直接用的githuab的源码,git clone 后output才有文件
直接gitclone他的源码用Vscode看
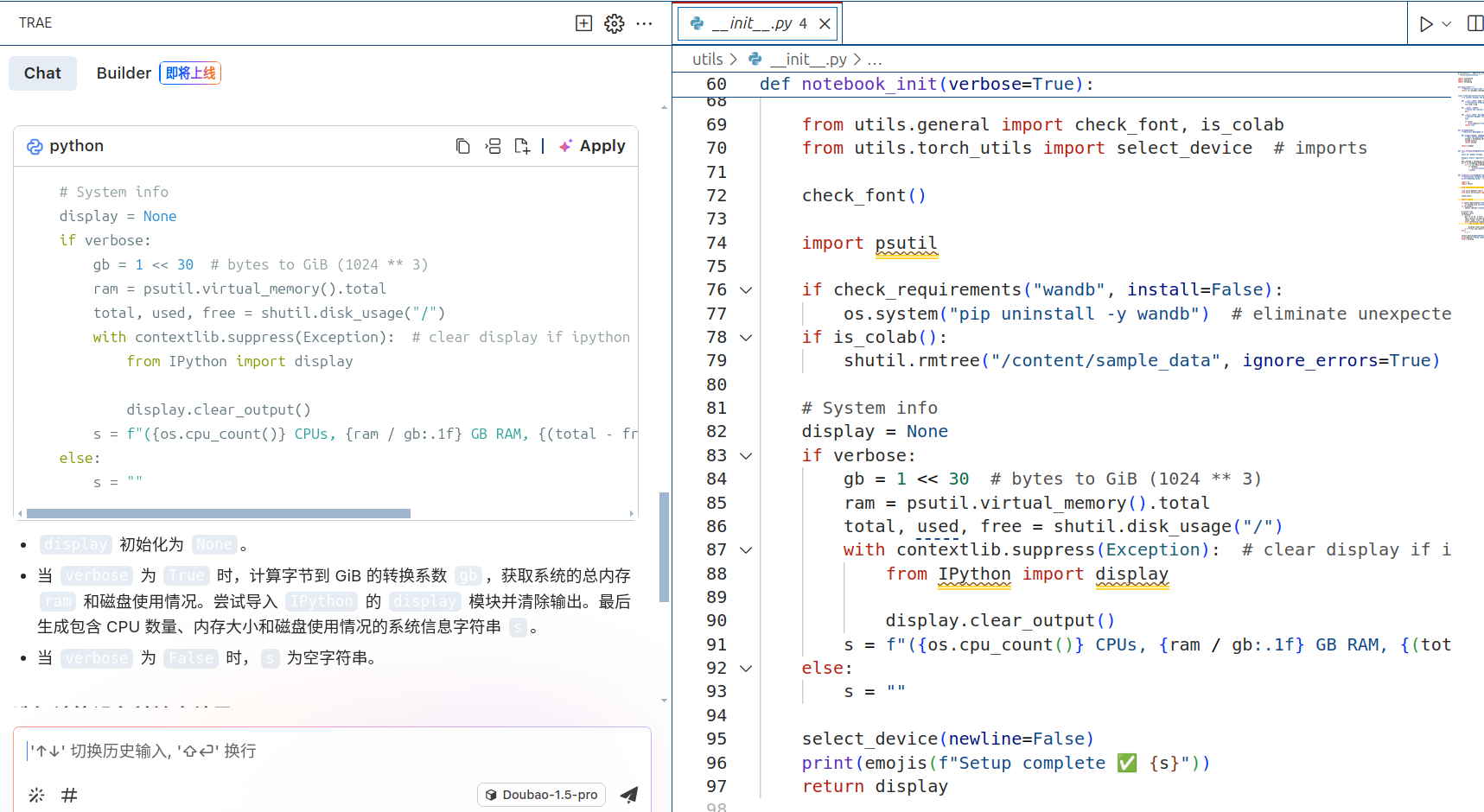
好久没见过16g了
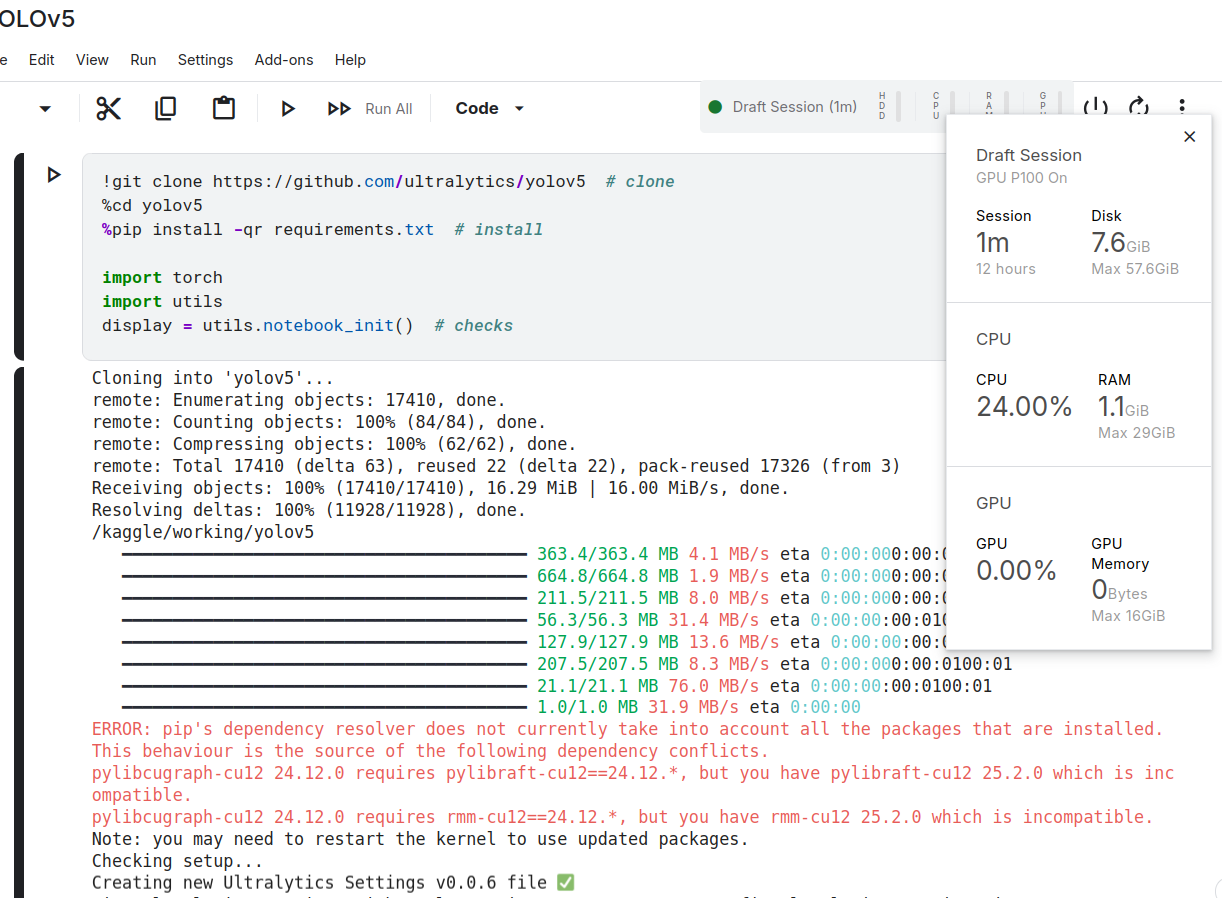
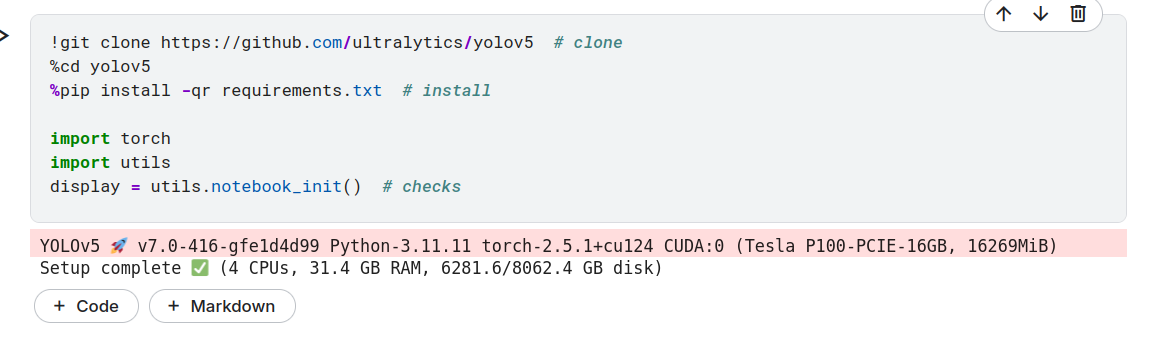
怎么这么便宜
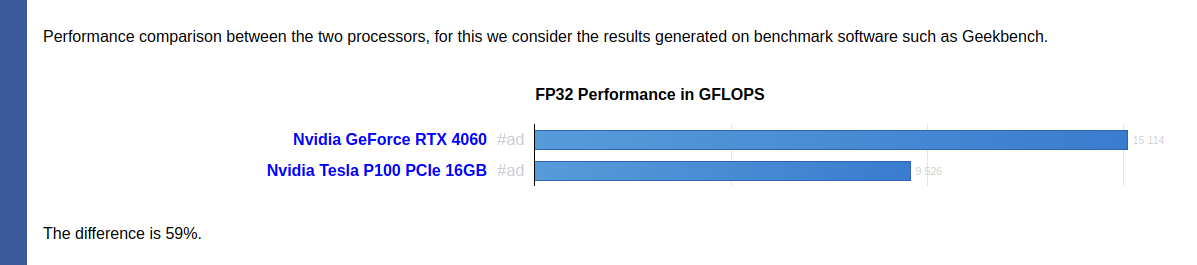
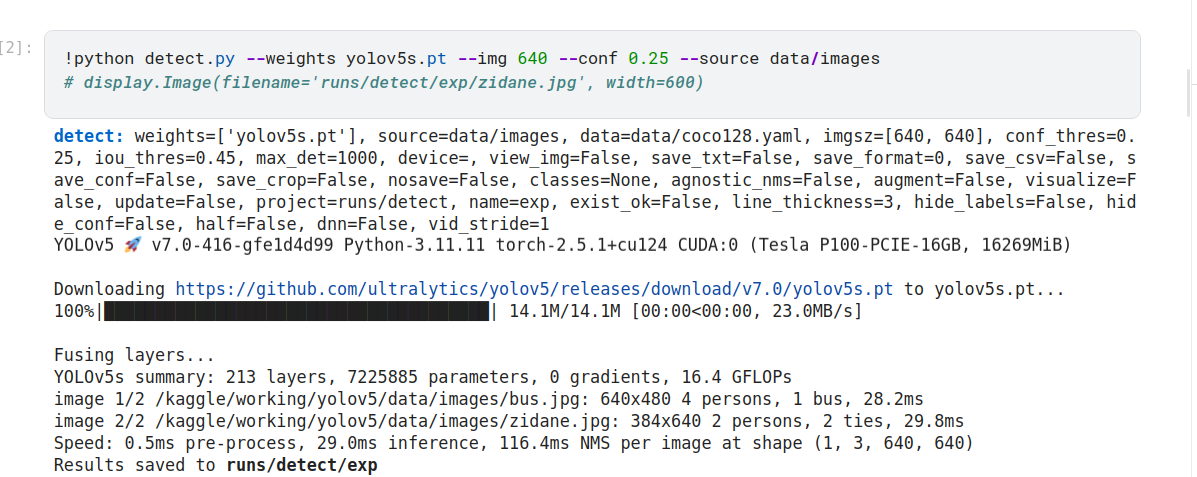
好的,从您提供的信息来看,您正在使用 YOLOv5 进行目标检测任务。以下是运行结果的详细解读:
运行配置
模型权重: 使用了预训练的 yolov5s.pt 模型(小型版本)。
数据源: 图片路径为 data/images。
数据集配置文件: data/coco128.yaml。
输入图片尺寸: [640, 640](模型会将图片缩放到此尺寸进行推理)。
置信度阈值 (conf_thres): 0.25(仅保留置信度高于此阈值的检测结果)。
IoU 阈值 (iou_thres): 0.45(用于非极大值抑制 NMS,过滤重叠框)。
最大检测目标数 (max_det): 1000。
设备: 默认使用 GPU(CUDA:0,Tesla P100-PCIE-16GB)。
保存选项:
未启用可视化窗口 (view_img=False)。
未保存识别结果为文本文件 (save_txt=False)。
未保存置信度分数 (save_conf=False)。
未保存裁剪的目标图片 (save_crop=False)。
其他设置:
结果保存路径为 runs/detect/exp。
线条粗细为 3 (line_thickness=3)。
未隐藏标签和置信度 (hide_labels=False, hide_conf=False)。
运行结果
模型加载与优化:
下载并加载了 yolov5s.pt 模型权重。
融合了部分层以优化推理速度。
模型总结:
层数:213
参数量:7,225,885
FLOPs:16.4 GFLOPs
图片检测:
第一张图片:
路径: /kaggle/working/yolov5/data/images/bus.jpg
尺寸: 640x480
检测结果:
4 个人 (person)
1 辆公交车 (bus)
推理时间: 28.2ms
第二张图片:
路径: /kaggle/working/yolov5/data/images/zidane.jpg
尺寸: 384x640
检测结果:
2 个人 (person)
2 条领带 (tie)
推理时间: 29.8ms
性能指标:
预处理时间: 0.5ms
推理时间: 29.0ms
NMS 时间: 116.4ms
总体来看,模型在单张图片上的平均推理速度较快。
结果保存:
检测结果已保存到 runs/detect/exp 文件夹中。
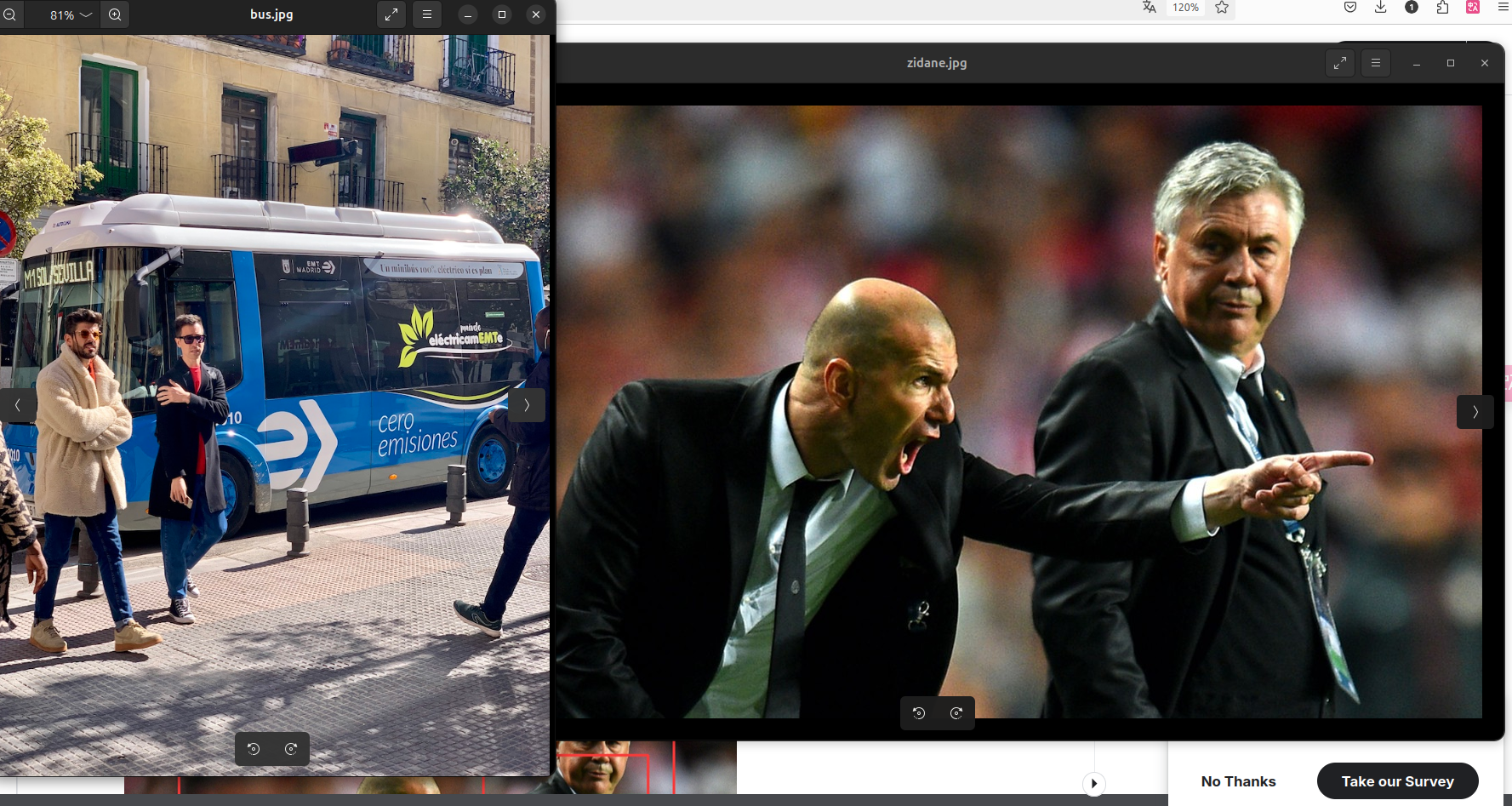
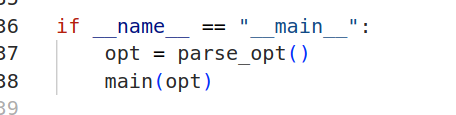
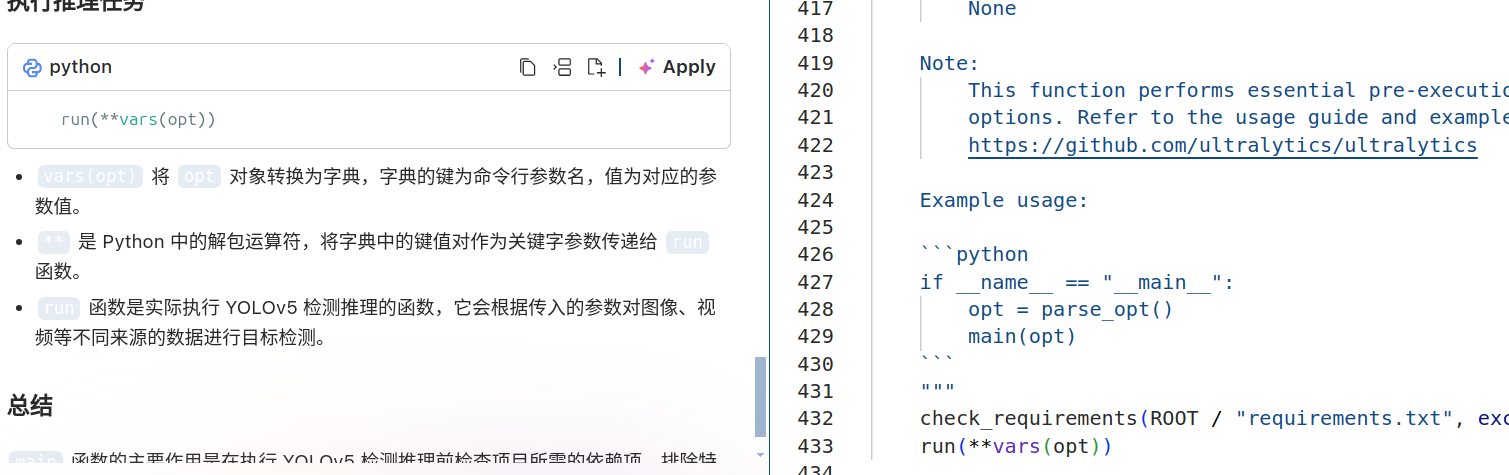
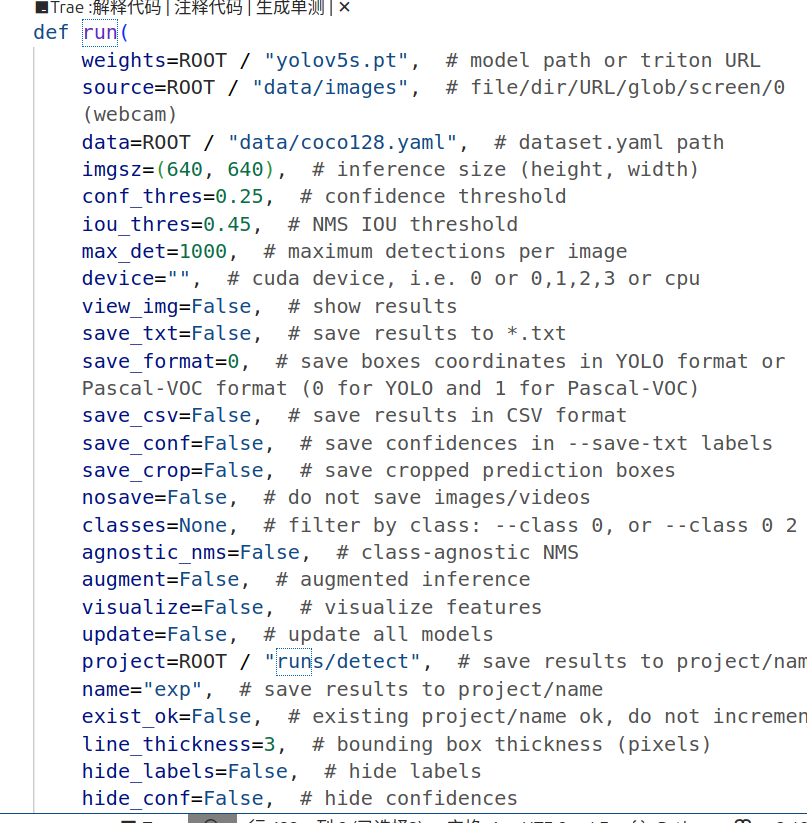
函数输入有一页
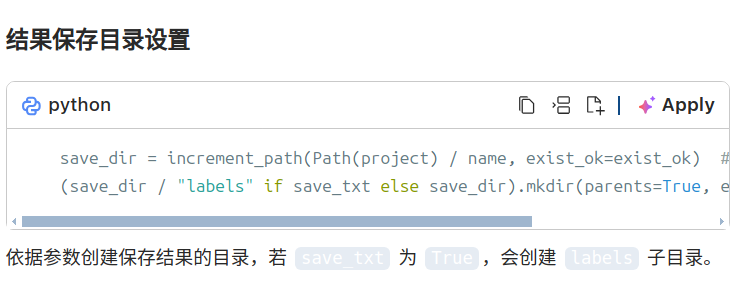
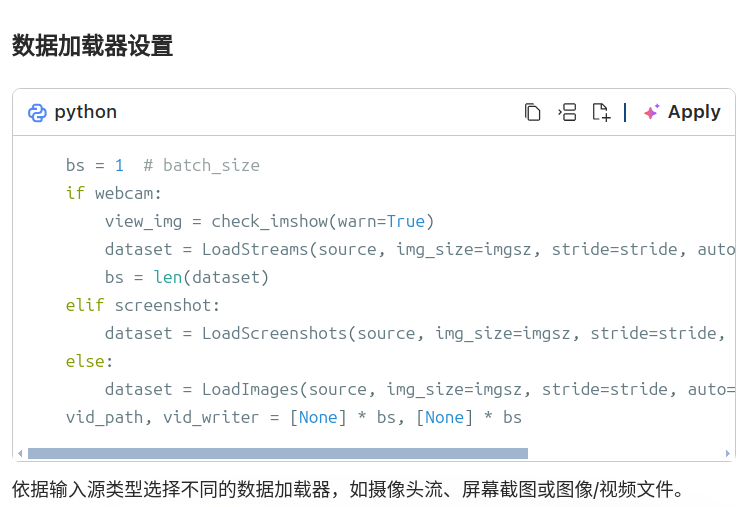
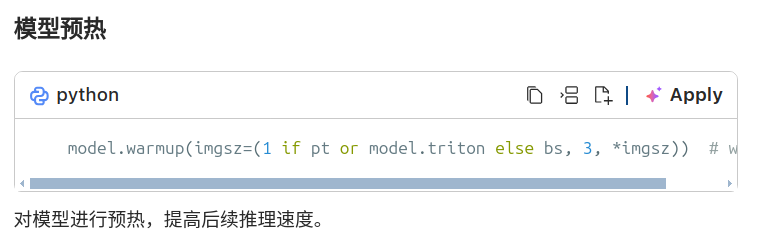
推理循环
python
seen, windows, dt = 0, [], (Profile(device=device), Profile(device=device), Profile(device=device))
for path, im, im0s, vid_cap, s in dataset:
# 预处理
with dt[0]:
im = torch.from_numpy(im).to(model.device)
im = im.half() if model.fp16 else im.float() # uint8 to fp16/32
im /= 255 # 0 - 255 to 0.0 - 1.0
if len(im.shape) == 3:
im = im[None] # expand for batch dim
if model.xml and im.shape[0] > 1:
ims = torch.chunk(im, im.shape[0], 0)
# 推理
with dt[1]:
visualize = increment_path(save_dir / Path(path).stem, mkdir=True) if visualize else False
if model.xml and im.shape[0] > 1:
pred = None
for image in ims:
if pred is None:
pred = model(image, augment=augment, visualize=visualize).unsqueeze(0)
else:
pred = torch.cat((pred, model(image, augment=augment, visualize=visualize).unsqueeze(0)), dim=0)
pred = [pred, None]
else:
pred = model(im, augment=augment, visualize=visualize)
# 非极大值抑制
with dt[2]:
pred = non_max_suppression(pred, conf_thres, iou_thres, classes, agnostic_nms, max_det=max_det)
对每个输入数据进行预处理、推理和非极大值抑制操作,记录各阶段耗时。
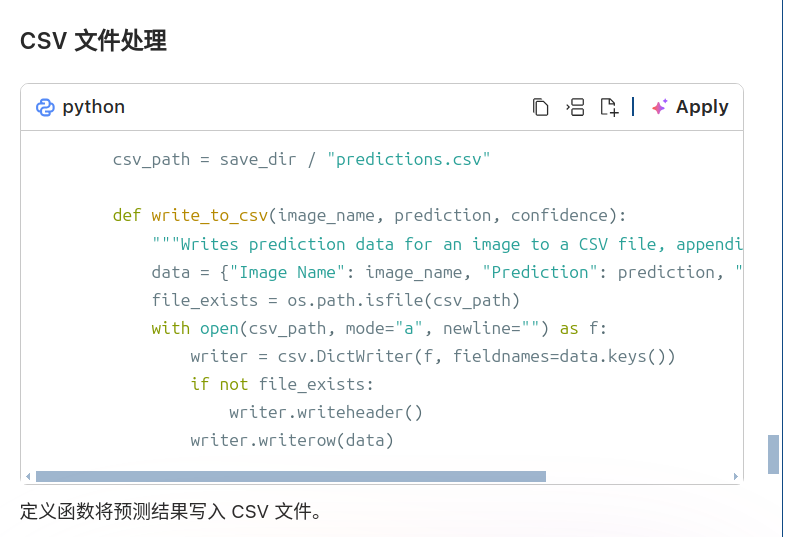
python
# Process predictions
for i, det in enumerate(pred): # per image
seen += 1
if webcam: # batch_size >= 1
p, im0, frame = path[i], im0s[i].copy(), dataset.count
s += f"{i}: "
else:
p, im0, frame = path, im0s.copy(), getattr(dataset, "frame", 0)
p = Path(p) # to Path
save_path = str(save_dir / p.name) # im.jpg
txt_path = str(save_dir / "labels" / p.stem) + ("" if dataset.mode == "image" else f"_{frame}") # im.txt
s += "{:g}x{:g} ".format(*im.shape[2:]) # print string
gn = torch.tensor(im0.shape)[[1, 0, 1, 0]] # normalization gain whwh
imc = im0.copy() if save_crop else im0 # for save_crop
annotator = Annotator(im0, line_width=line_thickness, example=str(names))
if len(det):
# Rescale boxes from img_size to im0 size
det[:, :4] = scale_boxes(im.shape[2:], det[:, :4], im0.shape).round()
# Print results
for c in det[:, 5].unique():
n = (det[:, 5] == c).sum() # detections per class
s += f"{n} {names[int(c)]}{'s' * (n > 1)}, " # add to string
# Write results
for *xyxy, conf, cls in reversed(det):
c = int(cls) # integer class
label = names[c] if hide_conf else f"{names[c]}"
confidence = float(conf)
confidence_str = f"{confidence:.2f}"
if save_csv:
write_to_csv(p.name, label, confidence_str)
if save_txt: # Write to file
if save_format == 0:
coords = (
(xyxy2xywh(torch.tensor(xyxy).view(1, 4)) / gn).view(-1).tolist()
) # normalized xywh
else:
coords = (torch.tensor(xyxy).view(1, 4) / gn).view(-1).tolist() # xyxy
line = (cls, *coords, conf) if save_conf else (cls, *coords) # label format
with open(f"{txt_path}.txt", "a") as f:
f.write(("%g " * len(line)).rstrip() % line + "\n")
if save_img or save_crop or view_img: # Add bbox to image
c = int(cls) # integer class
label = None if hide_labels else (names[c] if hide_conf else f"{names[c]} {conf:.2f}")
annotator.box_label(xyxy, label, color=colors(c, True))
if save_crop:
save_one_box(xyxy, imc, file=save_dir / "crops" / names[c] / f"{p.stem}.jpg", BGR=True)
# Stream results
im0 = annotator.result()
if view_img:
if platform.system() == "Linux" and p not in windows:
windows.append(p)
cv2.namedWindow(str(p), cv2.WINDOW_NORMAL | cv2.WINDOW_KEEPRATIO) # allow window resize (Linux)
cv2.resizeWindow(str(p), im0.shape[1], im0.shape[0])
cv2.imshow(str(p), im0)
cv2.waitKey(1) # 1 millisecond
# Save results (image with detections)
if save_img:
if dataset.mode == "image":
cv2.imwrite(save_path, im0)
else: # 'video' or 'stream'
if vid_path[i] != save_path: # new video
vid_path[i] = save_path
if isinstance(vid_writer[i], cv2.VideoWriter):
vid_writer[i].release() # release previous video writer
if vid_cap: # video
fps = vid_cap.get(cv2.CAP_PROP_FPS)
w = int(vid_cap.get(cv2.CAP_PROP_FRAME_WIDTH))
h = int(vid_cap.get(cv2.CAP_PROP_FRAME_HEIGHT))
else: # stream
fps, w, h = 30, im0.shape[1], im0.shape[0]
save_path = str(Path(save_path).with_suffix(".mp4")) # force *.mp4 suffix on results videos
vid_writer[i] = cv2.VideoWriter(save_path, cv2.VideoWriter_fourcc(*"mp4v"), fps, (w, h))
vid_writer[i].write(im0)
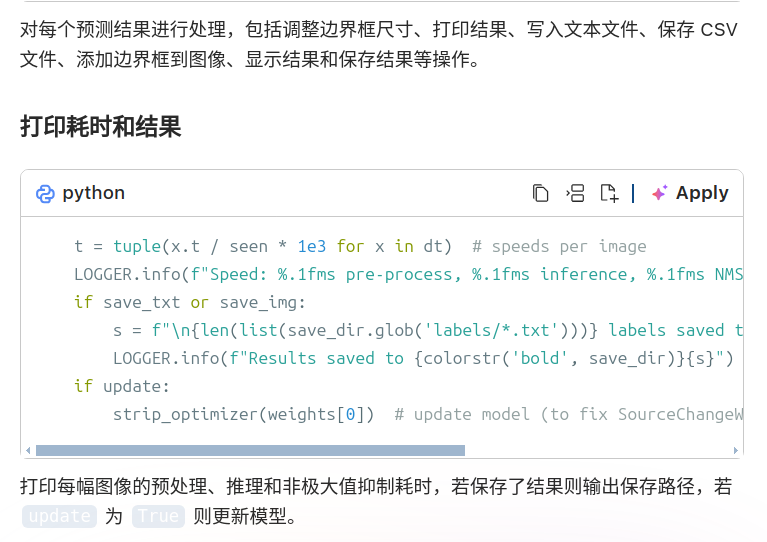
明天看训练部分