通过利用多实例多标签(MIML)范式,我们建模了图像与相应标签之间的复杂对应关系,从而实现了多对多的映射关系。同时,归纳组件将通过逻辑公式表达的专家知识集成到训练和推理过程中,帮助机器学习更好地理解和感知。实验结果表明,我们的框架在问题设置中达到了最先进的性能,并在多个实际应用中具有潜在的优势。
Figure
图
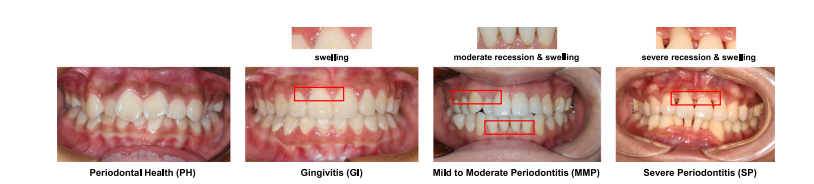
Fig. 1. Example images of four concerned categories of periodontal diseases. The red boxes highlight the typical affected patches, such as the swelling area of GI, the moderaterecession & swelling area of MMP, and the severe recession & swelling area of SP. They are all located in some specific local patches of the original images
图 1. 四种牙周疾病相关类别的示例图像。红色框突出显示了典型的受影响区域,如牙龈炎(GI)的肿胀区域、牙龈退缩和肿胀的中度区域(MMP),以及牙周炎(SP)的严重退缩和肿胀区域。它们都位于原始图像的特定局部区域。
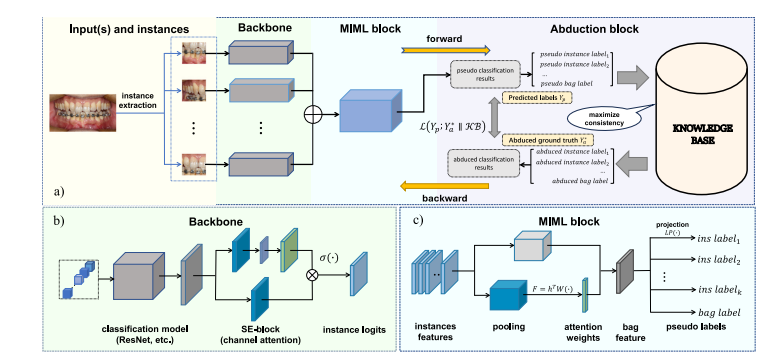
Fig. 2. The detailed illustration of our proposed Abductive Multi-Instance Multi-Label Learning (AB-MIML) framework for periodontal disease classification. Fig. 2 (b) and Fig. 2(c) shows the details of the Backbone and MIML block respectively
图 2. 我们提出的推理多实例多标签学习(AB-MIML)框架在牙周疾病分类中的详细说明。图 2(b)和图 2(c)分别展示了骨干网络(Backbone)和多实例多标签(MIML)模块的细节。
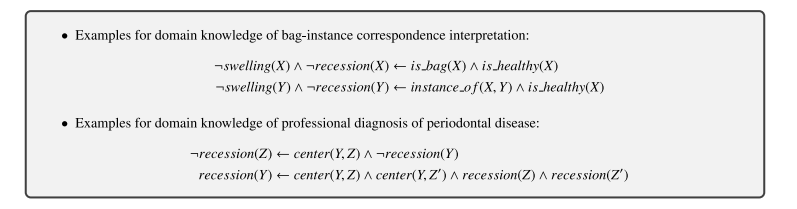
Fig. 3. Sample formulas in the knowledge base
图 3. 知识库中的示例公式
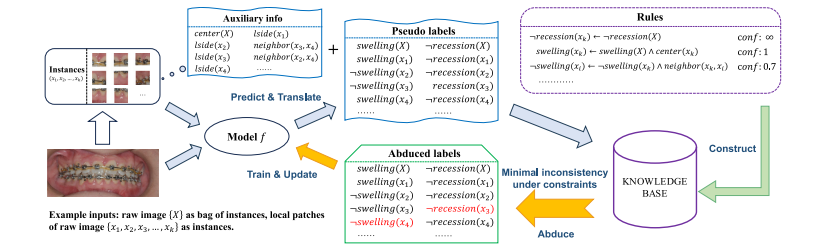
Fig. 4. The detailed illustration of the abduction process. The pseudo labels are first translated into logical variables and facts. Then we try to maximize the consistency betweenpseudo labels and the knowledge base by modifying some of the labels under cost constraints. For example, according to the logical rules, the bag-level annotation ''no recession''means that all instances shall not show signs of recession, so the ''recession'' label of 𝑥3 is modified. Also, according to the rules, since the instances around the center of theimage are more likely to show signs of affection, as well as all neighbors show no signs of swelling, the label ''swelling'' of 𝑥4 is modified, which is consistent with the groundtruth
Fig. 4. 归纳过程的详细示意图。伪标签首先被转换为逻辑变量和事实。然后,我们在成本约束下,通过修改一些标签,最大化伪标签与知识库之间的一致性。例如,根据逻辑规则,袋级注释 "无退缩" 表示所有实例都不应显示退缩迹象,因此 𝑥3 的 "退缩" 标签被修改。另外,根据规则,由于图像中心周围的实例更有可能显示受损迹象,并且所有邻近区域都没有肿胀迹象,因此 𝑥4 的 "肿胀" 标签被修改,这与真实标签一致。
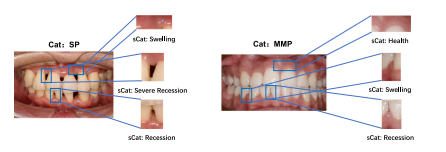
Fig. 5. Examples of multi-instance categories of periodontal diseases. Cat. is short forcategory, and sCat. stands for decomposed sub-labels.
Fig. 5. 牙周疾病的多实例类别示例。Cat. 是类别的缩写,sCat. 代表分解的子标签。
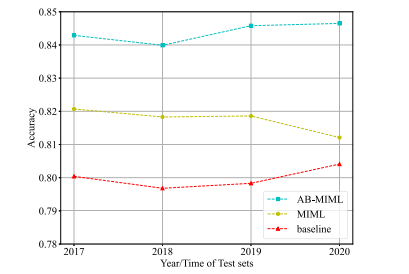
Fig. 6. An illustration of average performance of different methods across test datafrom 2017 to 2020.
Fig. 6. 展示了不同方法在2017年至2020年测试数据上的平均表现。
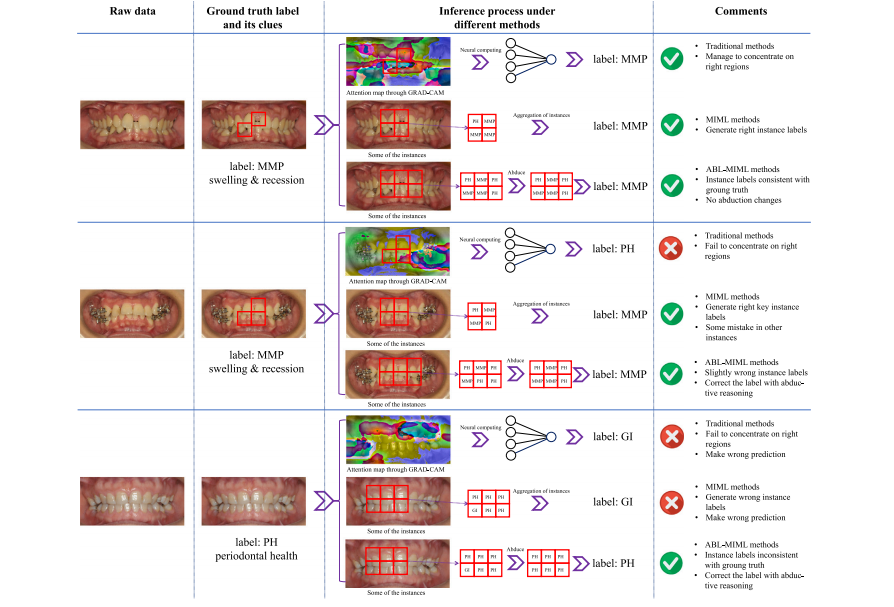
Fig. 7. Some typical examples to illustrate how our AB-MIML framework works. We select three examples, and each group shows the inference process of the base, MIML andAB-MIML methods respectively. The ''comments'' part contains additional descriptions on what occurs during the inference process.
Fig. 7. 一些典型示例,展示我们的AB-MIML框架如何工作。我们选择了三个示例,每组展示了基础方法、MIML方法和AB-MIML方法的推理过程。 ''评论''部分包含了关于推理过程中发生的事情的额外描述。
Table
表
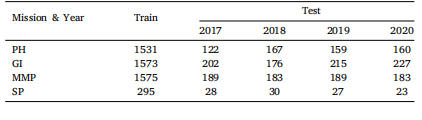
Table 1Detailed information of datasets. The training set is collected from the whole time span,while the test sets are organized by year.
Table 1 数据集的详细信息。训练集收集了整个时间跨度的数据,而测试集则按年份组织。
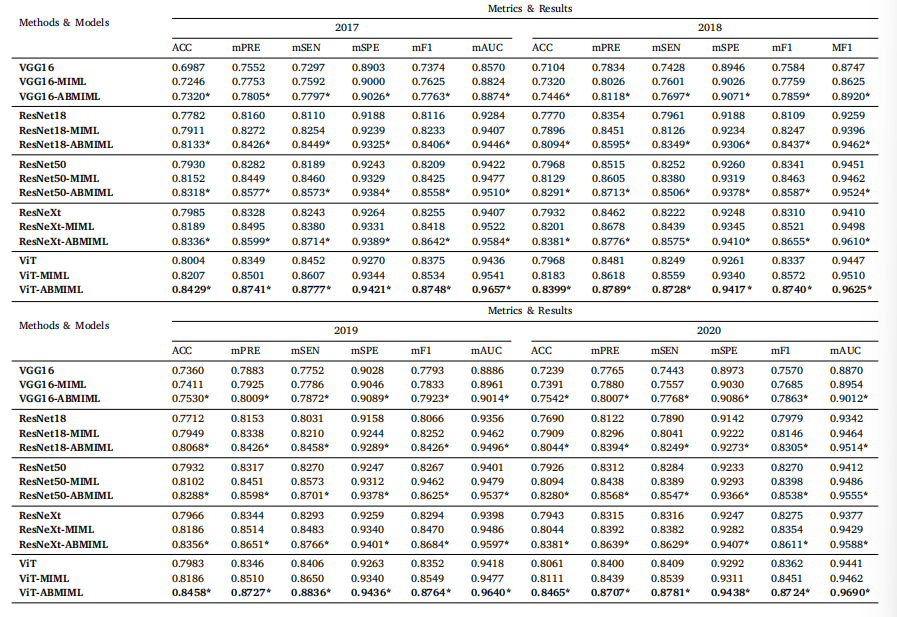
Table 2Experimental results of different methods and models evaluated on several main metrics from 2017 to 2020. We assume that the labels of the original images are ground truth.The results are organized by groups of backbones and times.
Table 2 展示了在2017年至2020年测试数据上,使用不同方法和模型进行评估的实验结果。我们假设原始图像的标签为真实标签。结果按主干网络和时间组进行组织。

Table 3A brief exhibition of experimental results of our proposed method compared with the detection models. The backbone models in the experiment are all implemented using thewell-established versions.
Table 3 简要展示了我们提出的方法与检测模型的实验结果比较。实验中的主干模型都使用了经过验证的版本实现。

Table 4Experimental results of pretrained and non-pretrained models. To be brief, we select two representative backbones to demonstrate the effectiveness of our approach. The 'NaN' inthe table indicates that the combination of methods can hardly converge to a feasible solution.
Table 4 预训练模型与非预训练模型的实验结果。为简洁起见,我们选择了两个具有代表性的主干模型来展示我们方法的有效性。表中的"NaN"表示方法组合几乎无法收敛到可行的解决方案。

Table 5A brief overview of experimental results of different methods evaluated on several key metrics of test datasets. We assume that there is a certain ratio of noise in the bag-levelannotation. The methods evaluated using a pretrained backbone are identified by the suffix ''(P)''. The ''NaN'' in the table indicates that the model can hardly converge to afeasible solution under such settings.
Table 5 各种方法在测试数据集的几个关键指标上的实验结果概览。我们假设袋级标注存在一定比例的噪声。使用预训练主干模型的评估方法通过后缀"(P)"标识。表中的"NaN"表示在这种设置下,模型几乎无法收敛到可行的解决方案。

Table 6Experimental results for class-wise analysis. The models used in the experiment are all pretrained
Table 6 类别分析的实验结果。实验中使用的模型均为预训练模型。