前言
最近在学习实例分割算法,参考b站视频课教程,使用labelme标注数据集,在csdn找到相关教程进行数据集格式转换,按照相关目标检测网络对数据集格式的训练要求划分数据集。
1.使用labelme标注图片
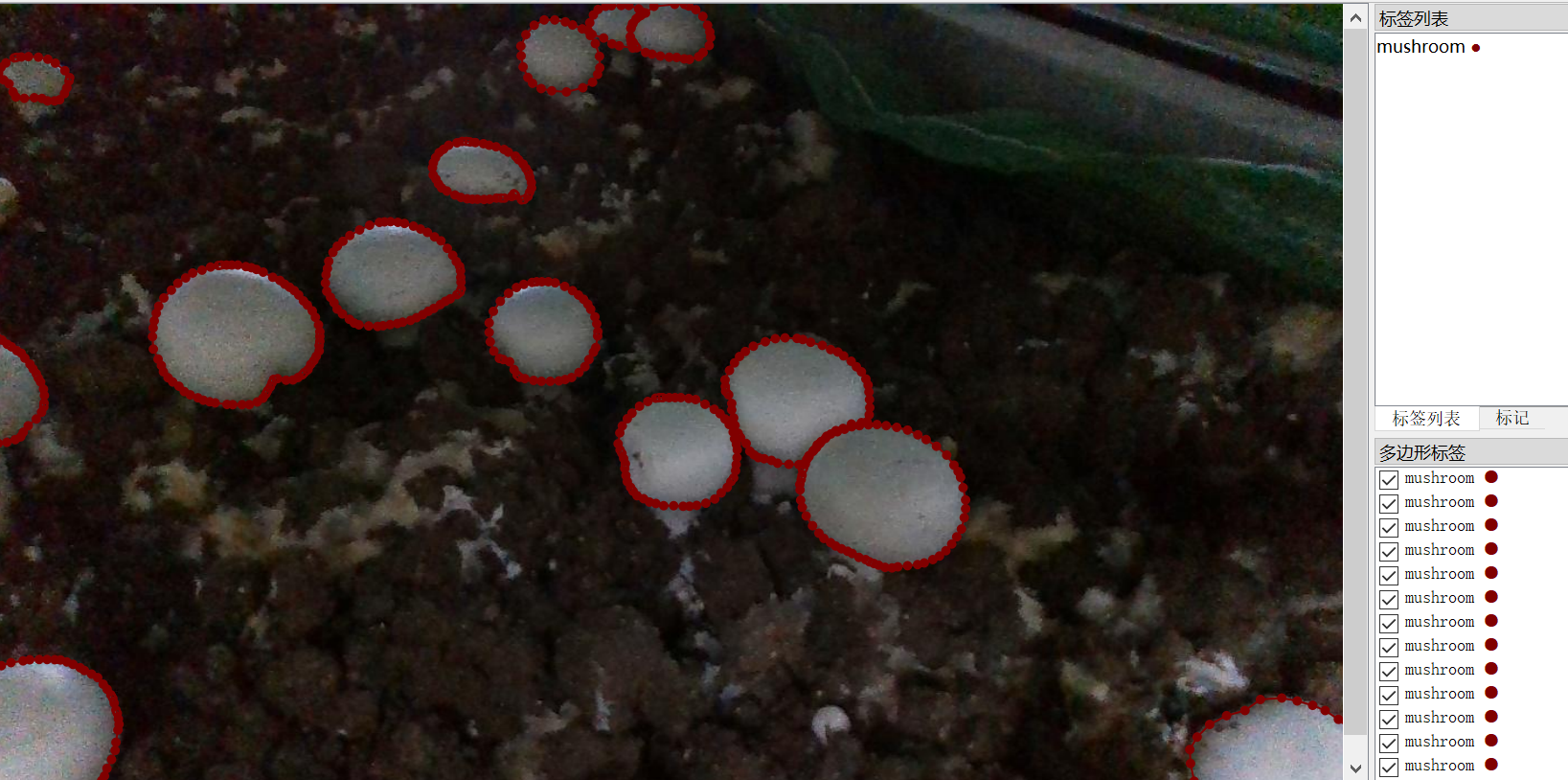
在网上随便找了几张蘑菇图片,使用多边形进行标注,标签设置为mushroom,
注意:实例分割标注疑惑:一张图片同类物体不同实例,如图片中显示多个蘑菇,该标注为mushroom1, mushroom2,mushroom3;或者全都标注为mushroom
在查看了一些实例相关的文献,发现以上两种情况均存在
在使用maskrcnn的文献中,一些是同类物体不同实例:显示不同掩膜颜色; 而另一些是同类物体不同实例:显示相同掩膜颜色。
最终决定选择全部标注为mushroom的原因:
1.参考github官方labelme的实例分割标注示例
E:\Github\github\labelme-main\examples\instance_segmentation\data_annotated
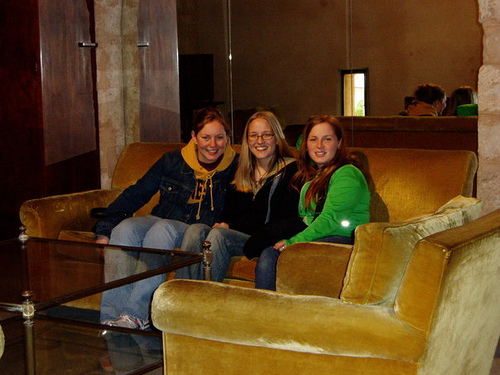
图片为2011_000006.jpg
labelme json标注信息,可以看到图片中有三个人,在json标签中三个实例同一种类(人)label均为person, 因此说明label指代是类别标签,而不是实例标签, 如果标注为person1,perosn2,person3,则是说明有三种不同的类别,如果再标注另一张带有人的标签,也标注为perosn1,perosn2,则会出现 每张图片不同的人,都有一个person1, 但是实际上他们类别都是person。 因此这样标显然不合理。
python
{
"version": "4.0.0",
"flags": {},
"shapes": [
{
"label": "person",
"points": [
[
204.936170212766,
108.56382978723406
],
[
183.936170212766,
141.56382978723406
],
[
166.936170212766,
150.56382978723406
],
[
108.93617021276599,
203.56382978723406
],
[
92.93617021276599,
228.56382978723406
],
[
95.93617021276599,
244.56382978723406
],
[
105.93617021276599,
244.56382978723406
],
[
116.93617021276599,
223.56382978723406
],
[
163.936170212766,
187.56382978723406
],
[
147.936170212766,
212.56382978723406
],
[
117.93617021276599,
222.56382978723406
],
[
108.93617021276599,
243.56382978723406
],
[
100.93617021276599,
325.56382978723406
],
[
135.936170212766,
329.56382978723406
],
[
148.936170212766,
319.56382978723406
],
[
150.936170212766,
295.56382978723406
],
[
169.936170212766,
272.56382978723406
],
[
171.936170212766,
249.56382978723406
],
[
178.936170212766,
246.56382978723406
],
[
186.936170212766,
225.56382978723406
],
[
214.936170212766,
219.56382978723406
],
[
242.936170212766,
157.56382978723406
],
[
228.936170212766,
146.56382978723406
],
[
228.936170212766,
125.56382978723406
],
[
216.936170212766,
112.56382978723406
]
],
"group_id": null,
"shape_type": "polygon",
"flags": {}
},
{
"label": "person",
"points": [
[
271.936170212766,
109.56382978723406
],
[
249.936170212766,
110.56382978723406
],
[
244.936170212766,
150.56382978723406
],
[
215.936170212766,
219.56382978723406
],
[
208.936170212766,
245.56382978723406
],
[
214.936170212766,
220.56382978723406
],
[
188.936170212766,
227.56382978723406
],
[
170.936170212766,
246.56382978723406
],
[
170.936170212766,
275.56382978723406
],
[
221.936170212766,
278.56382978723406
],
[
233.936170212766,
259.56382978723406
],
[
246.936170212766,
253.56382978723406
],
[
245.936170212766,
256.56382978723406
],
[
242.936170212766,
251.56382978723406
],
[
262.936170212766,
256.56382978723406
],
[
304.936170212766,
226.56382978723406
],
[
297.936170212766,
199.56382978723406
],
[
308.936170212766,
164.56382978723406
],
[
296.936170212766,
148.56382978723406
]
],
"group_id": null,
"shape_type": "polygon",
"flags": {}
},
{
"label": "person",
"points": [
[
308.936170212766,
115.56382978723406
],
[
298.936170212766,
145.56382978723406
],
[
309.936170212766,
166.56382978723406
],
[
297.936170212766,
200.56382978723406
],
[
305.936170212766,
228.56382978723406
],
[
262.936170212766,
258.56382978723406
],
[
252.936170212766,
284.56382978723406
],
[
272.936170212766,
291.56382978723406
],
[
281.936170212766,
250.56382978723406
],
[
326.936170212766,
235.56382978723406
],
[
351.936170212766,
239.56382978723406
],
[
365.936170212766,
223.56382978723406
],
[
371.936170212766,
187.56382978723406
],
[
353.936170212766,
168.56382978723406
],
[
344.936170212766,
143.56382978723406
],
[
336.936170212766,
115.56382978723406
]
],
"group_id": null,
"shape_type": "polygon",
"flags": {}
},
{
"label": "chair",
"points": [
[
309.7054009819968,
242.94844517184941
],
[
282.7054009819968,
251.94844517184941
],
[
271.7054009819968,
287.9484451718494
],
[
175.70540098199677,
275.9484451718494
],
[
149.70540098199677,
296.9484451718494
],
[
151.70540098199677,
319.9484451718494
],
[
160.70540098199677,
328.9484451718494
],
[
165.54250204582655,
375.38461538461536
],
[
486.7054009819968,
373.9484451718494
],
[
498.7054009819968,
336.9484451718494
],
[
498.7054009819968,
202.94844517184941
],
[
454.7054009819968,
193.94844517184941
],
[
435.7054009819968,
212.94844517184941
],
[
368.7054009819968,
224.94844517184941
],
[
351.7054009819968,
241.94844517184941
]
],
"group_id": null,
"shape_type": "polygon",
"flags": {}
},
{
"label": "person",
"points": [
[
425.936170212766,
82.56382978723406
],
[
404.936170212766,
109.56382978723406
],
[
400.936170212766,
114.56382978723406
],
[
437.936170212766,
114.56382978723406
],
[
448.936170212766,
102.56382978723406
],
[
446.936170212766,
91.56382978723406
]
],
"group_id": null,
"shape_type": "polygon",
"flags": {}
},
{
"label": "__ignore__",
"points": [
[
457.936170212766,
85.56382978723406
],
[
439.936170212766,
117.56382978723406
],
[
477.936170212766,
117.56382978723406
],
[
474.936170212766,
87.56382978723406
]
],
"group_id": null,
"shape_type": "polygon",
"flags": {}
},
{
"label": "sofa",
"points": [
[
183.936170212766,
140.56382978723406
],
[
125.93617021276599,
140.56382978723406
],
[
110.93617021276599,
187.56382978723406
],
[
22.936170212765987,
199.56382978723406
],
[
18.936170212765987,
218.56382978723406
],
[
22.936170212765987,
234.56382978723406
],
[
93.93617021276599,
239.56382978723406
],
[
91.93617021276599,
229.56382978723406
],
[
110.93617021276599,
203.56382978723406
]
],
"group_id": 0,
"shape_type": "polygon",
"flags": {}
},
{
"label": "sofa",
"points": [
[
103.93617021276599,
290.56382978723406
],
[
58.93617021276599,
303.56382978723406
],
[
97.93617021276599,
311.56382978723406
]
],
"group_id": 0,
"shape_type": "polygon",
"flags": {}
},
{
"label": "sofa",
"points": [
[
348.936170212766,
146.56382978723406
],
[
472.936170212766,
149.56382978723406
],
[
477.936170212766,
162.56382978723406
],
[
471.936170212766,
196.56382978723406
],
[
453.936170212766,
192.56382978723406
],
[
434.936170212766,
213.56382978723406
],
[
368.936170212766,
226.56382978723406
],
[
375.936170212766,
187.56382978723406
],
[
353.936170212766,
164.56382978723406
]
],
"group_id": 0,
"shape_type": "polygon",
"flags": {}
},
{
"label": "sofa",
"points": [
[
246.936170212766,
252.56382978723406
],
[
219.936170212766,
277.56382978723406
],
[
254.936170212766,
287.56382978723406
],
[
261.936170212766,
256.56382978723406
]
],
"group_id": 0,
"shape_type": "polygon",
"flags": {}
}
],
"imagePath": "2011_000006.jpg",
"imageData": null,
"imageHeight": 375,
"imageWidth": 500
}
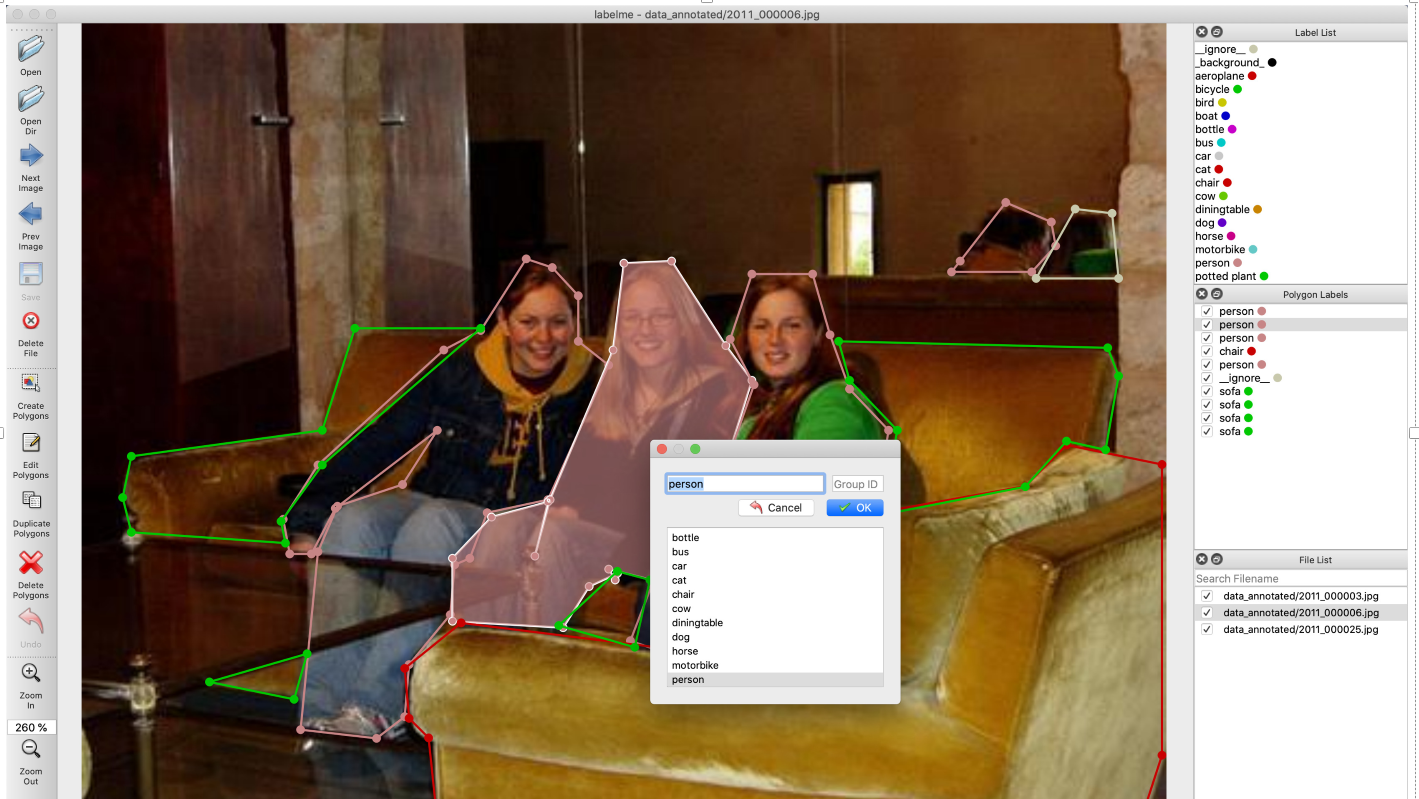
在github/labelme的官网中,作者是按上图进行标注的,三个person
2.github官方标注实例中voc数据集和coco数据集
labelme/voc数据集格式
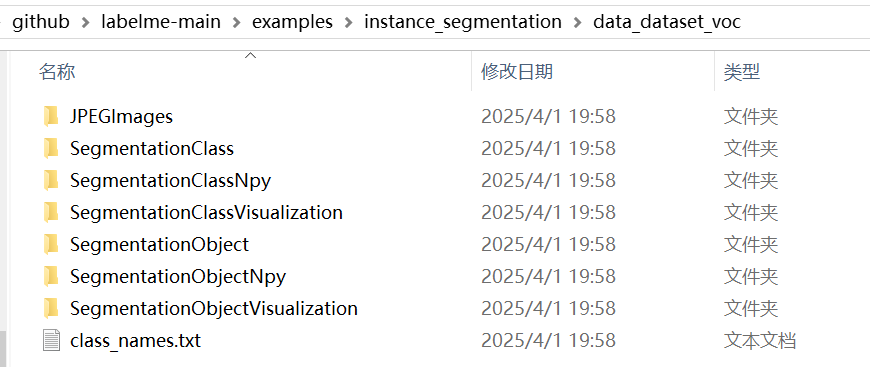
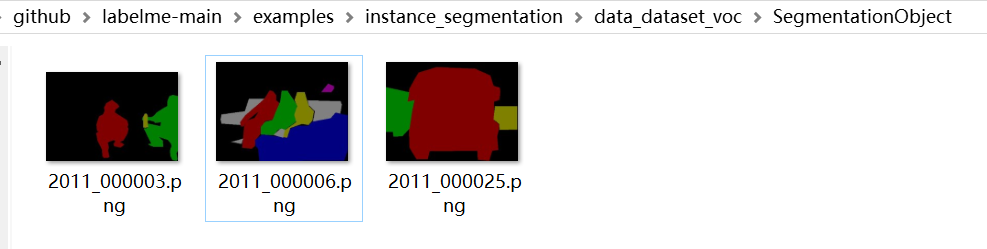
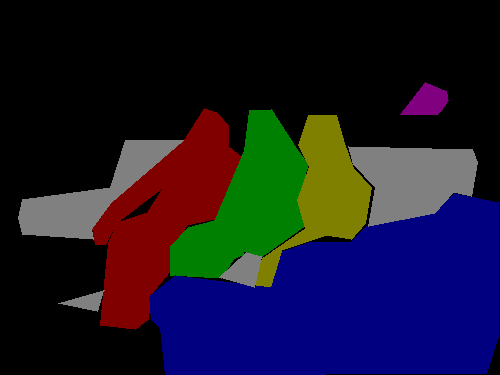
在github\labelme-main\examples\instance_segmentation\data_dataset_voc\SegmentationObject
文件中可以看到,实例分割标签中,三个人掩膜分别是不同的颜色
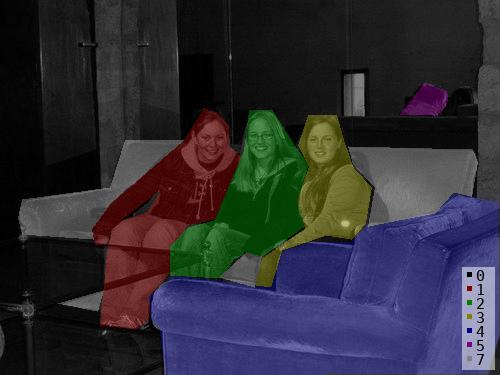
文件github\labelme-main\examples\instance_segmentation\data_dataset_voc\SegmentationObjectVisualization
在可视化实例分割标签中,可以看到不同人对应不同的数字和颜色
(红色1,绿色2,黄色3)
labelme/coco数据集格式
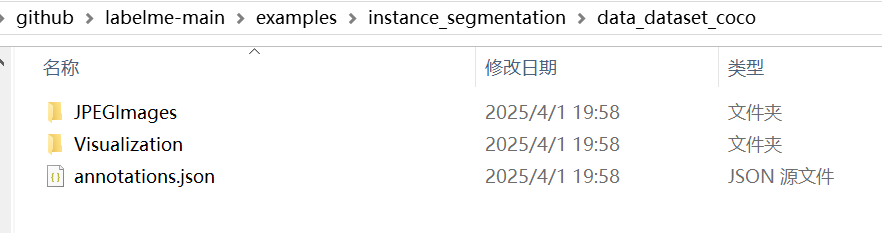
在github\labelme-main\examples\instance_segmentation\data_dataset_coco\Visualization文件夹中可以看到三个人,掩膜显示不同的颜色,标签均显示为person
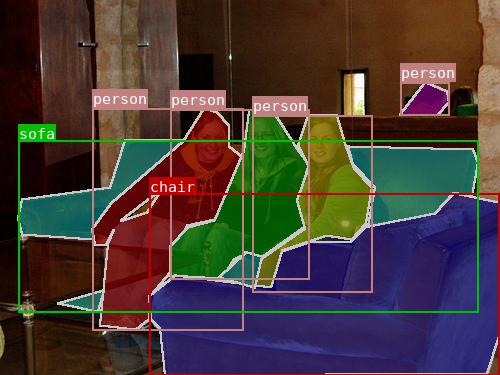
查看其annotations.json 标签文件,发现label 也都是person, 那怎么区分不同实例呢?
发现它这里是用id 来区分不同实例,每个id 代表不同种类不同实例的一个独立物体编号(不考虑背景)
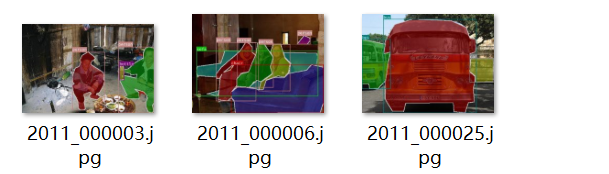
观察该图片,结合 annotations.json 标签文件分析
|-----------------|----------|-------------------------|--------------------------|
| 图片及其 | image_id | 物体 | annotations.json 中实例对应id |
| 2011_000003.jpg | 0 | (category_id=15) person | 0 |
| | | person | 1 |
| | | (category_id=5) bottel | 2 |
| 2011_000025.jpg | 1 | (category_id=6) bus | 3 |
| | | bus | 4 |
| | | (category_id=7) car | 5 |
| 2011_000006.jpg | 2 | person | 6 |
| | | person | 7 |
| | | person | 8 |
| | | (category_id=9) chair | 9 |
| | | person | 10 |
| | | (category_id=18) sofa | 11 |
| | | | |
注意:group_id
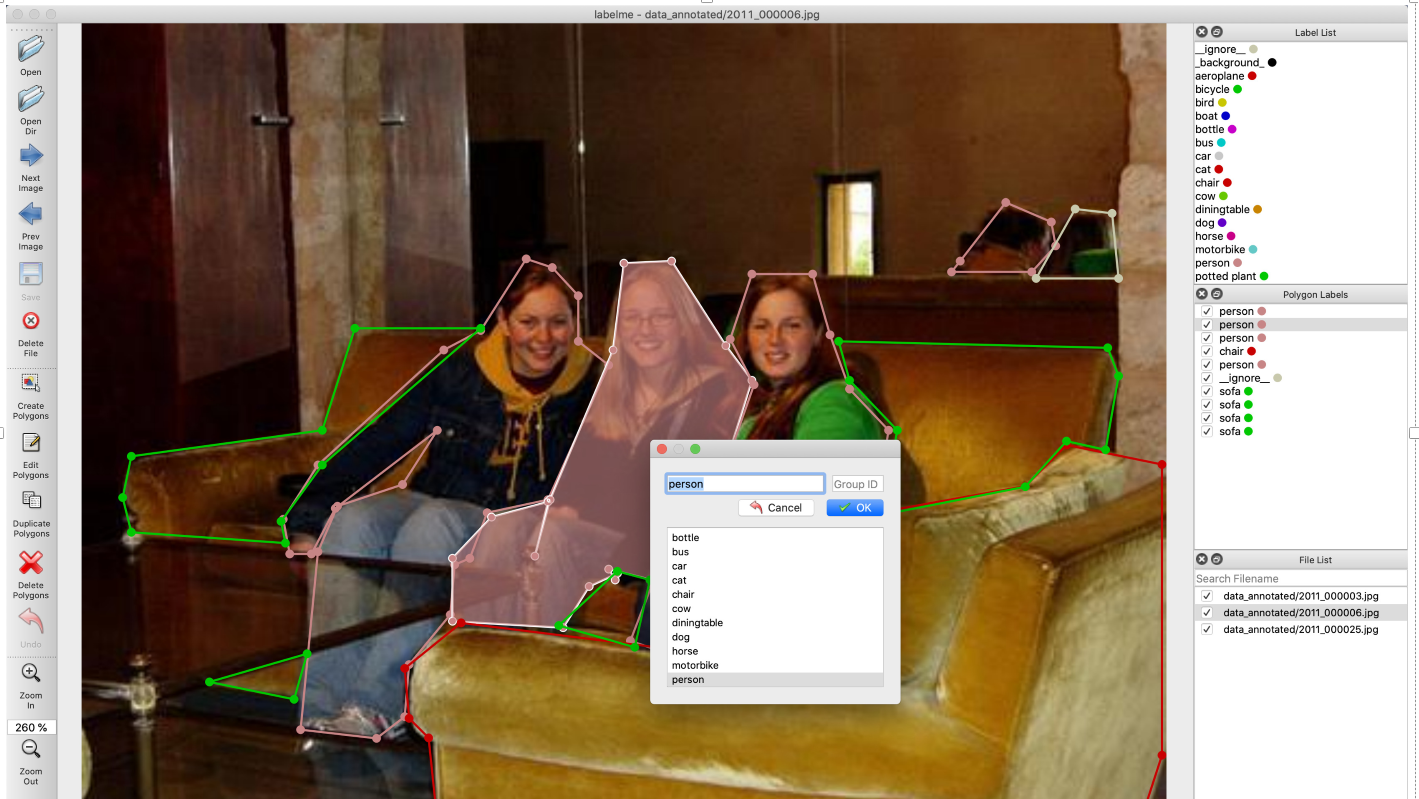
labelme标注生成的json标签文件中,可以看到4个 label为sofa, 并且group_id 均为0, 这表示这4个标注部分均属于同一个实例,而不是四个相互独立的实例(因为图片显示只有一张沙发,但是由于人遮挡关系,被分割为4个部分)
在voc数据集格式实例分割标签.png中,可以看到sofa的4个部分是同一个颜色(灰色),均属于图片中第7个实例(背景是第0个实例)
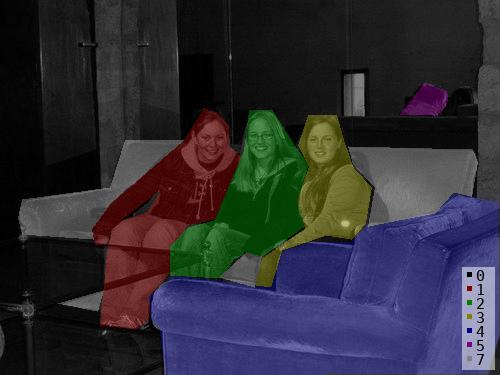
在coco数据集格式annotations.json文件中可以看到, 关于sofa的标注信息,内部使用四个分割列表组成,说明该实例对象有被遮挡,而成为几个不连贯的部分; 而其它实例对象是由一个分割列表组成,说明该实例对象没有被遮挡,内部连贯。
python
{"id": 11, "image_id": 2, "category_id": 18, "segmentation":
[[183.936170212766, 140.56382978723406, 125.93617021276599, 140.56382978723406, 110.93617021276599, 187.56382978723406, 22.936170212765987, 199.56382978723406, 18.936170212765987, 218.56382978723406, 22.936170212765987, 234.56382978723406, 93.93617021276599, 239.56382978723406, 91.93617021276599, 229.56382978723406, 110.93617021276599, 203.56382978723406],
[103.93617021276599, 290.56382978723406, 58.93617021276599, 303.56382978723406, 97.93617021276599, 311.56382978723406],
[348.936170212766, 146.56382978723406, 472.936170212766, 149.56382978723406, 477.936170212766, 162.56382978723406, 471.936170212766, 196.56382978723406, 453.936170212766, 192.56382978723406, 434.936170212766, 213.56382978723406, 368.936170212766, 226.56382978723406, 375.936170212766, 187.56382978723406, 353.936170212766, 164.56382978723406],
[246.936170212766, 252.56382978723406, 219.936170212766, 277.56382978723406, 254.936170212766, 287.56382978723406, 261.936170212766, 256.56382978723406]],
"area": 14001.0, "bbox": [18.0, 140.0, 460.0, 172.0],
"iscrowd": 0}
使用labelme自带的格式转换代码进行格式转换
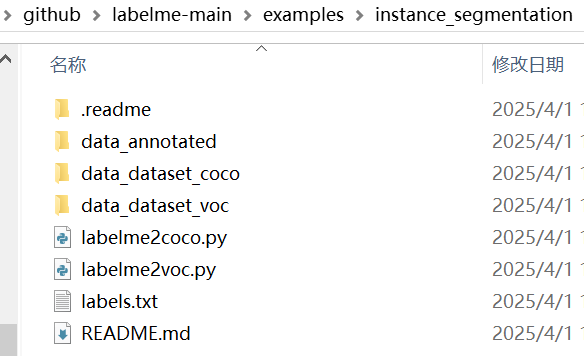
在github\labelme-main\examples\instance_segmentation 文件夹下,
有两个py文件
|-----------------|-------------------------------|
| labelme2coco.py | 将labelme标注的json标签转换为coco数据集格式 |
| labelme2voc.py | 将labelme标注的json标签转换为voc数据集格式 |
labelme2voc.py使用

在使用labelme-多边形标注完图片后,我们将得到 图片jpg,以及 标签json (存放于data_annotated文件夹)
在转化前,需要再建立一个labels.txt文件,存放类别
注意:如果标注多个类别,该如何排序,观察labelme给示例,发现类别的排序与 coco数据集格式的 supercategory 的ID 序号对应。
labels.txt内容如下,background 对应序号supercategory_id 为0, person 对应supercategory_id 为15 。
python
__ignore__
_background_
aeroplane
bicycle
bird
boat
bottle
bus
car
cat
chair
cow
diningtable
dog
horse
motorbike
person
potted plant
sheep
sofa
train
tv/monitor
coco数据集 annotations.json文件内容
python
"categories": [{"supercategory": null, "id": 0, "name": "_background_"}, {"supercategory": null, "id": 1, "name": "aeroplane"}, {"supercategory": null, "id": 2, "name": "bicycle"}, {"supercategory": null, "id": 3, "name": "bird"}, {"supercategory": null, "id": 4, "name": "boat"}, {"supercategory": null, "id": 5, "name": "bottle"}, {"supercategory": null, "id": 6, "name": "bus"}, {"supercategory": null, "id": 7, "name": "car"}, {"supercategory": null, "id": 8, "name": "cat"}, {"supercategory": null, "id": 9, "name": "chair"}, {"supercategory": null, "id": 10, "name": "cow"}, {"supercategory": null, "id": 11, "name": "diningtable"}, {"supercategory": null, "id": 12, "name": "dog"}, {"supercategory": null, "id": 13, "name": "horse"}, {"supercategory": null, "id": 14, "name": "motorbike"}, {"supercategory": null, "id": 15, "name": "person"}, {"supercategory": null, "id": 16, "name": "potted plant"}, {"supercategory": null, "id": 17, "name": "sheep"}, {"supercategory": null, "id": 18, "name": "sofa"}, {"supercategory": null, "id": 19, "name": "train"}, {"supercategory": null, "id": 20, "name": "tv/monitor"}]}
因此,创建labels.txt文件内容如下:
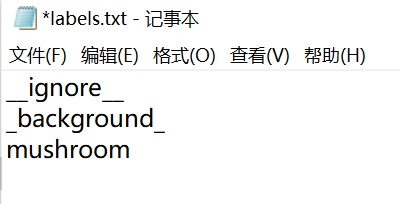
将下面四个文件存放在同一个文件夹, 程序py文件从github\labelme-main\examples\instance_segmentation 复制
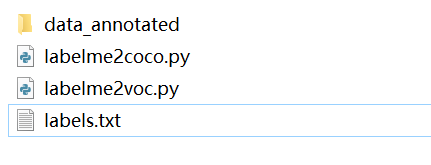
上面四个文件存放于文件夹test, 在该文件夹下打开终端, 激活虚拟环境,输入指令
python
python labelme2voc.py data_annotated data_dataset_voc --labels labels.txt
即可得到 VOC数据集格式
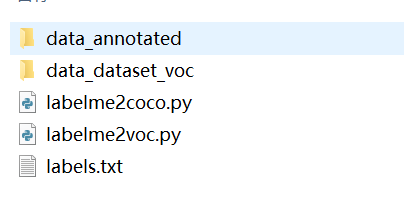
labelme2voc.py代码
python
#!/usr/bin/env python
from __future__ import print_function
import argparse
import glob
import os
import os.path as osp
import sys
import imgviz
import numpy as np
import labelme
def main():
parser = argparse.ArgumentParser(
formatter_class=argparse.ArgumentDefaultsHelpFormatter
)
parser.add_argument("input_dir", help="Input annotated directory")
parser.add_argument("output_dir", help="Output dataset directory")
parser.add_argument(
"--labels", help="Labels file or comma separated text", required=True
)
parser.add_argument(
"--noobject", help="Flag not to generate object label", action="store_true"
)
parser.add_argument(
"--nonpy", help="Flag not to generate .npy files", action="store_true"
)
parser.add_argument(
"--noviz", help="Flag to disable visualization", action="store_true"
)
args = parser.parse_args()
if osp.exists(args.output_dir):
print("Output directory already exists:", args.output_dir)
sys.exit(1)
os.makedirs(args.output_dir)
os.makedirs(osp.join(args.output_dir, "JPEGImages"))
os.makedirs(osp.join(args.output_dir, "SegmentationClass"))
if not args.nonpy:
os.makedirs(osp.join(args.output_dir, "SegmentationClassNpy"))
if not args.noviz:
os.makedirs(osp.join(args.output_dir, "SegmentationClassVisualization"))
if not args.noobject:
os.makedirs(osp.join(args.output_dir, "SegmentationObject"))
if not args.nonpy:
os.makedirs(osp.join(args.output_dir, "SegmentationObjectNpy"))
if not args.noviz:
os.makedirs(osp.join(args.output_dir, "SegmentationObjectVisualization"))
print("Creating dataset:", args.output_dir)
if osp.exists(args.labels):
with open(args.labels) as f:
labels = [label.strip() for label in f if label]
else:
labels = [label.strip() for label in args.labels.split(",")]
class_names = []
class_name_to_id = {}
for i, label in enumerate(labels):
class_id = i - 1 # starts with -1
class_name = label.strip()
class_name_to_id[class_name] = class_id
if class_id == -1:
assert class_name == "__ignore__"
continue
elif class_id == 0:
assert class_name == "_background_"
class_names.append(class_name)
class_names = tuple(class_names)
print("class_names:", class_names)
out_class_names_file = osp.join(args.output_dir, "class_names.txt")
with open(out_class_names_file, "w") as f:
f.writelines("\n".join(class_names))
print("Saved class_names:", out_class_names_file)
for filename in sorted(glob.glob(osp.join(args.input_dir, "*.json"))):
print("Generating dataset from:", filename)
label_file = labelme.LabelFile(filename=filename)
base = osp.splitext(osp.basename(filename))[0]
out_img_file = osp.join(args.output_dir, "JPEGImages", base + ".jpg")
out_clsp_file = osp.join(args.output_dir, "SegmentationClass", base + ".png")
if not args.nonpy:
out_cls_file = osp.join(
args.output_dir, "SegmentationClassNpy", base + ".npy"
)
if not args.noviz:
out_clsv_file = osp.join(
args.output_dir,
"SegmentationClassVisualization",
base + ".jpg",
)
if not args.noobject:
out_insp_file = osp.join(
args.output_dir, "SegmentationObject", base + ".png"
)
if not args.nonpy:
out_ins_file = osp.join(
args.output_dir, "SegmentationObjectNpy", base + ".npy"
)
if not args.noviz:
out_insv_file = osp.join(
args.output_dir,
"SegmentationObjectVisualization",
base + ".jpg",
)
img = labelme.utils.img_data_to_arr(label_file.imageData)
imgviz.io.imsave(out_img_file, img)
cls, ins = labelme.utils.shapes_to_label(
img_shape=img.shape,
shapes=label_file.shapes,
label_name_to_value=class_name_to_id,
)
ins[cls == -1] = 0 # ignore it.
# class label
labelme.utils.lblsave(out_clsp_file, cls)
if not args.nonpy:
np.save(out_cls_file, cls)
if not args.noviz:
clsv = imgviz.label2rgb(
cls,
imgviz.rgb2gray(img),
label_names=class_names,
font_size=15,
loc="rb",
)
imgviz.io.imsave(out_clsv_file, clsv)
if not args.noobject:
# instance label
labelme.utils.lblsave(out_insp_file, ins)
if not args.nonpy:
np.save(out_ins_file, ins)
if not args.noviz:
instance_ids = np.unique(ins)
instance_names = [str(i) for i in range(max(instance_ids) + 1)]
insv = imgviz.label2rgb(
ins,
imgviz.rgb2gray(img),
label_names=instance_names,
font_size=15,
loc="rb",
)
imgviz.io.imsave(out_insv_file, insv)
if __name__ == "__main__":
main()
更改版,如果不想在终端使用命令行的形式 运行,也可以在程序中输入文件夹路径直接运行
python
# 2025.4.12
"""
注意事项:
确保 labels.txt 文件存在且格式正确
输出目录不能预先存在(脚本会自动创建)
路径中的反斜杠建议使用原始字符串(字符串前加r)
如果出现权限问题,请以管理员身份运行
"""
# !/usr/bin/env python
from __future__ import print_function
import glob
import os
import os.path as osp
import sys
import imgviz
import numpy as np
import labelme
def main():
# ====================== 需要修改的配置 ======================
# 输入标注目录(包含.json文件)
input_dir = r"你的标注文件目录(包含.json文件)"
# 输出目录(会自动创建)
output_dir = r"输出目录(会自动创建)"
# 标签文件路径
labels_path = r"标签文件路径(例如:labels.txt)"
# 功能开关(True表示启用,False表示禁用)
noobject = False # 是否不生成实例分割
nonpy = False # 是否不生成.npy文件
noviz = False # 是否不生成可视化结果
# noobject = True # 设置为True则不生成实例分割相关文件
# nonpy = True # 设置为True则不生成.npy文件
# noviz = True # 设置为True则不生成可视化图片
# ===========================================================
# 检查输出目录
if osp.exists(output_dir):
print("Output directory already exists:", output_dir)
sys.exit(1)
os.makedirs(output_dir)
# 创建子目录
os.makedirs(osp.join(output_dir, "JPEGImages"))
os.makedirs(osp.join(output_dir, "SegmentationClass"))
if not nonpy:
os.makedirs(osp.join(output_dir, "SegmentationClassNpy"))
if not noviz:
os.makedirs(osp.join(output_dir, "SegmentationClassVisualization"))
if not noobject:
os.makedirs(osp.join(output_dir, "SegmentationObject"))
if not nonpy:
os.makedirs(osp.join(output_dir, "SegmentationObjectNpy"))
if not noviz:
os.makedirs(osp.join(output_dir, "SegmentationObjectVisualization"))
print("Creating dataset:", output_dir)
# 读取标签
with open(labels_path) as f:
labels = [label.strip() for label in f if label]
# 处理类别映射
class_names = []
class_name_to_id = {}
for i, label in enumerate(labels):
class_id = i - 1 # starts with -1
class_name = label.strip()
class_name_to_id[class_name] = class_id
if class_id == -1:
assert class_name == "__ignore__"
continue
elif class_id == 0:
assert class_name == "_background_"
class_names.append(class_name)
class_names = tuple(class_names)
print("class_names:", class_names)
# 保存类别名称
out_class_names_file = osp.join(output_dir, "class_names.txt")
with open(out_class_names_file, "w") as f:
f.write("\n".join(class_names))
print("Saved class_names:", out_class_names_file)
# 处理每个标注文件
for filename in sorted(glob.glob(osp.join(input_dir, "*.json"))):
print("Generating dataset from:", filename)
label_file = labelme.LabelFile(filename=filename)
base = osp.splitext(osp.basename(filename))[0]
out_img_file = osp.join(output_dir, "JPEGImages", base + ".jpg")
out_clsp_file = osp.join(output_dir, "SegmentationClass", base + ".png")
# 生成类别标签
img = labelme.utils.img_data_to_arr(label_file.imageData)
imgviz.io.imsave(out_img_file, img)
cls, ins = labelme.utils.shapes_to_label(
img_shape=img.shape,
shapes=label_file.shapes,
label_name_to_value=class_name_to_id,
)
ins[cls == -1] = 0 # 忽略被标记的区域
# 保存类别分割结果
labelme.utils.lblsave(out_clsp_file, cls)
if not nonpy:
np.save(osp.join(output_dir, "SegmentationClassNpy", base + ".npy"), cls)
if not noviz:
clsv = imgviz.label2rgb(
cls,
imgviz.rgb2gray(img),
label_names=class_names,
font_size=15,
loc="rb",
)
imgviz.io.imsave(
osp.join(output_dir, "SegmentationClassVisualization", base + ".jpg"),
clsv,
)
# 生成实例分割结果
if not noobject:
out_insp_file = osp.join(output_dir, "SegmentationObject", base + ".png")
labelme.utils.lblsave(out_insp_file, ins)
if not nonpy:
np.save(
osp.join(output_dir, "SegmentationObjectNpy", base + ".npy"), ins
)
if not noviz:
instance_ids = np.unique(ins)
instance_names = [str(i) for i in range(max(instance_ids) + 1)]
insv = imgviz.label2rgb(
ins,
imgviz.rgb2gray(img),
label_names=instance_names,
font_size=15,
loc="rb",
)
imgviz.io.imsave(
osp.join(
output_dir, "SegmentationObjectVisualization", base + ".jpg"
),
insv,
)
if __name__ == "__main__":
main()
data_dataset_voc文件夹内容如下:
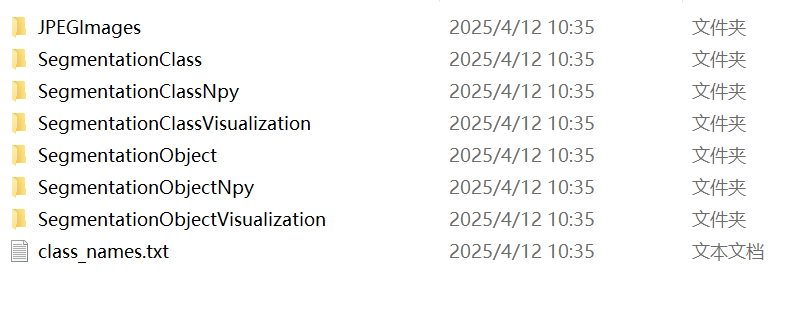
data_dataset_voc\JPEGImages(存放jpg图片)
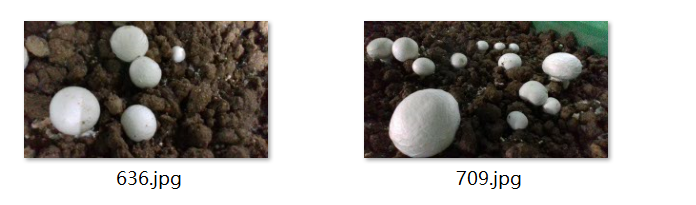
data_dataset_voc\SegmentationClass (存放语义分割标签)
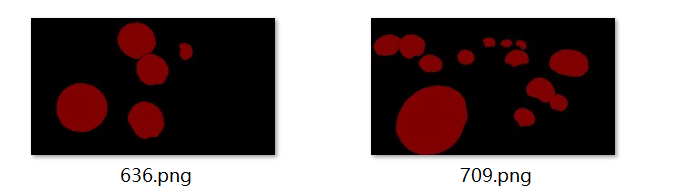
data_dataset_voc\SegmentationClassNpy (存放语义分割标签的npy格式文件, 好像训练时没有用到)

data_dataset_voc\SegmentationClassVisualization (语义分割标签-可视化)
data_dataset_voc\SegmentationObject (实例分割标签)
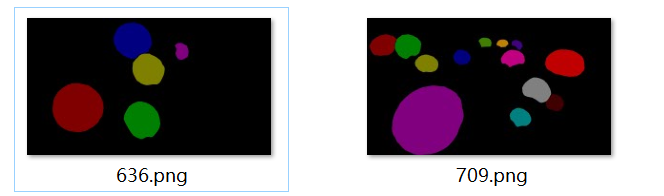
data_dataset_voc\SegmentationObjectNpy (存放实例分割标签的npy格式文件)

data_dataset_voc\SegmentationObjectVisualization (实例分割标签-可视化)
class_names.txt
内容
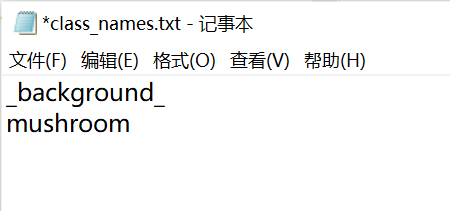
至此,labelme2voc.py 内容结束,但是在使用相关实例分割网络训练(mask-rcnn)的过程中,发现还需要xml文件,需要将labelme_json标签转换为xml标签,这个后续在其他文章再说。
labelme2coco.py使用
与上述一样,
在数据集文件夹中打开终端输入命令行参数
python
python labelme2coco.py data_annotated data_dataset_coco --labels labels.txt
或者像上述更改版代码那样,进行修改,直接输入路径值
data_dataset_coco文件夹内容如下:
python
data_dataset_coco
|__
|------JPEGImages 图片.jpg
|------Visualization 可视化实例分割标签 .png
|------annotations.json json标签信息 (所有图片)

data_dataset_coco\JPEGImages
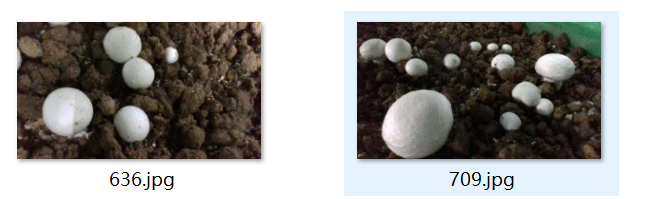
data_dataset_coco\Visualization
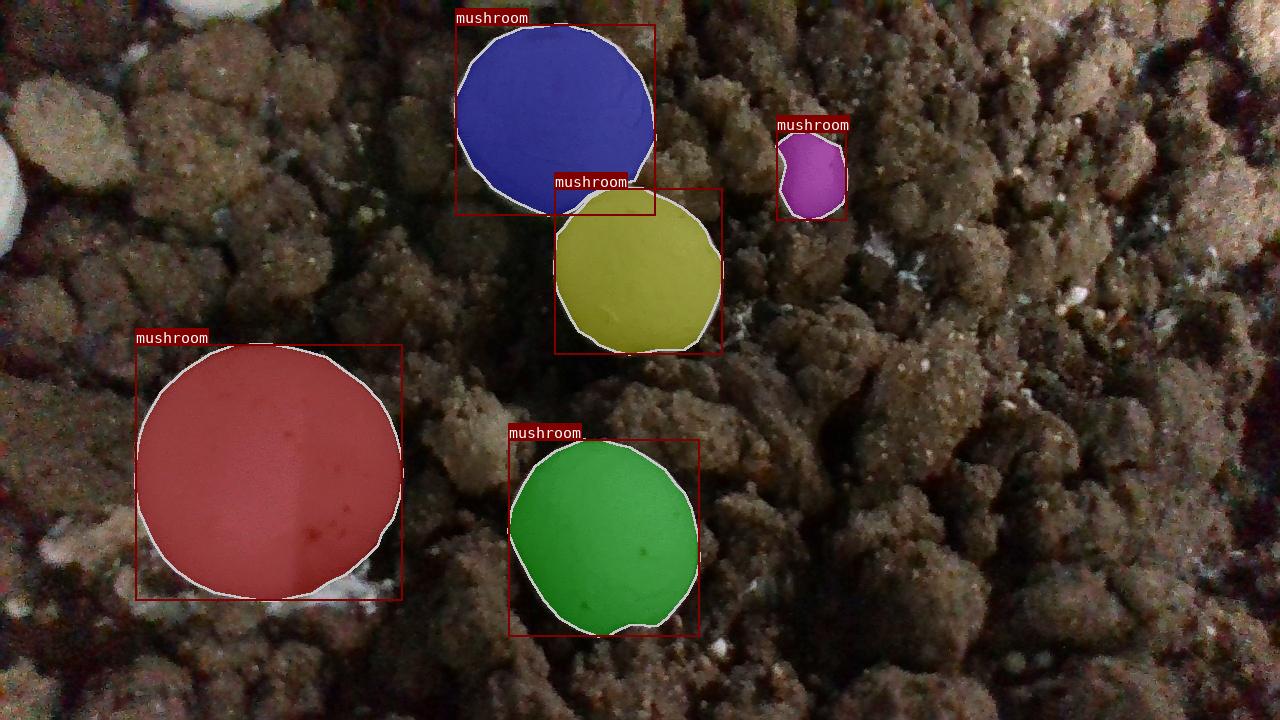
data_dataset_coco\annotations.json
内容太长,就不展示了